The Rise Disappearance And Retirement Of Google Co-Founders
/cdn.vox-cdn.com/uploads/chorus_image/image/65827722/528779824.jpg.0.jpg)
It’s a fitting end for two of the most mysterious tech leaders of a generation, who are both exiting their company as it hovers near $1 trillion in market cap. But it’s also a troubling time for Google. The search giant has faced increasing scrutiny from employees, media organizations, activists, regulators, and lawmakers since Page and Brin first stepped back in the summer of 2015. And many of those controversies are problems of Page and Brin’s creation, either because the duo didn’t foresee the ways in which Google could do harm or because they explicitly steered the company in a direction that flouted standard corporate ethics. In that context, it’s important to look back at the big moments in both men’s careers and how the actions they took have had an outsized impact not just on the tech industry, but on the internet and society itself. What Page and Brin have built will likely last for decades to come, and knowing how Google got to where it is today will be an important piece in the puzzle of figuring out where it goes in the future. ... Although Google is now one of the most powerful forces in online advertising on the planet, Page and Brin weren’t too keen on turning their prototype search engine into an ad-selling machine, at first.
Planning for an intelligent future
Compared to current planning activities, which invariably work on pre-defined cycles such as weekly or monthly processes, intelligent planning can be considered to have more of an ‘always-on’ approach. ... As such, any business that has access to data that exceeds the volume that humans can analyse and understand, will need intelligent planning to remain competitive. For example, a large retail organisation can harvest data from millions of daily transactions to make better buying, customer engagement, and operational decisions. But they don’t need to stop at short-term future actions; instead they should consider using social media sentiment and detailed demographics to make longer term, strategic decisions around areas such as range, store locations and customer experience. Financial services is another prime candidate for intelligent planning, particularly where understanding and influencing consumer behaviour is involved, for anything from calculating the probability of a customer renewing their insurance policy; the likelihood of a loan holder defaulting on their payments; or the future spending profile of credit card customers.
How AI is Transforming the Banking Sector
Banks are always under intense pressure from regulatory bodies to enforce the most recent regulations. These regulations are there to protect the banks and customers from fraudulent activities while at the same time, reducing financial crimes like money laundering, tax evasion, and terrorism financing. AI in banking also helps ensure that banks are compliant with the most recent regulations. AI relies on cognitive fraud analytics that watches customer behaviors, track transactions, recognize dubious activities and assess the data of different compliance systems. Businesses can remain up to date with compliance rules and regulations through the use of AI. AI systems can read compliance requirements and detect any changes in the requirements through deep learning and natural language processing. Through this, banks can remain on top of ever-evolving regulatory requirements and align their own regulations with them. Through technologies like analytics, deep learning, and machine learning, banks can remain compliant with regulations.
Augmented reality in retail: Virtual try before you buy
“Nike Fit is a transformative solution and an industry first—using a digital technology to solve for massive customer friction,” Nike writes in its press release for the launch of the app. “In the short term, Nike Fit will improve the way Nike designs, manufactures, and sells shoes—product better tailored to match consumer needs. A more accurate fit can contribute to everything from less shipping and fewer returns to better performance.” ... “The fashion industry has not traditionally been geared toward helping people understand how clothes will actually fit,” the company writes in its press release. “Gap is committed to winning customer trust by consistently presenting and delivering products that make customers look and feel great, and we are using technology to get there.” ... As the technology evolves and gives users more and more accurate renderings of how digital objects look in physical spaces, I expect that more and more brands and industries will hop onto the AR marketing bandwagon. From fashion and accessories to footwear and home décor, and beyond, AR has the potential to transform and completely reimagine customer experiences.
Why the sheer scale of DevOps testing now needs machine learning
The potential value of machine learning is particularly evident in mobile and web app testing because these are very fragmented and complex platforms to handle and understand. What ML can do in this context is to keep all those platforms visible, connected, and in a ready-state mode. In a test lab, ML helps to surface when something is outdated, disconnected from WiFi, or another problem – and moreover, help understand why that has happened. Another way in which ML helps is through showing trends and patterns, helping to not only visualise all that data but provide further insight and make sense of what has happened over the past weeks or months. For instance, it can identify the most problematic functional area in an application, such as the top 5 failing tests over the past 2-3 testing cycle, or which mobile/web platforms have been most error-prone over the past cycles. Was a failure caused by the lab, was it a pop-up, or a security alert? This really matters. Teams invest time, resources and money in automating test activities, but where all this really has an impact and add value is at the reporting stage.
The 10 most important cyberattacks of the decade
Yahoo deserves the first mention because of the sheer size of its breach and the damaging effect it had on the company's ability to compete as an email and search engine platform. In 2013, all three billion of Yahoo's accounts were compromised, making the breach the largest in the history of the internet. It took the company three years to notify the public that everyone's names, email addresses, passwords, birth dates, phone numbers and security answers had been sold on the Dark Web by hackers. Security experts say the Yahoo breach is notable because of how it was mishandled by the company and the devastating effect it had on Verizon's $4.8 billion acquisition. Yahoo initially discovered that a breach occurred in 2015 exposing 500 million accounts. ... The size of the Equifax breach pales in comparison to the value of the data exposed to hackers. As one of America's largest credit bureaus, the company had the most sensitive data on hundreds of millions of people. Hackers gained access to the information of 143 million Equifax customers, including their names, birth dates, drivers' license numbers, Social Security numbers and addresses. More than 200,000 credit card numbers were released and 182,000 documents with personally identifying information was accessed by cybercriminals.
To stop a tech apocalypse we need ethics and the arts
Matt Reaney, the chief executive and founder of Big Cloud – a recruitment company that specialises in data science, machine learning and AI employment – has argued that technology needs more people with humanities training. [The humanities] give context to the world we operate in day to day. Critical thinking skills, deeper understanding of the world around us, philosophy, ethics, communication, and creativity offer different approaches to problems posed by technology. Reaney proposes a “more blended approach” to higher education, offering degrees that combine the arts and STEM. Another advocate of the interdisciplinary approach is Joseph Aoun, President of Northeastern University in Boston. He has argued that in the age of AI, higher education should be focusing on what he calls “humanics”, equipping graduates with three key literacies: technological literacy, data literacy and human literacy. The time has come to answer the call for humanities graduates capable of crossing over into the world of technology so that our human future can be as bright as possible.
Learning algorithms and the self-supervised machine with Dr. Philip Bachman
Supervised learning is sort of what’s had the most immediate success and what’s driving a lot of the deep learning power technologies that are being used for doing things like speech recognition in phones or doing automated question answering for chat bots and stuff like that. So supervised learning refers to kind of a subset of the techniques that people apply when they have access to a large amount of data and they have a specific type of action that they want a model to perform when it processes that data. And what they do is, they get a person to go and label all the data and say, okay, well this is the input to the model at this point in time. And given this input, this is what the model should output. So you’re putting a lot of constraints on what the model is doing and constructing those constraints manually by having a person looking at a set of a million images and, for each image, they say, oh, this is a cat, this is a dog, this is a person, this is a car.
What a cloud-native approach to RPA could mean to your business
Enterprise’s affinity to cloud computing hasn’t traditionally been reflected by the RPA industry. That is, until now – with the world’s first cloud-native RPA platform, we’re bringing the advantages of cloud-native, intelligent RPA deployments to organisations worldwide. For business users, cloud-native RPA operates as a self-service technology accessed via a web-based graphical interface from anywhere. With a single click or drag-and-drop motion, users can automate those parts of any job that don’t require human creativity, problem-solving capabilities, empathy, or judgment. Just as with popular Software-as-a-Service (SaaS) apps, users can create what they need using an intuitive web interface within the browser. For many common bots, no coding is required. There are no large client downloads to install and manage or commands to memorise; automation and processes are exposed via drag-and-drop functionality and flow charts. Also, because there is no software client, IT doesn’t have to get involved. Infrastructure management costs go away, significantly reducing the total cost of ownership (TCO).
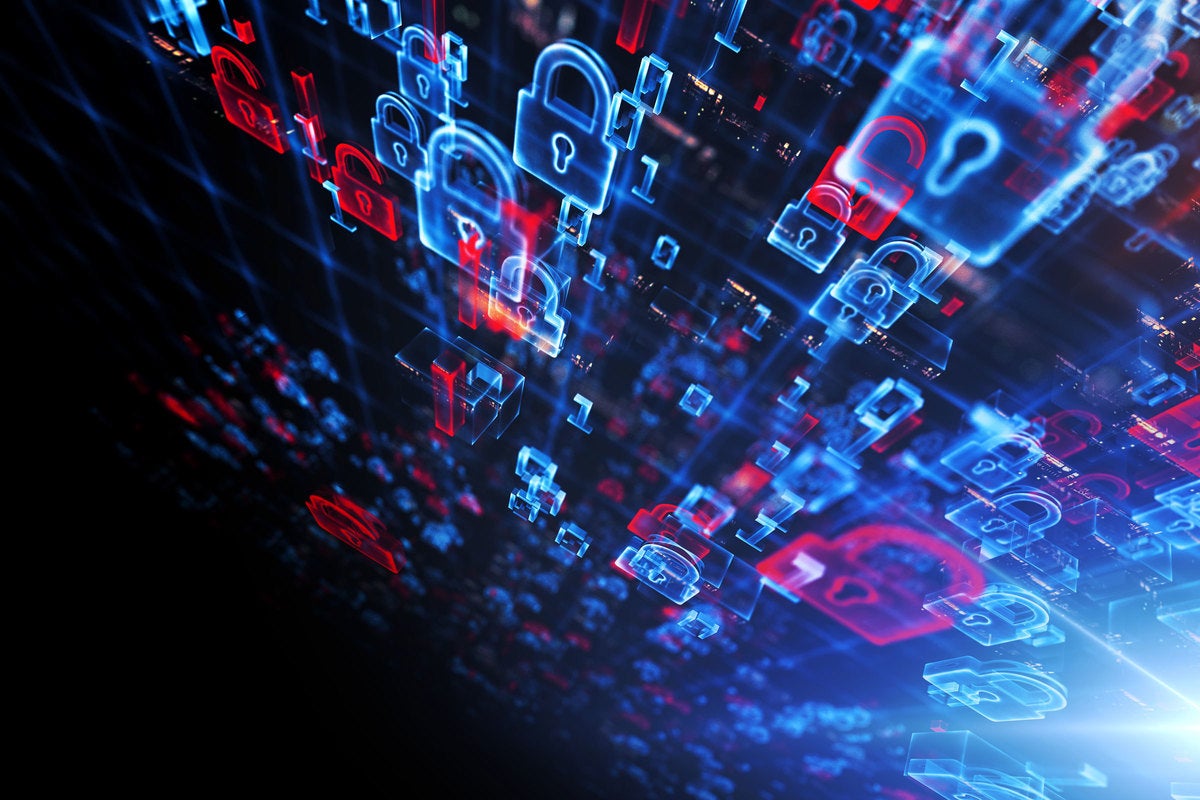
At an even more fundamental level, anyone looking at the state of enterprise security today understands that whatever we’re doing now isn’t working. “The perimeter-based model of security categorically has failed,” says Forrester principal analyst Chase Cunningham. “And not from a lack of effort or a lack of investment, but just because it’s built on a house of cards. If one thing fails, everything becomes a victim. Everyone I talk to believes that.” Cunningham has taken on the zero-trust mantle at Forrester, where analyst Jon Kindervag, now at Palo Alto Networks, developed a zero-trust security framework in 2009. The idea is simple: trust no one. Verify everyone. Enforce strict access-control and identity-management policies that restrict employee access to the resources they need to do their job and nothing more. Garrett Bekker, principal analyst at the 451 Group, says zero trust is not a product or a technology; it’s a different way of thinking about security. “People are still wrapping their heads around what it means. Customers are confused and vendors are inconsistent on what zero trust means. But I believe it has the potential to radically alter the way security is done.”
Quote for the day:
"Leadership is a journey, not a destination. It is a marathon, not a sprint. It is a process, not an outcome." -- John Donahoe
No comments:
Post a Comment