When Your Heartbeat Becomes Data: Benefits and Risk of Biometrics

We haven’t even discussed the abilities to detect your walking patterns
(already being used by some police agencies), monitor scents, track microbial
cells or identify you from your body shape. More and more organizations are
looking for contactless methods to authenticate, especially relevant today.
What all these biometrics technologies have in common is that they are using
some combination of physiological and behavioral methods to make sure you are
you. There are certain things people just can’t fake. You can’t fake a
heartbeat, which is as unique as a retinal scan or fingerprint. You can’t
easily fake how you walk. Even your typing and writing styles give off a
distinct and unique signature. ... Some of the best innovators are threat
actors. They may not be able to replicate your heartbeat today, but what about
tomorrow? The not-too-distant future could include a “Mission: Impossible“
scenario with 3D printers that generate a ‘body suit’ (think wetsuit) that can
have a simulated heartbeat uploaded into it. This all may sound like
science fiction right now, but not too long ago, would it have not been silly
to think that your heartbeat could be identified through clothes using a laser
from over 200 yards away?
What skills should modern IT professionals prioritise?

Though technical skills, like those accompanying cyber security and emerging
tech are a focus, IT professionals are coming to realise that non-technical
skills are a critical element of their career development and IT management.
When asked which of these were most important, IT pros listed project management
(69%), interpersonal communication (57%), and people management (53%). According
to the LinkedIn 2020 Emerging Jobs Report, the demand for soft skills like
communication, collaboration, and creativity will continue to rise across the
SaaS industry. Despite the budget and skills issues IT professionals report, 53%
of those surveyed said they’re comfortable communicating with business
leadership when requesting technology purchases, investing time/budget into team
trainings, and the like. Though developing tech skills is often informed by
current areas of expertise, the 2020 IT Trends Report reveals strong IT
performance is about more than IT skills. Interpersonal skills are commonly
referred to as “soft skills”, which is misleading. They rank highly in overall
importance, meaning soft skills aren’t optional. They’re human skills — everyone
needs to relate to other people and speak in a way they can understand. My
advice in this area would be to find a mentor, someone on your team who can help
you learn. Practice your communication skills and try your hand at new
specialties like project management.
Predictive analytics vs. AI: Why the difference matters

Fast forward to today. Within the information governance space, there are two
terms that have been used quite frequently in recent years: analytics and AI.
Often they are used interchangeably and are practically synonymous.
Organizations—as well as the software vendors that supply their needs—have
largely tapped analytics to provide deeper information beyond basic indexed
searching, which typically involves applying Boolean logic to keywords, date
ranges, and data types. Search concepts have expanded to filter out
application-specific metadata (e.g., parsing mail distribution lists,
application login time, login/logout/idle times in chat and collaborative
rooms, etc.). Today's search also includes advanced capabilities such as
stemming and lemmatization—methods for matching queries with different forms
of words—and proximity search, allowing searchers to find the elusive needle
in the haystack. The latest whiz-bang features that are all the buzz within
the information governance space are analytics (or predictive analytics) and
AI (or artificial intelligence/machine learning). These are here to stay, and
we are just beginning to scratch the surface of their many uses.
Too many AI researchers think real-world problems are not relevant

New machine-learning models are measured against large, curated data sets that
lack noise and have well-defined, explicitly labeled categories (cat, dog,
bird). Deep learning does well for these problems because it assumes a largely
stable world (pdf). But in the real world, these categories are constantly
changing over time or according to geographic and cultural context.
Unfortunately, the response has not been to develop new methods that address
the difficulties of real-world data; rather, there’s been a push for
applications researchers to create their own benchmark data sets. The goal of
these efforts is essentially to squeeze real-world problems into the paradigm
that other machine-learning researchers use to measure performance. But the
domain-specific data sets are likely to be no better than existing versions at
representing real-world scenarios. The results could do more harm than good.
People who might have been helped by these researchers’ work will become
disillusioned by technologies that perform poorly when it matters most.
Because of the field’s misguided priorities, people who are trying to solve
the world’s biggest challenges are not benefiting as much as they could from
AI’s very real promise.
Foundations of Deep Learning!!!

One may ask what is the difference between ANN and DL. The name Artificial
Neural Network is inspired from a rough comparison of it’s architecture with
human brain. Although some of the central concepts in ANNs were developed in
part by drawing inspiration from our understanding of the brain, ANN models
are not models of the brain. In reality, there is no great similarity between
an ANN and it’s method of operation with human brain, neurons, synapses and
it’s modus operandi. However the fact that the ANN is a consolidation of one
or more layers of neurons, that help in solving perceptual problems - which is
based human intuition, the name goes well. ANN essentially is a structure
consisting of multiple layers of processing units (i.e. neurons) that take
input data and process it through successive layers to derive meaningful
representations. The word deep in Deep Learning stands for this idea of
successive layers of representation. How many layers contribute to a model of
the data is called the depth of the model. Below diagram illustrates the
structure better as we have a simple ANN with only one hidden layer and a DL
Neural Network (DNN) with multiple hidden layers.
COVID-19 Data Compromised in 'BlueLeaks' Incident
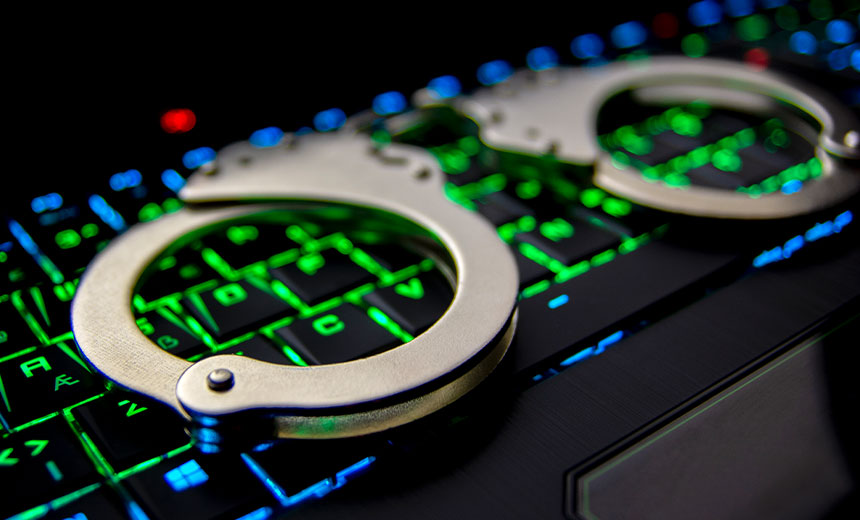
The Department of Homeland Security on June 29 issued an alert about
"BlueLeaks" hacking of Nesential, saying a criminal hacker group called
Distributed Denial of Secrets - also known as "DDS" and "DDoSecrets" - on June
19 "conducted a hack-and-leak operation targeting federal, state, and local
law enforcement databases, probably in support of or in response to nationwide
protests stemming from the death of George Floyd." The hacking group leaked 10
years of data from 200 police departments, fusion centers and other law
enforcement training and support resources around the globe, the DHS alert
noted. The 269 GB data dump was posted on June 19 to DDoSecrets' site, the
hacking group said in a tweet that has since been removed. The data came from
a wide variety of law enforcement sources and included personally identifiable
information and data concerning ongoing cases, DDoSecrets claimed in a tweet.
Several days after DDoSecrets revealed the law enforcement information through
its Twitter account in June, the social media platform permanently removed the
DDoSecrets account, citing Twitter rules concerning posting stolen data.
Top exploits used by ransomware gangs are VPN bugs, but RDP still reigns supreme

At the top of this list, we have the Remote Desktop Protocol (RDP). Reports
from Coveware, Emsisoft, and Recorded Future clearly put RDP as the most
popular intrusion vector and the source of most ransomware incidents in 2020.
"Today, RDP is regarded as the single biggest attack vector for ransomware,"
cyber-security firm Emsisoft said last month, as part of a guide on securing
RDP endpoints against ransomware gangs. Statistics from Coveware, a company
that provides ransomware incident response and ransom negotiation services,
also sustain this assessment; with the company firmly ranking RDP as the most
popular entry point for the ransomware incidents it investigated this year.
... RDP has been the top intrusion vector for ransomware gangs since last year
when ransomware gangs have stopped targeting home consumers and moved en-masse
towards targeting companies instead. RDP is today's top technology for
connecting to remote systems and there are millions of computers with RDP
ports exposed online, which makes RDP a huge attack vector to all sorts of
cyber-criminals, not just ransomware gangs.
Shoring Up the 2020 Election: Secure Vote Tallies Aren’t the Problem

“When looking at the ecosystem of election security, political campaigns can
be soft targets for cyberattacks due to the inability to dedicate resources to
sophisticated cybersecurity protections,” Woolbright said. “Campaigns are
typically short-term, cash strapped operations that do not have an IT staff or
budget necessary to promote long-term security strategies.” For state and
local governments, constituents are accessing online information about voting
processes and polling stations in noticeably larger numbers of late –
Cloudflare said that it has seen increases in traffic ranging from two to
three times the normal volume of requests since April. So perhaps it’s no
coincidence that the firm found that government election-related sites are
experiencing more attempts to exploit security vulnerabilities, with 122,475
such threats coming in per day (including an average of 199 SQL injection
attempts per day bent on harvesting information from site visitors). “We
believe there are a wide range of factors for traffic spikes including, but
not limited to, states expanding vote-by-mail initiatives and voter
registration deadlines due to emergency orders by 53 states and territories
throughout the United States,” Woolbright said.
iRobot launches robot intelligence platform, new app, aims for quarterly updates

"We were focused on the idea that autonomous was the same as intelligence,"
said Angle. "We were told that wasn't intelligent and customers wanted
collaboration." The COVID-19 pandemic pushed the collaboration theme with
customers and robots because there was no choice. People are home more than
ever so more cleaning coordination is needed. Meanwhile, iRobot found
customers were home more yet had less time to clean. More time at home also
meant more messes. Indeed, iRobot has seen strong demand during the COVID-19
pandemic. The company saw premium robot sales jump 43% in the second quarter
with strong performance across its international business. Roomba i7 Series,
s9 Series, and Braava jet m6 also performed well. For the second quarter,
iRobot delivered revenue of $279.9 million, up 8% from a year ago. First-half
revenue for 2020 was $472.4 million. iRobot reported second quarter earnings
of $2.07 a share. Julie Zeiler, CFO of iRobot, said that Roomba was 90% of the
product mix in the second quarter and the company's e-commerce business
performed well.
Google Engineers 'Mutate' AI to Make It Evolve Systems Faster Than We Can Code Them
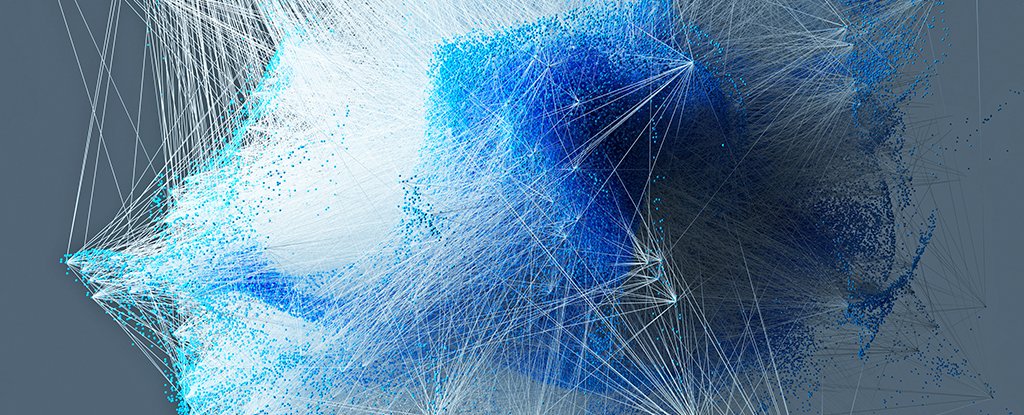
Using a simple three-step process - setup, predict and learn - it can be
thought of as machine learning from scratch. The system starts off with a
selection of 100 algorithms made by randomly combining simple mathematical
operations. A sophisticated trial-and-error process then identifies the best
performers, which are retained - with some tweaks - for another round of
trials. In other words, the neural network is mutating as it goes. When new
code is produced, it's tested on AI tasks - like spotting the difference
between a picture of a truck and a picture of a dog - and the best-performing
algorithms are then kept for future iteration. Like survival of the fittest.
And it's fast too: the researchers reckon up to 10,000 possible algorithms can
be searched through per second per processor (the more computer processors
available for the task, the quicker it can work). Eventually, this should see
artificial intelligence systems become more widely used, and easier to access
for programmers with no AI expertise. It might even help us eradicate human
bias from AI, because humans are barely involved. Work to improve AutoML-Zero
continues, with the hope that it'll eventually be able to spit out algorithms
that mere human programmers would never have thought of.
Quote for the day:
"Luck is what happens when preparation meets opportunity." -- Darrell Royal
No comments:
Post a Comment