AI R&D is booming, but general intelligence is still out of reach
/cdn.vox-cdn.com/uploads/chorus_image/image/65881915/acastro_181017_1777_brain_ai_0003.0.jpg)
For a start, the majority of these milestones come from defeating humans in video games and board games — domains that, because of their clear rules and easy simulation, are particularly amenable to AI training. Such training usually relies on AI agents sinking many lifetimes’ worth of work into a single game, training hundreds of years in a solar day: a fact that highlights how quickly humans learn compared to computers. Similarly, each achievements was set in a single domain. With very few exceptions, AI systems trained at one task can’t transfer what they’ve learned to another. A superhuman StarCraft II bot would lose to a five-year-old playing chess. And while an AI might be able to spot breast cancer tumors as accurately as an oncologist, it can’t do the same for lung cancer (let alone write a prescription or deliver a diagnosis). In other words: AI systems are single-use tools, not flexible intelligences that are stand-ins for humans. But — and yes, there’s another but — that doesn’t mean AI isn’t incredibly useful. As this report shows, despite the limitations of machine learning, it continues to accelerate in terms of funding, interest, and technical achievements.
Data Management Patterns for Microservices Architecture
For the applications where multiple transactions are possible, the Saga Pattern acts as a predominant microservices Data Management pattern. It is a series of local transactions where each transaction publishes an event stating the status of the queries being triggered. The other services are dependent on the previous services’ status, and hence, for the transactions with previously failing status, the saga will automatically undo the further transactions. When a customer places an order in an eCommerce store, the two services called customer service and order service will be working. When a customer service sends the order, the order will be in the pending state. The saga contacts the eCommerce store through the order service and will manage the placing of events. Once the order service gets the confirmation about the order, it sends the reply. Depending on the reply, the saga will approve or reject the order. The final status of the order is presented to the customer stating that the order will be delivered or having the buyer proceed to the payment method.
Algorithmia: 50% of companies spend between 8 and 90 days deploying a single AI model

Despite the fierce search for data science talent in the enterprise, nearly 55% of companies represented in the report say they haven’t yet deployed a machine learning model (up from 51% of companies last year). A full one-fifth are still evaluating use cases or plan to move models into production within the year, and just over 22% have had models in production for two years or fewer. That jibes with a recent study conducted by analysts at International Data Corporation (IDC), which found that of the organizations already using AI, only 25% have developed an “enterprise-wide” AI strategy. Firms responding to that survey blamed the cost of AI solutions and a lack of qualified workers, as well as biased data and unrealistic expectations. As alluded to earlier, moving models into production remains a challenge for most organizations, according to Algorithmia. At least 20% of companies of all sizes say their data scientists spend a quarter of their time deploying models, owing to pervasive scaling blockers like sourcing the hardware, data, and tools and performing the necessary optimizations.
:no_upscale()/cdn.vox-cdn.com/uploads/chorus_asset/file/19484592/GettyImages_1189384731.jpg)
But AI doesn’t just operate behind the scenes. If you’ve ever applied for a job and then been engaged by a text conversation, there’s a chance you’re talking to a recruitment bot. Chatbots that use natural-language understanding created by companies like Mya can help automate the process of reaching out to previous applicants about a new opening at a company, or finding out whether an applicant meets a position’s basic requirements — like availability — thus eliminating the need for human phone-screening interviews. Mya, for instance, can reach out over text and email, as well as through messaging applications like Facebook and WhatsApp. Another burgeoning use of artificial intelligence in job selection is talent and personality assessments. ... These systems typically operate on a scale greater than a human recruiter. For instance, HireVue claims the artificial intelligence used in its video platform evaluates “tens of thousands of factors.” Even if companies are using the same AI-based hiring tool, they’re likely using a system that’s optimized to their own hiring preferences. Plus, an algorithm is likely changing if it’s continuously being trained on new data.
Spatial computing comes to the enterprise
As we've become increasingly familiar with the positive effects AR has on attention and memory encoding, it was exciting to see AR's adoption expand outside of a marketing context. In the workplace we observed practical applications of AR in areas such as employee onboarding, training, and professional development, with empirical evidence highlighting AR's power to drive efficiencies, time to competency and memory recall — galvanizing a disconnected workforce and helping reduce overheads. Pizza chain Papa Murphy's, for example, continue to leverage AR for its employee onboarding program by creating AR-powered stations at key training locations. These types of use cases are becoming increasingly common across a variety of industries — from financial services to healthcare, large consumer goods conglomerates to higher education and vocational learning institutions. As more businesses trial the technology and best use cases get shared, the more adoption we'll see and the more mainstream AR will become as an L&D tool.
Predictions 2020: What's Going to Happen in Cloud Computing
Hyperconvergence emerged several years back to describe several data center elements consolidating into a single box. More recently, we’ve started to see the emergence of DHCI (distributed hyperconverged infrastructure), an approach that I see as is contradictory and antithetical. As our industry moves forward in 2020, a new category will capture the essence of software-defined everything, and I believe it will be the notion of hybrid cloud. Hardware will still be required, but it could be located anywhere; software will continue to coordinate the increasing complexity to the point where location of hardware will increasingly become irrelevant in 2020. ... Containerization and solution portability will become the new battleground for enterprise IT; vendors having "the best" deployment-specific point solutions will lose out to competitors that can span multiple domains (e.g., public cloud, private cloud, on-premises) with ubiquitous offerings, thereby providing freedom and leverage against lock-in. Advertising claims will soar.
AI's real impact? Freeing us from the tyranny of repetitive tasks
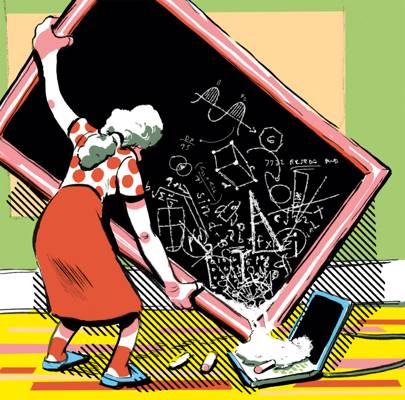
In 2020, AI will begin to live up to the hype by starting to generate real economic value through its application across industries. According to consulting firm PricewaterhouseCoopers, the widespread adoption of AI will add about $15.7 trillion (£12.8 trillion) to global GDP by 2030. Most of that business value will come not from AI-focused companies, but from the infusion of artificial intelligence into traditional industries. Early movers who embrace AI will become the winners. One defining area of AI infusion is in the automation of repetitive tasks, using technologies such as RPA (robotic process automation). RPA will see widespread application in the work performed by functions such as accounts payable, back-office processing and various forms of data management. Routine tasks associated with a large number of jobs will now lend themselves to automation, freeing up people’s time to focus on more complex endeavours. RPA is already creating some of the most valuable AI companies in the world. Another similar area of routine task replacement is the use of speech recognition and natural-language processing in customer service, telemarketing and telesales.
How to Effectively Achieve IT Resilience with Hybrid Cloud and Multi-cloud
As companies look to implement these alternative cloud models, it’s important that they fully understand the time and resource investments needed to ensure they’re not leaving the company susceptible to IT failures or cyberattacks. Orchestrating these environments in a way that meets both IT and business needs is no easy feat. ... There are a host of different cloud options that an organization can choose from. So it’s critical that companies take a pragmatic approach to evaluate their options and ensure they’re picking services that meet both IT and business needs. To do this, they should create a committee of key decision-makers to establish which data, systems, and applications are most critical to operations; set a budget; and discuss where data currently resides. This way, they have a full picture of the current status of their IT infrastructure and can establish parameters around what they’d ideally like the outcome of the project to be. The biggest mistake organizations make is embarking on these projects without identifying internal champions to lead the endeavor.
Like many new technologies, BCIs have attracted interest from the military, and US military emerging technology agency DARPA is investing tens of millions of dollars in developing a brain-computer interface for use by soldiers. More broadly, it's easy to see the appeal of BCIs for the military: soldiers in the field could patch in teams back at HQ for extra intelligence, for example, and communicate with each other without making a sound. Equally, there are darker uses that the army could put BCIs too -- like interrogation and espionage. ... There are currently two approaches to BCIs: invasive and non-invasive. Invasive systems have hardware that's in contact with the brain; non-invasive systems typically pick up the brain's signals from the scalp, using head-worn sensors. The two approaches have their own different benefits and disadvantages. With invasive BCI systems, because electrode arrays are touching the brain, they can gather much more fine-grained and accurate signals. However, as you can imagine, they involve brain surgery and the brain isn't always too happy about having electrode arrays attached to it -- the brain reacts with a process called glial scarring, which in turn can make it harder for the array to pick up signals.
As the saying goes, “You get out what you put in”. An organisation can have masses of data, but unless it is cleansed and normalised it can be useless. We do not take for granted knowing who the right John Smith is and being able to link a name with the correct address and date of birth. As usage-based insurance develops, whether through aftermarket telematics devices, smartphone apps, connected vehicles, even in the future from smart home data, all that data needs to be gathered, normalised, standardised so that consumers can enjoy an improved shopping experience based on their needs and preferences. In motor insurance we call this Driver DNA®, this allows insurers to verify and benchmark existing telematics scores. This market score becomes portable and allows drivers to take their driving score from one insurer and shop for insurance with another – in the same way as no claims discounts are universally applied. Image recognition ML techniques gives us the speed limits of UK roads, in real-time. Without this data we could not know with a good degree of confidence that a person may be travelling at twice the speed limit in an urban area.
Quote for the day:
"Any one can hold the helm when the sea is calm." -- Publilius Syrus
No comments:
Post a Comment