Is The Business World Ready For A Chief Data Ethics Officer?
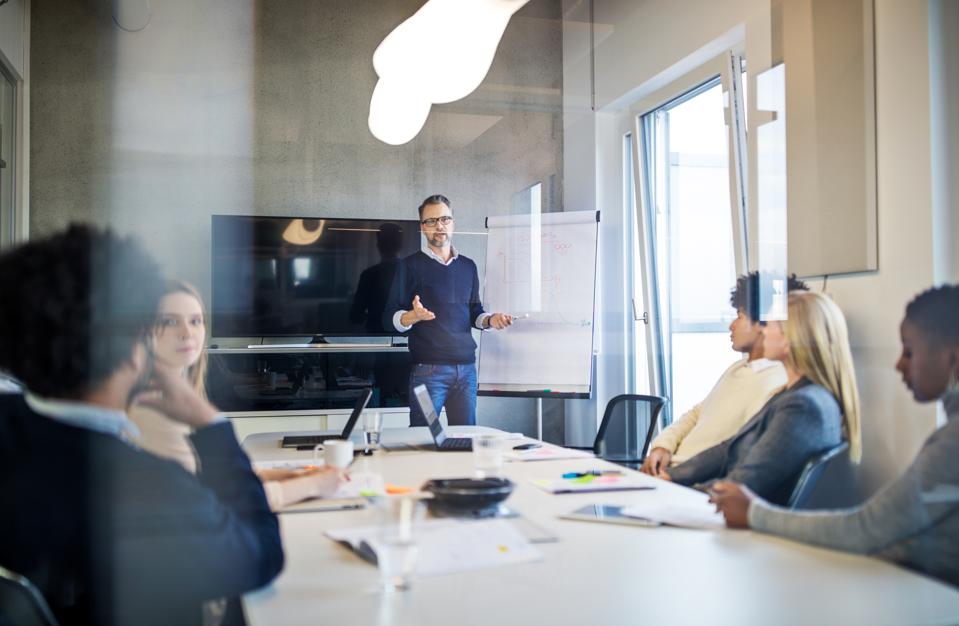
Data ethics is the new strategic imperative for leading corporations. In
NewVantage Partners 2019 Executive Survey, more than half of executives —
55.7% — pointed to data ethics as a top business priority. In a recent
Harvard Business Review article, Tom Davenport and I discussed the “seven key
types of Chief Data Officer (CDO) jobs”, noting that one of the emerging roles
of the Chief Data Officer is as Data Ethicist. We noted that CDO as Data
Ethicist is “growing in popularity, is [focused] on the ethics of data
management, specifically on how it’s collected, safeguarded and shared and who
controls it”. We confirmed that, “there is no doubt that consumers,
regulators, and legislators are becoming more concerned about the misuse of
data”. I spoke recently with Dennis Hirsch, Professor of Law and Director of
the Program on Data and Governance at The Ohio State University Moritz College
of Law, and research fellow at The Risk Institute, about data ethics and
corporate responsibility in the context of today’s emerging algorithmic
economy. Hirsch’s research is focused on how data analytics can pose ethical
risks, and how leading companies are responding.
SD-WAN and analytics: A marriage made for the new normal
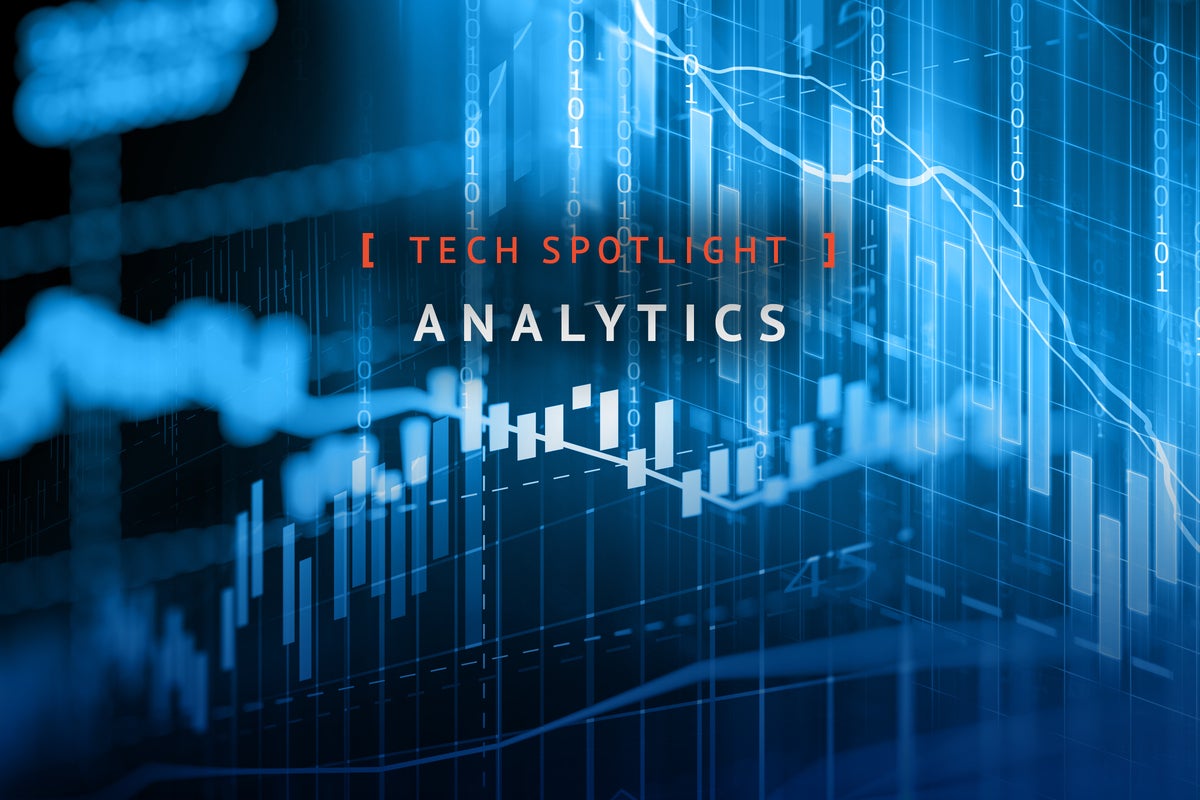
“With the rapid increase in use of cloud services including video and IoT
applications, which have only been accelerated with the ongoing global
pandemic, wide area networking and remote connectivity stays a mission
critical need for enterprise IT,” said Mehra. “Specifically, SD-WAN
emerged as an evolution from enterprise routing and WAN optimization to
address the needs of a more dynamic, intelligent architecture around these
evolving application needs,” Mehra said. Probes or agents in vendors’ SD-WAN
packages gather network, performance, security and other telemetry and combine
it with historic customer and vendor-gathered data. Analysis of this data
generates recommendations, policy changes or other actions to help IT keep the
overall WAN environment humming. Analytics programs can also reduce the number
of overall alerts IT teams deal with because the programs can focus on those
things enterprises consider most important. Vendors such as Cisco, VMware,
Versa, Silver Peak, Citrix, Cato and others have varying degrees of analytic
sophistication in their SD-WAN packages, but all of them are marching toward
supporting cloud-connected customers.
How enterprise IoT will evolve in a post-COVID world
With wired power and solar power both encumbered by significant drawbacks,
businesses have been searching for a new solution: long-range wireless
charging. Long-range wireless charging devices use infrared light or other
physical phenomena to deliver power at a distance. Facility managers need only
install the transmitter in a convenient area and then plug in or embed the
receiver, which is roughly the size of a thumb drive, into their IoT device of
choice. Long-range wireless charging can be installed from the ground up and
that’s often the case when it comes to new construction. The technology is
already being widely adapted. However, long-range wireless charging can often
be retrofitted into operations that are already in place. This is especially
important in light of the rapid changes enterprise-level businesses are
expected to make due to the COVID-19 pandemic. IT specialists are looking for
practical and flexible solutions that can enable them to resume operations
safely. It should be noted, of course, that for all its benefits, long-range
wireless charging may not be the solution for every business, just as IoT
won’t solve every COVID-19-related challenge. Long-range wireless charging
might have limitations in power delivery, range or other aspects.
Drive to improve flash reliability
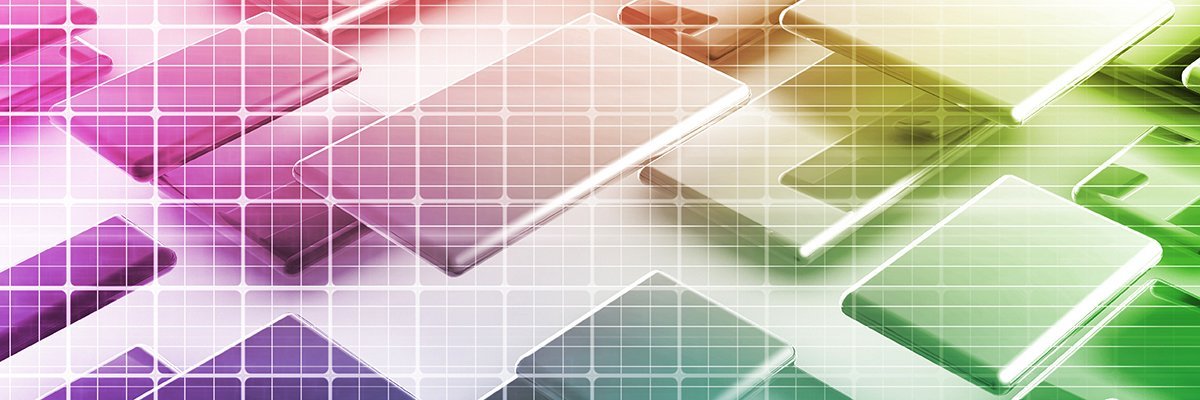
Originally, Nand flash was laid out in a plane, with each cell supporting two
levels or a single bit, writes Alex McDonald, chair, SNIA EMEA. This is a
single-level cell (SLC). Now we have progressed through MLC (four levels and
two bits), through TLC (eight levels and three bits) up to 16 levels and four
bits per cell (QLC), and improved densities by “building up” with a 3D stack
of cells. The unit of write is not a single cell, but a page of cells of
around 4KB. At a higher level, several tens of pages are organised into
blocks. Prior to writing to pages, a block needs to be erased in preparation –
program/erase (P/E) cycles. There are only so many P/E cycles that the flash
can tolerate before failing. The advantages of QLC and 3D-based technology is
their ability to build very high-density devices, with 32TB devices relatively
commonplace. Generally, with more bits per cell, fewer P/E cycles can be
performed. Interestingly, these limitations have not necessarily reduced the
practical reliability of high-density Nand flash SSDs, because there are many
techniques that are used to mitigate the possibly unreliable operation of
single cells.
5 reasons AI isn't being adopted at your organization

It's important to remember that AI solutions are built by imperfect humans.
We've seen examples of models that unintentionally generate discriminatory
outcomes because the underlying data was skewed towards a particular segment
of the population. Whether they resulted from bias in the dataset (e.g.,
exclusion or sample bias) or from humans' unconscious biases, these outcomes
rightly erode trust in the technology and slow adoption. We must balance
freedom, ethics and privacy with efficiency and other benefits AI makes
possible. This foundation for AI requires that people at all levels of an
organization understand their role in building a governance structure. A
strong governance system includes a set of ethical design and development
principles that are regularly reviewed, creating a "feedback loop." It's
important to consider these three points when developing a governance
framework for AI: 1. Prioritize ethics early. 2. Build robust, transparent,
and explainable systems that clearly yield an audit trail with the
understanding as the models learn these can adjust. 3. Ensure measured,
monitored roll-outs with robust governance and oversight, guided by clearly
document processes.
Digital ecosystems: the future of insurance innovation
The insurance industry stands on the precipice of a paradigm shift.
Digitisation is accelerating at pace, with new and innovative technologies,
the greater use of data and a mobile-first approach not only changing how the
industry operates, but also how customers expect it to operate. So too is the
competitive landscape changing the playing field for those incumbents in the
industry, forcing them towards a better defined, service-based approach
similar to that being adopted by many of the larger players in the financial
services industry. But, unlike that industry, the insurance sector has
typically been slower to adopt digitisation. That has to change. Digital can
no longer be the preserve of the innovators or pioneers in the sector, it
should permeate every level of the competitive landscape if insurers wish to
reshape their business in line with customer expectations. Indeed, according
to research by Accenture that analysed close to 20 industries, insurance is
among those most susceptible to future disruption. Accenture explained that,
by 2022, carriers that are slow to respond to digital - or ‘hyper-relevant’ -
competitors could suffer market share erosion close to $200bn and miss the
opportunity to pursue new growth activities worth $177bn.
Data Management: An Unwitting Game Of Russian Roulette
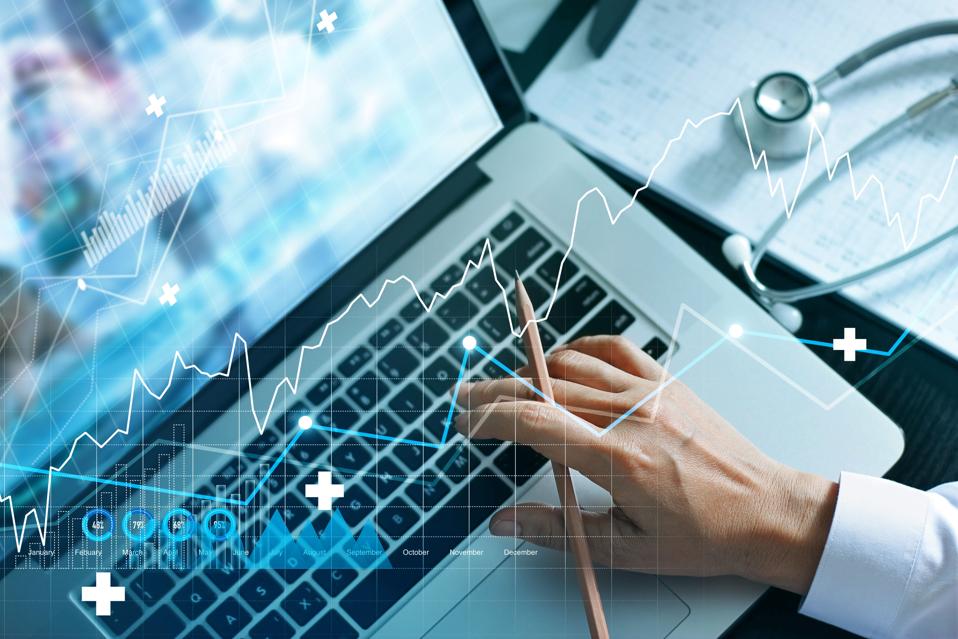
The fear all senior management teams should have is that, in the race to
quickly automate and complete the digital transformation journey, the
post-COVID-19 reality of fiscally austere conditions will cause their
organizations to skimp or ignore the unglamorous yet vital data management
systems and disciplines. In the cases where this happens, those companies
could face disastrous consequences, ranging from disruption of vital processes
to high-profile breaches of privacy and/or security. Of one thing we can be
sure: Without mastery of the data, the automated systems and their digital
platforms will fail. However, the consequences of these failures are hard to
predict. Some will be small and manageable; others could be severe.
Effectively, companies without data discipline and strong data management will
play Russian roulette. Every day they will spin the revolver chamber, put the
gun to their proverbial head and pull the trigger. Eventually, a live round
will go off. Despite the often frustratingly obscure nature of the problem and
the need for expensive investments without clear paybacks, the need to take
data management seriously and adequately provision it with talented teams
backed with the necessary investments is paramount.
The Future of Remote Work, According to Startups
No matter where in the world you log in from—Silicon Valley, London, and
beyond—COVID-19 has triggered a mass exodus from traditional office life. Now
that the lucky among us have settled into remote work, many are left wondering
if this massive, inadvertent work-from-home experiment will change work for
good. In the following charts, we feature data from a comprehensive survey
conducted by UK-based startup network Founders Forum, in which hundreds of
founders and their teams revealed their experiences of remote work and their
plans for a post-pandemic future. While the future remains a blank page, it’s
clear that hundreds of startups have no plans to hit backspace on remote work.
Based primarily in the UK, almost half of the survey participants were
founders, and nearly a quarter were managers below the C-suite. Prior to
pandemic-related lockdowns, 94% of those surveyed had worked from an external
office. Despite their brick-and-mortar setup, more than 90% were able to
accomplish the majority of their work remotely.
Lead Through Volatility With Adaptive Strategy
Strategy defines the long-term choices and actions the enterprise must take to
create, deliver and capture value as envisaged in the business model. But the
more time spent creating a plan, the less time there is to execute it,
increasing the risk that the world has moved on and the plan is out of date.
Implementing promptly also helps to surface the plan’s flaws and identify
where to improve. Adaptive strategy doesn’t require perfect or complete
information to execute; it uses available information to identify the most
immediate actions required to be successful. Given today’s highly
disrupted conditions, few enterprises can afford to wait a year to review
strategy as was typical when business context moved slowly, and disruption
happened infrequently, if at all. Some now review their strategy on a
quarterly or even a monthly basis, but a truly adaptive enterprise monitors
its business context on an ongoing basis, initiating a strategy review
whenever new information is available to reframe the context. The vision
that guides an adaptive strategy can still be long-term and bold — but should
be continually extended (not changed completely once every few years) to push
the boundaries of what the enterprise must do to succeed.
5 Key Research Findings on Enterprise Artificial Intelligence
The pandemic has caused a drastic shift in consumer behavior as individuals
stay at home and adjust their daily routines. Many travel, hospitality, and
restaurant workers are out of work, and those fortunate to still be employed
have shifted their spending patterns. This in turn has put pressure on AI and
machine learning teams to ensure the accuracy of their predictive models in
this changed environment, yet only 33% are monitoring their models in
production. ... While the board of directors and C-suite almost universally
appreciate the importance of AI (100% of respondents indicate is either or
fully accepted as a strategic imperative), it does appear that there will be
more pressure to show clear ROI and cut through the hype to provide a mature
and sophisticated approach to AI. With 65% reporting that building a team with
the right skills is a medium or large barrier for success, it’s likely that
teams will continue to invest in efficient processes, streamlined development
to production environments, and centralized approaches to AI governance and
skills and resource management.
Quote for the day:
No comments:
Post a Comment