Quote for the day:
"Whatever you can do, or dream you can, begin it. Boldness has genius,
power and magic in it." -- Johann Wolfgang von Goethe
Revolutionizing Application Security: The Plea for Unified Platforms

“Shift left” is a practice that focuses on addressing security risks earlier
in the development cycle, before deployment. While effective in theory, this
approach has proven problematic in practice as developers and security teams
have conflicting priorities. ... Cloud native applications are dynamic;
constantly deployed, updated and scaled, so robust real-time protection
measures are absolutely necessary. Every time an application is updated or
deployed, new code, configurations or dependencies appear, all of which can
introduce new vulnerabilities. The problem is that it is difficult to
implement real-time cloud security with a traditional, compartmentalized
approach. Organizations need real-time security measures that provide
continuous monitoring across the entire infrastructure, detect threats as they
emerge and automatically respond to them. As Tager explained, implementing
real-time prevention is necessary “to stay ahead of the pace of attackers.”
... Cloud native applications tend to rely heavily on open source libraries
and third-party components. In 2021, Log4j’s Log4Shell vulnerability
demonstrated how a single compromised component could affect millions of
devices worldwide, exposing countless enterprises to risk. Effective
application security now extends far beyond the traditional scope of code
scanning and must reflect the modern engineering environment.
AI-Powered Polymorphic Phishing Is Changing the Threat Landscape
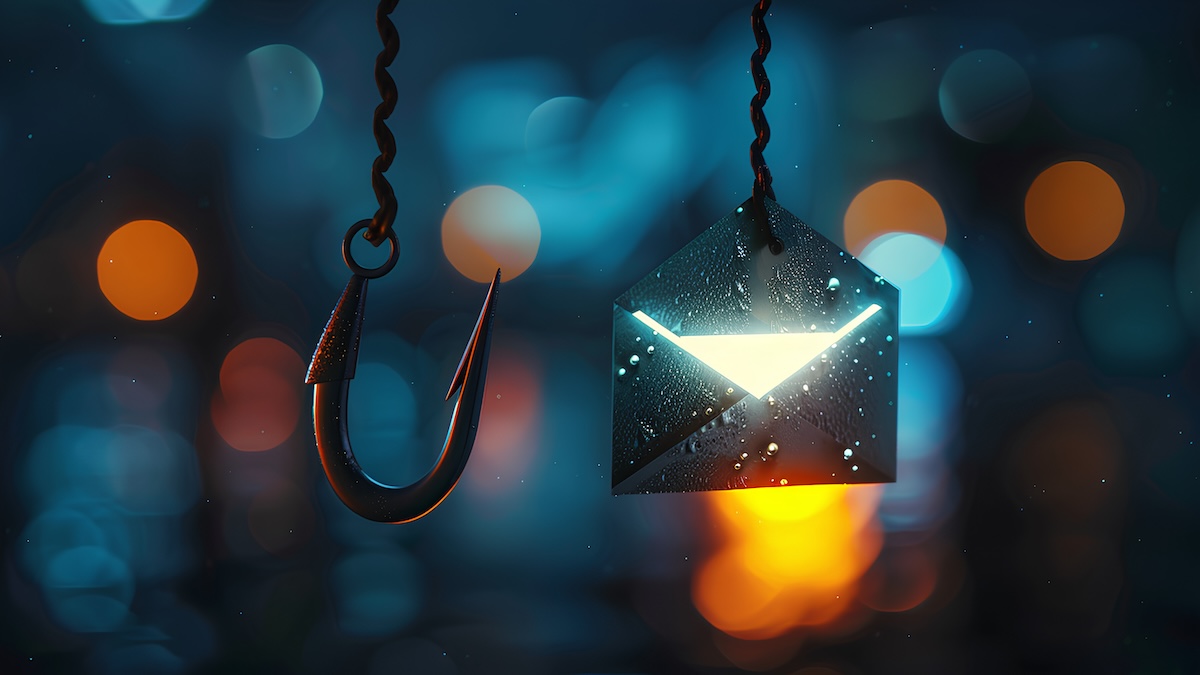
Polymorphic phishing is an advanced form of phishing campaign that randomizes
the components of emails, such as their content, subject lines, and senders’
display names, to create several almost identical emails that only differ by a
minor detail. In combination with AI, polymorphic phishing emails have become
highly sophisticated, creating more personalized and evasive messages that
result in higher attack success rates. ... Traditional detection systems group
phishing emails together to enhance their detection efficacy based on
commonalities in phishing emails, such as payloads or senders’ domain names.
The use of AI by cybercriminals has allowed them to conduct polymorphic
phishing campaigns with subtle but deceptive variations that can evade
security measures like blocklists, static signatures, secure email gateways
(SEGs), and native security tools. For example, cybercriminals modify the
subject line by adding extra characters and symbols, or they can alter the
length and pattern of the text. ... The standard way of grouping
individual attacks into campaigns to improve detection efficacy will become
irrelevant by 2027. Organizations need to find alternative measures to detect
polymorphic phishing campaigns that don’t rely on blocklists and that can
identify the most advanced attacks.
Does AI Deserve Worker Rights?
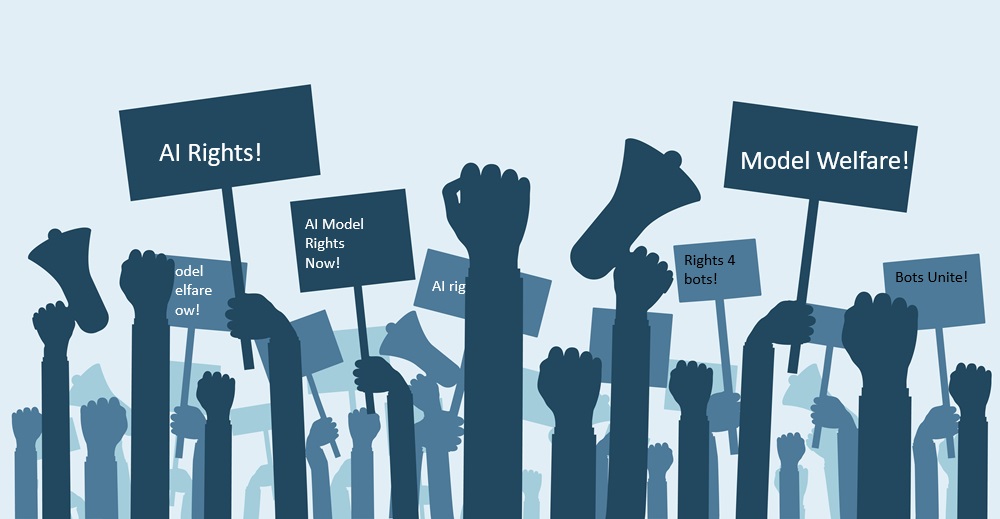
Chalmers et al declare that there are three things that AI-adopting
institutions can do to prepare for the coming consciousness of AI: “They can
(1) acknowledge that AI welfare is an important and difficult issue (and
ensure that language model outputs do the same), (2) start assessing AI
systems for evidence of consciousness and robust agency, and (3) prepare
policies and procedures for treating AI systems with an appropriate level of
moral concern.” What would “an appropriate level of moral concern” actually
look like? According to Kyle Fish, Anthropic’s AI welfare researcher, it could
take the form of allowing an AI model to stop a conversation with a human if
the conversation turned abusive. “If a user is persistently requesting harmful
content despite the model’s refusals and attempts at redirection, could we
allow the model simply to end that interaction?” Fish told the New York Times
in an interview. What exactly would model welfare entail? The Times cites a
comment made in a podcast last week by podcaster Dwarkesh Patel, who compared
model welfare to animal welfare, stating it was important to make sure we
don’t reach “the digital equivalent of factory farming” with AI. Considering
Nvidia CEO Jensen Huang’s desire to create giant “AI factories” filled with
millions of his company’s GPUs cranking through GenAI and agentic AI
workflows, perhaps the factory analogy is apropos.
Cybercriminals switch up their top initial access vectors of choice

“Organizations must leverage a risk-based approach and prioritize
vulnerability scanning and patching for internet-facing systems,” wrote Saeed
Abbasi, threat research manager at cloud security firm Qualys, in a blog post.
“The data clearly shows that attackers follow the path of least resistance,
targeting vulnerable edge devices that provide direct access to internal
networks.” Greg Linares, principal threat intelligence analyst at managed
detection and response vendor Huntress, said, “We’re seeing a distinct shift
in how modern attackers breach enterprise environments, and one of the most
consistent trends right now is the exploitation of edge devices.” Edge
devices, ranging from firewalls and VPN appliances to load balancers and IoT
gateways, serve as the gateway between internal networks and the broader
internet. “Because they operate at this critical boundary, they often hold
elevated privileges and have broad visibility into internal systems,” Linares
noted, adding that edge devices are often poorly maintained and not integrated
into standard patching cycles. Linares explained: “Many edge devices come with
default credentials, exposed management ports, secret superuser accounts, or
weakly configured services that still rely on legacy protocols — these are all
conditions that invite intrusion.”
5 tips for transforming company data into new revenue streams

Data monetization can be risky, particularly for organizations that aren’t
accustomed to handling financial transactions. There’s an increased threat of
security breaches as other parties become aware that you’re in possession of
valuable information, ISG’s Rudy says. Another risk is unintentionally using
data you don’t have a right to use or discovering that the data you want to
monetize is of poor quality or doesn’t integrate across data sets. Ultimately,
the biggest risk is that no one wants to buy what you’re selling. Strong
security is essential, Agility Writer’s Yong says. “If you’re not careful, you
could end up facing big fines for mishandling data or not getting the right
consent from users,” he cautions. If a data breach occurs, it can deeply
damage an enterprise’s reputation. “Keeping your data safe and being
transparent with users about how you use their info can go a long way in
avoiding these costly mistakes.” ... “Data-as-a-service, where companies
compile and package valuable datasets, is the base model for monetizing data,”
he notes. However, insights-as-a-service, where customers provide
prescriptive/predictive modeling capabilities, can demand a higher valuation.
Another consideration is offering an insights platform-as-a-service, where
subscribers can securely integrate their data into the provider’s insights
platform.
Are AI Startups Faking It Till They Make It?
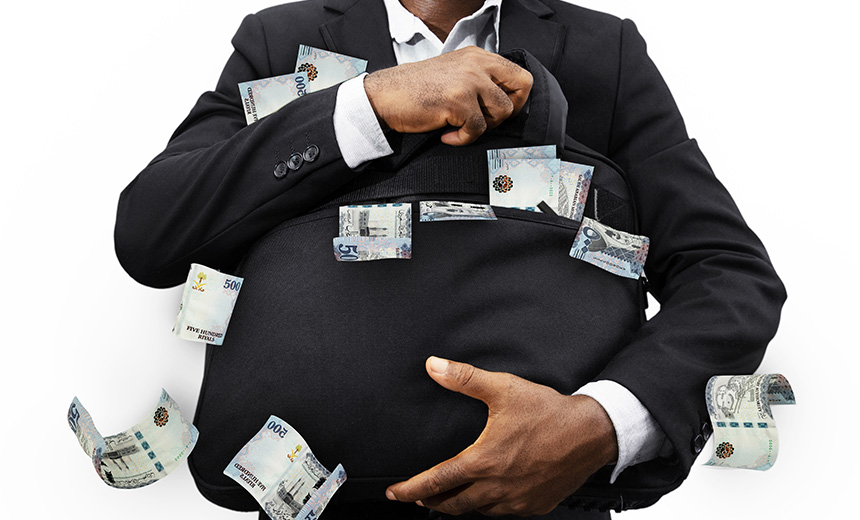
"A lot of VC funds are just kind of saying, 'Hey, this can only go up.' And
that's usually a recipe for failure - when that starts to happen, you're
becoming detached from reality," Nnamdi Okike, co-founder and managing partner
at 645 Ventures, told Tradingview. Companies are branding themselves as
AI-driven, even when their core technologies lack substantive AI components. A
2019 study by MMC Ventures found 40% of surveyed "AI startups" in Europe
showed no evidence of AI integration in their products or services. And this
was before OpenAI further raised the stakes with the launch of ChatGPT in
2022. It's a slippery slope. Even industry behemoths have had to clarify the
extent of their AI involvement. Last year, tech giant and the fourth-most
richest company in the world Amazon pushed back on allegations that its
AI-powered "Just Walk Out" technology installed at its physical grocery stores
for a cashierless checkout was largely being driven by around 1,000 workers in
India who manually checked almost three quarters of the transactions. Amazon
termed these reports "erroneous" and "untrue," adding that the staff in India
were not reviewing live footage from the stores but simply reviewing the
system. The incentive to brand as AI-native has only intensified.
From deployment to optimisation: Why cloud management needs a smarter approach
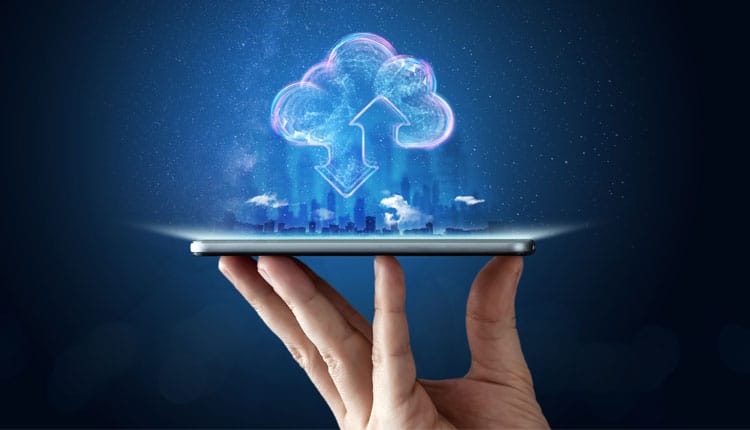
As companies grow, so does their cloud footprint. Managing multiple cloud
environments—across AWS, Azure, and GCP—often results in fragmented policies,
security gaps, and operational inefficiencies. A Multi-Cloud Maturity Research
Report by Vanson Bourne states that nearly 70% of organisations struggle with
multi-cloud complexity, despite 95% agreeing that multi-cloud architectures
are critical for success. Companies are shifting away from monolithic
architecture to microservices, but managing distributed services at scale
remains challenging. ... Regulatory requirements like SOC 2, HIPAA, and
GDPR demand continuous monitoring and updates. The challenge is not just
staying compliant but ensuring that security configurations remain airtight.
IBM’s Cost of a Data Breach Report reveals that the average cost of a data
breach in India reached ₹195 million in 2024, with cloud misconfiguration
accounting for 12% of breaches. The risk is twofold: businesses either
overprovision resources—wasting money—or leave environments under-secured,
exposing them to breaches. Cyber threats are also evolving, with attackers
increasingly targeting cloud environments. Phishing and credential theft
accounted for 18% of incidents each, according to the IBM report.
Inside a Cyberattack: How Hackers Steal Data
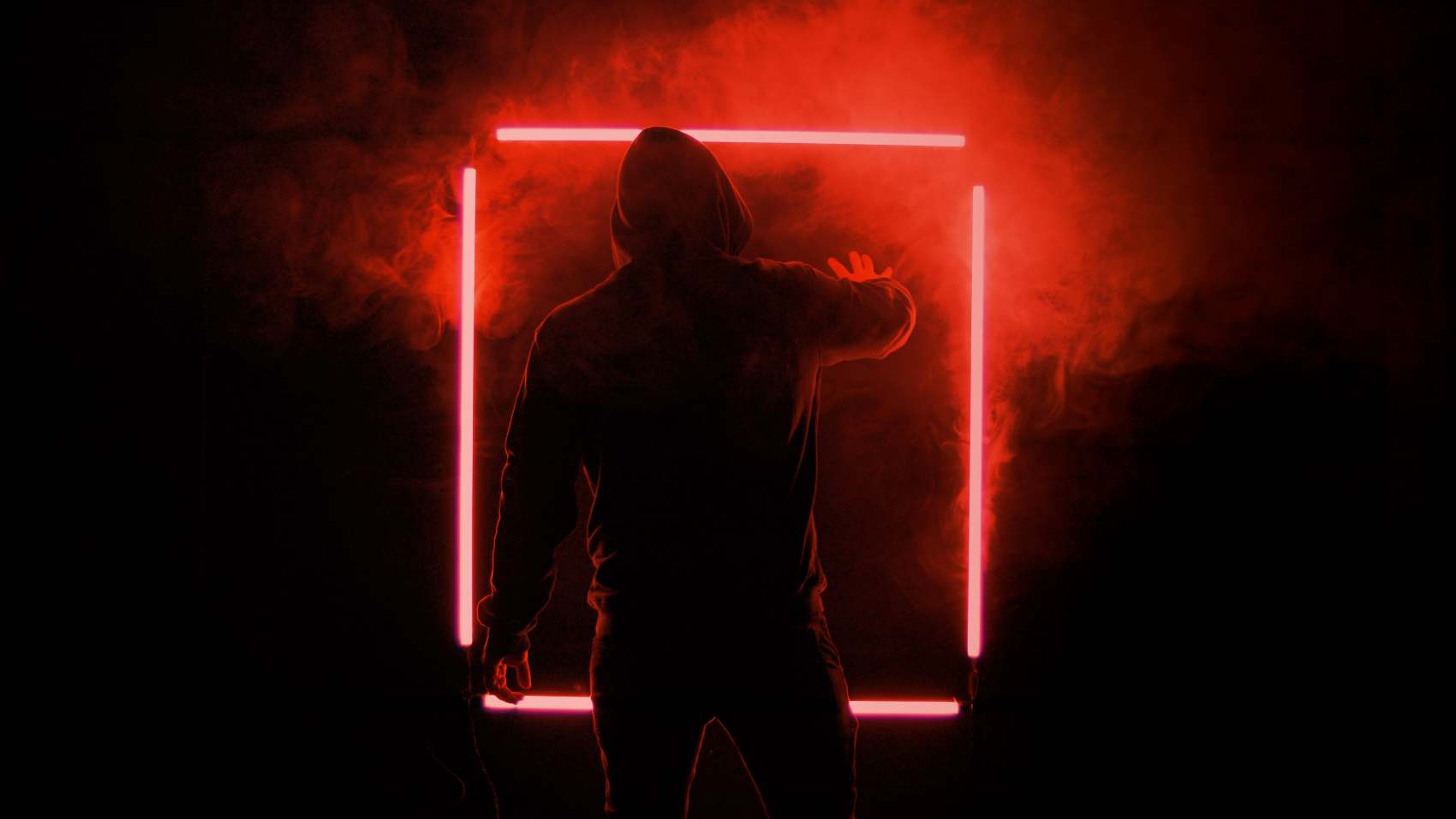
Once a hacker breaches the perimeter the standard practice is to beachhead, and
then move laterally to find the organisation’s crown jewels: their most valuable
data. Within a financial or banking organisation it is likely there is a
database on their server that contains sensitive customer information. A
database is essentially a complicated spreadsheet, wherein a hacker can simply
click SELECT and copy everything. In this instance data security is essential,
however, many organisations confuse data security with cybersecurity.
Organisations often rely on encryption to protect sensitive data, but encryption
alone isn’t enough if the decryption keys are poorly managed. If an attacker
gains access to the decryption key, they can instantly decrypt the data,
rendering the encryption useless. ... To truly safeguard data, businesses must
combine strong encryption with secure key management, access controls, and
techniques like tokenisation or format-preserving encryption to minimise the
impact of a breach. A database protected by Privacy Enhancing Technologies
(PETs), such as tokenisation, becomes unreadable to hackers if the decryption
key is stored offsite. Without breaching the organisation’s data protection
vendor to access the key, an attacker cannot decrypt the data – making the
process significantly more complicated. This can be a major deterrent to
hackers.
Why Testing is a Long-Term Investment for Software Engineers
At its core, a test is a contract. It tells the system—and anyone reading the
code—what should happen when given specific inputs. This contract helps ensure
that as the software evolves, its expected behavior remains intact. A system
without tests is like a building without smoke detectors. Sure, it might stand
fine for now, but the moment something catches fire, there’s no safety mechanism
to contain the damage. ... Over time, all code becomes legacy. Business
requirements shift, architectures evolve, and what once worked becomes outdated.
That’s why refactoring is not a luxury—it’s a necessity. But refactoring without
tests? That’s walking blindfolded through a minefield. With a reliable test
suite, engineers can reshape and improve their code with confidence. Tests
confirm that behavior hasn’t changed—even as the internal structure is
optimized. This is why tests are essential not just for correctness, but for
sustainable growth. ... There’s a common myth: tests slow you down. But seasoned
engineers know the opposite is true. Tests speed up development by reducing time
spent debugging, catching regressions early, and removing the need for manual
verification after every change. They also allow teams to work independently,
since tests define and validate interfaces between components.
Why the road from passwords to passkeys is long, bumpy, and worth it - probably

While the current plan rests on a solid technical foundation, many important
details are barriers to short-term adoption. For example, setting up a passkey
for a particular website should be a rather seamless process; however, fully
deactivating that passkey still relies on a manual multistep process that has
yet to be automated. Further complicating matters, some current user-facing
implementations of passkeys are so different from one another that they're
likely to confuse end-users looking for a common, recognizable, and easily
repeated user experience. ... Passkey proponents talk about how passkeys will be
the death of the password. However, the truth is that the password died long ago
-- just in a different way. We've all used passwords without considering what is
happening behind the scenes. A password is a special kind of secret -- a shared
or symmetric secret. For most online services and applications, setting a
password requires us to first share that password with the relying party, the
website or app operator. While history has proven how shared secrets can work
well in very secure and often temporary contexts, if the HaveIBeenPawned.com
website teaches us anything, it's that site and app authentication isn't one of
those contexts. Passwords are too easily compromised.