Quantum Computing: What Does It Mean For AI (Artificial Intelligence)?
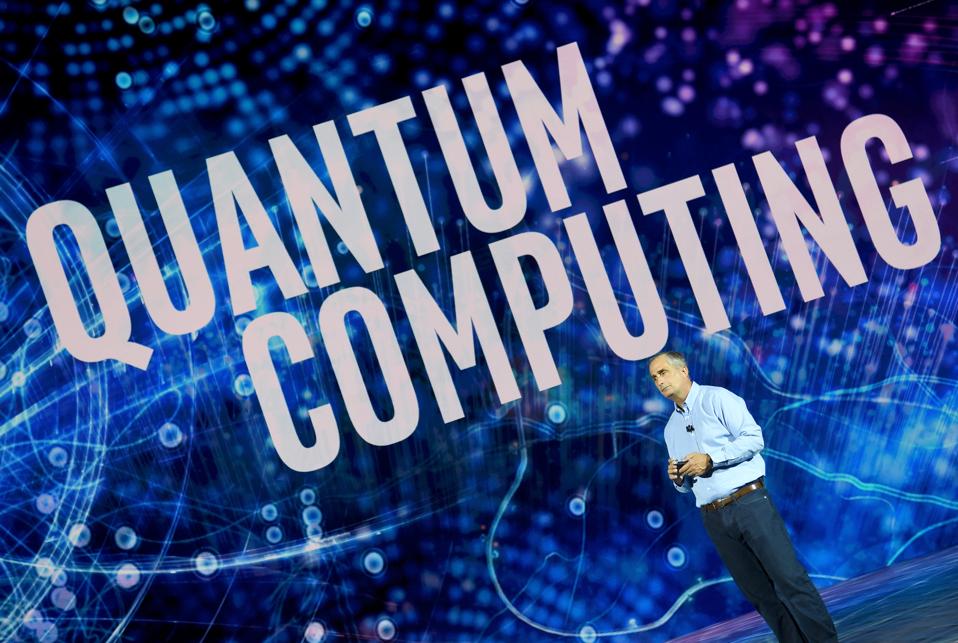
Roughly speaking, AI and ML are good ways to ask a computer to provide an
answer to a problem based on some past experience. It might be challenging
to tell a computer what a cat is, for instance. Still, if you show a neural
network enough images of cats and tell it they are cats, then the computer
will be able to correctly identify other cats that it did not see before. It
appears that some of the most prominent and widely used AI and ML algorithms
can be sped-up significantly if run on quantum computers. For some
algorithms we are even anticipate exponential speed-ups, which clearly does
not mean performing a task faster, but rather turning a previously
impossible task and making it possible, or even easy. While the potential is
undoubtedly immense, this still remains to be proven and realized with
hardware. ... One of the areas being looked at currently is in the area of
artificial intelligence within financial trading. Quantum physics is
probabilistic, meaning the outcomes constitute a predicted distribution. In
certain classes of problems, where outcomes are governed by unintuitive and
surprising relationships among the different input factors, quantum
computers have the potential to better predict that distribution thereby
leading to a more correct answer.
Help Reinforce Privacy Through the Lens of GDPR

There are several key questions about GDPR compliance which delivery teams
should consider. Where do you start on the GDPR compliance journey? What
GDPR TOM controls apply to project delivery and how can your team implement
them? What are the solution design guidelines for applicable GDPR TOMs? And,
what GDPR compliance evidence do you need to show? Initial concern on the
first anniversary (May 2019) of GDPR has faded. The second anniversary (May
2020) is the beginning of the enforcement wave. Delivery teams play a key
role in that enforcement. To answer the above questions, let us first
understand the compliance elements across the people, process and
technology pillars and view the compliance model through a delivery team
lens. ... The GDPR compliance model hooks the elements of people, process
and technology into the delivery lifecycle phases. By doing this, it
addresses delivery teams’ concerns about achieving and showing GDPR
compliance. It provides the guidelines for the inclusion of GDPR TOMs in a
project lifecycle. Below is a sample compliance model that demonstrates how
a client can integrate the compliance elements into the delivery lifecycle
phases.
For six months, security researchers have secretly distributed an Emotet vaccine across the world

Through trial and error and thanks to subsequent Emotet updates that refined
how the new persistence mechanism worked, Quinn was able to put together a
tiny PowerShell script that exploited the registry key mechanism to crash
Emotet itself. The script, cleverly named EmoCrash, effectively scanned a
user's computer and generated a correct -- but malformed -- Emotet registry
key. When Quinn tried to purposely infect a clean computer with Emotet, the
malformed registry key triggered a buffer overflow in Emotet's code and
crashed the malware, effectively preventing users from getting infected.
When Quinn ran EmoCrash on computers already infected with Emotet, the
script would replace the good registry key with the malformed one, and when
Emotet would re-check the registry key, the malware would crash as well,
preventing infected hosts from communicating with the Emotet
command-and-control server. Effectively, Quinn had created both an Emotet
vaccine and killswitch at the same time. But the researcher said the best
part happened after the crashes. "Two crash logs would appear with event ID
1000 and 1001, which could be used to identify endpoints with disabled and
dead Emotet binaries," Quinn said.
Ambiguous times are no time for ambiguous leadership
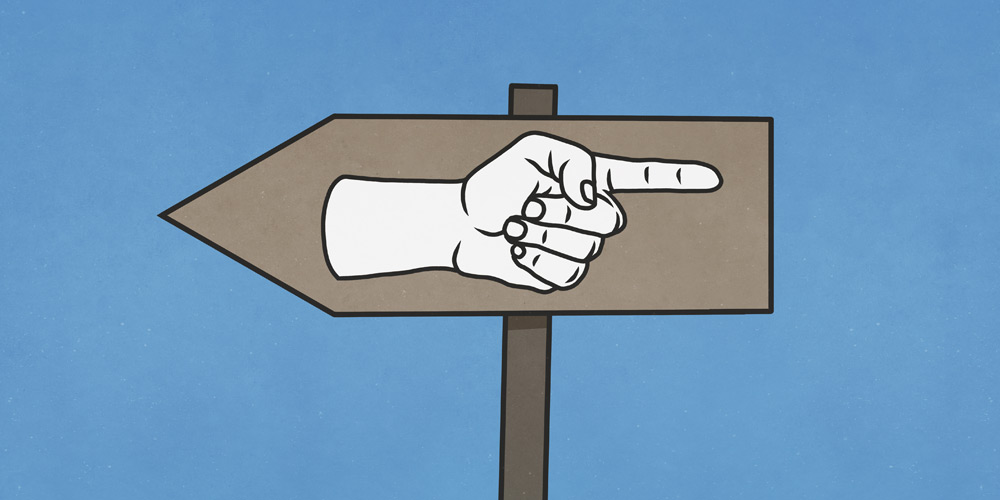
The nearly overnight rush to remote working has had clear benefits. It
reduces the wear and tear of commuting for both people and the planet. It
can also give employees more of a feeling of control over their lives, and,
when geography is no longer a consideration, companies can find new
opportunities for hiring talent. But if remote working is going to work,
leaders have to communicate more and be extra vigilant about removing as
much ambiguity as they can from their exchanges with staff, particularly in
email, in which the recipients don’t have the benefit of hearing the
sender’s tone. Leaders have to ensure that what is clear to them is also
clear to others, in language that doesn’t leave people scratching their
heads. The same is true for video meetings, conducted in small squares on
your computer screen that can make it hard to read nuances of body language.
There are some basic rules of human nature at play here. One of them is that
with less face-to-face contact with bosses, employees are more likely to
feel free-floating anxiety and wonder, “What do they think of me?” They may
study email as if they were amateur archaeologists, searching for hidden
meaning, often when none exists.
A reference architecture for multicloud

Data-focused multicloud deals with everything that’s stored inside and
outside of the public clouds. Cloud-native databases exist here, as do
legacy databases that still remain on-premises. The idea is to manage these
systems using common layers, such as management and monitoring, security,
and abstraction. Service-focused multicloud means that we deal with
behavior/services and the data bound to those services from the lower layers
of the architecture. It’s pretty much the same general idea as data-focused
multicloud, in that we develop and manage services using common layers of
technology that span from the clouds back to the enterprise data center. Of
course, there is much more to both layers. Remember that the objective is to
remove humans from having to deal with the cloud and noncloud complexity
using automation and other approaches. This is the core objective of
multicloud complexity management, and it seems to be growing in popularity
as a rising number of enterprises get bogged down by manual ops processes
and traditional tools. Also note that this diagram depicts a multicloud that
has very little to do with clouds, as I covered a few weeks ago.
5 Essential Business-Oriented Critical Thinking Skills For Data Science
What do we want to optimize for? Most businesses fail to answer this simple
question. Every business problem is a little different and should,
therefore, be optimized differently. For example, a website owner might ask
you to optimize for daily active users. Daily active users is a metric
defined as the number of people who open a product in a given day. But is
that the right metric? Probably not! In reality, it’s just a vanity metric,
meaning one that makes you look good but doesn’t serve any purpose when it
comes to actionability. This metric will always increase if you are spending
marketing dollars across various channels to bring more and more customers
to your site. Instead, I would recommend optimizing the percentage of users
that are active to get a better idea of how my product is performing. A big
marketing campaign might bring a lot of users to my site, but if only a few
of them convert to active, the marketing campaign was a failure and my site
stickiness factor is very low. You can measure the stickiness by the second
metric and not the first one. If the percentage of active users is
increasing, that must mean that they like my website.
How ClauseMatch is disrupting regulatory compliance through AI
Inevitably, the RegTech sector was still in its early days and beset with
challenges for Likhoded. He says banks were far from embracing cloud
technologies and preferred to use traditional methods in its operations. As
he put it: “Seven or eight years ago, not a single bank had departments
working in innovation and technology. “Initially it was challenging to
[convince] large financial institutions to use cloud platforms for their
confidential and internal documentation,” he continues. While financial
institutions were not easy to embrace technology, the times were a-changin,
he says. “Since 2014 we’ve seen a major shift and it’s been driven by the
increase in adoption of cloud technologies which were now cheaper and faster
to deploy,” Likhoded adds. As a result, in 2016 ClauseMatch signed up
Barclays as a client. This deal propelled the startup’s growth and various
other institutions started leveraging its regulation and compliance
function. Having tier one banks as clients proved advantageous when it came
to funding as VCs were able to see the use case for the RegTech startup.
“[VCs] saw that regulations are not reducing but increasing and compliance
departments have ballooned in size.
Answers To Today’s Toughest Endpoint Security Questions In The Enterprise

What’s important for CISOs to think about today is how they can lead their
organizations to excel at automated endpoint hygiene. It’s about achieving a
stronger endpoint security posture in the face of growing threats. Losing
access to an endpoint doesn’t have to end badly; you can still have options to
protect every device. It’s time for enterprises to start taking a more
resilient-driven mindset and strategy to protecting every endpoint – focus on
eliminating dark endpoints. One of the most proven ways to do that is to have
endpoint security embedded to the BIOS level every day. That way, each device
is still protected to the local level. Using geolocation, it’s possible to
“see” a device when it comes online and promptly brick it if it’s been lost or
stolen. ... What CISOs and their teams need is the ability to see endpoints in
near real-time and predict which ones are most likely to fail at compliance.
Using a cloud-based or SaaS console to track compliance down to the BIOS level
removes all uncertainty of compliance. Enterprises doing this today stay in
compliance with HIPAA, GDPR, PCI, SOX and other compliance requirements at
scale.
Recover your Data from a Back-up, not with a Ransom
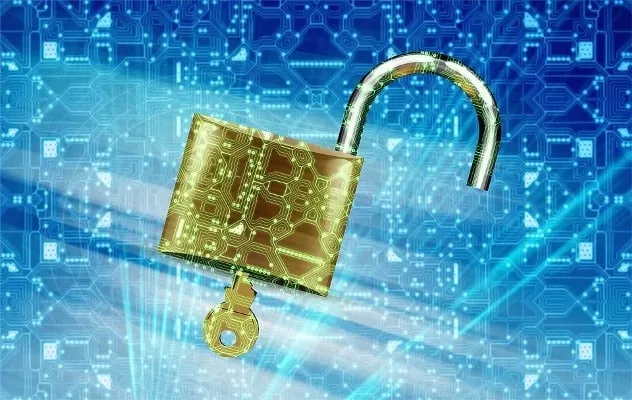
Securing against ransomware must consequently be top of the agenda for not
only IT leaders but also the c-suite executives in an organization. Endpoint
security and end user education are important elements of a multi-pronged
strategy to protect against ransomware, but data back-up is perhaps the key
here. Given the persistence of cybercriminals, ransomware attacks are being
perpetrated over a longer period and have taken the form of cyberattack
campaigns. The chances of them succeeding have also grown manifold. A
fragmented approach to data security adds to the risk. For instance, data
protection and cybersecurity are two important elements that are intermeshed,
but typically handled by two different teams. Lack of coordination between the
two creates a disjointed view of the data security big picture in an
organization. An integrated cybersecurity and data protection strategy is key
to closing the security gap and ensuring various pieces of the data security
puzzle fit together. But what if the unthinkable happens and a ransomware
attack succeeds in penetrating these security layers? A Business Continuity
and Disaster Recovery (BCDR) plan alongside effective cybersecurity is key in
case of an inevitable attack.
MLops: The rise of machine learning operations

As a software developer, you know that completing the version of an application
and deploying it to production isn’t trivial. But an even greater challenge
begins once the application reaches production. End-users expect regular
enhancements, and the underlying infrastructure, platforms, and libraries
require patching and maintenance. Now let’s shift to the scientific world where
questions lead to multiple hypotheses and repetitive experimentation. You
learned in science class to maintain a log of these experiments and track the
journey of tweaking different variables from one experiment to the next.
Experimentation leads to improved results, and documenting the journey helps
convince peers that you’ve explored all the variables and that results are
reproducible. Data scientists experimenting with machine learning models must
incorporate disciplines from both software development and scientific research.
Machine learning models are software code developed in languages such as Python
and R, constructed with TensorFlow, PyTorch, or other machine learning
libraries, run on platforms such as Apache Spark, and deployed to cloud
infrastructure. The development and support of machine learning models require
significant experimentation and optimization, and data scientists must prove the
accuracy of their models.
Quote for the day:
"To do great things is difficult; but to command great things is more difficult." -- Friedrich Nietzsche
No comments:
Post a Comment