AI Models in Cybersecurity: From Misuse to Abuse
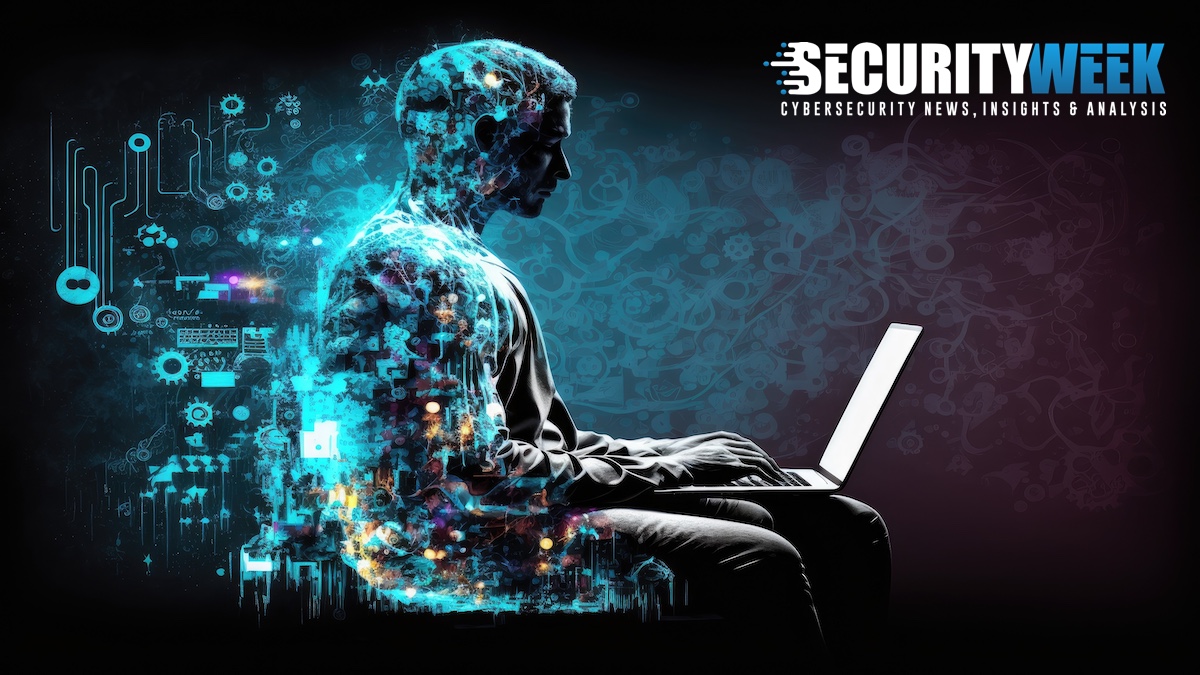
In a constant game of whack-a-mole, both defenders and attackers are harnessing
AI to tip the balance of power in their respective favor. Before we can
understand how defenders and attackers leverage AI, we need to acknowledge the
three most common types of AI models currently in circulation. ... Generative
AI, Supervised Machine Learning, and Unsupervised Machine Learning are three
main types of AI models. Generative AI tools such as ChatGPT, Gemini, and
Copilot can understand human input and can deliver outputs in a human-like
response. Notably, generative AI continuously refines its outputs based on user
interactions, setting it apart from traditional AI systems. Unsupervised machine
learning models are great at analyzing and identifying patterns in vast
unstructured or unlabeled data. Alternatively, supervised machine learning
algorithms make predictions from well-labeled, well-tagged, and well-structured
datasets. ... Despite the media hype, the usage of AI by cybercriminals is still
at nascent stage. This doesn’t mean that AI is not being exploited for malicious
purposes, but it’s also not causing the decline of human civilization like some
purport it to be. Cybercriminals use AI for very specific tasks
Meet Aria: The New Open Source Multimodal AI That's Rivaling Big Tech
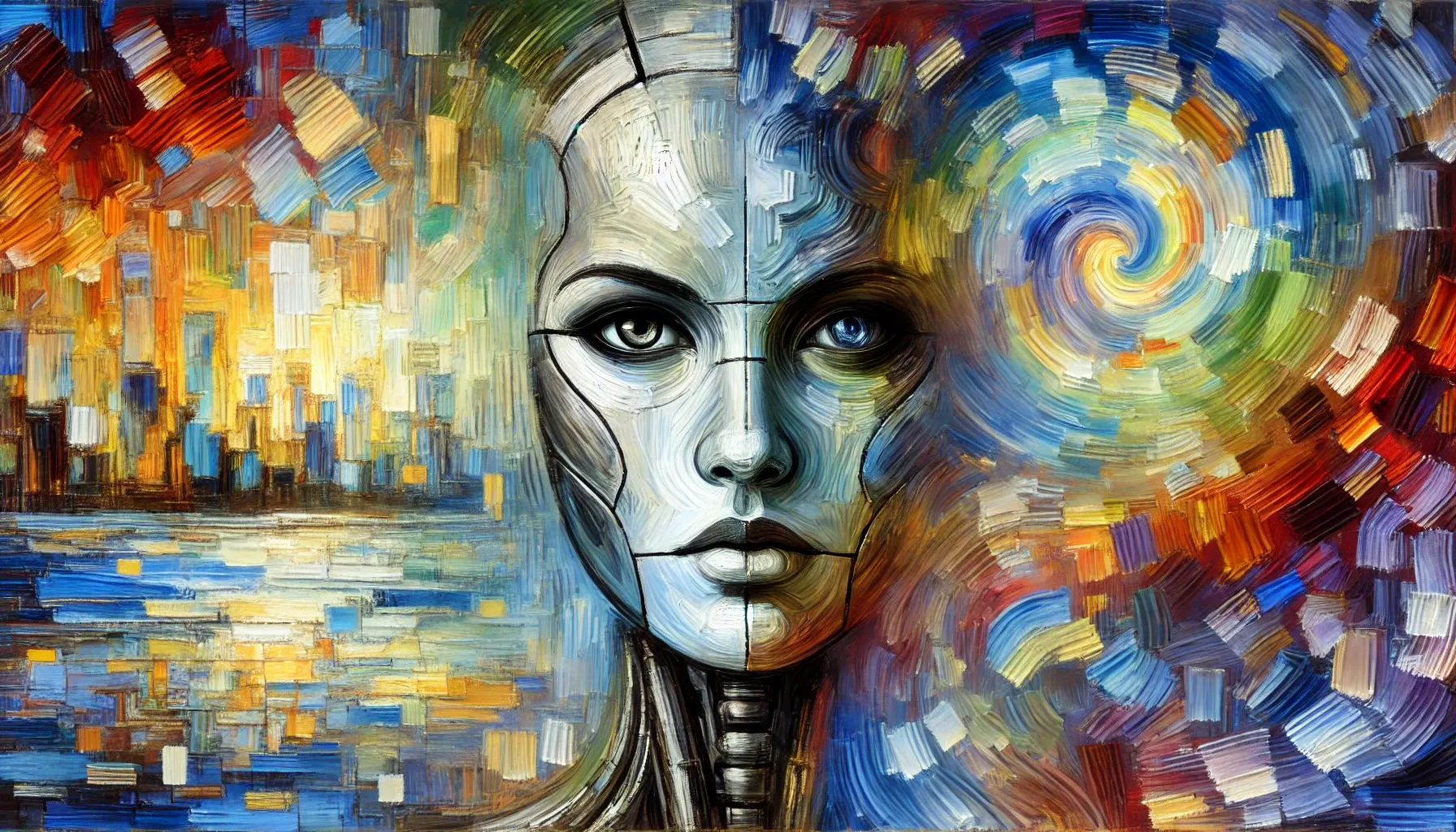
Rhymes AI has released Aria under the Apache 2.0 license, allowing developers
and researchers to adapt and build upon the model. It is also a very powerful
addition to an expanding pool of open-source AI models led by Meta and Mistral,
which perform similarly to the more popular and adopted closed-source models.
Aria's versatility also shines across various tasks. In the research paper, the
team explained how they fed the model with an entire financial report and it was
capable of performing an accurate analysis, it can extract data from reports,
calculate profit margins, and provide detailed breakdowns. When tasked with
weather data visualization, Aria not only extracted the relevant information but
also generated Python code to create graphs, complete with formatting details.
The model's video processing capabilities also seem promising. In one
evaluation, Aria dissected an hour-long video about Michelangelo's David,
identifying 19 distinct scenes with start and end times, titles, and
descriptions. This isn't simple keyword matching but a demonstration of
context-driven understanding. Coding is another area where Aria excels. It can
watch video tutorials, extract code snippets, and even debug them.
Preparing for IT failures in an unpredictable digital world
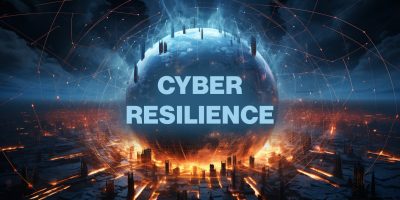
By embracing multiple vendors and hybrid cloud environments, organizations
would be better prepared so that if one platform goes down, the others can
pick up the slack. While this strategy increases ecosystem complexity, it buys
down the risk accepted by ensuring you’re prepared to recover and resilient to
widespread outages in complex, hybrid, and cloud-based environments. ... It’s
clear that IT failures aren’t just a possibility — they are inevitable. Simply
waiting for things to go wrong before reacting is a high-risk approach that’s
asking for trouble. Instead, organizations must go on the front foot and adopt
a strategy that focuses on early detection, continuous monitoring, and risk
prevention. This means planning for worst-case scenarios, but also preparing
for recovery. After all, one of the planks of IT infrastructure management is
business continuity. It’s about optimal performance when things are going well
while ensuring that systems recover quickly and continue operating even in the
face of major disruptions. This requires a holistic approach to IT management,
where failures are anticipated, and recovery plans are in place.
CIOs must adopt startup agility to compete with tech firms
CIOs often struggle with soft skills, despite knowing what needs to be done.
We engage with CEOs and CFOs to foster alignment among the leadership team, as
strong support from them is crucial. CIOs also need help gaining buy-in from
other CXOs, particularly when it comes to automation initiatives. Our approach
emphasises unlocking bandwidth within IT departments. If 90% of their
resources are spent on running the business, there’s little time for
innovation. We help them automate routine tasks, which allows their best
people to focus on transformative efforts. ... CIOs play a crucial role in
driving innovation and maintaining cost efficiency while justifying tech
investments, especially as organisations become digital-first. A key challenge
is controlling cloud costs, which often escalate as IT spending moves outside
central control. To counter this, CIOs should streamline access to central
services, reduce redundant purchases, and negotiate larger contracts for
better discounts. They must also recognise that cloud services are not always
cheaper; cost-efficiency depends on application types and usage.
AI makes edge computing more relevant to CIOs

Many user-facing situations could benefit from edge-based AI. Payton
emphasizes facial recognition technology, real-time traffic updates for
semi-autonomous vehicles, and data-driven enhancements on connected devices
and smartphones as possible areas. “In retail, AI can deliver personalized
experiences in real-time through smart devices,” she says. “In healthcare,
edge-based AI in wearables can alert medical professionals immediately when it
detects anomalies, potentially saving lives.” And a clear win for AI and edge
computing is within smart cities, says Bizagi’s Vázquez. There are numerous
ways AI models at the edge could help beyond simply controlling traffic
lights, he says, such as citizen safety, autonomous transportation, smart
grids, and self-healing infrastructures. To his point, experiments with AI are
already being carried out in cities such as Bahrain, Glasgow, and Las Vegas to
enhance urban planning, ease traffic flow, and aid public safety.
Self-administered, intelligent infrastructure is certainly top of mind for
Dairyland’s Melby since efforts within the energy industry are underway to use
AI to meet emission goals, transition into renewables, and increase the
resilience of the grid.
Deepfake detection is a continuous process of keeping up with AI-driven fraud: BioID
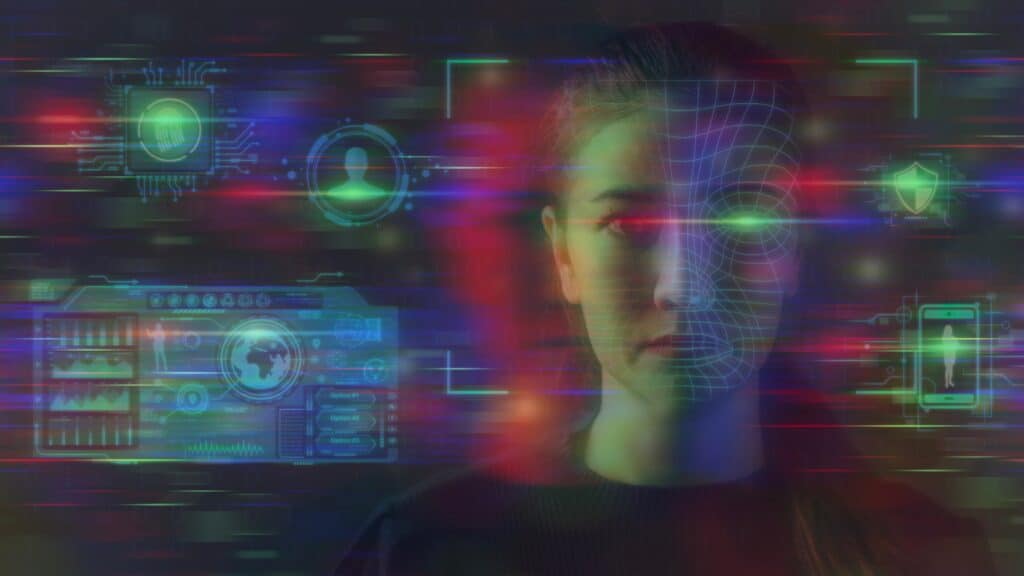
BioID is part of the growing ecosystem of firms offering algorithmic defenses
to algorithmic attacks. It provides an automated, real-time deepfake detection
tool for photos and videos that analyzes individual frames and video
sequences, looking for inter-frame or video codec anomalies. Its algorithm is
the product of a German research initiative that brought together a number of
institutions across sectors to collaborate on deepfake detection strategy. But
it is also continuing to refine its neural network to keep up with the
relentless pace of AI fraud. “We are in an ongoing fight of AI against AI,”
Freiberg says. “We can’t just just lean back and relax and sell what we have.
We’re continuously working on increasing the accuracy of our algorithms.” That
said, Freiberg is not only offering doom and gloom. She points to the
Ukrainian Ministry of Foreign Affairs AI ambassador, Victoria Shi, as an
example of deepfake technology used with non-fraudulent intention. The silver
lining is reflected in the branding of BioID’s “playground” for AI deepfake
testing. At playground.bioid.com, users can upload media to have BioID judge
whether or not it is genuine.
How Manufacturing Best Practices Shape Software Development

Manufacturers rely on bills of materials (BOMs) to track every component in
their products. This transparency enables them to swiftly pinpoint the source
of any issues that arise, ensuring they have a comprehensive understanding of
their supply chain. In software, this same principle is applied through
software bills of materials (SBOMs), which list all the components,
dependencies and licenses used in a software application. SBOMs are
increasingly becoming critical resources for managing software supply chains,
enabling developers and security teams to maintain visibility over what’s
being used in their applications. Without an SBOM, organizations risk being
unaware of outdated or vulnerable components in their software, making it
difficult to address security issues. ... It’s nearly impossible to monitor
open source components manually at scale. But with software composition
analysis, developers can automate the process of identifying security risks
and ensuring compliance. Automation not only accelerates development but also
reduces the risk of human error, so teams can manage vast numbers of
components and dependencies efficiently.
Striking The Right Balance Between AI & Innovation & Evolving Regulation
The bottom line is that integrating AI comes with complex challenges to how an
organisation approaches data privacy. A significant part of this challenge
relates to purpose limitation – specifically, the disclosure provided to
consumers regarding the purpose(s) for data processing and the consent
obtained. To tackle this hurdle, it’s vital that organisations maintain a high
level of transparency that discloses to users and consumers how the use of
their data is evolving as AI is integrated. ... Just as the technology
landscape has evolved, so have consumer expectations. Today, consumers are
more conscious of and concerned with how their data is used. Adding to this,
nearly two-thirds of consumers worry about AI systems lacking human oversight,
and 93% believe irresponsible AI practices damage company reputations. As
such, it’s vital that organisations are continuously working to maintain
consumer trust as part of their AI strategy. With this said, there are many
consumers who are willing to share their data as long as they receive a better
personalised customer experience, showcasing that this is a nuanced landscape
that requires attention and balance.
WasmGC and the future of front-end Java development

The approach being offered by the WasmGC extension is newer. The extension
provides a generic garbage collection layer that your software can refer to; a
kind of garbage collection layer built into WebAssembly. Wasm by itself
doesn’t track references to variables and data structures, so the addition of
garbage collection also implies introducing new “typed references” into the
specification. This effort is happening gradually: recent implementations
support garbage collection on “linear” reference types like integers, but
complex types like objects and structs have also been added. ... The
performance potential of languages like Java over JavaScript is a key
motivation for WasmGC, but obviously there’s also the enormous range of
available functionality and styles among garbage-collected platforms. The
possibility for moving custom code into Wasm, and thereby making it
universally deployable, including to the browser, is there. More broadly, one
can’t help but wonder about the possibility of opening up the browser to other
languages beyond JavaScript, which could spark a real sea-change to the
software industry. It’s possible that loosening JavaScript’s monopoly on the
browser will instigate a renaissance of creativity in programming
languages.
Mind Your Language Models: An Approach to Architecting Intelligent Systems
The reason why we wanted a smaller model that's adapted to a certain task is,
it's easier to operate, and when you're running LLMs, it's going to be much
economical, because you can't run massive models all the time because it's
very expensive and takes a lot of GPUs. Currently, we're struggling with
getting GPUs in AWS. We searched all EU Frankfurt, Ireland, North Virginia.
It's seriously a challenge now to get big GPUs to host your LLMs. The second
part of the problem is, we started getting data. It's high quality. We started
improving the knowledge graph. The one thing that is interesting when you
think about semantic search is that when people interact with your system,
even if they're working on the same problem, they don't end up using the same
language. Which means that you need to be able to translate or understand the
range of language that your users can actually interact with your system. ...
We converted these facts with all of their synonyms, with all of the different
ways one could potentially ask for this piece of data, and put everything into
the knowledge graph itself. You could use LLMs to generate training data for
your smaller models.
Quote for the day:
"You may only succeed if you desire
succeeding; you may only fail if you do not mind failing." --
Philippos
No comments:
Post a Comment