The road to successful change is lined with trade-offs
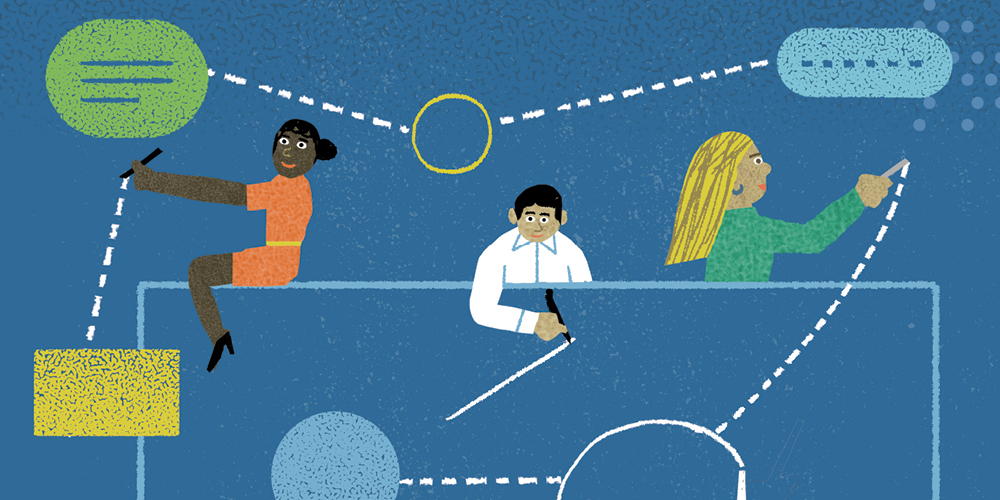
Leaders should borrow an important concept from the project management world:
Go slow to go fast. There is often a rush to dive in at the beginning of a
project, to start getting things done quickly and to feel a sense of
accomplishment. This desire backfires when stakeholders are overlooked, plans
are not validated, and critical conversations are ignored. Instead, project
managers are advised to go slow — to do the work needed up front to develop
momentum and gain speed later in the project. The same idea helps reframe
notions about how to lead organizational change successfully. Instead of doing
the conceptual work quickly and alone, leaders must slow down the initial
planning stages, resist the temptation and endorphin rush of being a “heroic”
leader solving the problem, and engage people in frank conversations about the
trade-offs involved in change. This does not have to take long — even just a
few days or weeks. The key is to build the capacity to think together and to
get underlying assumptions out in the open. Leaders must do more than just get
the conversation started. They also need to keep it going, often in the face
of significant challenges.
AI, ML can bolster cybersecurity, and vice versa, professor says
Machine learning and artificial intelligence for cybersecurity. It's like two
ways we are tackling the problems. For example, machine learning has good
results in machine vision, computer vision, or you can look at the games. For
example, chess was built that was using machine learning and artificial
intelligence. As a result the chess game, played by computer, beat the
smartest human a couple of years ago. And it has been a very promising
application of AI and machine learning. So at the same time, you can look at
how machine learning algorithms are compromised. If you recall, there was a
Tesla car speeding. And I think it slipped by at 55 miles on the road [with a
speed limit] of 35 miles per hour, just because of a smart piece of tape [on a
sign]. In that case we are trying to use the advantage or the benefits
that AI and machine learning offer for securing systems that we are
envisioning in the years to come. But again, at the same time, can we use the
other way around too, right. That's why my research is focusing on AI and
machine learning for cybersecurity. At the same time, cybersecurity for AI
because we want to secure the system that is working for a greater good.
Another example that I could explain is if you are using, let's say a machine
learning algorithm to filter out the applicants from the application pool to
hire somebody.
Low Code: CIOs Talk Challenges and Potential

Former CIO Dave Kieffer says that “cloud ERP can't be customized, but they can
be extended. CRM's can provide full platforms. Extension, not customization,
should be the goal no matter the platform.” However, Sacolick suggests when
“CIO can seamlessly, integrate data and workflow with ERP and CRM, then
customization will be less needed. It can become an architecture decision that
provides a lot more business flexibility. Low Code and No Code apps,
integrations are one approach.” Deb Gildersleeve agrees and says, “a lot of
these legacy systems can’t be customized, and for those that can be, most
organizations don’t want to spend the time, money and resources needed to
customize them. That’s where low code can come in to complement these systems
and work within your existing tech stack.” Francis, meanwhile, suggests,
“there will likely always be need for an amount of high coding. Sacolick says,
“I call highcoding, DIYCoding. But the real challenge is getting app
developers on board with using low code /no code where it makes sense. Many
really love coding, and some lose sight that their role is to provide
solutions and innovations.”
Where are we really with AI and ML? 5 takeaways from the AI Innovators Forum
The reality is AI and ML can’t be applied to every scenario. For example,
one company created a sophisticated ML approach to examining marketing
analytics and performance so AI could predict the most effective marketing
channels, but it required an incredible number of data integrations, and it
wasn’t predictably better at suggesting marketing channels than existing
experts using their expertise. Likewise it is not yet possible to
train enough scenarios of kids running in the street or a car swerving into
your lane for self driving cars to learn and act accordingly. What is the
safe default? Should the car stop when it doesn’t know what to do? Should it
revert to a manual mode? These are just some of the ongoing challenges in
training the ‘last 10%” of AI despite the vast majority of driving decisions
being more efficient and accurate than a human driving on the road
today. Beyond the more obvious self-driving car scenarios, many AI and
ML use cases bring ethical considerations that should be taken seriously.
Like any other technology at scale, there must be frameworks and guardrails
to help understand potential impact, mitigation paths, and when to forgo the
use of these technologies altogether.
Ensuring Data Residency and Portability
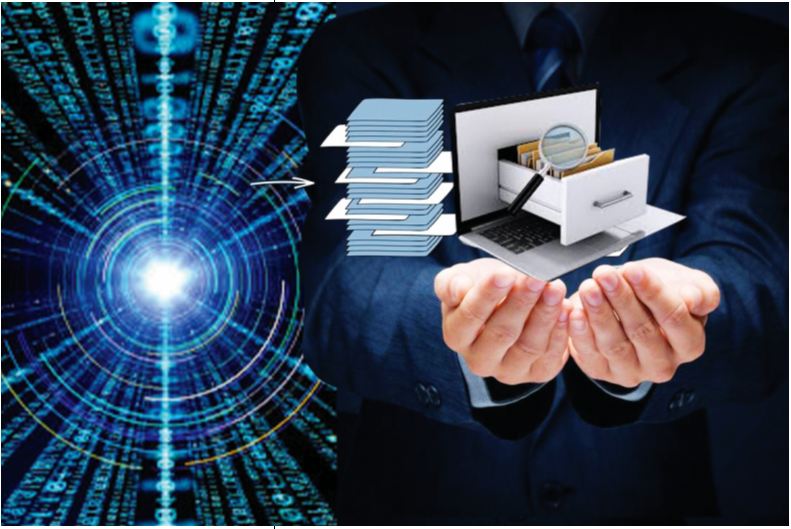
Even if the server is based in California and stores only Indian data, it
does not come within the sovereign jurisdiction of India or Indian courts.
While it may be technically possible to isolate a set of data within the
server that is deemed perverse or critical evidence and electronically
“seal” it, the California company might not be interested in blocking large
space within its server or servers for which it has invested millions, for a
court case in faraway India. This is just one example of the limits of
national laws versus the limitless, borderless movements of data, made
possible by technology. Hence, data localisation is an issue that has been a
hot topic for governments around the world. Alongside comes the issue of
data portability. What does the right to data portability mean? This is a
right that allows anybody who has put a set of data into one service or
site, to obtain it from that service or site and reuse it for their own
purposes across different services. The sense of portability is in the
moving, copying and transferring personal data from one to another service
without compromising on security. This right will also incorporate the right
to have the quality of data undiminished or unchanged. Within this right is
also incorporated the caveat that all such data will have only been gathered
from the user with his or her consent.
Countries that retaliate too much against cyberattacks make things worse for themselves
“If one country becomes more aggressive, then the equilibrium response is
that all countries are going to end up becoming more aggressive,” says
Alexander Wolitzky, an MIT economist who specializes in game theory. “If
after every cyberattack my first instinct is to retaliate against Russia and
China, this gives North Korea and Iran impunity to engage in cyberattacks.”
But Wolitzky and his colleagues do think there is a viable new approach,
involving a more judicious and well-informed use of selective retaliation.
“Imperfect attribution makes deterrence multilateral,” Wolitzky says. “You
have to think about everybody’s incentives together. Focusing your attention
on the most likely culprits could be a big mistake.” The study is a joint
project, in which Sandeep Baliga, the John L. and Helen Kellogg Professor of
Managerial Economics and Decision Sciences at Northwestern University’s
Kellogg School of Management added to the research team by contacting
Wolitzky, whose own work applies game theory to a wide variety of
situations, including war, international affairs, network behavior, labor
relations, and even technology adoption.
Driving autonomous vehicles forward with intelligent infrastructure
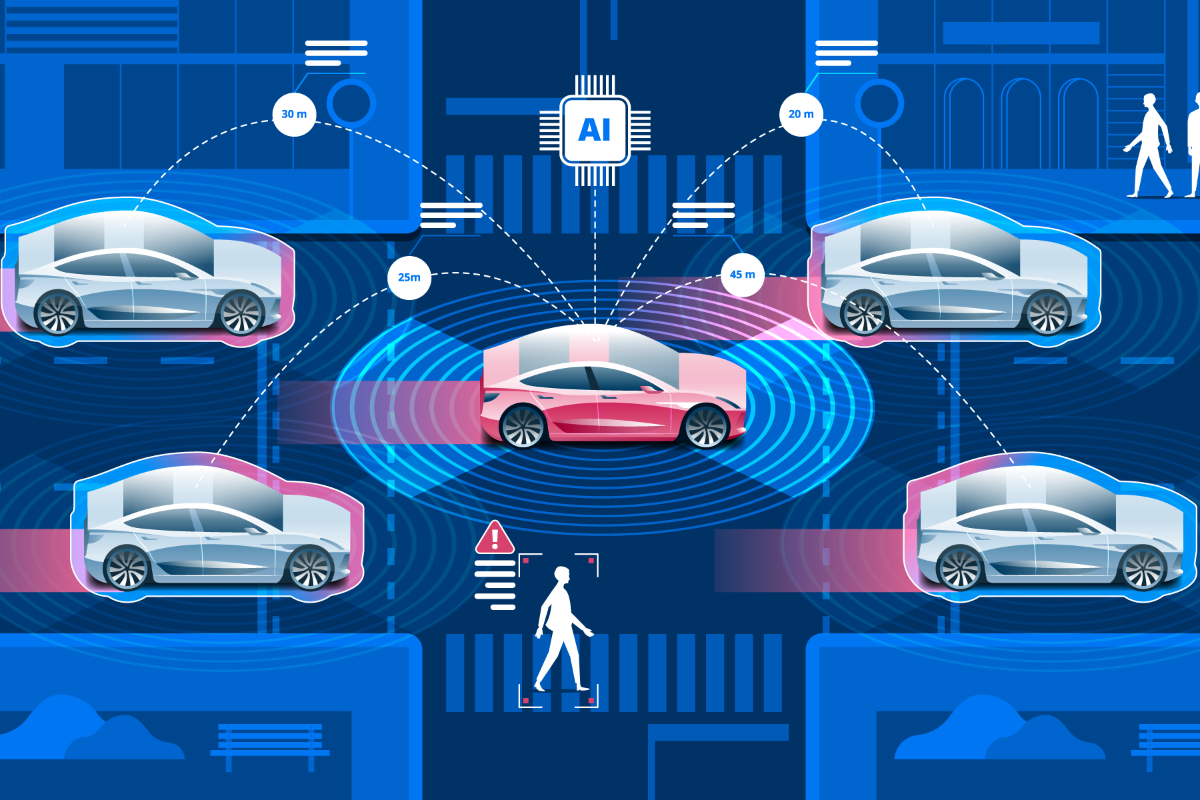
As cars are becoming more autonomous, cities are becoming more intelligent
by using more sensors and instruments. To drive this intelligence forward,
smart city IT infrastructure must be able to capture, store, protect and
analyze data from autonomous vehicles. Similarly, autonomous vehicles could
greatly improve their performance by integrating data from smart cities. In
smart city planning, stakeholders must consider how they will enable the
sharing of data in both directions, to and from autonomous vehicles, and how
that data can be analysed and acted upon in real-time, so traffic keeps
moving and drivers, passengers and pedestrians are kept safe. This means
that a city needs physical infrastructure to handle the growing numbers of
autonomous vehicles that will be on the streets and an IT infrastructure
that can easily manage data storage, performance, security, resilience,
mobilisation and protection from a central management console. For example,
there’s a case to be made that cities should already be building networks of
smart sensors along the roadside. These would have the capability to measure
traffic conditions and potentially even monitor obstacles such as fallen
trees, traffic collisions or black ice.
How teaching 'future resilient' skills can help workers adapt to automation
Automation itself isn’t a problem, but without a reskilling strategy it will
be. Here’s how quality non-degree credentials can help. This fear of
automation is not new. As the late Harvard professor Calestous Juma laid out
in his seminal book Innovation and Its Enemies: Why People Resist New
Technologies, technological progress has always come with some level of
public concern. The bellhops feared automatic elevators and so did bowling
pin resetters. Video did indeed “kill the radio star” and it wasn’t long
before internet media streaming services made video retailers obsolete in
the mid-2000s. This “creative destruction” means that automation-enabling
technologies will destroy jobs, but they will also increase productivity,
lower prices and create new (hopefully better) jobs too. Some have even
advocated that in order to help low-income workers, we should speed up the
automation of low-income jobs. Non-degree credentials can help workers
adapt. Automation can change the world for the better, but only we if
prepare for it. To be sure, non-degree credentials are no silver bullet to
automation displacement. A number of policy recommendations can help our
world transition to new, high-quality jobs.
ML-Powered Digital Twin For Predictive Maintenance — Notes From Tiger Analytics

In the last decade, the Industrial Internet of Things (IIoT) has
revolutionised predictive maintenance. Sensors record operational data in
real-time and transmit it to a cloud database. This dataset then feeds a
digital twin, a computer-generated model that mirrors the physical operation
of each machine. The concept of the digital twin has enabled manufacturing
companies not only to plan maintenance but to get early warnings of the
likelihood of a breakdown, pinpoint the cause, and run scenario analyses in
which operational parameters can be varied at will to understand their impact
on equipment performance. Several eminent ‘brand’ products exist to create
these digital twins, but the software is often challenging to customise,
cannot always accommodate the specific needs of every manufacturing
environment, and significantly increases the total cost of ownership.
ML-powered digital twins can address these issues when they are purpose-built
to suit each company’s specific situation. They are affordable, scalable,
self-sustaining, and, with the right user interface, are extremely useful in
telling machine operators the exact condition of the equipment under their
care.
Artificial intelligence stands at odds with the goals of cutting greenhouse emissions. Here’s why
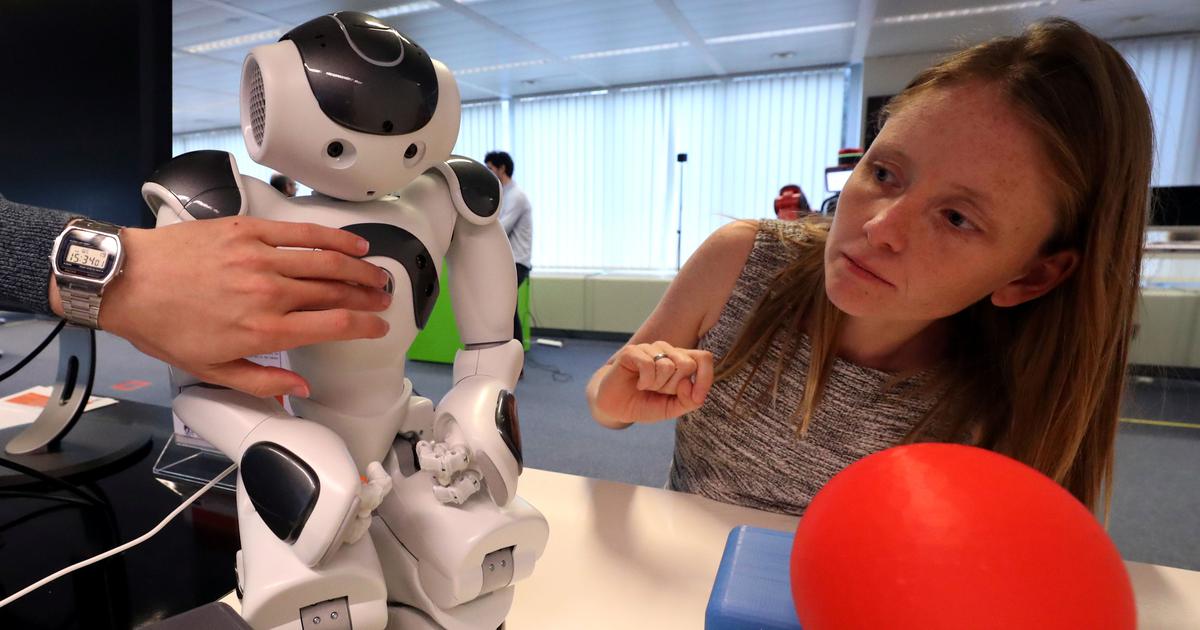
What does this mean for the future of AI research? Things may not be as bleak as
they look. The cost of training might come down as more efficient training
methods are invented. Similarly, while data center energy use was predicted to
explode in recent years, this has not happened due to improvements in data
center efficiency, more efficient hardware and cooling. There is also a
trade-off between the cost of training the models and the cost of using them, so
spending more energy at training time to come up with a smaller model might
actually make using them cheaper. Because a model will be used many times in its
lifetime, that can add up to large energy savings. In my lab’s research, we have
been looking at ways to make AI models smaller by sharing weights or using the
same weights in multiple parts of the network. We call these shapeshifter
networks because a small set of weights can be reconfigured into a larger
network of any shape or structure. Other researchers have shown that
weight-sharing has better performance in the same amount of training time.
Looking forward, the AI community should invest more in developing
energy-efficient training schemes.
Quote for the day:
"Any man who has ever led an army, an expedition, or a group of Boy Scouts has sadism in his bones." -- Tahir Shah
No comments:
Post a Comment