Solving the security challenges of public cloud

Compounding matters is the lack of a unified framework for dealing with public
cloud security. End users and cloud consumers are forced to deal with increased
spend on security infrastructure such as SIEMs, SOAR, security data lakes,
tools, maintenance and staff — if they can find them — to operate with an
“adequate” security posture. Public cloud isn’t going away, and neither is the
increase in data and security concerns. But enterprise leaders shouldn’t have to
continue scrambling to solve these problems. We live in a highly standardized
world. Standard operating processes exist for the simplest of tasks, such as
elementary school student drop-offs and checking out a company car. But why
isn’t there a standardized approach for dealing with security of the public
cloud — something so fundamental now to the operation of our society? The ONUG
Collaborative had the same question. Security leaders from organizations such as
FedEx, Raytheon Technologies, Fidelity, Cigna, Goldman Sachs and others came
together to establish the Cloud Security Notification Framework. The goal is to
create consistency in how cloud providers report security events, alerts and
alarms, so end users receive improved visibility and governance of their
data.
Building A Global AI Brand With End-To-End Data Science & Engineering Solutions
At present, people think more from the model design perspective when speaking
about data science and analytics than about data engineering. Going forward,
model design will not matter much because most algorithms will be available as
APIs. In fact, companies like Tredence are building algorithms that have a high
degree of verticalization across industries and can be made available as APIs.
AI as API is a good differentiation. It allows data scientists to spend less
time building algorithms from scratch. Having said that, readily available
algorithms can offer only up to 90% accuracy. The true test of a data scientist
would be whether he/she can take the accuracy from 90 to 99%. It requires domain
expertise, analytical thinking, and the ability to identify edge use cases.
Working around biases and long-tail use cases of AI systems would also become
very important. While designing algorithms, data scientists often assume that
the end-user is AI and not human. There is a need for humanising these systems.
Design thinking has seeped into how software is built, next up it should enter
AI algorithms.
How to Keep an Innovative Mindset Present In Your Business

It’s essential that managers and executives take accountability and engage
employees in more creative ways and foster innovative mindsets. You can do so in
many ways, starting with rewarding innovative progress and changing company
dynamics. First, you can offer bonuses or other incentives to employees who come
up with new ideas for the company. These innovations don’t need to be fully
formed or implemented right away. However, this kind of reward system encourages
more of the same behavior. Employees will seek to create and flourish once they
have the time, resources, and motivation. Then, you can change the way employees
interact with the business itself. In traditional models, shareholders or
executives own the business. In newer, more innovative dynamics, though,
employees can now own parts of the company as well through shares that accrue
over time. ... Technology is one of the best signs of innovation. It combines
practicality, accessibility, and functionality, which helps it constantly
evolve. Something like a smartphone builds on countless previous innovations and
uses them to keep creating. The same concept applies to the workplace.
RBI to issue cybersecurity norms for payment services
While the standards for fintech-driven payment services providers will be
similar to cyber hygiene norms issued recently for banks and non-banking finance
companies, the RBI is quite clear that firms will have to do more than observe
the minimum standards to ensure safety as digital transactions gain further
traction. “On cyber frauds, Reserve Bank of India has issued very recently basic
guidelines on cyber hygiene and cybersecurity for banks and certain NBFCs,” said
RBI executive director T. Rabi Sankar. “We would follow that up with respect to
other entities such as payments systems operators in the payments space. Those
are getting finalised and will be issued soon,” he added. “Having said that, the
minimum standards set by the regulator for the regulated entities are needed,
but they would never be enough. As digitisation increases in any sphere,
payments or otherwise, as people do more and more digital transactions,
institutions themselves will have to do more than the minimum standards that
regulators set, to deal with any cybersecurity threats,” he said, adding that
individual users would also need to be alert as there is no alternative to being
aware of the risks in undertaking digital transactions.
The differences between data analytics, machine learning and AI
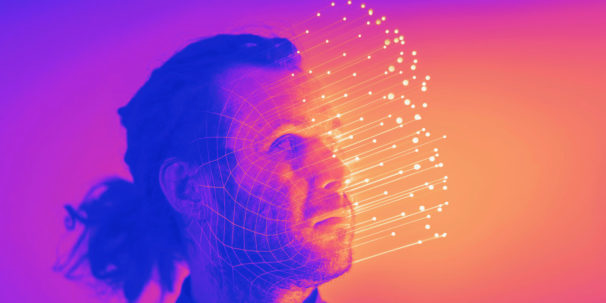
So, we have three distinct areas of expertise we’ve outlined there. Each has its
own applications, subsets, and specialisations, making them very different
fields. However, as you may have noticed already, there are certainly some areas
where they overlap. Below, we’ve outlined just some of the ways in which machine
learning, data analytics, and AI overlap: Data-driven. Each of these areas
relies on analysing huge amounts of data. The more information available, the
more effective they are at producing results. It often takes a lot of computer
processing power to manage such large data sets; Insights. Data analytics,
AI, and machine learning can all be used to produce detailed insights in
particular areas. By examining data, each can identify patterns, highlight
trends, and provide valuable and actionable outcomes; Predictive models.
These technologies can also help to create forecasts and predictions based on
existing data. Again, this process can help organisations of all kinds plan for
the future and make informed decisions. Of course, many other areas relate
closely to those of AI, ML, and data analytics.
GoodData unveils analytics as a set of microservices in data-as-a-service platform

The ability to deploy GoodData.CN anywhere is crucial because multiple centers
of data gravity will always exist in the enterprise, noted Stanek. It’s unlikely
any major enterprise is ever going to be able to standardize on a single data
warehouse or data lake, he said. The GoodData.CM platform provides all the
metadata capabilities required to maintain a single source of truth across what
are rapidly becoming highly federated environments, noted Stanek. A programmable
API also makes it feasible to deploy a headless data-as-a-service platform for
processing analytics that can be readily accessed and consumed as a service by
multiple applications. Previously, individual developers had to take the time
and effort to embed analytics capabilities directly within their application,
noted Stanek. The GoodData.CM platform makes applications more efficient and, as
a consequence, smaller. That is because more analytics processing is offloaded
to the headless platform, added Stanek. Pressure to embed analytics in every
application is mounting as end users seek to make faster and better fact-based
decisions.
Why Technology is More Important Than Ever to Financial Services Organisations
Larger financial institutions have sometimes drawn criticism for their pace with
digital innovation, with suggestions that a risk-averse culture impedes
innovative new projects. But it is important to note that they are not out of
the game. They can still rely on their great customer access, brand cachet and
understanding of regulations to compete with nimble challengers. Additionally,
data is at the heart of digital transformation, a resource that retail and
private banking companies have in abundance. Blending deep, data-powered insight
with their powerful human-centric brands gives these organisations an
opportunity to create real differentiation when it comes to customer experience.
If this is done correctly, they can become smarter, faster and more resilient,
while retaining their brand identity. Attitudes are also changing. Some 79% of
all organisations in our research now believe traditional business models are
being radically disrupted, and that innovation is clearly underway. A further
92% believe that their business embraces change rather than tries to resist
it.
The future of work is uniquely human

Re-architecting work is not about simply automating tasks and activities. At its
core, it is about configuring work to capitalize on what humans can accomplish
when work is based on their strengths. In the survey, executives identified two
factors related to human potential as the most transformative for the workplace:
building an organizational culture that celebrates growth, adaptability and
resilience (45%), and building workforce capability through upskilling,
reskilling, and mobility (41%). Leaders should find ways to create a shared
sense of purpose that mobilizes people to pull strongly in the same direction as
they face the organization’s current and future challenges, whether the mission
is, like Delta’s, to keep people connected, or centered on goals such as
inclusivity, diversity or transparency. They should trust people to work in ways
that allow them to fulfill their potential, offering workers a degree of choice
over the work they do to align their passions with organizational needs. And
they should embrace the perspective that reimagining work is key to the ability
to achieve new and better outcomes—in a world that is itself being constantly
reimagined.
Why applied AI requires skills and knowledge beyond data science
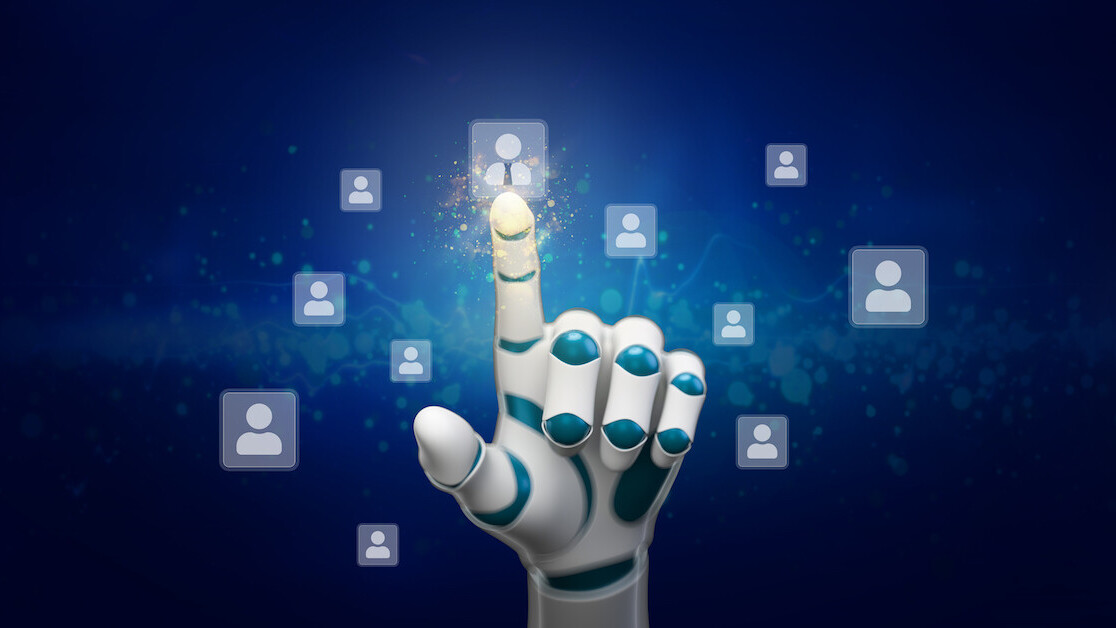
“A business problem that can be solved by a model alone is very unusual. Most
problems are multifaceted and require an assortment of skills—data pipelines,
infrastructure, UX, business risk analysis,” Rochwerger and Pang write in Real
World AI. “Put another way, machine learning is only useful when it’s
incorporated into a business process, customer experience or product, and
actually gets released.” Applied machine learning needs a cross-functional team
that includes people from different disciplines and backgrounds. And not all of
them are technical. Subject matter experts will need to verify the veracity of
training data and the reliability of the model’s inferences. Product managers
will need to establish the business objectives and desired outcomes for the
machine learning strategy. User researchers will help to validate the model’s
performance through interviews with and feedback from end-users of the system.
And an ethics team will need to identify sensitive areas where the machine
learning models might cause unwanted harm.
Machine learning, explained
Machine learning starts with data — numbers, photos, or text, like bank
transactions, pictures of people or even bakery items, repair records, time
series data from sensors, or sales reports. The data is gathered and prepared to
be used as training data, or the information the machine learning model will be
trained on. The more data, the better the program. From there, programmers
choose a machine learning model to use, supply the data, and let the computer
model train itself to find patterns or make predictions. Over time the human
programmer can also tweak the model, including changing its parameters, to help
push it toward more accurate results. (Research scientist Janelle Shane’s
website AI Weirdness is an entertaining look at how machine learning algorithms
learn and how they can get things wrong — as happened when an algorithm tried to
generate recipes and created Chocolate Chicken Chicken Cake.) Some data is held
out from the training data to be used as evaluation data, which tests how
accurate the machine learning model is when it is shown new data. The result is
a model that can be used in the future with different sets of data.
Quote for the day:
"You may be good. You may even be better
than everyone esle. But without a coach you will never be as good as you could
be." -- Andy Stanley
No comments:
Post a Comment