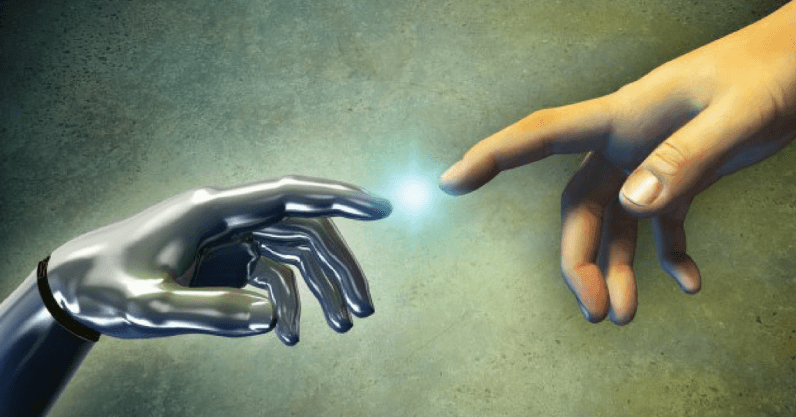
Let’s start with the data part. Contrary to computers, humans are terrible at storing and processing information. For instance, you must listen to a song several times before you can memorize it. But for a computer, memorizing a song is as simple as pressing “Save” in an application or copying the file into its hard drive. Likewise, unmemorizing is hard for humans. Try as you might, you can’t forget bad memories. For a computer, it’s as easy as deleting a file. When it comes to processing data, humans are obviously inferior to AI. In all the examples iterated above, humans might be able to perform the same tasks as computers. However, in the time that it takes for a human to identify and label an image, an AI algorithm can classify one million images. The sheer processing speed of computers enable them to outpace humans at any task that involves mathematical calculations and data processing. However, humans can make abstract decisions based on instinct, common sense and scarce information. A human child learns to handle objects at a very young age. For an AI algorithm, it takes hundreds of years’ worth of training to perform the same task.
What is Industry 5.0?
The handshake between a human being and a robot symbolized of the new reality, even by knowing that it will not be the reality in the future, as most automation, machine intelligence and even robots are working in the background, supporting the workforce or taking on large portions of work, like in production and manufacturing. Investment banking systems are already in use since more than a decade to negotiate and define the share price and sell- / buy-decisions within Nano-seconds independent form any human interaction. The next wave of industrial revolution needs to define, how we collaborate and how we define the rules between human and machine interaction. When artificial intelligence is taking decisions, like we could see in an impressive example during Google I/O 2018 presented by Sundar Pichai, CEO of Google, where a voice assistant called to make an appointment and the woman answering the call didn’t have a chance to recognize, that she was speaking to a robot.
Why Cybersecurity Is Becoming A Top-Priority Investment

Using tools like Privnote is one way to securely transfer valuable data. Privnote is a platform that securely transfers data online and then self-destructs. For protecting large amounts of data, the smartest way to go about finding the right cybersecurity company is to ask around for referrals. You’re better off doing this than making a blind Google search and hoping for the best. If a cybersecurity company is good enough for your colleagues and peers, then it will likely be good enough for your business. My business develops engaging content that attracts the millennial generation, which means we launch a considerable amount of online advertising campaigns. Some of these campaigns require creating B2B accounts with other platforms, so I’m not only protecting my clients’ information, but also my own. Additionally, your product itself needs to be protected. Cyber thieves will try to steal your products’ Amazon standard identification number code and profit from your online sales.
Empowering executives with data security effectiveness evidence
Your leaders are making decisions predicated on these non-security measures every day to increase value for their shareholders, address stakeholder requirements, and mitigate business risks. Security is simply another variable in the business risk equation. In fact, your security program isn’t about security risk in and of itself, but rather, the financial, brand, and operational risk from security incidents. One area where the need for security effectiveness evidence is profusely obvious is around rationalization. For example, many auditors no longer ask, “Do you have security tools in place to mitigate risk?” because the answer is always, “Yes, but we need more tools, training, and people anyhow.” Now auditors are asking for rationalization in terms of, “Can you prove, with quantitative measures, that our security tools are adding value? And can you supply proof regarding the necessity for future security investment?”
Using Neuroscience to Make Feedback Work and Feel Better
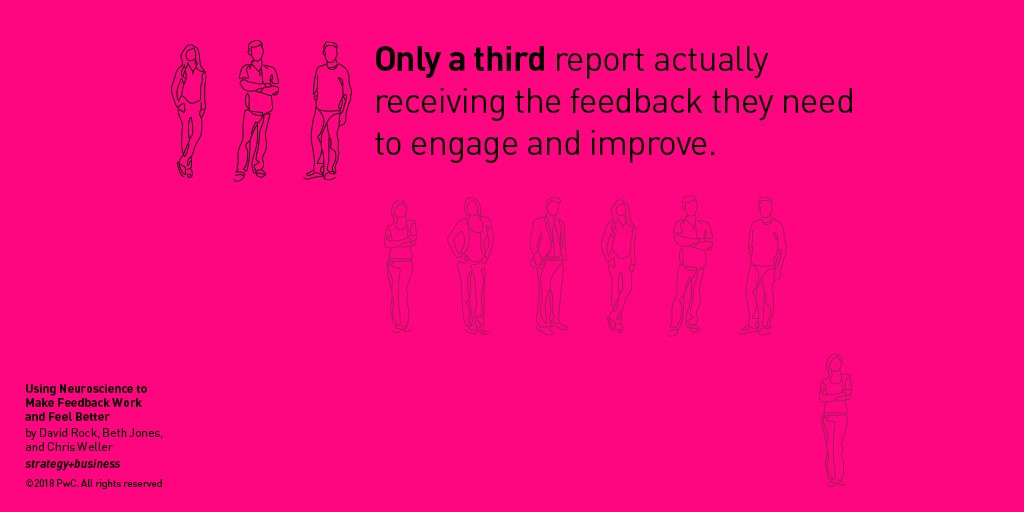
Modern humans base their decisions on many of the same pro-social, consensus-building impulses. We make polite chitchat at work, even in our most antisocial states, so others will see us as friendly. We avoid talking to the attractive stranger at the bar because something deep and ancient in us registers the possibility of rejection as a matter of life and death. When neuroscientists conduct brain scans of people exposed to social threats, such as a nasty look or gesture, the resulting images look just like the scans of people exposed to physical threats. Our bodies react in much the same ways. Our faces flush, our hearts race, and our brains shut down. No matter if we’re giving a speech to thousands or coming face-to-face with a jungle cat, our body’s response is the same: We want out. Feedback conversations, as they exist today, activate this social threat response. In West and Thorson’s study, participants’ heart rates jumped as much as 50 percent during feedback conversations.
Big Data And ML: A Marriage Between Giants!
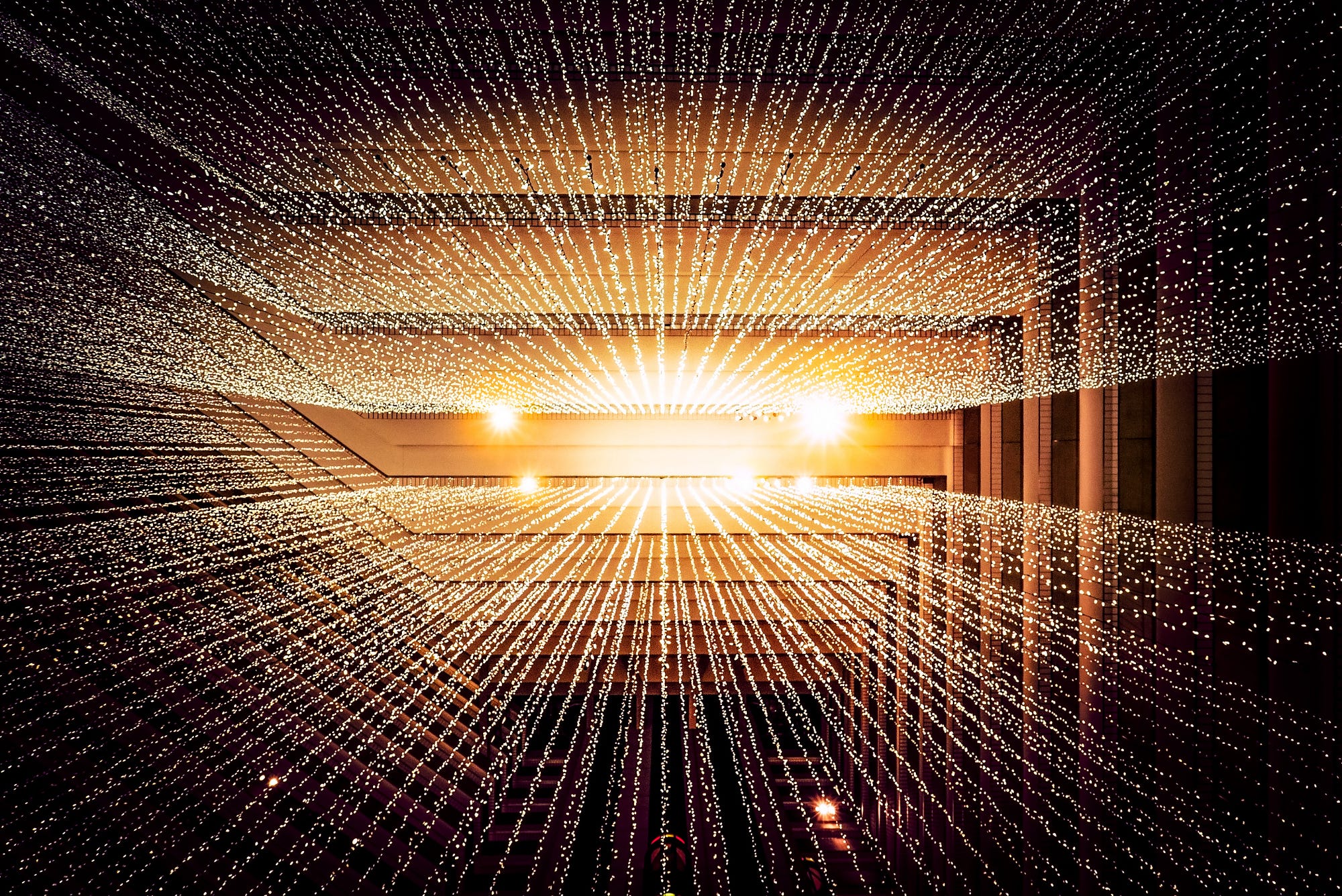
We live in an age where ‘information’ is packaged, shared and valued, quite literally, more than anything else! And, there is enhanced engagement in this information exchange. All this activity is resulting in tons of data being pumped out — Big Data. To those listening, this data can be harnessed and mined for answers. Whether it is regarding business profitability, marketing strategy or identifying and mitigating risk, companies can ascertain any and every detail. Aiding in these pursuits is the growing computational power of systems. There is abundant storage available for all the data. In-memory is adding to the speed of performance. Cloud and pay-as-you-go models are making engagements feasible. And, the economies of scale are making these systems highly accessible and affordable. High-tech companies, technological corporations, and data scientists, all, predict the remarkable, dominant and disruptive power of ML and Big Data combined.
Confronting the Greatest Risks To Financial Services’ Future
In a behavioral study done among international bankers, it was found that bank executives take significantly less risk when reminded of their role as bankers. In the study, they invested about 20% less in the risky asset category relative to the control group. In other words, when they were ‘acting in a ‘banker mentality’ – reminded about banking, and their bank, and their banking careers – they will be more conservative than they would otherwise be. When the same people were not reminded of their banker role, they took greater risk, indicating that the risk in banking doesn’t come from culture but from structure. The question become, is there something about the culture and structure of banks that makes bankers risk-averse? Or is this something that is just evident now? From my perspective, I have seen that “bankers being bankers” tends to result in lower acceptance of change; an adherence to legacy policies, processes, and thought patterns; and the resultant risk of not being able to keep up with consumer demands.
Thinking outside-of-the-black-box of machine learning

“Speech separation or overlapped speech recognition is paramount for far-field conversational speech recognition,”, said Yoshioka. “It has a wide range of potential applications, such as meeting assistance and medical dialog transcription. As computers begin to sense the world better and get smarter, they will be able to provide us more effective assistance and help us focus on more important things.” In the accompanying paper titled, “Layer Trajectory LSTM”, Microsoft AI researchers Jinyu Li and fellow researchers Changliang Liu and Yifan Gong, successfully reassessed the potential for innovation in traditional time-based LSTM networks. Jinyu Li described his conceptual approach saying, “Sometimes deep learning is treated as a black box and researchers just keep trying different model structures without taking a couple of steps back and thinking about why the models work – and what else might be possible.” Traditional LSTM networks in recurrent neural networks (RNNs), well-suited to classifying and making predictions based on time series data such as speech
Eclipse Releases Versions 1.4 and 2.0 of MicroProfile
Both of these Eclipse projects have merit and are making progress in their respective domains, with MicroProfile technologies building upon those being contributed to Jakarta EE. But are the projects themselves ready to be merged? IMHO, no. MicroProfile has grown tremendously from its humble beginnings. We have several new component features and versions that extend the Enterprise Java programming model for microservices development. And we have done this in a relatively short amount of time: Six major MicroProfile releases with sixteen component releases in less than two years. Due to the enormity and complexities of this move, Jakarta EE is not yet ready to match this rate of progress. And, as Jakarta EE has not yet completed the definition of its specification process, it is not yet ready to accept the fast-paced release cycle required by MicroProfile. The big difference here is that MicroProfile has never tried to be a standards body.
Think AI Is Too Scary? This Expert Wants to Calm Your Fears
The first thing to tell you is that I really see this as a listening experience, at least initially, so I can be responsive to what the community is looking for. Having said that, one big area is to enhance and strengthen AAAI links with industry. Our annual conference has a lot of participants from industry but I'd like to see more presence from industry research labs. Traditionally it's been a very academic conference but today, many professors spend time in industry. We need to give that sector a lot more presence. That's a major focus. I am also looking to include underserved communities in our membership to diversify it strongly; launch K-12 initiatives to grow the pipeline; and ensure we include professionals in other areas. ... We need to look at employing ethics within AI at every level: how systems need to be designed with different mechanisms to respond ethically to events; understand when an AI system could do harm; and so on.
Quote for the day:
"The great leaders have always stage-managed their effects." -- Charles de Gaulle
No comments:
Post a Comment