Cloud, containers, AI and RPA will spur a strong tech spending rebound in 2021
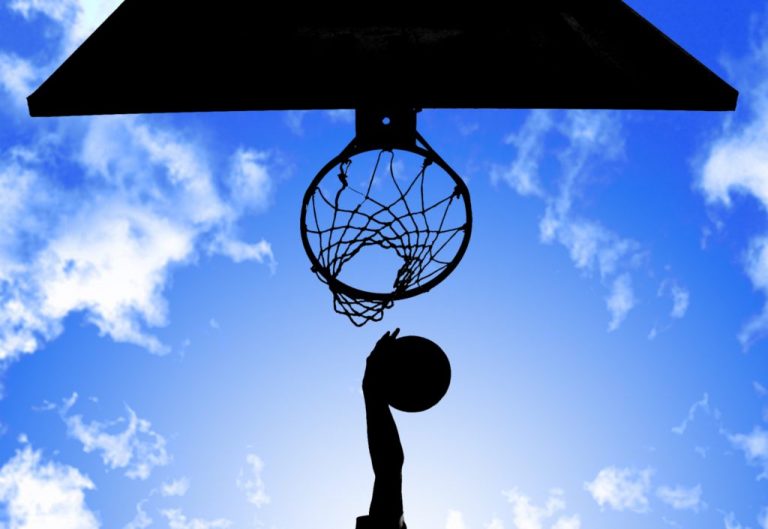
Not surprisingly, the ability to work remotely has been a critical factor.
Forty-four percent of respondents cited Business Continuity Plans as a key
factor. Several customers have told us, however, that their business
continuity plans were far too focused on disaster recovery and as such they
made tactical investments to shore up their digital capabilities. C-suite
backing and budget flexibility were cited as major factors. We see this as a
real positive in that the corner office and boards of directors are tuned into
digital. They understand the importance of getting digital “right” and we
believe that they now have good data from the past 10 months on which
investments will yield the highest payback. As such, we expect further funding
toward digital initiatives. Balance sheets are strong for many companies as
several have tapped corporate debt and taken advantage of the low interest
rate climate. Twenty-seven percent cited the use of emerging technologies as a
factor. Some of these, it could be argued, fall into the first category –
working remotely. The bottom line is we believe that the 10-month proof of
concept that came from COVID puts organizations in a position to act quickly
in 2021 to accelerate their digital transformations further by filling gaps
and identifying initiatives that will bring competitive advantage.
Digital transformation teams in 2021: 9 key roles

“Data analytics is a good place to start with any transformation, to make
sound decisions and design the proper solutions,” says Carol Lynn Thistle,
managing director at CIO executive recruiting firm Heller Search Associates.
One foundational IT position is the enterprise data architect or (in some
cases) a chief data officer. These highly skilled professionals can look at
blueprints, align IT tooling with information assets, and connect to the
business strategy, Thistle explains. ... “Digital transformation is about
automation of business processes using relevant technologies such AI, machine
learning, robotics, and distributed ledger,” says Fay Arjomandi, founder and
CEO of mimik Technology, a cloud-edge platform provider. “Individuals with
business knowledge that can define the business process in excruciating
detail. This is an important role, and we see a huge shortage in the market.”
... “[Organizations need] a digitally savvy person at the CXO level who will
help other executives buy into the culture change that will be required to
truly transform the organization into one that is digital-first,” says Mike
Buob, vice president of customer experience and innovation for Sogeti, the
technology and engineering services division of Capgemini.
Quantum Computing Marks New Breakthrough, Is 100 Trillion Times More Efficient

Jiuzhang, as the supercomputer is called, has outperformed Google’s
supercomputer, which the company had claimed last year to have achieved
quantum computing supremacy. The supercomputer by Google named Sycamore is a
54-qubit processor, consisting of high-fidelity quantum logic gates that could
perform the target computation in 200 seconds. The researchers explored
Boson sampling, a task considered to be a strong candidate to demonstrate
quantum computational advantage. As the researcher cited in the research
paper, they performed Gaussian boson sampling (GBS), which is a new paradigm
of boson sampling, one of the first feasible protocols for quantum
computational advantage. In boson sampling and its variants, nonclassical
light is injected into a linear optical network, which generates highly random
photon-number, measured by single-photon detectors. Researchers sent 50
indistinguishable single-mode squeezed states into a 100-mode ultralow-loss
interferometer with full connectivity and random matrix. They further shared
that the whole optical setup is phase-locked and that the sampling of output
was done using 100 high-efficiency single-photon detectors.
Why Edge Computing Matters in IoT

The Edge basically means “not Cloud” because what constitutes the Edge can
differ depending on the application. To explain, let’s look at an example. In
a hospital, you might want to know the location of all medical assets (e.g.,
IV pumps, EKG machines, etc.) and use a Bluetooth indoor tracking IoT
solution. The solution has Bluetooth Tags, which you attach to the assets you
want to track (e.g., an IV pump). You also have Bluetooth Hubs, one in each
room, that listens for signals from the Tags to determine which room each Tag
is in (and therefore what room the asset is in). In this scenario, both the
Tags and the Hubs could be considered the “Edge.” The Tags could perform some
simple calculations and only send data to the Hubs if there’s a large sensory
data change. ... One of the issues with the term ”IoT” is how broadly it’s
defined. Autonomous vehicles that cost tens of thousands of dollars collect
Terabytes of data and use 4G cellular networks are considered IoT. At the same
time, sensors that cost a couple of dollars collect just bytes of data and use
Low-Power Wide-Area Networks (LPWANS) are also considered IoT. The problem is
that everyone is focusing on high bandwidth IoT applications like autonomous
vehicles, the smart home, and security cameras.
Could AI become dangerous?
When asked about the dangers of AI, Arman asserted that ‘danger has always
existed in every technological innovation in history, from the
ever-increasing trail of pollution caused by the first Industrial Revolution
to the idea of Nuclear power generation to free use of pesticides everywhere
into genetic modification of food and so on.’ AI is only a part of that as
‘it is on its path to outgrow human’s capacity to fully understand how it
makes decisions and what is the base of its outcomes.’ Indeed, this would be
the first time that our intellectual superiority would be taken away. To
shed some light on this, Arman retells a conversation he had with one AI
lead from key players in Silicon Valley during a meeting in 2017: ‘After 2
hours of discussing, brainstorming and trying to picture a path, we ended up
having no firm idea on where AI was leading us. The final outcome was that
each individually announced that they believe it is too early to predict
anything and we can’t even say with certainty where we will be in 18 months.
They also refused to acknowledge the risk that was brought up through
research from my team projecting that – back in 2017, even with AI still
being in its infancy – it had the ability to take away over 1 billion jobs
across the globe.
What’s New on F#: Q&A With Phillip Carter
FP and Object-Oriented Programming (OOP) aren’t really at odds with each
other, at least not if you use each as if they were a tool rather than a
lifestyle. In FP, you generally try to cleanly separate your data definitions
from functionality that operates on. In OOP, you’re encouraged to combine them
and blur the differences between them. Both can be incredibly helpful
depending on what you’re doing. For example, in the F# language we encourage
the use of objects to encapsulate data and expose functionality conveniently.
That’s a far cry from encouraging people to model everything using inheritance
hierarchies, and at the end of the day you still tend to work with an object
in a functional way, by calling methods or properties that just produce
outputs. Both styles can work well together if you don’t “all in” on one
approach or the other. ... What’s interesting is that even though F# runs on
.NET, which often has an “enterprisey” kind of reputation, F# itself doesn’t
really suffer the negative aspects of that kind of reputation. It can be used
for enterprise work, but it’s usually seen as lightweight and its community is
engaged and available as opposed to stuck behind a corporate firewall.
3 questions to ask before adopting microservice architecture

Teams may take different routes to arrive at a microservice architecture,
but they tend to face a common set of challenges once they get there. John
Laban, CEO and co-founder of OpsLevel, which helps teams build and manage
microservices told us that “with a distributed or microservices based
architecture your teams benefit from being able to move independently from
each other, but there are some gotchas to look out for.” Indeed, the linked
O’Reilly chart shows how the top 10 challenges organizations face when
adopting microservices are shared by 25%+ of respondents. While we discussed
some of the adoption blockers above, feedback from our interviews
highlighted issues around managing complexity. The lack of a coherent
definition for a service can cause teams to generate unnecessary overhead by
creating too many similar services or spreading related services across
different groups. One company we spoke with went down the path of
decomposing their monolith and took it too far. Their service definitions
were too narrow, and by the time decomposition was complete, they were left
with 4,000+ microservices to manage. They then had to backtrack and
consolidate down to a more manageable number.
IT careers: 10 critical skills to master in 2021

The key to adaptability, virtual collaboration, and digital transformation
(and agile) is distributed leadership and self-managed teams. This requires
that everyone have core leadership skills, and not just people in the
positions of managers and above. For the past 11 years, I’ve been training
and coaching IT professionals at every job level – from individual
contributors up to CIOs – in what I believe are the six key core leadership
skills that every IT professional needs to master, even more so today than
at any time in the past. ... "Yes, IT professionals need to know the
underpinnings of technology and tech trends. But what many fail to realize
is how heavily IT leaders rely on effective communication skills to do their
jobs successfully. As CIO of ServiceNow, my role demands clear, consistent
communication – both within my organization and across other functions – to
make sure that everyone is aligned on the right outcomes. Communication is
the key to digital transformation and IT professionals need to communicate
with employees across departments on what this means for their work.” -
Chris Bedi, CIO, ServiceNow
How to industrialize data science to attain mastery of repeatable intelligence delivery

As you look at the amount of productive time data scientists spend creating
value, that can be pretty small compared to their non-productive time — and
that’s a concern. Part of the non-productive time, of course, has been with
those data scientists having to discover a model and optimize it. Then they
would do the steps to operationalize it. But maybe doing the data and
operations engineering things to operationalize the model can be much more
efficiently done with another team of people who have the skills to do that.
We’re talking about specialization here, really. But there are some other
learnings as well. I recently wrote a blog about it. In it, I looked at the
modern Toyota production system and started to ask questions around what we
could learn about what they have learned, if you like, over the last 70
years or so. It was not just about automation, but also how they went about
doing research and development, how they approached tooling, and how they
did continuous improvement. We have a lot to learn in those areas. For an
awful lot of organizations that I deal with, they haven’t had a lot of
experience around such operationalization problems. They haven’t built that
part of their assembly line yet.
What is neuromorphic computing? Everything you need to know about how it is changing the future of computing
First, to understand neuromorphic technology it make sense to take a quick
look at how the brain works. Messages are carried to and from the brain
via neurons, a type of nerve cell. If you step on a pin, pain receptors in the
skin of your foot pick up the damage, and trigger something known as an action
potential -- basically, a signal to activate -- in the neurone that's
connected to the foot. The action potential causes the neuron to release
chemicals across a gap called a synapse, which happens across many neurons
until the message reaches the brain. Your brain then registers the pain, at
which point messages are sent from neuron to neuron until the signal reaches
your leg muscles -- and you move your foot. An action potential can be
triggered by either lots of inputs at once (spatial), or input that builds up
over time (temporal). These techniques, plus the huge interconnectivity of
synapses -- one synapse might be connected to 10,000 others -- means the brain
can transfer information quickly and efficiently. Neuromorphic computing
models the way the brain works through spiking neural networks. Conventional
computing is based on transistors that are either on or off, one or zero.
Quote for the day:
"Every great leader has incredible odds to overcome." -- Wayde Goodall
No comments:
Post a Comment