Time to Modernize Your Data Integration Framework
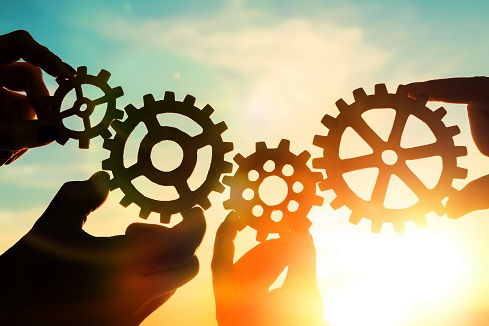
You need to be able to orchestrate the ebb and flow of data among multiple nodes, either as multiple sources, multiple targets, or multiple intermediate aggregation points. The data integration platform must also be cloud native today. This means the integration capabilities are built on a platform stack that is designed and optimized for cloud deployments and implementation. This is crucial for scale and agility -- a clear advantage the cloud gives over on-premises deployments. Additionally, data management centers around trust. Trust is created through transparency and understanding, and modern data integration platforms give organizations holistic views of their enterprise data and deep, thorough lineage paths to show how critical data traces back to a trusted, primary source. Finally, we see modern data analytic platforms in the cloud able to dynamically, and even automatically, scale to meet the increasing complexity and concurrency demands of the query executions involved in data integration. The new generation of some data integration platforms also work at any scale, executing massive numbers of data pipelines that feed and govern the insatiable appetite for data in the analytic platforms.
Will codeless test automation work for you?
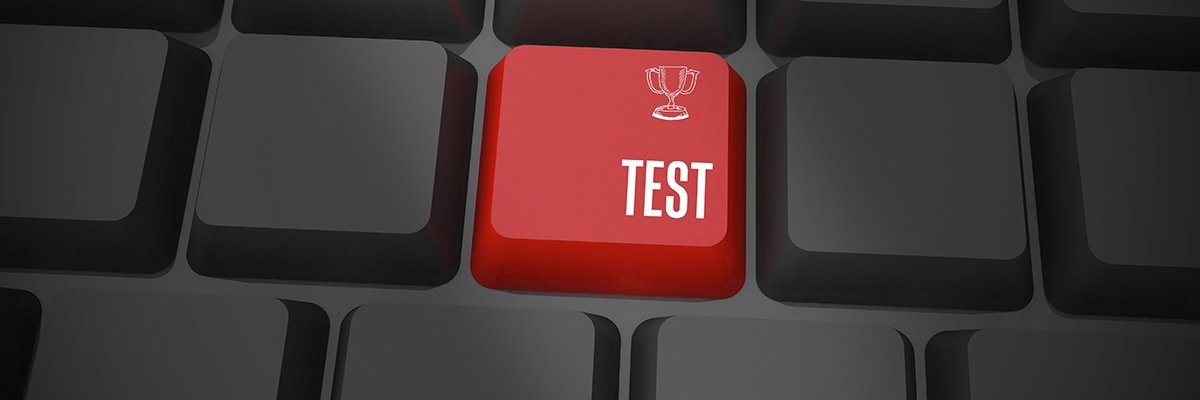
While outsiders view testing as simple and straightforward, it's anything but
true. Until as recently as the 1980s, the dominant idea in testing was to do the
same thing repeatedly and write down the results. For example, you could type
2+3 onto a calculator and see 5 as a result. With this straightforward, linear
test, there are no variables, looping or condition statements. The test is so
simple and repeatable, you don't even need a computer to run this test. This
approach is born from thinking akin to codeless test automation: Repeat the same
equation and get the same result each time for every build. The two primary
methods to perform such testing are the record and playback method, and the
command-line test method. Record and playback tools run in the background and
record everything; testers can then play back the recording later. Such tooling
can also create certification points, to check the expectation that the answer
field will become 5. Record and playbook tools generally require no programming
knowledge at all -- they just repeat exactly what the author did. It's also
possible to express tests visually. Command-driven tests work with three
elements: the command, any input values and the expected results.
Ghost in the Shell: Will AI Ever Be Conscious?
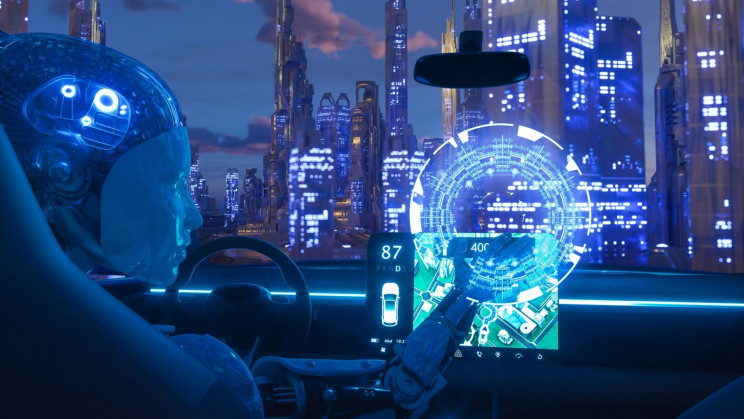
It’s certainly possible that the scales are tipping in favor of those who
believe AGI will be achieved sometime before the century is out. In 2013, Nick
Bostrom of Oxford University and Vincent Mueller of the European Society for
Cognitive Systems published a survey in Fundamental Issues of Artificial
Intelligence that gauged the perception of experts in the AI field regarding the
timeframe in which the technology could reach human-like levels. The report
reveals “a view among experts that AI systems will probably (over 50%) reach
overall human ability by 2040-50, and very likely (with 90% probability) by
2075.” Futurist Ray Kurzweil, the computer scientist behind music-synthesizer
and text-to-speech technologies, is a believer in the fast approach of the
singularity as well. Kurzweil is so confident in the speed of this development
that he’s betting hard. Literally, he’s wagering Kapor $10,000 that a machine
intelligence will be able to pass the Turing test, a challenge that determines
whether a computer can trick a human judge into thinking it itself is human, by
2029.
Is your technology partner a speed boat or an oil tanker?
The opportunity here really cannot be underestimated. It is there for the taking
by organisations who are willing to approach technological transformation in a
radically different way. This involves breaking away from monolithic technology
platforms, obstructive governance procedures, and the eye-wateringly expensive
delivery programmes so often facilitated by traditional large consulting firms.
The truth is, you simply don’t need hundreds of people to drive significant
change or digital transformation. What you do need is to adopt new technology
approaches, re-think operating models and work with partners who are agile
experts, who will fight for their clients' best interests and share their
knowledge to upskill internal staff. Hand picking a select group of top
individuals to work in this way provides a multiplier of value when compared to
hiring greater numbers of less experienced staff members. Of course, external
partners must be able to deliver at the scale required by the clients they work
with. But just as large organisations have to change in order to embrace the
benefits of the digital age, consulting models too must adapt to offer the
services their clients need at the value they deserve.
Best data migration practices for organizations
the internal IT team needs to work closely with the service provider. To
thoroughly understand and outline the project requirements and deliverables.
This is to ensure that there is no aspect that is overlooked, and both sides are
up to speed on the security and regulatory compliance requirements. Not just the
vendor, but the team members and all the tools used in the migration need to
meet all the necessary certifications to carry out a government project. Of
course, certain territories will have more stringent requirements than others.
Finally, an effective transition or change management strategy will be important
to complete the transition. Proper internal communications and comprehensive
training for employees will help everyone involved be aware of what’s required
from them, including grasping any new processes or protocols and
circumnavigating any productivity loss during the data migration. While the
nitty-gritty of a public sector migration might be similar to a private
company’s, a government data migration can be a much longer and unwieldy
process, especially with the vast number of people and the copious amounts of
sensitive data involved.
Will AI dominate in 2021? A Big Question
Agreeing with the fact that the technologies are captivating us completely with
their interesting innovations and gadgets. From Artificial intelligence to
machine learning, IoT, big data, virtual and augmented reality, Blockchain, and
5G; everything seems to take over the world way too soon. Keeping it to the
topic of Artificial Intelligence, this technology has expanded its grip on our
lives without even making us realize that fact. In the days of the pandemic, the
IT experts kept working from home and the tech-grounds kept witnessing smart
ideas and AI-driven innovations. Artificial Intelligence is also the new
normal. Artificial Intelligence is going to be the center of our new normal
and it will be driving the other nascent technologies to the point towards
success. Soon, AI will be the genius core of automated and robotic operations.
In the blink of an eye, Artificial Intelligence can be seen adopted by companies
so rapidly and is making its way into several sectors. 2020 has seen this
deployment on a wider scale as the AI experts were working from home but the
progress didn’t see a stop in the tech fields.
The promise of the fourth industrial revolution
There are some underlying trends in the following vignettes. The internet of
things and related technologies are in early use in smart cities and other
infrastructure applications, such as monitoring warehouses, or components of
them, such as elevators. These projects show clear returns on investment and
benefits. For instance, smart streetlights can make residents’ lives better by
improving public safety, optimizing the flow of traffic on city streets, and
enhancing energy efficiency. Such outcomes are accompanied with data that’s
measurable, even if the social changes are not—such as reducing workers’
frustration from spending less time waiting for an office elevator. Early
adoption is also found in uses in which the harder technical or social problems
are secondary, or, at least, the challenges make fewer people nervous. While
cybersecurity and data privacy remain important for systems that control water
treatment plants, for example, such applications don’t spook people with
concerns about personal surveillance. Each example has a strong connectivity
component, too. None of the results come from “one sensor reported this”—it’s
all about connecting the dots.
How Hundred-Year-Old Enterprises Improve IT Ops using Data and AIOps

Sam Chatman, VP of IT Ops at OneMain Financial, explains the impact of levering
AIOps is, “Being able to understand what is released, when it’s released, and
the potential impacts of that release. We are overcoming alert fatigue, and
BigPanda will be our Watson of the Enterprise Monitoring Center (EMC) by
automating alerts, opening incident tickets, and identifying those actions to
improve our mean time to recovery. This helps us keep our systems up when our
users and customers need them to be.” For other organizations, it might help to
visualize what naturally happens to IT operations’ monitoring programs over
time. Every time systems go down and IT gets thrown under the bus for a major
incident, they add new monitoring systems and alerts to improve their response
times. As new multicloud, database, and microservice technologies emerged, they
add even more monitoring tools and increased observability capabilities. Having
more operational data and alerts is a good first step, but then alert fatigue
kicks in when tier-one support teams respond and must make sense over dozens to
thousands of alerts.
A perfect storm: Why graphics cards cost so much now
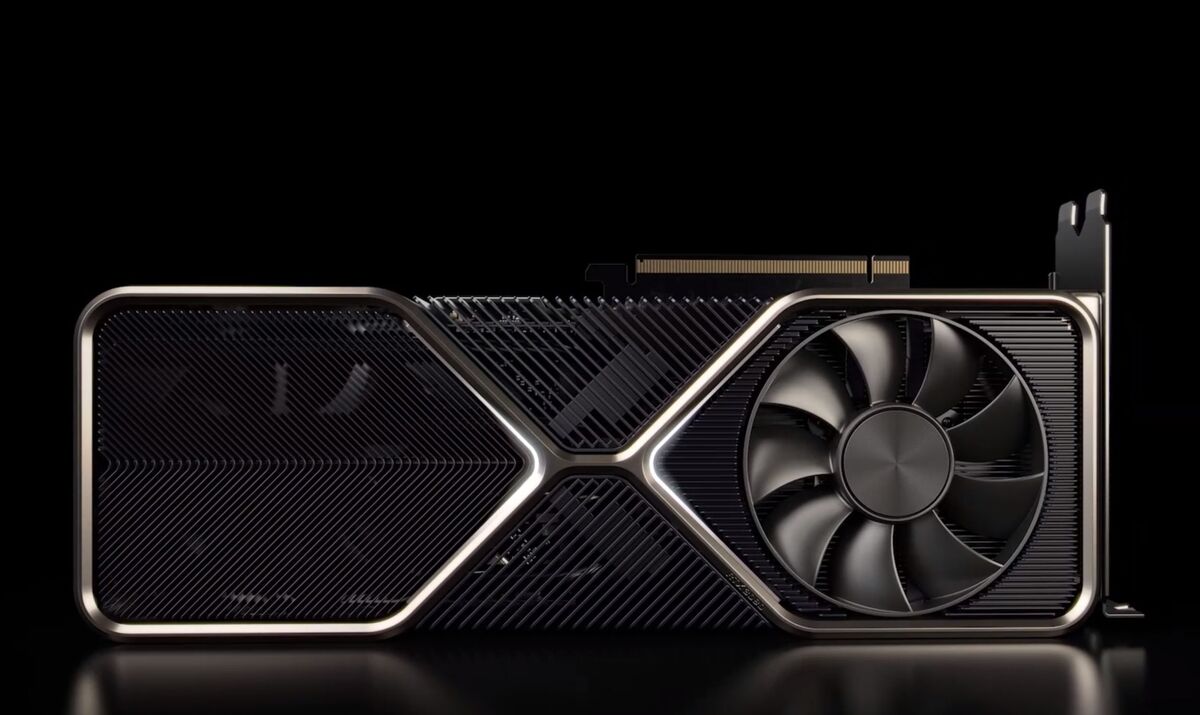
Demand for gaming hardware blew up during the pandemic, with everyone bored and
stuck at home. In the early days of the lockdowns in the United States and
China, Nintendo’s awesome Switch console became red-hot. Even replacement
controllers and some games became hard to find. ... Beyond the AMD-specific TSMC
logjam, the chip industry in general has been suffering from supply woes. Even
automakers and Samsung have warned that they’re struggling to keep up with
demand. We’ve heard whispers that the components used to manufacture chips—from
the GDDR6 memory used in modern GPUs to the substrate material fundamentally
used to construct chips—have been in short supply as well. Seemingly every
industry is seeing vast demand for chips of all sorts right now. ... High demand
and supply shortages are the perfect recipe for folks looking to flip graphics
cards and make a quick buck. The second they hit the streets, the current
generation of GPUs were set upon by “entrepreneurs” using bots to buy up stock
faster than humans can, then selling their ill-gotten wares for a massive markup
on sites like Ebay, StockX, and Craigslist.
How to sharpen machine learning with smarter management of edge cases

Production is when AI models prove their value, and as AI use spreads, it
becomes more important for businesses to be able to scale up model production to
remain competitive. But as Shlomo notes, scaling production is exceedingly
difficult, as this is when AI projects move from the theoretical to the
practical and have to prove their value. “While algorithms are deterministic and
expected to have known results, real world scenarios are not,” asserts Shlomo.
“No matter how well we will define our algorithms and rules, once our AI system
starts to work with the real world, a long tail of edge cases will start
exposing the definition holes in the rules, holes that are translated to
ambiguous interpretation of the data and leading to inconsistent modeling.”
That’s much of the reason why more than 90% of c-suite executives at leading
enterprises are investing in AI, but fewer than 15% have deployed AI for
widespread production. Part of what makes scaling so difficult is the sheer
number of factors for each model to consider. In this way, HITL enables faster,
more efficient scaling, because the ML model can begin with a small, specific
task, then scale to more use cases and situations.
Quote for the day:
"A true dreamer is one who knows how to
navigate in the dark" -- John Paul Warren
No comments:
Post a Comment