The challenges and opportunities of shadow IT

As more organizations adopt practices like self-service SaaS and BYOD, the
need for greater visibility into their overarching corporate network of
devices becomes even greater. Many organizations faced this crunch when moving
their workforce remote only a few months ago as a response to COVID-19.
Typically, the larger and more widespread an ecosystem of devices is, the more
difficult it becomes for IT teams to maintain visibility and consequently
cyber hygiene of those devices. We can expect many of the challenges around
Shadow IT to only grow in the next few years as more enterprises adopt
practices like BYOD, or even on an operational level, more flexible remote
work policies. Consequently, enterprises will put a greater focus on
automation to better identify and secure devices across their widened
infrastructure. ... SaaS tools bring immediate dangers of freely shared file
data that is not classified or labeled. Or to say this in a more technical
manner, there is zero data governance in collaborative hybrid work
environments over shared files. DLP tools fail to bring effective results in
shared environments. For effective data protection, organizations must have
virtual file labeling that offers an automated process in which all the
relevant security, privacy, and operational policies are considered, and
continually fine-tuned.
Open Banking – The Novel Mainstay of Digital Banking

Open banking is a safe way to give suppliers access to your financial data. It
is establishing a statistics architecture, where a group of organizations can
share the information via Application Programming Interfaces (APIs). These
APIs are used by banking and financial companies to exchange data between
them, thus helping to serve consumers better. Open banking allows banks to
offer customized financial services to their consumers, majorly payment
solutions. The revolution is both developing the industry toward
platform-based, hyper-relevant distribution, and offering banks a precious
opportunity to develop their networks and extend reach. In short, we can say
open banking is more about sharing financial data by electronic means,
securely, and only under circumstances when consumers agree. Therefore, when
you share data voluntarily owing to legal reasons, you become a part of the
open banking community. Gear up for a world of websites and apps, where one
can select modern economic services and products from providers policed by the
Financial Conduct Authority (FCA) and European equivalents.
Balancing UX and Privacy With IoT

When we consider the compromised privacy of individuals, we are talking about
each individual’s loss of control over personal information. When people
invest in these interconnected devices, they are not entirely aware of how
much of their personal information is tracked and saved by the manufacturer in
a bid to improve user experience. An individual can lose control if someone
hacks into their smartphone or computer and remotely operates other devices.
There’s no doubt that our smartphones carry a majority of our information.
They are linked to our bank accounts, email accounts and even systems that
need authorization. In fact, experts predict that there would be about 31
billion connected IoT devices by the year 2021. Usually, hackers employ
methods that are undetected, so more connections would mean an increase in
hacking activities as well. The data collected from an individual’s smartphone
or laptop can give hackers a detailed look into their activities, including
internet searches and purchasing power. The information is typically used to
work on user experience, but also can be used to target particular products to
the individual. Sometimes, this data is even sold to other organizations that
are looking for a target audience to sell their products.
Improving data management in the life sciences industry

If an email account is breached, the data on that user’s account will be
visible to the attacker. Should emails featuring product artwork or containing
sensitive information be visible to the employee, they will also be accessible
to a cybercriminal who has admittance to the account. Despite stricter
serialisation regulations and the efforts of the wider industry, the full
supply chain remains at risk of this information being sold to counterfeiters.
Addressing this possibility should be a priority for regulators now that a
number of serialisation laws in key markets are over the line. New
technologies provide opportunities to deliver better communication and
collaboration while ensuring compliance and security. Often, this cannot be
guaranteed by unsecure tools like email. Using platforms or systems that
offer a shared workspace, accessible by multiple organisations, enables
collaborative project management, with a clear, immutable audit trail. They
also support companies in the gathering and analysis of data which has a
number of high value use cases. One of the most promising and impactful
will be improved supply and demand forecasting.
How Does Data Management Drive Efficiency for Organizations?
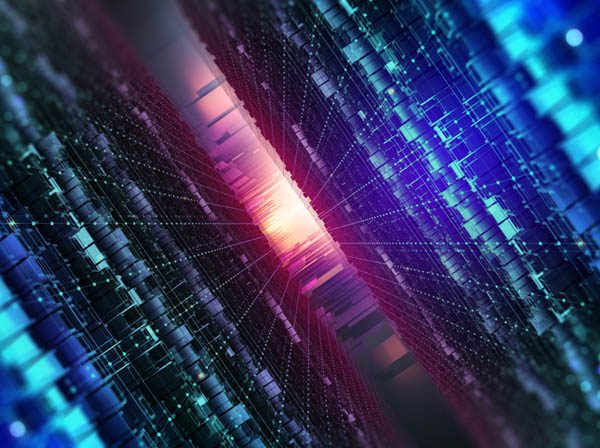
In an ideal business world, many different Data Management professionals
collaborate and execute best practices to extract the maximum business value
from their enterprise data assets. These professionals are data architects,
data engineers, data modelers, DBAs, developers, data quality experts, and
data governance experts, who work alongside executives and high-level,
decision-makers to conceptualize, design, develop, and implement the desired
Data Management infrastructure. Data Management teams often work with
real-time data, which requires superior data capture, data integration, data
preparation, and data analytics platforms — now available due to AI and ML.
Many associated technologies like data fabric, graph processing, IoT, big
data, edge computing, and so on need to work in conjunction with each other to
make the unified Data Management system work. At a more nitty-gritty,
technical level, complex Data Management tasks happen through Metadata
Management, Master Data Management, advanced data compliance tasks, and
continuous monitoring. A relatively new Data Management effort creates “data
catalogs” to document which data is available where, including business
glossaries, data dictionaries, and data lineage records.
Automating Machine Learning: Google AutoML-Zero Evolves ML Algorithms From Scratch
Evolutionary algorithm (EA) is a subset of evolutionary computation, a family
of population-based trial and error problem solvers with a metaheuristic or
stochastic optimization character. In evolutionary computation, an initial set
of candidate solutions is first generated and then iteratively updated. Each
new generation is produced by stochastically removing less-desired solutions
and introducing small random changes. Evolutionary algorithms use mechanisms
inspired by biological evolution such as reproduction, mutation,
recombination, and selection. EAs often perform well in approximating
solutions to a range of problems that would otherwise take too long to
exhaustively process. The use of evolutionary principles for automated
problem-solving was formally proposed and developed more than 50 years ago.
Artificial evolution became a widely recognized optimization method as a
result of the work of German researcher Ingo Rechenberg, who used evolution
strategies to solve complex engineering problems in the 1960s and early 1970s.
In 1987, Jürgen Schmidhuber published his first paper on genetic programming,
and later that year described first general-purpose learning algorithms in his
diploma thesis, Evolutionary Principles in Self-Referential Learning.
Rise of automation creates new RPA job descriptions
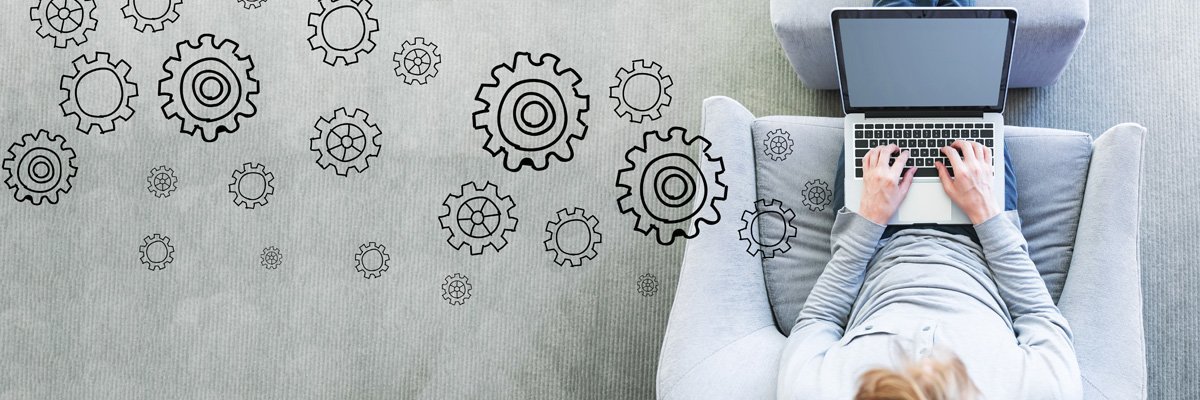
Automation engineers by nature need to have a broad set of capabilities in
order to support a mix of no-code platforms, API integrations and traditional
coding practices to build fully functional offerings for clients. Traditional
development teams often look for talent that has deep capabilities in narrow
fields. In contrast, Cottongim said automation engineers should be conversant
in a wide variety of tools and techniques but not necessarily a master in any
one. Automation engineers will also need to have skills beyond traditional
roles for engaging with their business partners and being able to distill
business needs into rapidly executed automation offerings. They will also need
to be able to apply a customer-centric view and build in an agile manner,
while partnering closely with their business teams. Cottongim also expects to
see more demand for cloud architects and cloud engineers that can support
intelligent automation needs. They will need to understand how to create
applications built from a mix of VMs, databases, networking and
high-availability management techniques.
Is Open Finance worth getting excited about, or is it just spin?
On the bright side, there are protections in place and limitations; overseen
by the regulator. Users completely own their data and can revoke the access
they give to third-parties at any time. There are also restrictions on
companies’ ability to sell the data directly to third-parties. Instead,
companies holding the data can monetise it by recommending new pension
providers and taking a commission fee, for instance, or charging consumers for
the service (like Monzo has done). “What’s going to make or break the success
longer term is ‘do you feel confident that you know where this data is
going?'” Grose noted, highlighting the need to educate users on their data
rights and companies’ use of their data. Nonetheless, Levine warned that some
companies might be tempted to charge a so-called ‘privacy premium’, whereby
consumers get a worse deal or product based on their financial data. “It only
takes one kind of major loss of trust or issue that we find ourselves in a
place where actually the whole industry is hurt, and we may be going
backwards,” Levine said. Meanwhile, Vans-Colina added there’s a big risk that
open banking and finance data will get hacked and leaked.
Data Governance: Stay Non-Invasive in Your Approach
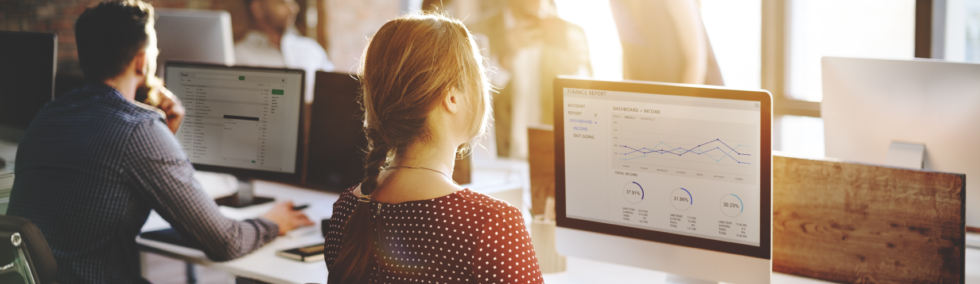
People naturally rebel against the idea of being governed. Data governance is
known in some circles as “People Governance” because it is people’s behavior –
how they define, produce and use data – that is being governed. In other
words, the data will do what we tell it to do, so we must govern people’s
behavior if we want to improve the quality, value, and understanding of the
data. Therefore, the approach the organization takes to govern the data (and
the people) can make or break whether the data governance program is accepted
or rejected by the organization. I have been known to say that, “the data will
not govern itself.” Let me add to that with, “the documentation about the
data, or the metadata, will not govern itself either.” Most of us have
experienced data and metadata that has been left ungoverned. Why? Because
people are not held responsible for the quality and/or value of the data or
the documentation. As a result, there is no way to improve the efficiency and
effectiveness of the way data assets are being leveraged. Ungoverned data is
replicated many times over with many different versions of the “same”
data.
Creating a modern data governance strategy to accelerate digital transformation
Though it’s early in our journey toward modern data governance, we do have a few
best practices to share. Primarily, we recommend that you address your data
governance strategy holistically. As illustrated below, we designed our approach
so that standards, embedded into the engineering process and data centralization
on the modern data foundation worked together to ensure end-to-end modern data
governance. Build standards into your existing process and implement them as
engineering solutions. By approaching data governance during the design phase of
the larger Enterprise Data strategy, we have been able to institutionalize
“governance by design” into the engineering DNA—and apply it to data at every
touchpoint. We are building our data governance controls into the centralized
analytics infrastructure and analytics processes. Consider implementing a
modern data foundation with integrated toolsets. The EDL, with its built-in
governance services and capabilities, does more than scale data governance
efforts—it enables enterprise analytics for the whole organization.
Quote for the day:
No comments:
Post a Comment