What Is Quantum Networking, and What Might It Mean for Data Centers?
.jpg?width=1280&auto=webp&quality=95&format=jpg&disable=upscale)
Conventional networks shard data into packets and move them across wires or
radio waves using long-established networking protocols, such as TCP/IP. In
contrast, quantum networks move data using photons or electrons. It leverages
unique aspects of quantum physics to enable powerful new features like
entanglement, which effectively makes it possible to verify the source of data
based on the quantum state of the data itself. ... Because quantum networking
remains a theoretical and experimental domain, it's challenging to say at
present exactly how quantum networks might change data centers. What does seem
clear, however, is that data center operators seeking to offer full support for
quantum devices will need to implement fundamentally new types of network
infrastructure. They'll need to deploy infrastructure resources like quantum
repeaters, while also ensuring that they can support whichever networking
standards might emerge in the quantum space. The good news for the fledgling
quantum data center ecosystem is that true quantum networks aren't a
prerequisite for connecting quantum computers. It's possible for quantum
machines themselves to send and receive data over classical networks by using
traditional computers and networking devices as intermediaries.
Unmasking Big Tech’s AI Policy Playbook: A Warning to Global South Policymakers
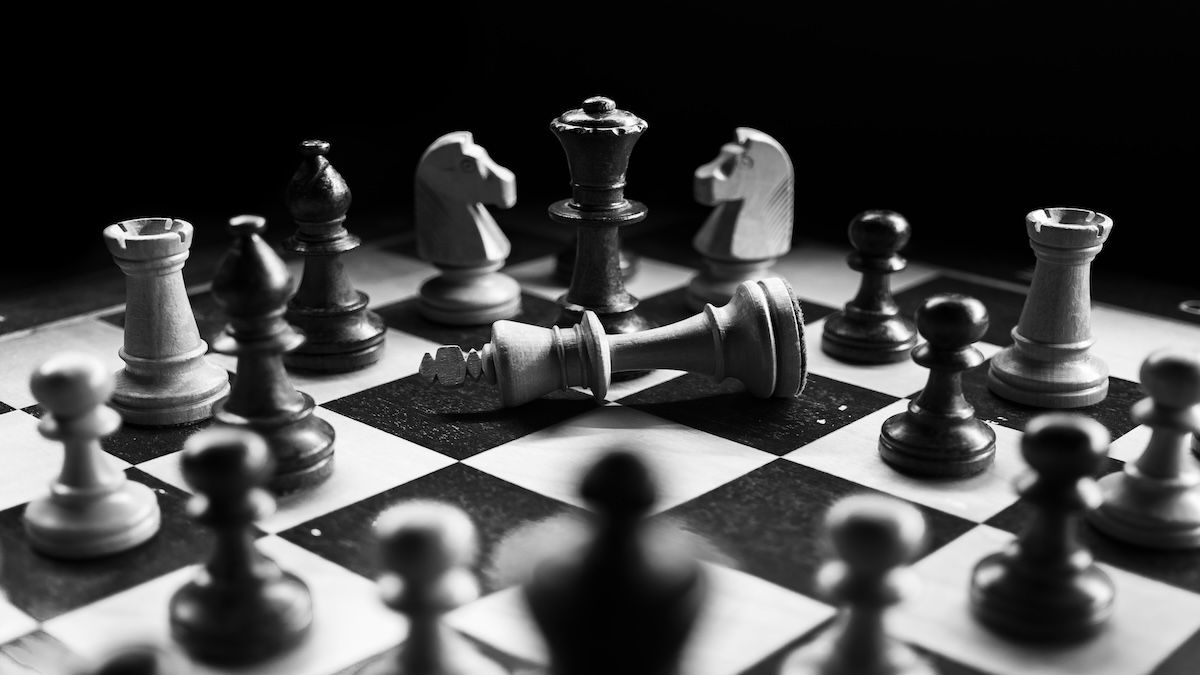
Rather than a genuine, inclusive discussion about how governments should
approach AI governance, what we are witnessing instead is a clash of seemingly
competing narratives swirling together to obfuscate the real aspirations of
big tech. The advocates of open-source large language models (LLMs) present
themselves as civic-minded, democratic, and responsible, while closed-source
proponents position themselves as the responsible stewards of secure,
walled-garden AI development. Both sides dress their arguments with warnings
about dire consequences if their views aren’t adopted by policymakers. ... For
years, tech giants have employed scare tactics to convince policymakers that
any regulation will stifle innovation, lead to economic decline, and exclude
countries from the prestigious digital vanguard. These dire warnings are
frequently targeted, especially in the Global South, where policymakers often
lack the resources and expertise to keep pace with rapid technological
advancements, including AI. Big tech’s polished lobbyists offer what seems
like a reasonable solution, workable regulation" — which translates to
delayed, light-touch, or self-regulation of emerging technologies.
AI Agents: A Comprehensive Introduction for Developers

The best way to think about an AI agent is as a digital twin of an employee
with a clear role. When any individual takes up a new job, there is a
well-defined contract that establishes the essential elements — such as job
definition, success metrics, reporting hierarchy, access to organizational
information, and whether the role includes managing other people. These
aspects ensure that the employee is most effective in their job and
contributes to the overall success of an organization. ... The persona of an
AI agent is the most crucial aspect that establishes the key trait of an
agent. It is the equivalent of a title or a job function in the traditional
environment. For example, a customer support engineer skilled in handling
complaints from customers is a job function. It is also the persona of an
individual who performs this job. You can easily extend this to an AI agent.
... A task is an extension of the instruction that focuses on a specific,
actionable item within the broader scope of the agent’s responsibilities.
While the instruction provides a general framework covering multiple potential
actions, a task is a direct, concrete action that the agent must take in
response to a particular user input.
AI in compliance: Streamlining HR processes to meet regulatory standards
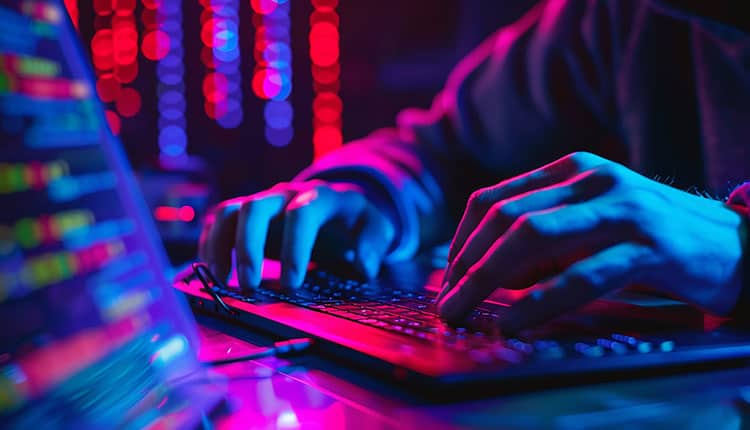
With the increasing focus on data protection laws like the General Data
Protection Regulation (GDPR), the California Consumer Privacy Act (CCPA), and
India’s Information Technology (Reasonable Security Practices and Procedures
and Sensitive Personal Data or Information) Rules, 2011 under the Information
Technology Act, 2000, maintaining the privacy and security of employee data
has become paramount. The Indian IT Privacy Law mandates that companies ensure
the protection of sensitive personal data, including employee information, and
imposes strict guidelines on how data must be collected, processed, and
stored. AI can assist HR teams by automating data management processes and
ensuring that sensitive information is stored securely and only accessed by
authorized personnel. AI-driven tools can also help monitor compliance with
data privacy regulations by tracking how employee data is collected,
processed, and shared within the organization. ... This proactive monitoring
reduces the likelihood of non-compliance and minimizes risks associated with
data breaches, helping organizations align with both international and
domestic privacy laws like the Indian IT Privacy Law.
Are humans reading your AI conversations?

Tools like OpenAI’s ChatGPT and Google’s Gemini are being used for all sorts
of purposes. In the workplace, people use them to analyze data and speed up
business tasks. At home, people use them as conversation partners, discussing
the details of their lives — at least, that’s what many AI companies hope.
After all, that’s what Microsoft’s new Copilot experience is all about — just
vibing and having a chat about your day. But people might share data that’d be
better kept private. Businesses everywhere are grappling with data security
amid the rise of AI chatbots, with many banning their employees from using
ChatGPT at work. They might have specific AI tools they require employees to
use. Clearly, they realize that any data fed to a chatbot gets sent to that AI
company’s servers. Even if it isn’t used to train genAI models in the future,
the very act of uploading data could be a violation of privacy laws such as
HIPAA in the US. ... Companies that need to safeguard business data and follow
the relevant laws should carefully consider the genAI tools and plans they
use. It’s not a good idea to have employees using a mishmash of tools with
uncertain data protection agreements or to do anything business-related
through a personal ChatGPT account.
CIOs recalibrate multicloud strategies as challenges remain

Like many enterprises, Ally Financial has embraced a primary public cloud
provider, adding in other public clouds for smaller, more specialized
workloads. It also runs private clouds from HPE and Dell for sensitive
applications, such as generative AI and data workloads requiring the highest
security levels. “The private cloud option provides us with full control over
our infrastructure, allowing us to balance risks, costs, and execution
flexibility for specific types of workloads,” says Sathish Muthukrishnan,
Ally’s chief information, data, and digital officer. “On the other hand, the
public cloud offers rapid access to evolving technologies and the ability to
scale quickly, while minimizing our support efforts.” Yet, he acknowledges a
multicloud strategy comes with challenges and complexities — such as moving
gen AI workloads between public clouds or exchanging data from a private cloud
to a public cloud — that require considerable investments and planning.
“Aiming to make workloads portable between cloud service providers
significantly limits the ability to leverage cloud-native features, which are
perhaps the greatest advantage of public clouds,” Muthukrishnan says.
DevOps and Cloud Integration: Best Practices
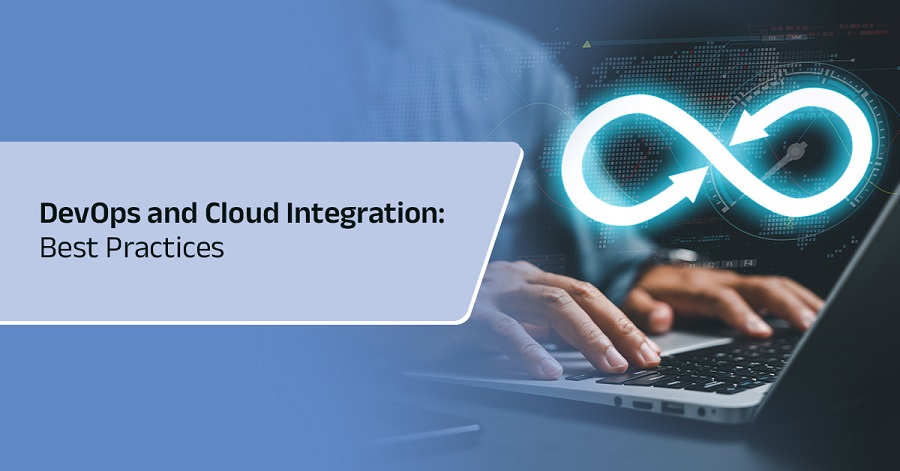
CI/CD practices are crucial for DevOps implementation with cloud services.
Continuous integration regularly merges code changes into a shared repository,
where automated tests are run to spot issues early. On the other hand,
continuous deployment improves this practice by automatically deploying
changes (once they pass tests) to production. The CI/CD approach can
accelerate the release cycle and enhance the overall quality of the software.
... Infrastructure as Code (IaC) empowers teams to oversee and provision
infrastructure via code rather than manual processes. This DevOps methodology
guarantees uniformity across environments and facilitates infrastructure
scalability in cloud-based settings. It represents a pivotal element in
transforming any enterprise's DevOps strategy. ... According to DevOps
experts(link is external), security needs to be a part of every step in the
DevOps process, called DevSecOps. This means adding security checks to the
CI/CD pipeline, using security tools for the cloud, and always checking for
security issues. DevOps professionals usually stress how important it is to
tackle security problems early in the development process, called "shifting
left."
Data Resilience & Protection In The Ransomware Age
Backups are considered the primary way to recover from a breach, but is this
enough to ensure that the organisation will be up and running with minimal
impact? Testing is a critical component to ensuring that a company can recover
after a breach and provides valuable insight into the steps that the company
will need to take to recover from a variety of scenarios. Unfortunately, many
organisations implement measures to recover but fail on the last step of their
resilience approach, namely testing. Without this step, they cannot know if
their recovery strategy is effective. Testing is a critical component as it
provides valuable insight into the steps it needs to take to recover, what
works, and what areas it needs to focus on for the recovery process, the
amount of time it will take to recover the files and more. Without this,
companies will not know what processes to follow to restore data following a
breach, as well as timelines to recovery. Equally, they will not know if they
have backed up their data correctly before an attack if they have not
performed adequate testing. Although many IT teams are stretched and struggle
to find the time to do regular testing, it is possible to automate the testing
process to ensure that it occurs frequently.
Is data gravity no longer centered in the cloud?

The need for data governance and security is escalating as AI becomes more
prevalent. Organizations are increasingly aware of the risks associated with
cloud environments, especially regarding regulatory compliance. Maintaining
sensitive data on premises allows for tighter controls and adherence to
industry standards, which are often critical in AI applications dealing with
personal or confidential information. The convergence of these factors signals
a broader reevaluation of cloud-first strategies, leading to hybrid models
that balance the benefits of cloud computing with the reliability of
traditional infrastructures. This hybrid approach facilitates a tailored fit
for various workloads, optimizing performance while ensuring compliance and
security. ... Data can exist on any platform, and accessibility should not be
problematic regardless of whether data resides on public clouds or on
premises. Indeed, the data location should be transparent. Storing data
on-prem or with public cloud providers affects how much an enterprise spends
and the data’s accessibility for major strategic applications, including AI.
Currently, on-prem is the most cost-effective AI platform—for most data sets
and most solutions.
Choosing Between Cloud and On-Prem MLOps: What's Best for Your Needs?
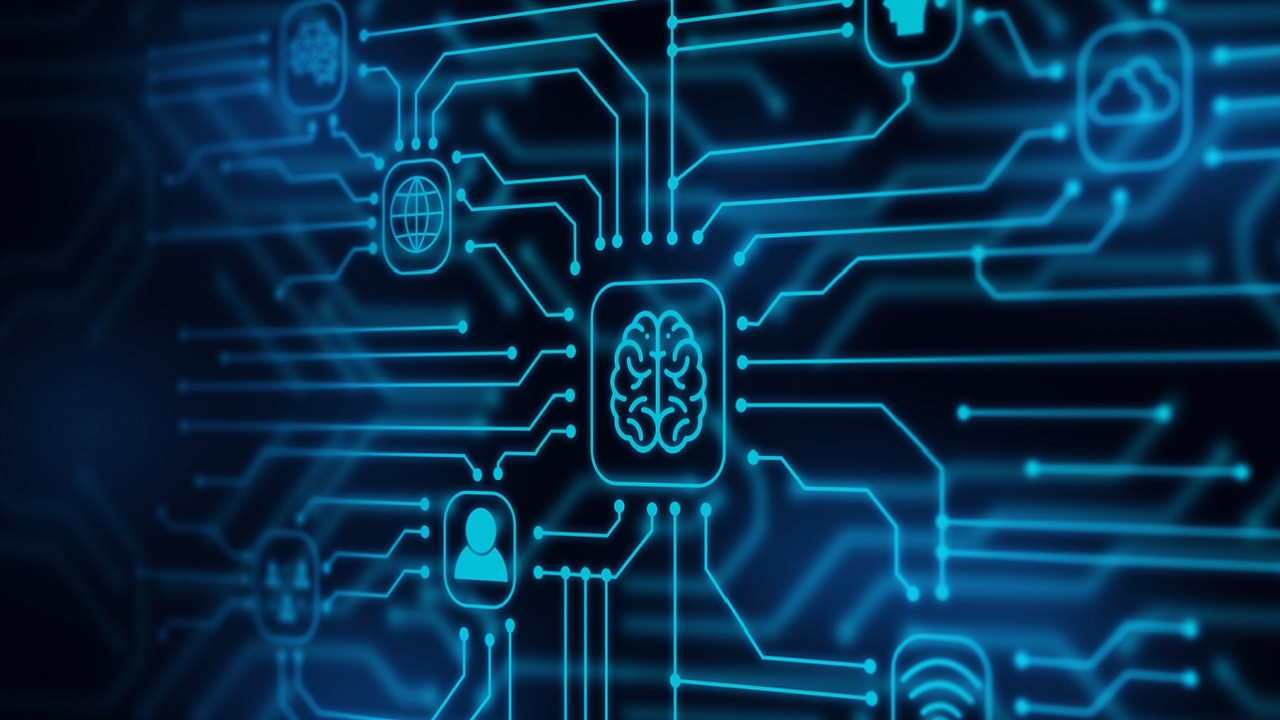
The big benefit of cloud MLOps is the availability of virtually unlimited
quantities of CPU, memory, and storage resources. Unlike on-prem environments,
where resource capacity is limited by the amount of servers available and the
resources each one provides, you can always acquire more infrastructure in the
cloud. This makes cloud MLOps especially beneficial for ML use cases where
resource needs vary widely or are unpredictable. ... On-prem MLOps may also
offer better performance. On-prem environments don't require you to share
hardware with other customers (which the cloud usually does), so you don't
have to worry about "noisy neighbors" slowing down your MLOps pipeline. The
ability to move data across fast local network connections can also boost
on-prem MLOps performance, as can running workloads directly on bare metal,
without a hypervisor layer reducing the amount of resources available to your
workloads. ... You could also go on, under a hybrid MLOps approach, to deploy
your model either on-prem or in the cloud depending on factors like how many
resources inference will require.
Quote for the day:
"You'll never get ahead of anyone as
long as you try to get even with him." -- Lou Holtz
No comments:
Post a Comment