Beyond the breach: How cloud ransomware is redefining cyber threats in 2024
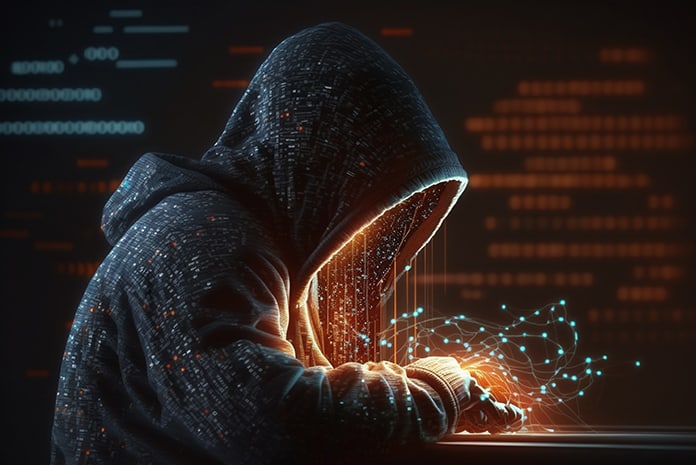
Unlike conventional ransomware that targets individual computers or on-premises
servers, attackers are now setting their sights on cloud infrastructures that
host vast amounts of data and critical services. This evolution represents a new
frontier in cyber threats, requiring Indian cybersecurity practitioners to
rethink and relearn defence strategies. Traditional security measures and last
year’s playbooks are no longer sufficient. Attackers are exploiting
misconfigured or poorly secured cloud storage platforms such as Amazon Web
Services (AWS) Simple Storage Service (S3) and Microsoft Azure Blob Storage. By
identifying cloud storage buckets with overly permissive access controls,
cybercriminals gain unauthorised entry, copy data to their own servers, encrypt
or delete the original files, and then demand a ransom for their
return. ... Collaboration and adaptability are essential. By understanding
the unique challenges posed by cloud security, Indian organisations can
implement comprehensive strategies that not only protect against current threats
but also anticipate future ones. Proactive measures—such as strengthening access
controls, adopting advanced threat detection technologies, training employees,
and staying informed—are crucial steps in defending against these evolving
attacks.
Harnessing AI’s Potential to Transform Payment Processing
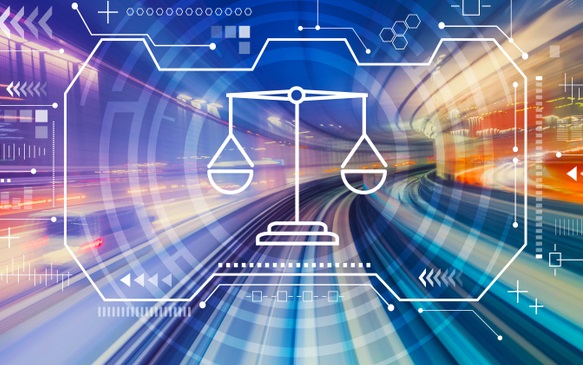
There are many use cases that show how AI increases the speed and convenience
of payment processing. For instance, Apple Pay now offers biometric
authentication, which uses AI facial recognition and fingerprint scanning to
authenticate users. This enables mobile payment customers to use quick and
secure authentication without remembering passwords or PINs. Similarly, Apple
Pay’s competitor, PayPal, uses AI for real-time fraud detection, employing ML
algorithms to monitor transactions for signs of fraud and ensure that
customers’ financial information remains secure. ... One issue is AI systems
rely on massive amounts of data, including sensitive data, which can lead to
data breaches, identity theft, and compliance issues. In addition, AI
algorithms trained on biased data can perpetuate those biases. Making matters
worse, many AI systems lack transparency, so the bias may grow and lead to
unequal access to financial services. Another issue is the potential
dependence on outside vendors, which is common with many AI technologies. ...
To reduce the current risks associated with AI and safely unleash its full
potential to improve payment processing, it is imperative for organizations to
take a multi-layered approach that includes technical safeguards,
organizational policies, and regulatory compliance.
Do you need an AI ethicist?

The goal of advising on ethics is not to create a service desk model, where
colleagues or clients always have to come back to the ethicist for additional
guidance. Ethicists generally aim for their stakeholders to achieve some level
of independence. “We really want to make our partners self-sufficient. We want
to teach them to do this work on their own,” Sample said. Ethicists can
promote ethics as a core company value, no different from teamwork, agility,
or innovation. Key to this transformation is an understanding of the
organization’s goal in implementing AI. “If we believe that artificial
intelligence is going to transform business models…then it becomes incumbent
on an organization to make sure that the senior executives and the board never
become disconnected from what AI is doing for or to their organization,
workforce, or customers,” Menachemson said. This alignment may be especially
necessary in an environment where companies are diving head-first into AI
without any clear strategic direction, simply because the technology is in
vogue. A dedicated ethicist or team could address one of the most foundational
issues surrounding AI, notes Gartner’s Willemsen. One of the most frequently
asked questions at a board level, regardless of the project at hand, is
whether the company can use AI for it, he said.
Why We Need Inclusive Data Governance in the Age of AI
Inclusive data governance processes involve multiple stakeholders, giving
equal space in this decision making to diverse groups from civil society, as
well as space for direct representation of affected communities as active
stakeholders. This links to, but is an idea broader than, the concept of
multi-stakeholder governance for technology, which first came to prominence at
the international level, in institutions such as the Internet Corporation for
Assigned Names and Numbers and the Internet Governance Forum. ... Involving
the public and civil society in decisions about data is not cost-free. Taking
the steps that are needed to surmount the practical challenges, and skepticism
about the utility of public involvement in a technical and technocratic field,
frequently requires arguments that go beyond it being the right thing to do.
... The risks for people, communities and society, but also for organizations
operating within the data and AI marketplace and supply chain, can be reduced
through greater inclusion earlier in the design process. But organizational
self-interest will not motivate the scope or depth that is required. Reducing
the reality and perception of “participation-washing” means requirements for
consultation in the design of data and AI systems need to be robust and
enforceable.
Strategies to navigate the pitfalls of cloud costs

If cloud customers spend too much money, it’s usually because they created
cost-ineffective deployments. It’s common knowledge that many enterprises
“lifted and shifted” their way to the clouds with little thought about how
inefficient those systems would be in the new infrastructure. ... Purposely or
not, public cloud providers created intricate pricing structures that are
nearly incomprehensible to anyone who does not spend each day creating cloud
pricing structures to cover every possible use. As a result, enterprises often
face unexpected expenses. Many of my clients frequently complain that they
have no idea how to manage their cloud bills because they don’t know what
they’re paying for. ... Cloud providers often encourage enterprises to
overprovision resources “just in case.” Enterprises still pay for that unused
capacity, so the misalignment dramatically elevates costs without adding
business value. When I ask my clients why they provision so much more storage
or computing resources beyond what their workload requires, the most common
answer is, “My cloud provider told me to.” ... One of the best features of
public cloud computing is autoscaling so you’ll never run out of resources or
suffer from bad performance due to insufficient resource provisioning.
However, autoscaling often leads to colossal cloud bills because it often is
triggered without good governance or purpose.
Your IT Team Isn't Ready For Change Management If They Can't Answer These 3 Questions
Testing software before you encounter failure rates is key, but never should
you be exposed to failure rates with this level of real world impact. Whether
it’s due to third party systems or the companies themselves, their brand will
be the one in tatters due to the end customer experience. Enter Change
Management and the possibility for, if done right, the prevention of these
kinds of enormous IT failures. ... The ever-evolving nature of technology,
including cloud scaling, infrastructure as code, and frequent updates such as
‘Patch Tuesday’ means that organisations must constantly adapt to change.
However, this constant change introduces challenges such as “drift”—a term
that refers to the unplanned deviations from standard configurations or
expected states within an IT environment. Think of it like a pesky monkey in
the machine. Drift can occur subtly and often goes unnoticed until it causes
significant disruptions. It also increases uncertainty and doubt in the
organisation making Change Management and Release Management harder, creating
difficulties to plan and execute changes safely. ... To be effective, Change
Management needs to be able to detect and understand drift in the environment
to have a full understanding of Current State, Risk Assessment and Expected
Outcomes.
RIP Open Core — Long Live Open Source

Open-core was originally popular because it allowed companies to build a
community around a free product version while charging for a more full,
enterprise-grade version. This setup thrived in the 2010s, helping companies
like MongoDB and Redis gain traction. But times have changed, and today,
instead of enhancing a company’s standing, open-core models often create more
problems than they solve. ... While open-core and source-available models had
their moment, companies are beginning to realize the importance of true open
source values and are finding their way back. This return to open source is a
sign of growth, with businesses realigning with the collaborative spirit at
the core (wink) of the OSS community. More companies are adopting models that
genuinely prioritize community engagement and transparency rather than using
them as marketing or growth tactics. ... As the open-core model fades, we’re
seeing a more sustainable approach take shape: the Open-Foundation model. This
model allows the open-source offering to be the backbone of a commercial
offering without compromising the integrity of the OSS project. Rather, it
reinforces it as a valuable, standalone product that supports the commercial
offering instead of competing against it.
Why SaaS Backup Matters: Protecting Data Beyond Vendor Guarantees
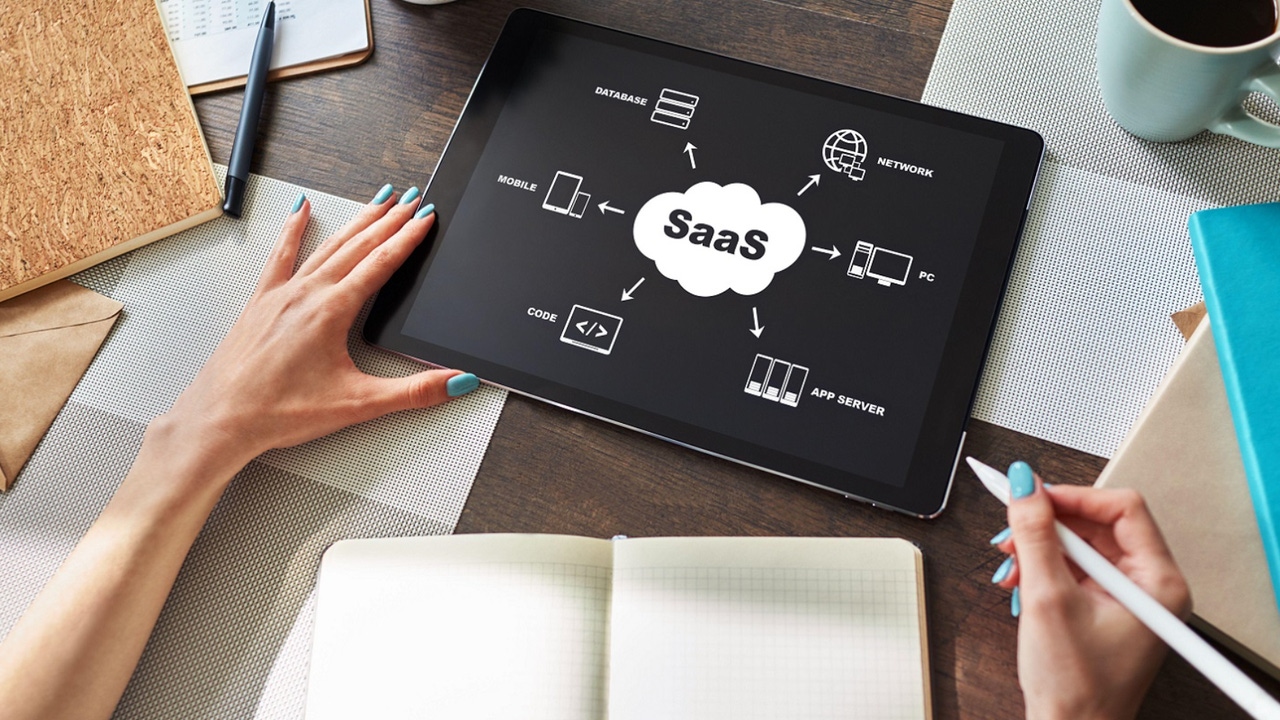
Most IT departments have long recognized the importance of backup and recovery
for applications and data that they host themselves. When no one else is
managing your workloads and backing them up, having a recovery plan to restore
them if necessary is essential for minimizing the risk that a failure could
disrupt business operations. But when it comes to SaaS, IT operations teams
sometimes think in different terms. That's because SaaS applications are
hosted and managed by external vendors, not the IT departments of the
businesses that use SaaS apps. In many cases, SaaS vendors provide uptime or
availability guarantees. They don't typically offer details about exactly how
they back up applications and data or how they'll recover data in the event of
a failure, but a backup guarantee is typically implicit in SaaS products. ...
Historically, SaaS apps haven't featured prominently, if at all, in backup and
recovery strategies. But the growing reliance on SaaS apps — combined with the
many risks that can befall SaaS application data even if the SaaS vendor
provides its own backup or availability guarantees — makes it critical to
integrate SaaS apps into backup and recovery plans. The risks of not backing
up SaaS have simply become too great.
Biometrics in the Cyber World
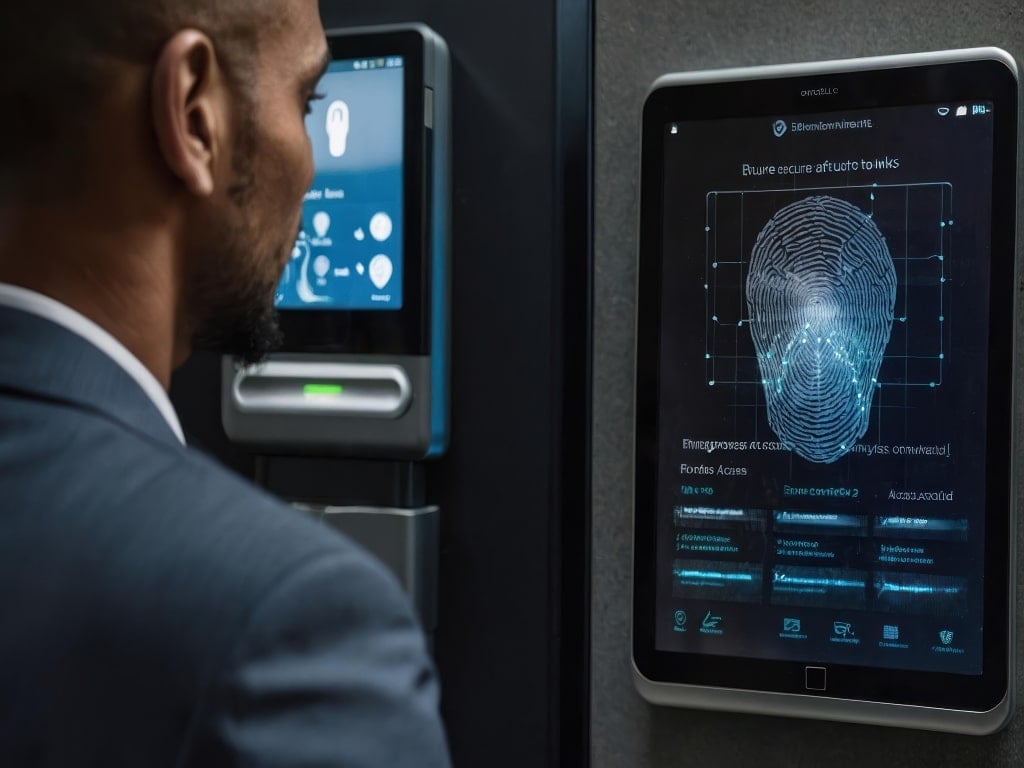
Biometrics is known to strengthen security in many ways. Some ways this could
include stronger authentication, user convenience, and reduction of risk of
identity theft. With the uniqueness of the user, this adds a layer of security
to authentication. Traditional authentication, such as passwords, contains
weak combinations that can easily be breached, so using biometrics can prevent
that. People constantly forget their passwords and always end up resetting
them. Since biometrics is entirely connected with one’s identity, it will no
longer be an inconvenience to forget a password. Since it is very usual for
hackers to attempt to get into an account by guessing the password, biometrics
does not accommodate this since a hacker cannot guess a password when the
uniqueness of one’s identity is what is sought for authentication. This is one
of the pros of biometric systems, which should reduce the risk of identity
theft. Some challenges that biometrics could have include privacy concerns,
false positives and negatives, and bias. Since it is personal information,
biometric data can lead to privacy violations. Storage of personal data, which
is sensitive, needs to be following the regulations on privacy such as GDPR
and CCPA.
Data Architectures in the AI Era: Key Strategies and Insights
Accelerating results in data architecture initiatives can be achieved in a
much quicker fashion if you start with the minimum needed and build from there
for your data storage. Begin by considering all use cases and finding the one
component needed to develop so a data product can be delivered. Expansion can
happen over time with use and feedback, which will actually create a more
tailored and desirable product. ... Educating your key personnel on the
importance of being able and ready to make the shift from previously familiar
legacy data systems to modern architectures like data lakehouses or hybrid
cloud platforms. Migration to a unified, hybrid, or cloud-based data
management system may seem challenging initially, but it is essential for
enabling comprehensive data lifecycle management and AI-readiness. By
investing in continuous education and training, organizations can enhance data
literacy, simplify processes, and improve long-term data governance,
positioning themselves for scalable and secure analytics practices. ... By
being prepared for the typical challenges of AI, problems can be predicted and
anticipated which can help to reduce downtime and frustration in the
modernization of data architecture.
Quote for the day:
“The final test of a leader is that he
leaves behind him in other men the conviction and the will to carry on.” –
Walter Lippmann