How to successfully implement an AI system
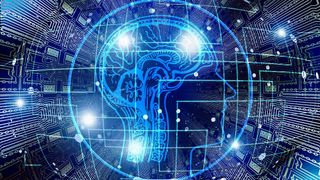
Companies should calculate the anticipated cost savings that would be gained with a successful AI deployment, using that as a starting point for investment so that costs of errors or short falls on expectations are minimised if they occur. The cost savings should be based on efficiency gains, as well as the increased productivity that can be harnessed in other areas of the business by freeing up staff from administration tasks. This ensures companies do not over-invest at the beginning before seeing initial results and if changes are necessary they do not cannibalise potential ROI and companies can still potentially switch to other viable alternative use cases. Before advising companies on what solution they should invest in, it's important to first establish what they want to achieve. Digital colleagues can provide a far superior level of customer service however, they require greater resource to set up. Most chatbots are not scalable, once deployed they cannot be integrated into other business areas as they are designed to answer FAQs based on a static set of rules. Unlike digital colleagues, they cannot understand complex questions or perform several tasks at once.
How adidas is Creating a Digital Experience That's Premium, Connected, and Personalized
Take something like a product description. How do we really have the product descriptions and offerings so that if you're interested in sports we will help you find exactly the product that you need for the sport that you're interested in? We will also educate you and bring you back at different points in time to help you find out what you need when you need it, or with an engagement program. Ultimately, like the membership program, that it has something that's sticky, that you can give back to something, even more, you can participate in events and experiences. For us, a lot of it’s really deepening those experiences but also exploring new technologies and new areas. Omnichannel was kind of the original wave which happened and I said it was the freight train that came past us a couple of years ago. Now we're also looking at what those next freight trains are, whether it's technologies like blockchain or experiencing picking up a new channel. For example, we're working extensively with Salesforce on automation, how we can automate consumer experiences.
What Deep Learning Can Offer to Businesses
With the capabilities of artificial intelligence, the way the words are processed and interpreted can be changed dramatically. It turns out we can define the meaning of the word based on its position in the text without the need of using a dictionary. ... One of the most recent successful appliances of deep learning for image recognition came from Large Scale Visual Recognition Challenge, when Alex Krizhevsky applied convolutional neural networks to organize images from ImageNet, a dataset containing 1.2 million pictures, into 1,000 different classes. In 2012, Krizhevsky’s network, AlexNet, achieved a top-5 test error rate of 15.3%, outperforming traditional computer vision solutions with more than 10% accuracy. The experience of Alex Krizhevsky changed the landscape of the data science and artificial intelligence field from the perspective of the research and business application. In 2012, AlexNet was the only deep learning model at ILSVRC (ImageNet Large Scale Visual Recognition Competition). Two years later, in 2014, there were no conventional computer vision solutions among the winners.
Can Global Semantic Context Improve Neural Language Models?
Global co-occurrence count methods like LSM lead to word representations that can be considered genuine semantic embeddings, because they expose statistical information that captures semantic concepts conveyed within entire documents. In contrast, typical prediction-based solutions using neural networks only encapsulate semantic relationships to the extent that they manifest themselves within a local window centered around each word (which is all that’s used in the prediction). Thus, the embeddings that result from such solutions have inherently limited expressive power when it comes to global semantic information. Despite this limitation, researchers are increasingly adopting neural network-based embeddings. Continuous bag-of-words and skip-gram (linear) models, in particular, are popular because of their ability to convey word analogies of the type “king is to queen as man is to woman.”
Big Data and Machine Learning Won’t Save Us from Another Financial Crisis

Machine learning can be very effective at short-term prediction, using the data and markets we have encountered. But machine learning is not so good at inference, learning from data about underlying science and market mechanisms. Our understanding of markets is still incomplete. And big data itself may not help, as my Harvard colleague Xiao-Li Meng has recently shown in “Statistical Paradises and Paradoxes in Big Data.” Suppose we want to estimate a property of a large population, for example, the percentage of Trump voters in the U.S. in November 2016. How well we can do this depends on three quantities: the amount of data (the more the better); the variability of the property of interest (if everyone is a Trump voter, the problem is easy); and the quality of the data. Data quality depends on the correlation between the voting intention of a person and whether that person is included in the dataset. If Trump voters are less likely to be included, for example, that may bias the analysis.
Spending on cognitive and AI systems to reach $77.6 billion in 2022
Banking and retail will be the two industries making the largest investments in cognitive/AI systems in 2018 with each industry expected to spend more than $4.0 billion this year. Banking will devote more than half of its spending to automated threat intelligence and prevention systems and fraud analysis and investigation while retail will focus on automated customer service agents and expert shopping advisors & product recommendations. Beyond banking and retail, discrete manufacturing, healthcare providers, and process manufacturing will also make considerable investments in cognitive/AI systems this year. The industries that are expected to experience the fastest growth on cognitive/AI spending are personal and consumer services (44.5% CAGR) and federal/central government (43.5% CAGR). Retail will move into the top position by the end of the forecast with a five-year CAGR of 40.7%. On a geographic basis, the United States will deliver more than 60% of all spending on cognitive/AI systems throughout the forecast, led by the retail and banking industries.
5 ways industrial AI is revolutionizing manufacturing
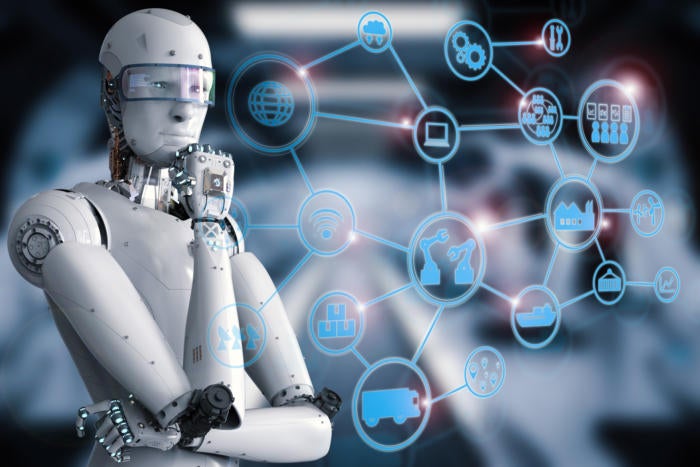
In manufacturing, ongoing maintenance of production line machinery and equipment represents a major expense, having a crucial impact on the bottom line of any asset-reliant production operation. Moreover, studies show that unplanned downtime costs manufacturers an estimated $50 billion annually, and that asset failure is the cause of 42 percent of this unplanned downtime. For this reason, predictive maintenance has become a must-have solution for manufacturers who have much to gain from being able to predict the next failure of a part, machine or system. Predictive maintenance uses advanced AI algorithms in the form of machine learning and artificial neural networks to formulate predictions regarding asset malfunction. This allows for drastic reductions in costly unplanned downtime, as well as for extending the Remaining Useful Life (RUL) of production machines and equipment. In cases where maintenance is unavoidable, technicians are briefed ahead of time on which components need inspection and which tools and methods to use, resulting in very focused repairs that are scheduled in advance.
Data Centers Must Move from Reducing Energy to Controlling Water
While it is a positive development that overall energy for data centers is being reduced around the globe, a key component that has — for the most part — been washed over is water usage. One example of this is the continued use of open-cell towers. They take advantage of evaporative cooling to cool the air with water before it goes into the data center. And while this solution reduces energy, the water usage is very high. Raising the issue of water reduction is the first step in creating ways our industry can do something about it. As we experience the continued deluge of the “Internet of Things”—projected to exceed 20 billion devices by 2020, we will only be able to ride this wave if we keep energy low and start reducing water usage. The first question becomes how can cooling systems reject heat more efficiently? Let’s say heat is coming off the server at 100 degrees Fahrenheit. The idea is to efficiently capture heat and bring it to the atmosphere as close to that temperature as possible — but it is all dependent on the absorption system.
AI and Automation to Have Far Greater Effect on Human Jobs by 2022
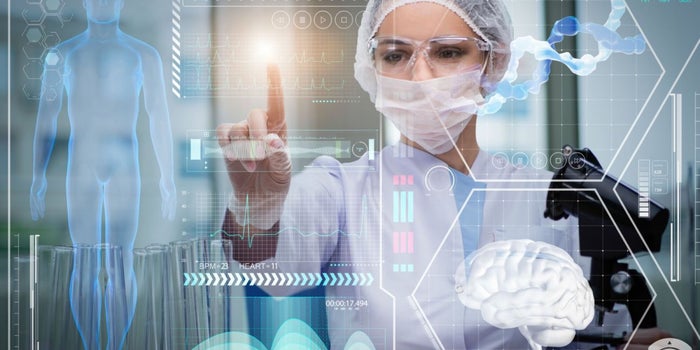
With the domination of automation in a business framework, the workforce can be extended to new productivity-enhancing roles. More than a quarter of surveyed businesses expect automation to lead to the creation of new roles in their enterprise. Apart from allotting contractors more task-specialized work, businesses plan to engage workers in a more flexible manner, utilizing remote staffing beyond physical offices and decentralization of operations. Among all, AI adoption has taken the lead in terms of automation for the reduction of time and investment in end-to-end processes. “Currently, AI is the most rapidly growing technology and will for sure create a new era of the modern world. It is the next revolution- relieving humans not only from physical work but also mental efforts and simplifies tasks extensively,” opined Kuppa. While human-performed tasks dominate today’s work environment, the frontier is expected to change in the coming years.
Modeling Uncertainty With Reactive DDD
Reactive is a big thing these days, and I'll explain later why it's gaining a lot of traction. What I think is really interesting is that the way DDD was used or implemented, say back in 2003, is quite different from the way that we use DDD today. If you've read my red book, Implementing Domain-Driven Design, you're probably familiar with the fact that the bounded contexts that I model in the book are separate processes, with separate deployments. Whereas, in Evan's blue book, bounded contexts were separated logically, but sometimes deployed in the same deployment unit, perhaps in a web server or an application server. In our modern day use of DDD, I’m seeing more people adopting DDD because it aligns with having separate deployments, such as in microservices. One thing to keep clear is that the essence of Domain-Driven Design is really still what it always was -- It's modeling a ubiquitous language in a bounded context. So, what is a bounded context? Basically, the idea behind bounded context is to put a clear delineation between one model and another model.
Quote for the day:
"A company is like a ship. Everyone ought to be prepared to take the helm." -- Morris Wilks
No comments:
Post a Comment