What is cyber risk quantification, and why is it important?

Put simply, the idea behind quantification is to prioritize risks according to
their potential for financial loss, thus allowing responsible people in a
company to create budgets based on mitigation strategies that afford the best
protection and return on investment. Now to the difficult part: how to
incorporate cyber risk quantification. "Risk quantification starts with the
evaluation of your organization's cybersecurity risk landscape," explained
Tattersall. "As risks are identified, they are annotated with a potential loss
amount and frequency which feeds a statistical model that considers the
probability of likelihood and the financial impact." Tattersall continued, "When
assessing cybersecurity projects, risk quantification supports the use of loss
avoidance as a proxy for return on investment. Investments in tighter controls,
assessment practices and risk management tools are ranked by potential
exposure." According to Tattersall, companies are already employing cyber risk
quantification. He offered the FAIR Institute's Factor Analysis of Information
Risk as an example. The FAIR Institute website mentions their platform provides
a model for understanding, analyzing and quantifying cyber risk and operational
risk in financial terms.
What We Know (and Don't Know) So Far About the 'Supernova' SolarWinds Attack
It's not unusual for multiple nation-state attacker groups to target the same
victim organization, nor even to reside concurrently and unbeknownst to one
another while conducting their intelligence-gathering operations. But Supernova
and the Orion supply chain attack demonstrate how nation-states also can have
similar ideas yet different methods regarding how they target and ultimately
burrow into the networks of their victims. Supernova homed in on SolarWinds'
Orion by exploiting a flaw in the software running on a victim's server;
Sunburst did so by inserting malicious code into builds for versions of the
Orion network management platform. The digitally signed builds then were
automatically sent to some 18,000 federal agencies and businesses last year via
a routine software update process, but the attackers ultimately targeted far
fewer victims than those who received the malicious software update, with fewer
than 10 federal agencies affected as well as some 40 of Microsoft's own
customers. US intelligence agencies have attributed that attack to a Russian
nation-state group, and many details of the attack remain unknown.
World Backup Day 2021: what businesses need to know post-pandemic

For many businesses, the shift to remote working that occurred worldwide last
year due to the Covid-19 outbreak brought with it an ‘always on’, omnichannel
approach to customer service. As this looks set to continue meeting the needs of
consumers, organisations must consider how they can protect their data
continuously, with every change, update or new piece of data protected and
available in real time. “Continuous data protection (CDP) is enabling this
change, saving data in intervals of seconds – rather than days or months – and
giving IT teams the granularity to quickly rewind operations to just seconds
before disruption occurred,” said Levonai. “Completely flexible, CDP enables an
IT team to quickly recover anything, from a single file or virtual machine right
up to an entire site. “As more organisations join the CDP backup revolution,
data loss may one day become as harmless as an April Fool’s joke. Until then, it
remains a real and present danger.”... Businesses should back up their data by
starting in reverse. Effective backup really starts with the recovery
requirements and aligning to the business needs for continued service.
DevOps is Not Enough for Scaling and Evolving Tech-Driven Organizations
DevOps has been an evolution of breaking silos between Development and
Operations to enable technical teams to be more effective in their work.
However, in most organizations we still have other silos, namely: Business
(Product) and IT (Tech). "BizDevOps" can be seen as an evolution from DevOps,
where the two classical big silos in organizations are broken into having
teams with the product and tech disciplines needed to build a product. This
evolution is happening in many organizations, most of the times these are
called "Product Teams". Is it enough to maximize impact as an organization? I
don't think so, and that is the focus of my DevOps Lisbon Meetup talk and
ideas around sociotechnical architecture and systems thinking I have been
exploring. In a nutshell: we need empowered product teams, but teams must be
properly aligned with value streams, which in turn must be aligned to maximize
the value exchange with the customer. To accomplish this, we need to have a
more holistic view and co-design of the organization structures and technical
architecture.
This CEO believes it’s time to embrace idealogical diversity and AI can help
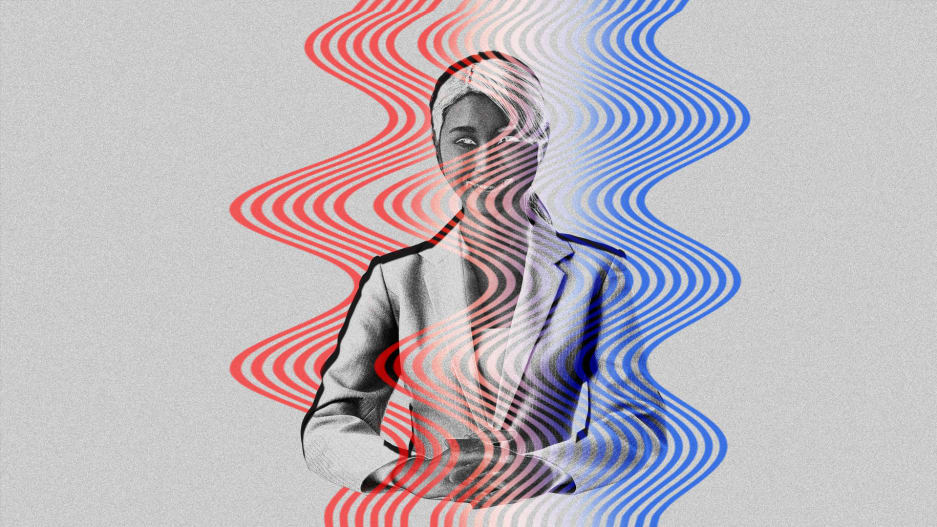
It’s important to remember that each decision from a recruiter or hiring
manager contributes to a vast dataset. AI utilizes these actions and learns
the context of companies’ hiring practices. This nature makes it susceptible
to bias when used improperly, so it is extremely critical to deploy AI models
that are designed to minimize any adverse impact. Organizations can make sure
humans are in the loop and providing feedback, steering AI to learn based on
skill preferences and hiring requirements. With the ongoing curation of
objective data, AI can help companies achieve recruiting efficiency while
still driving talent diversity. One way hiring managers can distance
themselves from political bias is by relying on AI to “score” candidates based
on factors such as proficiency and experience, rather than data like where
they live or where they attended college. In the future, AI might also be able
to mask details such as name and gender to further reduce the risk of bias.
With AI, team leaders receive an objective second opinion on hiring decisions
by either confirming their favored candidate or compelling them to question
whether their choice is the right one.
Why AI can’t solve unknown problems

Throughout the history of artificial intelligence, scientists have regularly
invented new ways to leverage advances in computers to solve problems in
ingenious ways. The earlier decades of AI focused on symbolic systems. This
branch of AI assumes human thinking is based on the manipulation of symbols,
and any system that can compute symbols is intelligent. Symbolic AI requires
human developers to meticulously specify the rules, facts, and structures that
define the behavior of a computer program. Symbolic systems can perform
remarkable feats, such as memorizing information, computing complex
mathematical formulas at ultra-fast speeds, and emulating expert
decision-making. Popular programming languages and most applications we use
every day have their roots in the work that has been done on symbolic AI. But
symbolic AI can only solve problems for which we can provide well-formed,
step-by-step solutions. The problem is that most tasks humans and animals
perform can’t be represented in clear-cut rules.
The ‘why’ of digital transformation is the key to unlocking value

Ill-prepared digital transformation projects have ripple effects. One
digitalization effort that fails to produce value doesn’t just exist in a
vacuum. If a technical upgrade, cloud migration, or ERP merge results in a
system that looks the same as before, with processes that aren’t delivering
anything new, then the decision makers will see that lack of ROI and lose
interest in any further digitalization because they believe the value just
isn’t there. Imagine an IT team leader saying they want fancy new dashboards
and new digital boardroom features. But a digital transformation project that
ends with just implementing new dashboards doesn’t change the underlying facts
about what kind of data may be read on those dashboards. And if your fancy
dashboards start displaying incorrect data or gaps in data sets, you haven’t
just undermined the efficacy and “cool factor” of those dashboards; you’ve
also made it that much harder to salvage the credibility of the project and
advocate for any new digitalization in the future. What’s the value in new
dashboards if you haven’t fixed the data problems underneath?
New Security Signals study shows firmware attacks on the rise
Microsoft has created a new class of devices specifically designed to
eliminate threats aimed at firmware called Secured-core PCs. This was recently
extended to Server and IOT announced at this year’s Microsoft Ignite
conference. With Zero Trust built in from the ground up, this means SDMs will
be able to invest more of their resources in strategies and technologies that
will prevent attacks in the future rather than constantly defending against
the onslaught of attacks aimed at them today. The SDMs in the study who
reported they have invested in secured-core PCs showed a higher level of
satisfaction with their security and enhanced confidentiality, availability,
and integrity of data as opposed to those not using them. Based on analysis
from Microsoft threat intelligence data, secured-core PCs provide more than
twice the protection from infection than non-secured-core PCs. Sixty percent
of surveyed organizations who invested in secured-core PCs reported supply
chain visibility and monitoring as a top concern.
7 Traits of Incredibly Efficient Data Scientists
Believe it or not, not every data analysis requires machine learning and
artificial intelligence. The most efficient way to solve a problem is to use
the simplest tool possible. Sometimes, a simple Excel spreadsheet can yield
the same result as a big fancy algorithm using deep learning. By choosing the
right algorithms and tools from the start, a data science project becomes much
more efficient. While it’s cool to impress everyone with a super complex tool,
it doesn’t make sense in the long run when less time could be spent using a
more simple, efficient solution. ... Doing the job right the first time is the
most efficient way to complete any project. When it comes to data science,
that means writing code using a strict structure that makes it easy to go back
and review, debug, change, and even make your code production-ready. Clear
syntax guidelines make it possible for everyone to understand everyone else’s
code. However, syntax guidelines aren’t just there so you can understand
someone else’s chicken scratch — they’re also there so you can focus on
writing the cleanest, most efficient code possible.
How insurers can act on the opportunity of digital ecosystems
First, insurers must embrace the shift to service dominant strategies and
gradually establish a culture of openness and collaboration, which will be
necessary for the dynamic empowerment of all players involved. Second,
insurers must bring to the platform the existing organizational capabilities
required for customer-centric value propositions. This means establishing
experts in the respective ecosystems—for example, in mobility, health, home,
finance, or well-being—and building the technological foundations necessary to
integrate partners into terms-of-service catalogs and APIs, as well as to
create seamless customer journeys. Finally, insurers must engage customers and
other external actors by integrating resources and engaging in service
exchange for mutual value generation. My wife, for example, has just signed up
for a telematics policy with an insurance company that offers not only
incentives for driving behavior but also value-added services, including car
sales and services. She now regularly checks whether her driving style reaches
the maximum level possible.
Quote for the day:
"When we lead from the heart, we don't
need to work on being authentic we just are!" --
Gordon Tredgold
No comments:
Post a Comment