The Future Of Work Is Not What You Think

Technology in its broadest sense is having profound impacts on society – both
good and bad – as it always has. Much of the research points towards an
acceleration of technology impact and much more profound structural changes
than before. The growth of platforms, ecosystems, what I call ‘self’
technologies – those technologies that complete actions ‘by themselves’ (AI,
ML, RPA, Blockchain, Nano, Smart etc) – along with advances in biotechnology
are already having strong impacts to how society works, and how work works.
The explosion of data and the promise of quantifying everything is already
creating new challenges around privacy and what is appropriate to track and
measure. Many of these technologies are creating fundamental contradictions
that are new and will need significant creative thinking in how best to
extract true net benefits. Platform technologies supported by growing
ecosystems are fully enabling sustainable ‘Self Careers’. A plethora of tools
are widely available for individuals to deliver quality output, design their
employment journeys and create their own portfolios of work.
Challenges facing data science in 2020 and four ways to address them

Despite the popularity of open-source software in the data science world, 30%
of respondents said they aren't doing anything to secure their open-source
pipeline. Open-source analytics software is preferred by respondents because
they see it as innovating faster and more suitable to their needs, but
Anaconda concluded that the security problems may indicate that organizations
are slow to adopt open-source tools. "Organizations should take a proactive
approach to integrating open-source solutions into the development pipeline,
ensuring that data scientists do not have to use their preferred tools outside
of the policy boundary," the report recommended. ... Ethics, responsibility,
and fairness are all problems that have started to spring up around machine
learning and artificial intelligence, and Anaconda said enterprises "should
treat ethics, explainability, and fairness as strategic risk vectors and treat
them with commensurate attention and care." Despite the importance of
addressing bias inherent in machine learning models and data science, doing so
isn't happening: Only 15% of respondents said they had implemented a bias
mitigation solution, and only 19% had done so for explainability.
Apple Watch, Fitbit data can spot if you are sick days before symptoms show up
The current study, which is a collaboration between Stanford Medicine, Scripps
Research, and Fitbit, will use data gathered from the wearables to create
algorithms that can detect the physiological changes in someone that show
they're coming down with an infection, potentially before they even know
they're sick. Once the signs of infection -- such as an increase in resting
heart rate -- have been detected, the user will be alerted through the app
that they may be getting sick, allowing them to self-isolate earlier and so
spread the infection to fewer people. The lab has been investigating the
potential of wearable devices to shed light on changes in users' health for
some years. Researchers published a study in 2017 that showed devices could
pick up changes in physical parameters before the wearer noticed any
symptoms. The algorithm from that research, known as 'change of heart',
detected that changes in heart rate could signal an early infection, and the
lab is now building on that research for the current pandemic. "We continued
to improve the algorithm, then when the COVID-19 outbreak came, as you might
imagine, we started scaling at full force," Michael Snyder, professor and
chair of genetics at the Stanford School of Medicine, told ZDNet.
9 career pitfalls every software developer should avoid
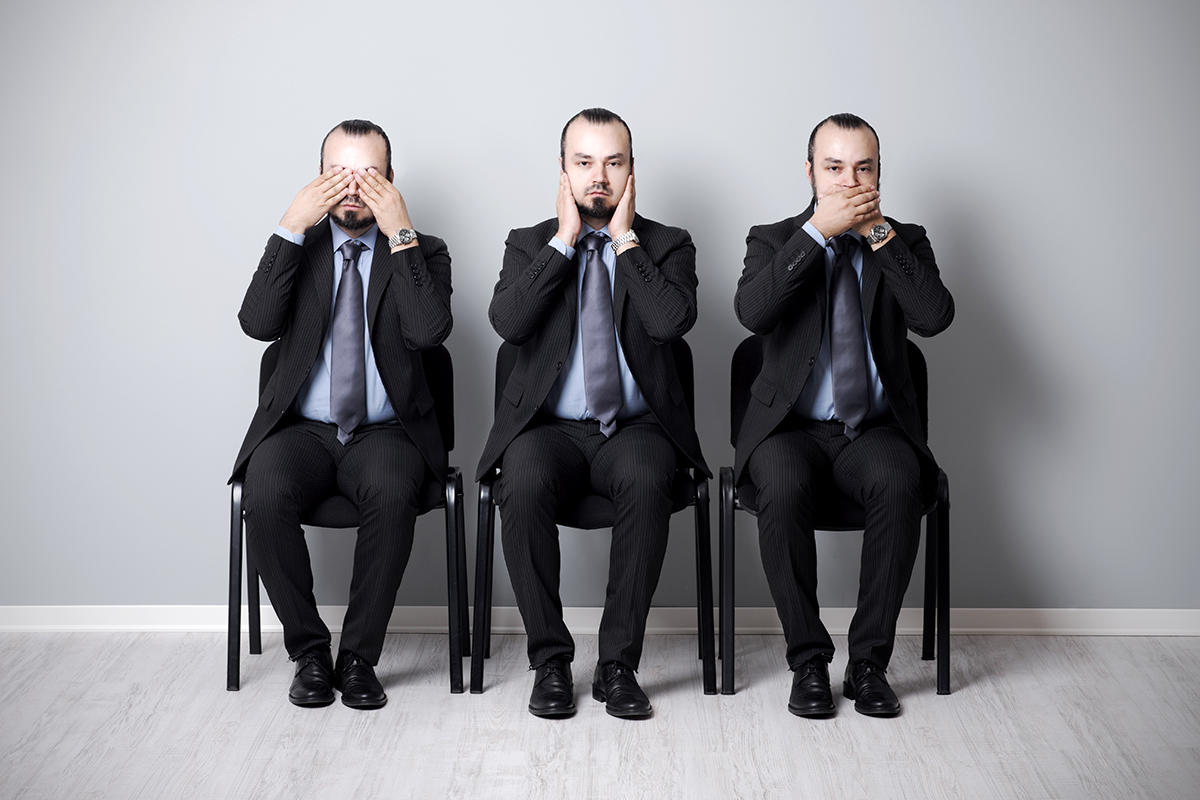
It seems easy and safe to become an expert in whatever is dominant. But then
you’re competing with the whole crowd both when the technology is hot and when
the ground suddenly shifts and you need an exit plan. For example, I was a
Microsoft and C++ guy when Java hit. I learned Java because everyone wanted me
to have a lot more experience with C or C++. Java hadn’t existed long enough
to have such requirements. So I learned it and was able to bypass the
stringent C and C++ requirements, and instead I got in early on Java. A few
years back, it looked like Ruby would be ascendant. At one point, Perl looked
like it would reach the same level that Java eventually did. Predicting the
future is hard, so hedging your bets is the safest way to ensure relevance.
... “I’m just a developer, I don’t interest myself in the business.” That’s
career suicide. You need to know the score. Is your company doing well? What
are its main business challenges? What are its most important projects? How
does technology or software help achieve them? How does your company fit into
its overall industry? If you don’t know the answers to those questions, you’re
going to work on irrelevant projects for irrelevant people in irrelevant
companies for a relatively irrelevant amount of money.
Top 13 Challenges Faced In Agile Testing By Every Tester
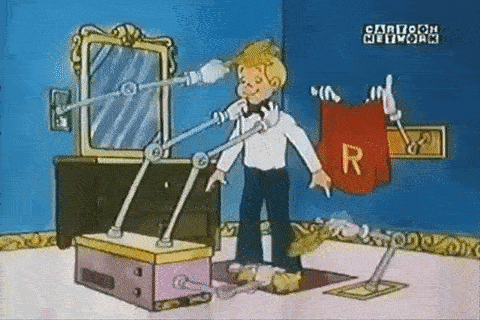
Speaking strictly in business terms, time is money. If you fail to accommodate
automation in your testing process, the amount of time to run tests is high,
this can be a major cause of challenges in Agile Testing as you’d be spending
a lot running these tests. You also have to fix glitches after the release
which further takes up a lot of time. Automation for browser testing is done
with the help of Selenium framework, in case you’re wondering, What is
Selenium, refer to the article linked. ... Most teams emphasize maximizing
their velocity with each sprint. For instance, if a team did 60 Story Points
the last time. So, this time, they’ll at least try to do 65. However, what if
the team could only do 20 Story Points when the sprint was over? Did you
realize what just happened? Instead of making sure that the flow of work
happened on the scrum board seamlessly from left to right, all the team
members were concentrating on keeping themselves busy. Sometimes, committing
too much during sprint planning can cause challenges in Agile Testing. With
this approach, team members are rarely prepared in case something unexpected
occurs.
Brute-Force Attacks Targeting RDP on the Rise
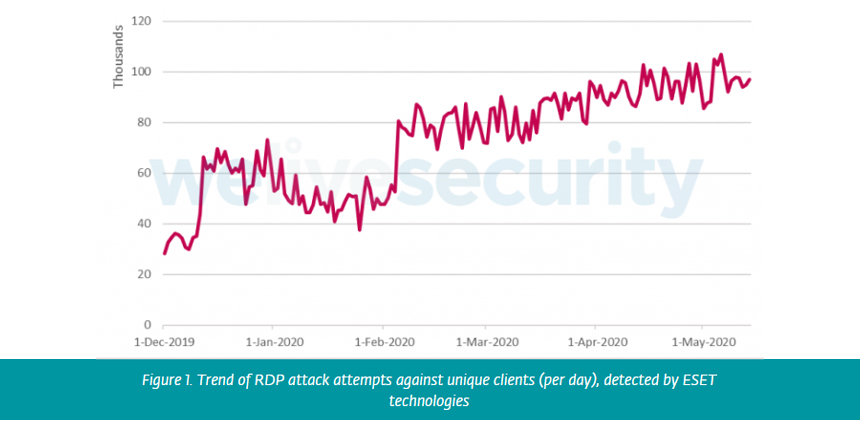
Since the start of the COVID-19 pandemic, the number of brute-force attacks
targeting remote desktop protocol connections used with Windows devices has
steadily increased, spiking to 100,000 incidents per day in April and May,
according to an analysis by security firm ESET. By waging brute-force attacks
against RDP connections, attackers can gain access to an IT network, enabling
them to install backdoors, launch ransomware attacks and plant cryptominers,
according to ESET's analysis. RDP is a proprietary Microsoft communications
protocol that allows system administrators and employees to connect to
corporate networks from remote computers. With the COVID-19 pandemic forcing
employees all over the world to work at home, many organizations have
increased their use of RDP but have overlooked security concerns. "Despite the
increasing importance of RDP, organizations often neglect its settings and
protection. When employees use easy-to-guess passwords, and with no additional
layers of authentication or protection, there is little that can stop
cybercriminals from compromising an organization's systems," Ondrej Kubovič, a
security analyst with ESET, notes in the report.
3 CIOs talk driving IT strategy during COVID-19 pandemic
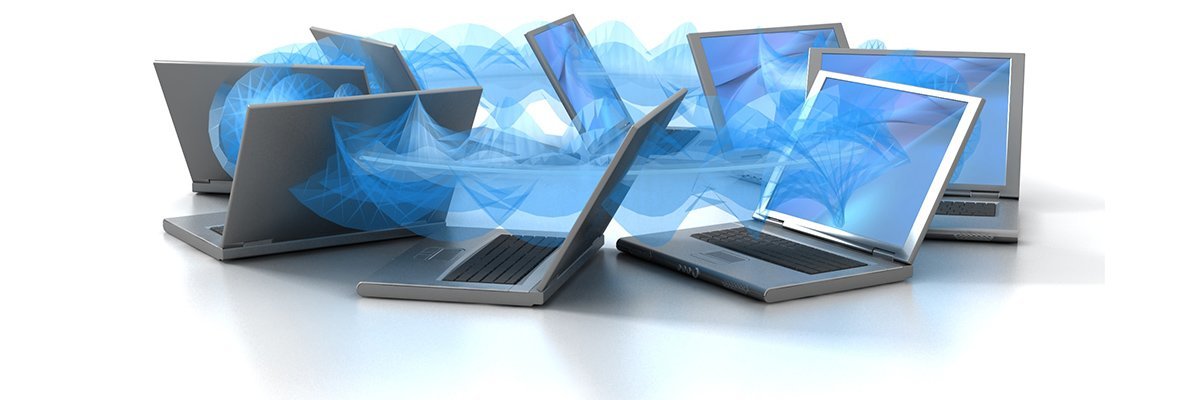
As a result of the COVID-19 pandemic, many IT leaders faced the challenge of
having to transition organizations to a work-from-home environment. In less
than 10 days, the technology and operations team at Travelers Insurance
managed to get the company that has offices all over the world to being fully
online, with almost 100% of employees working remotely, said Lefebvre. In
addition to having access to digital capabilities, the IT department's ability
to respond quickly and effectively was in large part a result of building and
engineering a culture with deep expertise, according to Lefebvre. Tariq echoed
this during the online panel, describing the importance of having a culture
that allows for a more innovative mindset as part of the IT strategy. "Like
any organization, we focus on results, [but] we also equally focus on creating
a healthy and inclusive culture -- a culture where every team member feels
that they have a voice, they are heard, where they can be themselves and be
their best," he said. "[It's] a culture that is focused on continuous
improvement and value generation. When you do that, magic happens."
Smart cities will track our every move. We will need to keep them in check
For James, it is key to make sure that citizens trust the organizations that
own the data about them. "We need to know how this data is governed, who owns
it, and who has access to the platform that does it," he says. "Otherwise,
there is a risk that you won't bring citizens along with you." James points to
the smart city initiative led by an Alphabet-owned urban design business in
Toronto. The project was recently axed due to the economic uncertainty caused
by the pandemic, but was already running into a series of problems because of
backlash from privacy concerned leaders who were worried about surveillance.
Ensuring public trust, therefore, is critical; and especially because the cost
of abandoning smart city technology, in the context of COVID-19, will be far
greater than in normal times. "You have to think about what happens, in the
long term, if you don't implement these processes," says James. Smart sensors
and IoT devices won't only be used by city planners to monitor the immediate
impact of measures linked to the pandemic. In the next few months, they will
also be key to the recovery of local businesses, as policy makers start
identifying where residents work, shop, eat out or go for drinks.
For data scientists, drudgery is still job #1
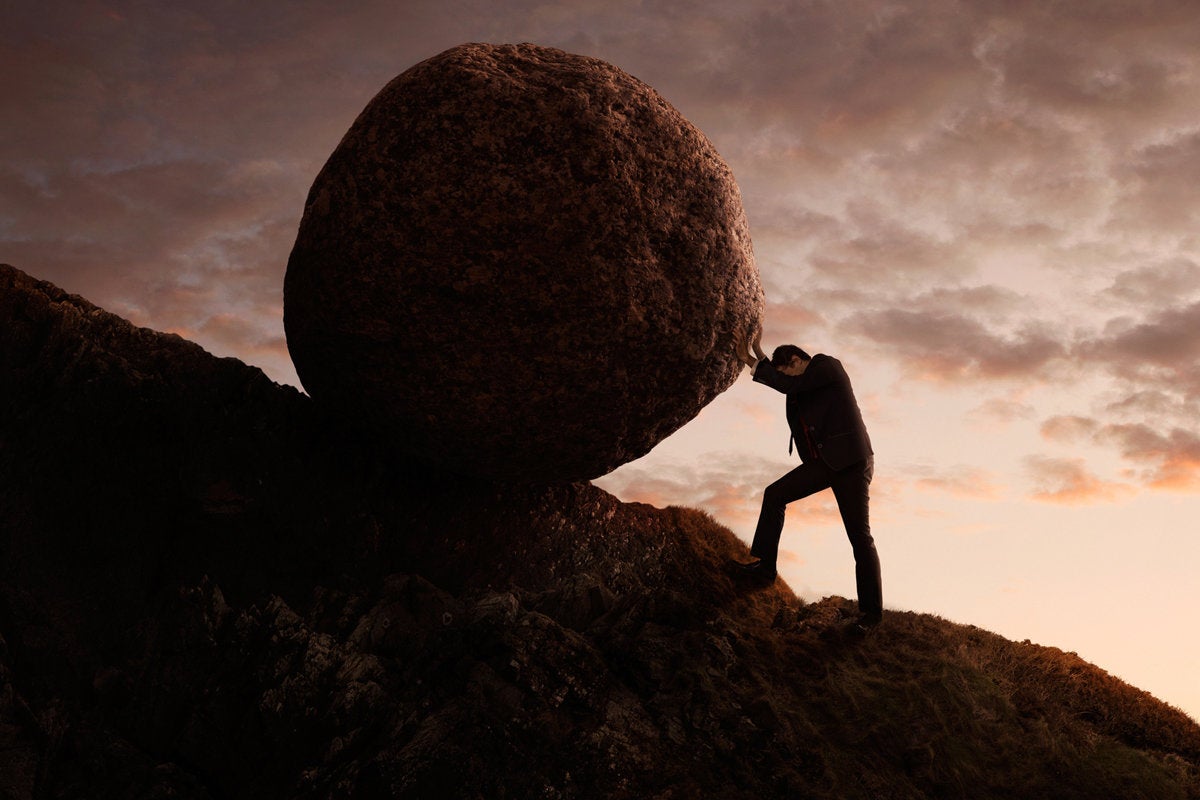
Despite all the advances in recent years in data science work environments,
data drudgery remains a major part of the data scientist’s workday. According
to self-reported estimates by the respondents, data loading and cleaning took
up 19% and 26% of their time, respectively—almost half of the total. Model
selection, training/scoring, and deployment took up about 34% total (around
11% for each of those tasks individually). When it came to moving data science
work into production, the biggest overall obstacle—for data scientists,
developers, and sysadmins alike—was meeting IT security standards for their
organization. At least some of that is in line with the difficulty of
deploying any new app at scale, but the lifecycles for machine learning and
data science apps pose their own challenges, like keeping multiple open source
application stacks patched against vulnerabilities. Another issue cited by the
respondents was the gap between skills taught in institutions and the skills
needed in enterprise settings. Most universities offer classes in statistics,
machine learning theory, and Python programming, and most students load up on
such courses.
Deep learning's role in the evolution of machine learning
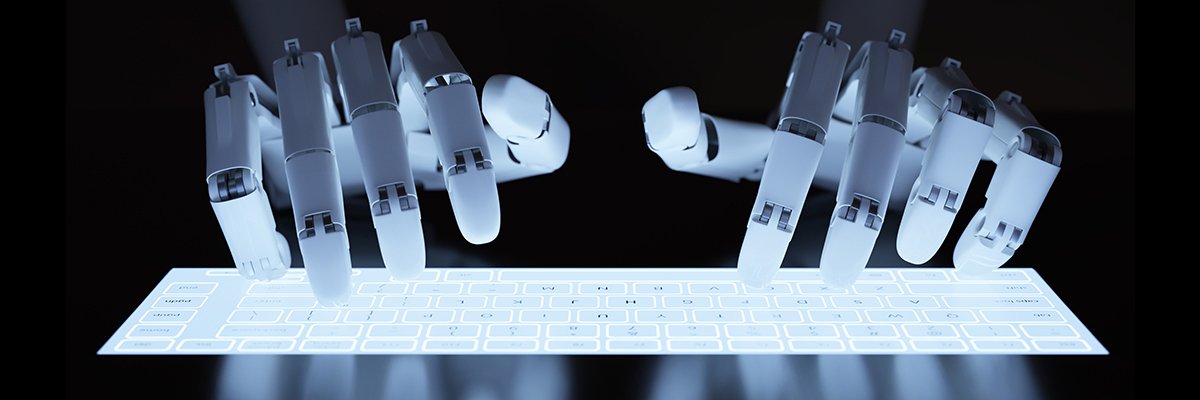
"There are many problems that we didn't think of as prediction problems that
people have reformulated as prediction problems -- language, vision, etc. --
and many of the gains in those tasks have been possible because of this
reformulation," said Nicholas Mattei, assistant professor of computer science
at Tulane University and vice chair of the Association for Computing
Machinery's special interest group on AI. In language processing, for example,
a lot of the focus has moved toward predicting what comes next in the text. In
computer vision as well, many problems have been reformulated so that, instead
of trying to understand geometry, the algorithms are predicting labels of
different parts of an image. The power of big data and deep learning is
changing how models are built. Human analysis and insights are being replaced
by raw compute power. "Now, it seems that a lot of the time we have
substituted big databases, lots of GPUs, and lots and lots of machine time to
replace the deep problem introspection needed to craft features for more
classic machine learning methods, such as SVM [support vector machine] and
Bayes," Mattei said, referring to the Bayesian networks used for modeling the
probabilities between observations and outcomes.
Quote for the day:
No comments:
Post a Comment