Data Scientists: Machine Learning Skills are Key to Future Jobs

SlashData queried some 20,500 respondents from 167 countries, which means this is a pretty comprehensive survey from a global perspective. Responses were additionally weighted in order to “derive a representative distribution for platforms, segments, and types of IoT [projects],” according to the report accompanying the data. According to the survey, some 45 percent of developers want to either learn or improve their existing data science/machine learning skills. This outpaces the desire to learn UI design (33 percent of respondents), cloud native development such as containers (25 percent), project management (24 percent), and DevOps (23 percent). “The analysis of very large datasets is now made possible and, more importantly, affordable to most due to the emergence of cloud computing, open-source data science frameworks and Machine Learning as a Service (MLaaS) platforms,” the report added. “As a result, the interest of the developer community in the field is growing steadily.”
Did You Forget the Ops in DevOps?
This person with deep operational knowledge was "too busy" fighting fires in production environments, and had not been included in the devops transformation conversations for this large organization. He worked for a different legal entity in a different building, despite being part of the same group, and he was about to leave due to lack of motivation. Yet the organization was claiming to do "devops". The action we took in this case was to take offline a number of experts who were effectively bottlenecks to the flow of work (if you’ve read the book "The Phoenix Project" you will recognize the "Brent" character here). We asked them to build the new components they needed with infrastructure-as-code under a Scrum approach. We even took them to a different city so they wouldn't get disturbed by their regular coworkers. After a couple of months, they rejoined their previous teams but now had a totally new approach of working. Even the oldest Unix sysadmin had now become an agile evangelist that preached infrastructure as code rather than manually hot fixing production.
Is your approach to enterprise architecture relevant in today’s world?

In today’s fast-changing market, the role of enterprise architecture is more important than ever to prevent organisations from creating barriers to future change or expensive technical debt. To remain relevant, modern enterprise architecture approaches must be customer experience (CX)-driven, agile, and deliver the right level of detail just in time for when it needs to be consumed. Static business capabilities are no longer the only anchor point for architecting enterprise technology environments. CX is now a dominant driver of strategy and so businesses need to understand how stakeholders (customers, employees, partners, etc.) consume services and how they can be enabled by technology and platforms. The importance of capturing, managing, analysing and exposing data grows each year. Therefore, enterprise architecture needs to reinvent itself again to incorporate the needs of a rapidly evolving digital world. In a CX-driven planning approach, customer journeys are used to define the services and channels of engagement.
Edge Computing – Key Drivers and Benefits for Smart Manufacturing
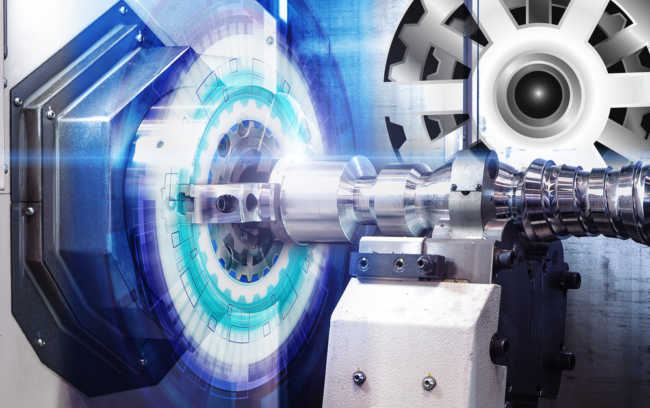
Edge computing means faster response times, increased reliability and security. A lot has been said about how the Internet of Things ( IoT ) is revolutionizing the manufacturing world. Many studies have already predicted more than 50 billion devices will be connected by 2020. It is also expected over 1.44 billion data points will be collected per plant per day. This data will be aggregated, sanitized, processed, and used for critical business decisions. This means unprecedented demand and expectations on connectivity, computational power, and speed of quality of service. Can we afford any latency in critical operations such as operator hand trapped in a rotor, fire situation, or gas leakage? This is the biggest driver for edge computing. More power closer to the data source-the “Thing” in IoT. Rather than a conventional central controlling system, this distributed control architecture is gaining popularity as an alternative to the light version of data center and where control functions are placed closer to the devices.
63% Of Executives Say AI Leads To Increased Revenues And 44% Report Reduced Costs
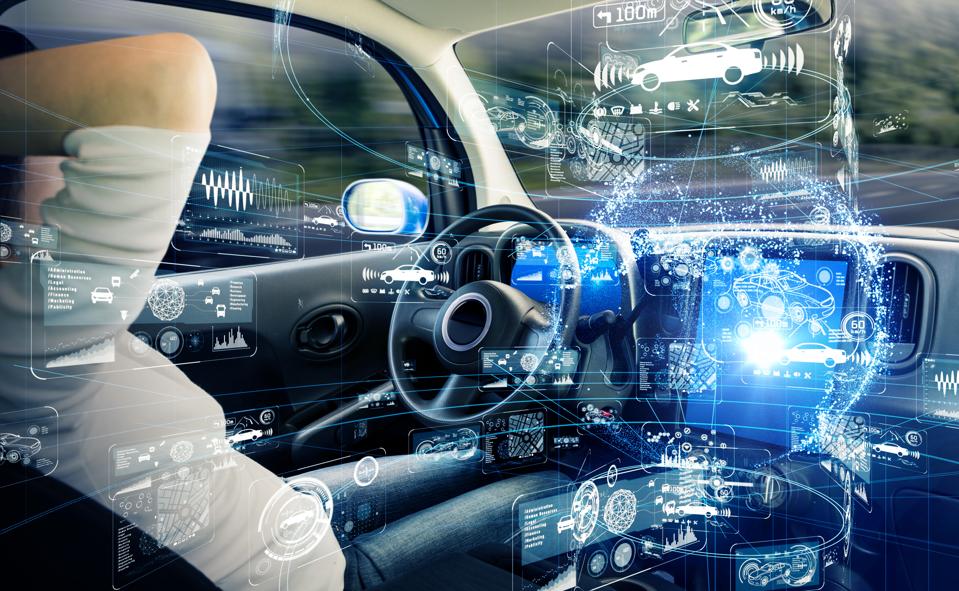
The McKinsey global survey found a nearly 25% year-over-year increase in the use of AI in standard business processes, with a sizable jump from the past year in companies using AI across multiple areas of their business; 58% of executives surveyed report that their organizations have embedded at least one AI capability into a process or product in at least one function or business unit, up from 47% in 2018; retail has seen the largest increase in AI use, with 60% of respondents saying their companies have embedded at least one AI capability in one or more functions or business units, a 35-percentage point increase from 2018; 74% of respondents whose companies have adopted or plan to adopt AI say their organizations will increase their AI investment in the next three years; 41% say their organizations comprehensively identify and prioritize their AI risks, citing most often cybersecurity and regulatory compliance. 84% of C-suite executives believe they must leverage AI to achieve their growth objectives, yet 76% report they struggle with how to scale AI;
How Europe’s AI ecosystem could catch up with China and the U.S.

Europe edges out the U.S. in total number of software developers (5.7 million to 4.4 million), and venture capital spending in Europe continues to rise to historically high levels. Even so, the U.S. and China beat Europe in venture capital spending, startup growth, and R&D spending. The U.S. also outpaces Europe in AI, big data, and quantum computing patents. A Center for Data Innovation study released last month also concluded that the U.S. is in the lead, followed by China, with Europe lagging behind. Multiple surveys of business executives have found that businesses around the world are struggling to scale the use of AI, but European firms trail major U.S. companies in this metric too, with the exception of smart robotics companies. This trend could be in part due to lower levels of data digitization, Bughin said. About 3-4% of businesses surveyed by McKinsey were found to be using AI at scale. The majority of those are digital native companies, he said, but 38% of major companies in the U.S. are digital natives compared to 24% in Europe.
Singapore government must realise human error also a security breach

More importantly, before dismissing man-made mistakes as "not a security risk", organisations such as the SAC need to consider the stats. "Inadvertent" breaches brought about by human error and system glitches accounted for 49% of data breaches, according to an IBM Security report conducted by Ponemon Institute, which estimated that human errors alone cost companies $3.5 million. In fact, cybersecurity vendor Kaspersky described employees as a major hole in an organisation's fight against cyber attacks. Some 52% viewed their staff as the biggest weakness in IT security, where their careless actions put the company's security strategy at risk. It added that 47% of businesses were concerned most about employees sharing inappropriate data via mobile devices, while careless or uninformed staff were the second-most likely cause of a serious security breach--second only to malware. Some 46% of cybersecurity incidents in the past year were attributed to careless or uninformed staff. Kaspersky further described human error on the part of staff as the "attack vector" that businesses were falling victim to.
6-essential practices to successfully implement machine learning solutions

Here’s a golden rule to remember: a machine learning algorithm is only as good as the data it’s fed. So, to use machine learning effectively, you must have the right data for the problem you’re trying to solve. And not just a few data points. Machines need a lot of data to learn — think hundreds of thousands of data points. Your data will need to be formatted, cleaned, and organized for your algorithm, and you will need two datasets: one to train the model and one to evaluate its performance. So after picking up the use cases, filter out the ones where there is data available and the ones that can quickly generate value across the board. Go for multiple smaller wins and have a clear data strategy. ... With a worldwide shortage of trained data scientists, you need to empower your data analytics professionals and other domain information experts with the tools and support they need to become citizen data scientists.
The hardest part of AI & analytics is not AI - it’s data management

“This is going to enable organisations to train their AI and ML algorithms with a more complete, more comprehensive and less biased sets of data.” According to Hanson, this can be done by using good data engineering tools with AI built-in. “What we actually need is not just artificial intelligence in the analytics layer — in terms of generating graphical views of data and making decisions in real-time around data — we need to make sure that we’ve got artificial intelligence in the backend to ensure we’ve got well-curated data going into our analytics engines.” He warned that if organisations fail to do this, they won’t see the benefit of analytical AI going forward. “In my opinion, a lot of mistakes could be made, some serious mistakes, if we don’t make sure that we train our analytical AI with high quality, well-curated data,” said Hanson. He added, if the data sets aren’t good, then AI advocates in organisations are not going to get the results they expect. This could hinder any future investment in the technology.
How to Advance Your Enterprise Risk Management Maturity

Before you can determine whether you want to advance your ERM maturity, you must first define your appetite for risk to make a proper assessment. Not all companies require the same level of risk maturity. In fact, the highest level of maturity does not necessarily equal the best ERM program. Rather than immediately aiming for the highest level of maturity, companies need to take a step back and identify their priorities to understand what is best for their organization’s specific circumstances. ... Effective risk culture is one that empowers business functions to be intellectually honest about the risks they face and encourages them to align risks with strategic objectives. To accomplish this, companies must remain patient. Changing a culture of any sized organization takes time and is not something that can be done by any single meeting or memo to the staff. It takes time to educate team members properly and for leaders to demonstrate the importance of the change. ... Once you determine who should hold primary responsibility for the risk management program and have received the necessary buy-in, you will need to measure your progress towards greater ERM maturity. One way to measure progress is to compare yourself to your peers.
Quote for the day:
"The science of today is the technology of tomorrow." -- Edward Teller
No comments:
Post a Comment