5 priorities for CIOs in 2021

2020 was undeniably the year of digital. Organizations that had never dreamed
of digitizing their operations were forced to completely transform their
approach. And automation was a big part of that shift, enabling companies to
mitigate person-to-person contact and optimize costs while ensuring
uninterrupted operations. In 2021, hyperautomation seems to be the name of the
game. According to Gartner, “Hyperautomation is the idea that anything that
can be automated in an organization should be automated.” Especially for
companies that implemented point solutions to adapt and survive in 2020, now
is the time to intelligently automate repeatable, end-to-end processes by
leveraging bots. With hyperautomation, CIOs can implement new-age technologies
such as business process management, robotic process automation, and
artificial intelligence (AI) to drive end-to-end automation and deliver
superior customer experience. A steadily growing customer experience trend is
to “be where the customer is.” Over the past decade, forward-thinking
organizations have been working to engage customers according to their
preferences of when, where, and how.
Reducing the Risk of Third-Party SaaS Apps to Your Organization
It's vital first to understand the risk of third-party applications. In an ideal
world, each potential application or extension is thoroughly evaluated before
it's introduced into your environment. However, with most employees still
working remotely and you and your administrators having limited control over
their online activity, that may not be a reality today. However, reducing the
risk of potential data loss even after an app has been installed is still
critically important. The reality is that in most cases, the threats from
third-party applications come from two different perspectives. First, the
third-party application may try to leak your data or contain malicious code. And
second, it may be a legitimate app but be poorly written (causing security
gaps). Poorly coded applications can introduce vulnerabilities that lead to data
compromise. While Google does have a screening process for developers (as
its disclaimer mentions), users are solely responsible for compromised or lost
data (it sort of tries to protect you … sort of). Businesses must take hard and
fast ownership of screening third-party apps for security best practices. What
are the best practices that Google outlines for third-party application
security?
3 Trends That Will Define Digital Services in 2021

Cloud native environments and applications such as mobile, serverless and
Kubernetes are constantly changing, and traditional approaches to app
security can’t keep up. Despite having many tools to manage threats,
organizations still have blind spots and uncertainty about exposures and
their impact on apps. At the same time, siloed security practices are
bogging down teams in manual processes, imprecise analyses, fixing things
that don’t need fixing, and missing the things that should be fixed. This is
building more pressure on developers to address vulnerabilities in
pre-production. In 2021, we’ll increasingly see organizations adopt
DevSecOps processes — integrating security practices into their DevOps
workflows. That integration, within a holistic observability platform that
helps manage dynamic, multicloud environments, will deliver continuous,
automatic runtime analysis so that teams can focus on what matters,
understand vulnerabilities in context, and resolve them proactively. All
this amounts to faster, more secure release cycles, greater confidence in
the security of production as well as pre-production environments, and
renewed confidence in the idea that securing applications doesn’t have to
come at the expense of innovation and faster release cycles.
Meeting the Challenges of Disrupted Operations: Sustained Adaptability for Organizational Resilience
While one might argue that an Agile approach to software development is the
same as resilience - since at its core it is about iteration and adaptation.
However, Agile methods do not guarantee resilience or adaptive capacity by
themselves. Instead, a key characteristic of resilience lies in an
organization’s capacity to put the ability to adapt into play across ongoing
activities in real time; in other words, to engineer resilience into their
system by way of adaptive processes, practices, coordinative networks in
service of supporting people in making necessary adaptations. Adaptability, as
a function of day-to-day work, means to revise assessments, replan,
dynamically reconfigure activities, reallocate & redeploy resources as the
conditions and demands change. Each of these "re" activities belies an
orientation towards change as a continuous state. This seems self-evident -
the world is always changing and the faster the speed and greater the scale -
the more likely changes are going to impact your plans and activities.
However, many organizations do not recognize the pace of change until it’s too
late. Late stage changes are more costly - both financially at the macro level
and attentionally for individuals at a micro-level.
You don’t code? Do machine learning straight from Microsoft Excel

To most people, MS Excel is a spreadsheet application that stores data in
tabular format and performs very basic mathematical operations. But in
reality, Excel is a powerful computation tool that can solve complicated
problems. Excel also has many features that allow you to create machine
learning models directly into your workbooks. While I’ve been using Excel’s
mathematical tools for years, I didn’t come to appreciate its use for
learning and applying data science and machine learning until I picked up
Learn Data Mining Through Excel: A Step-by-Step Approach for Understanding
Machine Learning Methods by Hong Zhou. Learn Data Mining Through Excel takes
you through the basics of machine learning step by step and shows how you
can implement many algorithms using basic Excel functions and a few of the
application’s advanced tools. While Excel will in no way replace Python
machine learning, it is a great window to learn the basics of AI and solve
many basic problems without writing a line of code. ... Beyond regression
models, you can use Excel for other machine learning algorithms. Learn Data
Mining Through Excel provides a rich roster of supervised and unsupervised
machine learning algorithms, including k-means clustering, k-nearest
neighbor, naive Bayes classification, and decision trees.
Four ways to improve the relationship between security and IT
For too long in too many organizations, IT and security have viewed themselves
as two different disciplines with fundamentally different missions that have
been forced to work together. In companies where this tension exists, the
disconnect stems from the CIO’s focus on delivery and availability of digital
services for competitive advantage and customer satisfaction – as quickly as
possible – while the CISO is devoted to finding security and privacy risks in
those same services. The IT pros tend to think of the security teams as the
“Department of No.” Security pros view the IT teams as always putting speed
ahead of safety. Adding to the strain, CISOs are catching up to CIOs in
carving out an enhanced role as business strategists, not merely technology
specialists. The CIO’s main role was once to deliver IT reliably and
cost-effectively across the organization, but while optimizing infrastructure
remains a big part of the job, today’s CIO is expected to be a key player in
leading digital transformation initiatives and driving revenue-generating
innovation. The CISO is rapidly growing into a business leader as well.
Key cyber security trends to look out for in 2021

Working from home means many of us are now living online for between 10 and
12 hours a day, getting very little respite with no gaps between meetings
and no longer having a commute. We’ll see more human errors causing cyber
security issues purely driven by employee fatigue or complacency. This means
businesses need to think about a whole new level of IT security education
programme. This includes ensuring people step away and take a break, with
training to recognise signs of fatigue. When you make a cyber security
mistake at the office, it’s easy to go down and speak to a friendly member
of your IT security team. This is so much harder to do at home now without
direct access to your usual go-to person, and it requires far more
confidence to confess. Businesses need to take this human error factor into
consideration and ensure consistent edge security, no matter what the
connection. You can no longer just assume that because core business apps
are routing back through the corporate VPN that all is as it should be. ...
It took most companies years to get their personally identifiable
information (PII) ready for GDPR when it came into force in 2018. With the
urgent shift to cloud and collaboration tools driven by the lockdown this
year, GDPR compliance was challenged.
Ransomware 2020: A Year of Many Changes
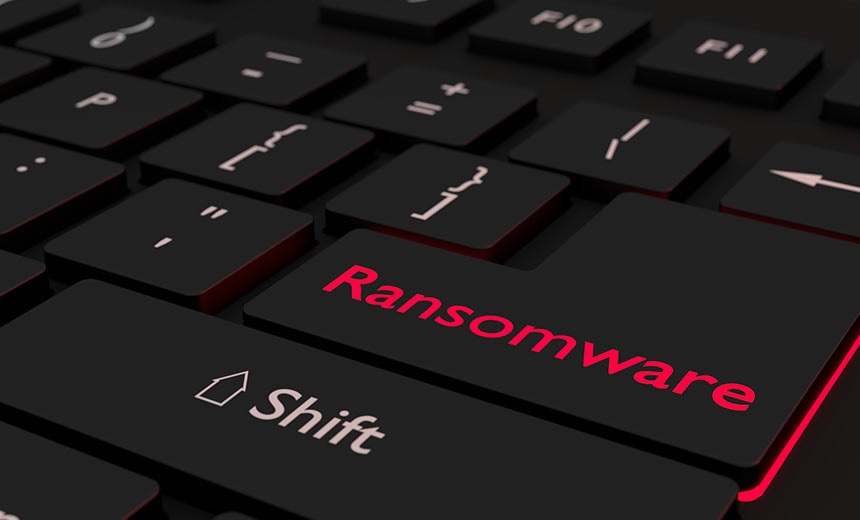
The tactic of adding a layer of data extraction and then a threat to make
the stolen information public if the victim refuses to pay the ransom became
the go-to tactic for many ransomware groups in 2020. This technique first
appeared in late 2019 when the Maze ransomware gang attacked Allied
Universal, a California-based security services firm, Malwarebytes reported.
"Advanced tools enable stealthier attacks, allowing ransomware operators to
target sensitive data before they are detected, and encrypt systems.
So-called 'double extortion' ransomware attacks are now standard operating
procedures - Canon, LG, Xerox and Ubisoft are just some examples of
organizations falling victim to such attacks," Cummings says. This exploded
this year as both Maze and other gangs saw extortion as a way to strong-arm
even those who prepared for a ransomware attack by properly backing up their
files but could not risk the data being exposed, says Stefano De Blasi,
threat researcher at Digital Shadows. "This 'monkey see, monkey do' approach
has been extremely common in 2020, with threat actors constantly seeking to
expand their offensive toolkit by mimicking successful techniques employed
by other criminal groups," De Blasi says.
Finding the balance between edge AI vs. cloud AI
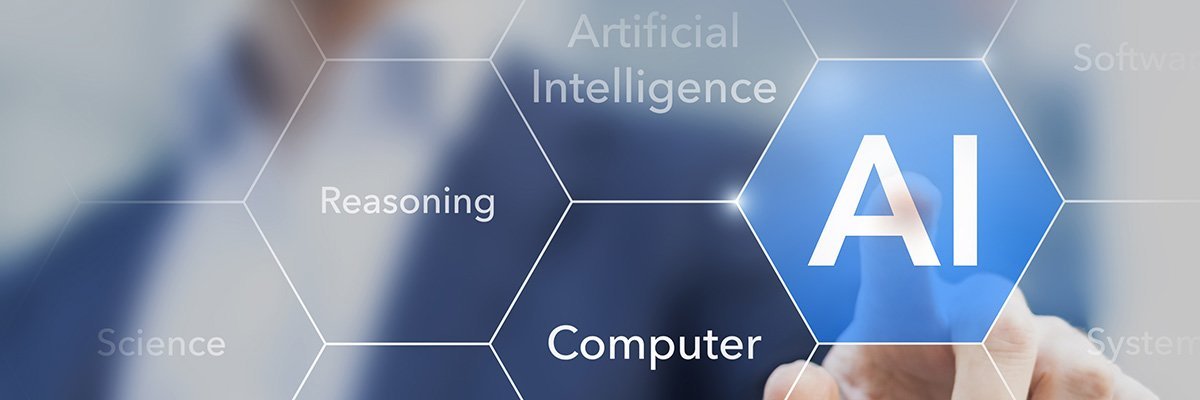
Most experts see edge and cloud approaches as complementary parts of a
larger strategy. Nebolsky said that cloud AI is more amenable to batch
learning techniques that can process large data sets to build smarter
algorithms to gain maximum accuracy quickly and at scale. Edge AI can
execute those models, and cloud services can learn from the performance of
these models and apply to the base data to create a continual learning loop.
Fyusion's Miller recommends striking the right balance -- if you commit
entirely to edge AI, you've lost the ability to continuously improve your
model. Without new data streams coming in, you have nothing to leverage.
However if you commit entirely to cloud AI, you risk compromising the
quality of your data -- due to the tradeoffs necessary to make it
uploadable, and lack of feedback to guide the user to capture better data --
or the quantity of data. "Edge AI complements cloud AI in providing access
to immediate decisions when they are needed and utilizing the cloud for
deeper insights or ones that require a broader or more longitudinal data set
to drive a solution," Tracy Ring, managing director at Deloitte said. For
example, in a connected vehicle, sensors on the car provide a stream of
real-time data that is processed constantly and can make decisions, like
applying the brakes or adjusting the steering wheel.
Experiences from Testing Stochastic Data Science Models
We can ensure the quality of testing by: Making sure we have enough
information about a new client requirement and the team understands
it; Validating results including results which are stochastic; Making
sure the results make sense; Making sure the product does not
break; Making sure no repetitive bugs are found, in other words, a bug
has been fixed properly; Making sure to pair up with developers and data
scientists to understand a feature better; If you have a front end
dashboard showcasing your results, making sure the details all make sense and
have done some accessibility testing on it too; and Testing the
performance of the runs as well, if they take longer due to certain
configurations or not. ... As mentioned above, I learned that having
thresholds was a good option for a model that delivers results to optimise a
client’s requests. If a model is stochastic then that means certain parts will
have results which may look wrong, but they are actually not. For instance, 5
+ 3 = 8 for all of us but the model may output 5.0003, which is not wrong, but
with a stochastic model what was useful was adding thresholds of what we could
and couldn’t accept. I would definitely recommend trying to add
thresholds;
Quote for the day:
“Failure is the opportunity to begin again more intelligently.” -- Henry Ford