As the Internet of Things (IoT) and edge computing continue to evolve, doors open for conducting business in new ways, especially when it comes to data management on the edge. Until recently, most data collected on the edge (at or near the collection point) was sent to the cloud or a data center for analysis and storage or discarded. A new, third option has become available: Databases that operate on edge hardware. However, not just any database will do. It should be a database that is specifically built for use in the unique environment of the edge. A database that is truly built for use on the edge will empower organizations with the ability to store and process their data at or near the collection point, setting the stage for mission-critical, and possibly, life-saving decisions to be made much faster and very reliably, without the need to rely solely on the cloud. There is no single database solution that fits all, rather each business should look to its unique case on the edge in order to determine the best choice. Having said that, there are a number of questions that should be asked when choosing a database for use on the edge:
NCSC issues core questions to help boards assess cyber risk
The NCSC’s advice comes after the FTSE 350 Cyber governance health check report 2017 found almost 70% of boards have no training in how to deal with cyber incidents, and 10% have no plans in place should they face a cyber threat. Martin claimed that since cyber security is now a major business risk, board members should aim to understand it “in the same way they understand financial risk, or health and safety risk”. This means encouraging boards to ask questions about the state of cyber in their businesses to make sure they are as much a part of the discussion around security as they are other parts of the firm. Boards were used as focus groups by the NCSC to develop appropriate guidelines that teach board members and their staff to understand, recognise and address threats to their businesses. Martin said these were a “taster of the sort of simple, useful but technically authoritative guidance we will be putting out to business” before the launch of a broader toolkit, developed by experts in cyber security, which will be released later this year.
6G will achieve terabits-per-second speeds
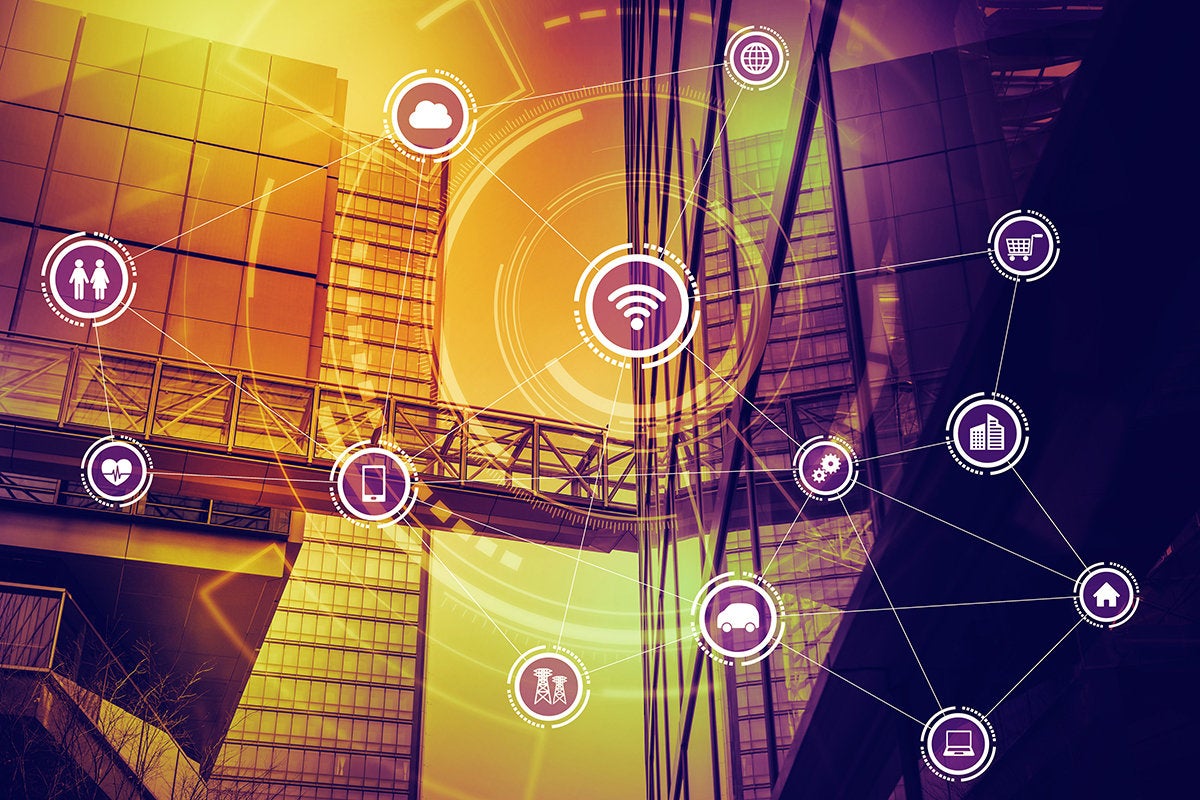
“Millisecond latency [found in 5G] is simply not sufficient,” Pouttu said. It’s “too slow.” One of the problems that will be encountered in 5G overall is related to required scalability, he said. The issue is that the entire network stack is going to be run on non-traditional, software-defined radio. That method inherently introduces network slowdowns. Each orchestration, connection or process decelerates the communication. It’s a problem in part because the thinking is that “there will be 1,000 radios per person in the next ten years.” That’s going to be because the millimeter frequencies that are being used in 5G, while being copious in bandwidth, are short in travel distance. One will need lots of radioheads and antennas—millions—all needing to be connected. And it is why one needs to think up better ways of doing it at scale—hence 6G’s efforts. ... “Data is going to be the key,” Pouttu said. The algorithm’s connection needs a trusted, low-latency and high-bandwidth application. That is where 6G comes in.
Everything You Need to Know About AutoML and Neural Architecture Search

Many people are calling AutoML the new way of doing deep learning, a change in the entire system. Instead of designing complex deep networks, we’ll just run a preset NAS algorithm. Google recently took this to the extreme by offering Cloud AutoML. Just upload your data and Google’s NAS algorithm will find you an architecture, quick and easy! This idea of AutoML is to simply abstract away all of the complex parts of deep learning. All you need is data. Just let AutoML do the hard part of network design! Deep learning then becomes quite literally a plugin tool like any other. Grab some data and automatically create a decision function powered by a complex neural network. Cloud AutoML does have a steep price of $20 USD and unfortunately you can’t export your model once it’s trained; you’ll have to use their API to run your network on the cloud. There are a few other alternatives that are completely free, but do require a tad bit more work.
10 Questions To Ask Before You Use Blockchain
According to Gartner (via PwC), 82% of use cases for blockchain were in the financial industry in 2017, but 2018 has seen a broadening out of use cases, with only 46% related to financial services. Other big verticals where blockchain experimentation is going on include transportation, retail, utilities, manufacturing, insurance, health care and government. The biggest use cases are asset tracking in transportation and government; record-keeping in utilities, health care and insurance; provenance in retail; and securities trading. Because of the hype surrounding blockchain, we are increasingly seeing it being used in situations where better or simpler methods may suffice, such as a database with application logic. Brian Scriber's recent paper in issue No. 4 of IEEE Software gives a great framework for evaluating if a blockchain is applicable in a given situation. Based on that paper and framework, we developed the following simplified list of 10 questions leaders should ask before they embark on using blockchain to address a specific need
Digital Twins Concept Gains Traction Among Enterprises

Digital twins are software models of sensor-enabled physical assets and designed to monitor performance and help reduce costly unplanned equipment outages. The convergence of advanced technologies such as sensors, cloud services, big data and machine learning has brought this idea to fruition. By 2020, at least half of manufacturers with annual revenues in excess of $5 billion will have at least one digital twin initiative launched for either products or assets, according to Gartner Inc. Schneider is among several companies selling software to help customers develop digital representations of physical assets, such as pumps and motors at oil and gas plants and machine building companies. One part of the software also allows customers to calculate the long-term maintenance and estimated potential profit of operating, say, a turbine for a few hours more a day. This is a new source of revenue for the company, with business has accelerating after Schneider’s reverse takeover of British engineering software provider Aveva Group PLC
AI is fueling smarter collaboration
The first is improving the ability of individuals to access data. "Today, finding a document could be tedious [and] analyzing data may require writing a script or form," Lazar said. With AI, a user could perform a natural language query -- such as asking the Salesforce.com customer relationship management (CRM) platform to display third quarter projections and how they compare with the second quarter -- and generate a real-time report. Then, asking the platform to share this information with the user's team and get its feedback could launch a collaborative workspace, Lazar said. The second possible benefit is predictive. "The AI engine could anticipate needs or next steps, based on learning of past activities," Lazar said. "So if it knows that every Monday I have a staff call to review project tasks, it may have required information ready at my fingertips before the call. Perhaps it suggests things that I'll need to focus on, such as delays or anomalies from the past week." Another example is improving the use of meeting tools.
In particular, several new business models are emerging in the media and entertainment industries, where monetizing value has been — and continues to be — a significant challenge. Newspapers and magazines, for instance, still struggle to monetize value in the face of plentiful free content and limited mechanisms for protecting intellectual property. Advertising revenue, long an important income source for publications, has shifted to social media and search platforms, and media companies must figure out how to compensate. In the music world, to cite another example, digital content distribution via streaming is beneficial to major record labels and top-tier artists. But it isn’t commercially viable for smaller labels or average musicians, who receive only a tiny fraction of the revenue generated from their music. Some experts think blockchain may increase the share of revenue captured by content creators and producers by introducing new mechanisms for monetization. However, the current hype about blockchain, the diversity of use cases being proposed, and their potential disruptive effects make it difficult for companies to judge what might be possible for them and what’s merely a pipe dream.
The Four X Factors of Exceptional Leaders
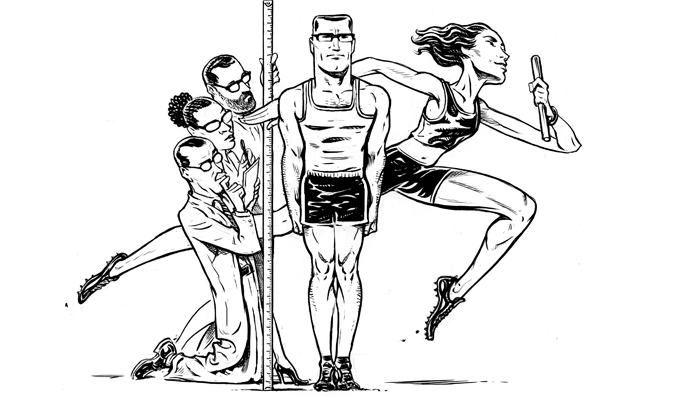
In defining “best-performing leaders,” we focused on a number of factors, but gave priority to actual delivery against the organization’s strategy: the clarity and alignment those leaders generated and the pace of the transformation they were able to drive successfully. In other words, we prioritized the “how” of their leadership, while also considering the “what” of their results. Although our analysis did consider share price as a factor, we weighted it lower than actual performance against strategic goals. For example, one tech company in our data set announced a major shift to mobility and the cloud, and subsequently initiated a round of expensive acquisitions, most of which ended up being wound down or spun off at a discount because they weren’t scalable. The share price remained steady during the period, mainly because of efficiencies created in managing the legacy business, but the future planks of its strategy remained largely unrealized. Our analysis discounted leadership’s effectiveness based on that failure.
Tech Companies Poach AI Talent from Universities
It's not a new trend, and many companies have done it, from Microsoft to Google to Facebook. But it seems to have picked up steam in recent years as organizations are scrambling to hire machine learning and AI talent in a very tight market. "We've done it too," said Eric Haller, executive vice president and global head of Experian DataLabs, an analytics and machine learning R&D organization inside the credit reporting bureau company. He recently spoke with InformationWeek in an interview. "We've recruited professors in London and Sao Paulo. Our chief scientist there was a top professor and still teaches at the University of Sao Paulo…It's definitely a trend." For some academics, making a move to industry can mean a big boost in pay -- after all, AI and machine learning skills are in high demand and highly paid. Even universities that pay their top professors well won't be able to compete with the likes of Google and Facebook. There are benefits beyond financial rewards, too.
Quote for the day:
"The mediocre leader tells The good leader explains The superior leader demonstrates The great leader inspires." -- Buchholz and Roth
No comments:
Post a Comment