Generative AI isn’t coming for you — your reluctance to adopt it is

Faced with a growing to-do list and the new balancing act of returning from
maternity leave to an expanded role leading public relations for a
publicly-traded tech company, I opened Jasper AI. I admittedly smirked at some
of the functionality. Changing the tone? Is this AI emotionally intelligent?
Maybe more so than some former colleagues. I began on a blank screen. I started
writing a few lines and asked the AI to complete the piece for me. I reveled in
the schadenfreude of its failure. It summarized what I had written at the top of
the document and just spit it out below. Ha! I had proven my superiority. I went
back into my cave, denying myself and my organization the benefits of this
transformative technology. The next time I used gen AI, something in me changed.
I realized how much prompting matters. You can’t just type a few initial
sentences and expect the AI to understand what you want. It still can’t read our
minds (I think). But there are dozens of templates that the AI understands. For
PR professionals, there are templates for press releases, media pitches, crisis
communications statements, press kits and more.
What's Preventing CIOs From Achieving Their AI Goals?
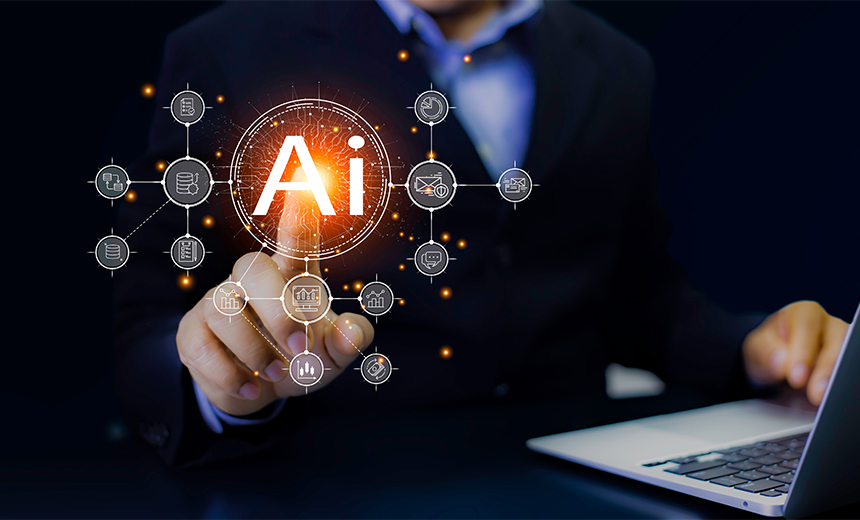
"While no CIO wants to be left behind, they are also prudent about their AI
adoption journeys and how they implement the technology for business in a
responsible manner," said Dr. Jai Ganesh, chief product officer, HARMAN
International. "While there are many business use cases, enterprises are
prioritizing these on a must-have immediately to implement basis." ... He also
oversees AI implementation across his company. Technology leaders say it will
take at least two to three years before AI becomes mainstream across the
enterprise. Rakesh Jayaprakash, chief analytics evangelist at ManageEngine, told
ISMG that we would start to see "very tangible results" at a larger scale in
another one or two years. "Tangible results" refer to commoditization of AI,
which accelerates the ROI, he said. "While there is a lot of hype around AI now,
the true value comes when the organizations are able to see the outcomes,"
Jayaprakash said. "Right now, many organizations jump in with very high
expectations of what is possible through AI, because we've started to use tools
such as ChatGPT to accomplish very simple tasks. But when it comes to
organization-level use cases, those are a little more complex."
Bridging the Data Gap: The Role of Industrial DataOps in Digital Transformation
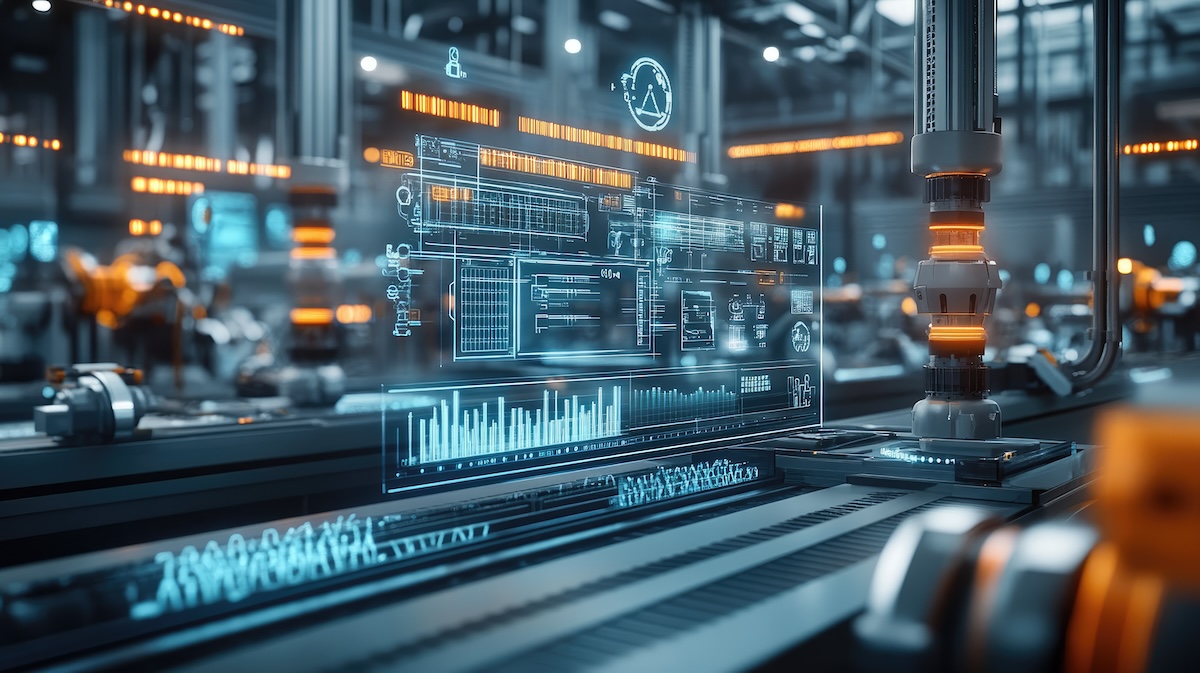
One of the main issues faced by organizations is the lack of context in
industrial data. Unlike IT systems, where data is typically well-defined and
structured, data from industrial environments often lacks the necessary
context to be useful. For example, a temperature reading from a manufacturing
machine might be labeled simply as “temperature sensor 1,” leaving operators
to guess its relevance without proper identification. This lack of
contextualization—when applied to thousands of data points across multiple
facilities— Is a major barrier to advanced analytics and digitalization
programs. ... By implementing Industrial DataOps, organizations can address
this gap by contextualizing data as close to the source as possible—ideally at
the edge of the network. This approach empowers operators who have tribal
knowledge of the data and its sources to deliver ready-to-use data to IT and
line of business users in their organization. Decisions become faster and more
informed. The ultimate goal is to transform raw data into valuable insights
that drive operational improvements. ... As organizations adopt Industrial
DataOps, they unlock the potential for rapid innovation. With a solid data
management framework in place, OT teams can easily explore new use cases and
validate hypotheses.
Ensuring AI-readiness of Data Is a Long-term Commitment
Data becomes an intellectual property when one enters the world of GenAI, and
it is the way with which one can customize algorithms to reflect the brand
voice and deliver great client services. Keeping the scenario in mind,
Birkhead states that modernizing data and ensuring its AI-readiness is a
long-term commitment. While organizations can make incremental progress year
after year, building an analytic factory to produce AI models that support the
business takes strategy, investment, and an enabling leadership team.
Highlighting JPMC’s data strategy, Birkhead states that the components include
data design principles, operating models, principles around platforms,
tooling, and capabilities. Additionally, talent, governance, data, and AI
ethics also come into play, but the ultimate goal is to have incredibly
high-quality data that is self-describing and understandable by both humans
and machines. From Birkhead’s standpoint, to be AI-ready with data,
organizations have to get data to a state where a data scientist, user, or AI
researcher can go into a marketplace and understand everything about the
data.
Business Etiquette Classes Boom as People Relearn How to Act at Work
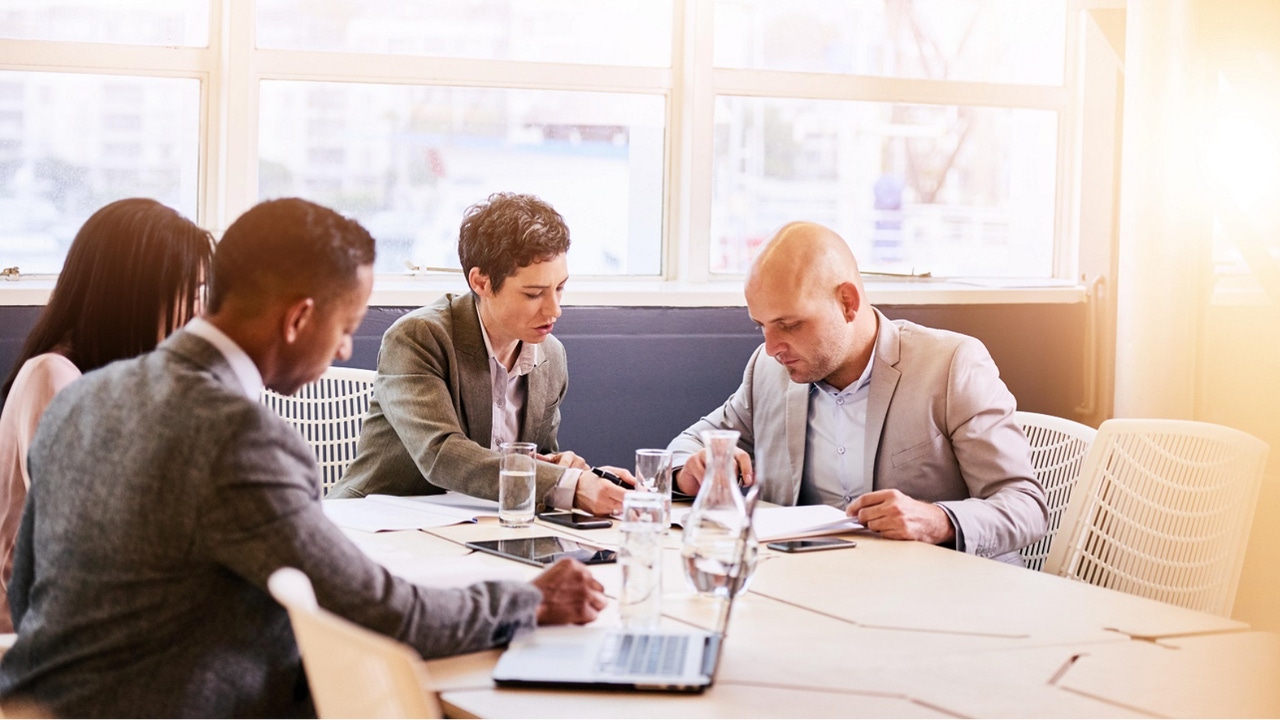
Workers who had substantial professional experience before the pandemic,
including managers and executives, still need help adapting to hybrid and
remote work, Senning said. He has been coaching leaders on best practices for
such things as communicating through your calendar and deciding whether to
call, text or use Slack to reach an employee. stablishing etiquette for video
meetings has also been a challenge for many firms, he notes. Bad behavior in
virtual meetings has occasionally made headlines in recent years, such as the
backlash against Vishal Garg, CEO of the mortgage lending firm Better.com, for
announcing mass layoffs over Zoom ahead of the holidays in 2021. "If I had a
magic button that I could push that could get people to treat video meetings
with 50 percent of the same level of professionalism they treat an in-person
meeting, I would make a lot of HR, personnel managers, and executives very,
very happy," Senning said. Tech companies also are paying for etiquette and
professionalism training for their workers, especially if they're bringing in
employees who have never worked in person before, according to Crystal Bailey,
director of the Etiquette Institute of Washington, who counts Amazon among her
clients.
Exploring the Power of AI in Software Development - Part 1: Processes
AI holds the power to significantly enhance the requirement analysis and
planning processes at the early stages of the software development life cycle
(SDLC). It can analyze massive amounts of data in order to identify user needs
and preferences, allowing developers to make informed decisions about features
and functionality. ... AI can also look at coding rates per user story within
an app architecture context and allow Product Managers to better determine
project timelines and resource needs. In doing so, they can more accurately
predict the risk-reward of time-to-market versus high quality for every
release, knowing that no software will be 100% defect-free. ... With AI, you
have a pair programmer who has infinite patience. Someone who will not judge
you for seemingly "stupid" questions. Having this kind of support can increase
an engineer's capabilities and productivity. So often as a junior engineer, I
was afraid to ask the senior engineers on my team questions because I thought
I should know the answer. Engineers can use AI without the worry of judgment,
so no question is stupid, no answer should be known.
How AI is Shaping the Future of Product Development
/dq/media/media_files/2024/10/28/B0lIAyZN9ss9nhIMXCVp.png)
Product testing and iteration processes are also being revolutionized by AI,
which results in shorter development cycles and better product outcomes as
well. While tried and true testing methods can work well, they often have long
cycles or may miss problems. Quiet contrary to traditional testing, AI-driven
automation suggests a new degree of efficiency and accuracy. AI tools for
early-stage testing makes it possible to discover issues quickly and try out
potential applications, which lowers the demand on manual resources spent in
validating components or debugging. Not just that, AI's ability to analyze
code bases comprehensively provides targeted insights for ongoing
improvements. By integrating AI into testing processes, businesses can
accelerate development cycles, reduce costs, and deliver products that better
align with user expectations. ... By embedding AI into their growth
strategies, companies can benefit in numerous ways. It allows for more
targeted and personalized experience to be delivered, subsequently
personalizing the products or services provided by companies. Such a
custom-built solution not only enhances user experience but also helps create
brand loyalty. Additionally, AI allows companies to have data-driven decision
making that facilitates strategic planning and execution.
From Safety to Innovation: How AI Safety Institutes Inform AI Governance
According to the report, this “first wave” of AISIs has three common
characteristics:Safety-focus: The first wave of AISIs was informed by the
Bletchley AI Safety Summit, which declared that “AI should be designed,
developed, deployed, and used in a manner that is safe, in such a way as to be
human-centric, trustworthy, and responsible.” These institutes are
particularly concerned with mitigating abuse and safeguarding frontier AI
models. Government-led: These AISIs are governmental institutions, providing
them with the “authority, legitimacy, and resources” needed to address AI
safety issues. Their governmental status helps them access leading AI models
to run evaluations, and importantly, it gives them greater leverage in
negotiating with companies unwilling to comply. Technical: AISIs are focused
on attracting technical experts to ensure an evidence-based approach to AI
safety. The report also points out some key ways AISIs are unique. For one,
AISIs are not a “catch-all” entity to tackle the complex and ever-evolving AI
governance landscape. They are also relatively free of the bureaucracy
commonly associated with governmental agencies. This may be due to the fact
that these institutes have very little regulatory authority and focus more on
establishing best practices and conducting safety evaluations to inform
responsible AI development.
Current Top Trends in Data Analytics
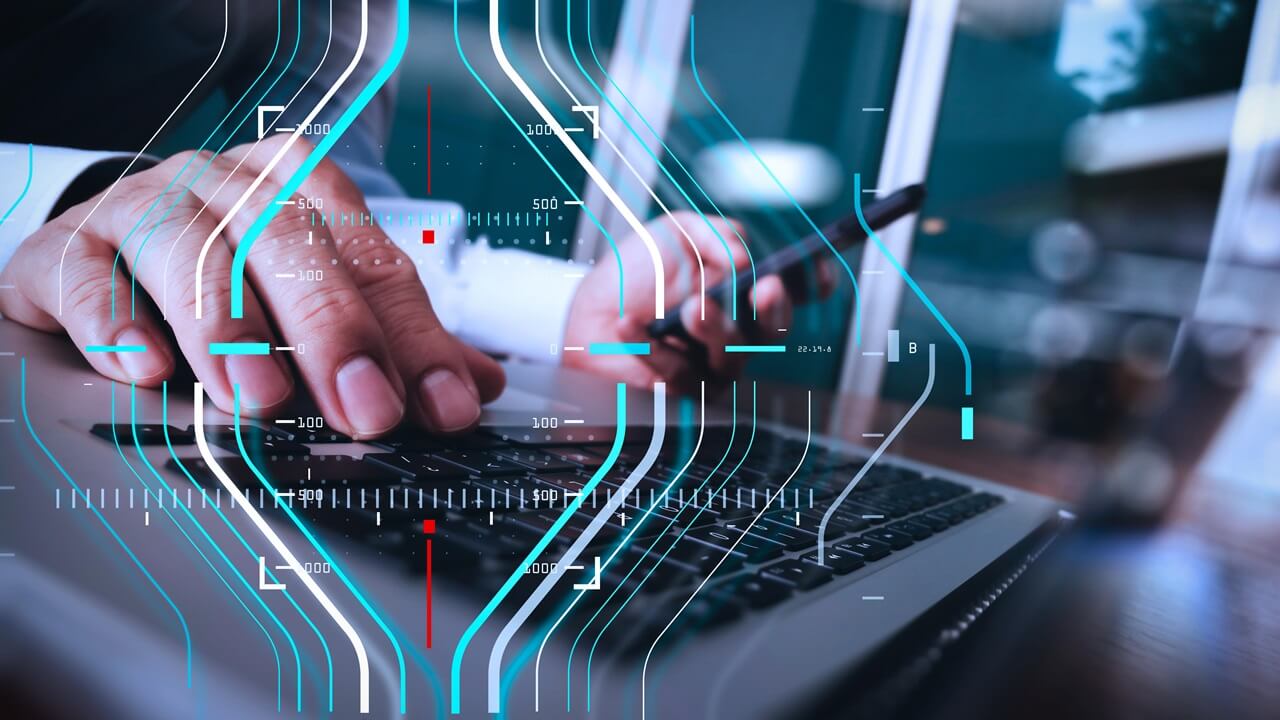
One of the most impactful data analytics trends right now is the integration
of AI and machine learning (ML) into analytics frameworks, observes Anil
Inamdar, global head of data services at data monitoring and management firm
Instaclustr by NetApp, an online interview. "We are seeing the emergence of a
new data 4.0 era, which builds on previous shifts that focused on automation,
competitive analytics, and digital transformation," Inamdar states. "This
distinct new phase leverages AI/ML and generative AI to significantly enhance
data analytics capabilities," he says. While the transformative potential is
now here for the taking, enterprises must carefully strategize across several
key areas. ... Data governance should be a top concern for all enterprises.
"If it isn't yours, you’re heading for a world of hurt," warns Kris Moniz,
national data and analytics practice lead for business and technology advisory
firm Centric Consulting, via email. Data governance dictates the rules under
which data should be managed, Moniz says. "It doesn’t just do this by
determining who gets access to what," he notes. "It also does it by defining
what your data is, setting processes that can guarantee its quality, building
frameworks that align disparate systems across common domains, and setting
standards for common data that all systems should consume."
Effective Data Mesh Begins Wtih Robust Data Governance
When implemented correctly, removing the dependency on centralised systems and
IT teams can truly transform the way organisations operate. However,
introducing a data mesh can also raise fears and concerns relating to storage,
duplication, management, and compliance, all of which must be addressed if it
is to succeed. With decentralised data management, it’s also critical that
everyone follows the same stringent set of rules, particularly regarding the
creation, storage, and protection of data. If not, issues will quickly arise.
Additionally, if any team leaders or department heads put their own tools or
processes in place, the results may cause far more problems than they solve.
Trusting individuals to stick to data guidelines is too risky. Instead,
adherence should be enforced in a way that ensures standards are followed,
without impacting agility or frustrating users. This may sound impractical,
but a computational governance approach can impose the necessary restrictions,
while at the same time accelerating project delivery. Naturally, not everyone
will be quick (or keen) to adjust, but with additional support and training
even the most reluctant individuals can learn how to adopt a more
entrepreneurial mindset.
Quote for the day:
"Trust is the lubrication that makes
it possible for organizations to work." -- Warren G. Bennis