AI projects yield little business value so far
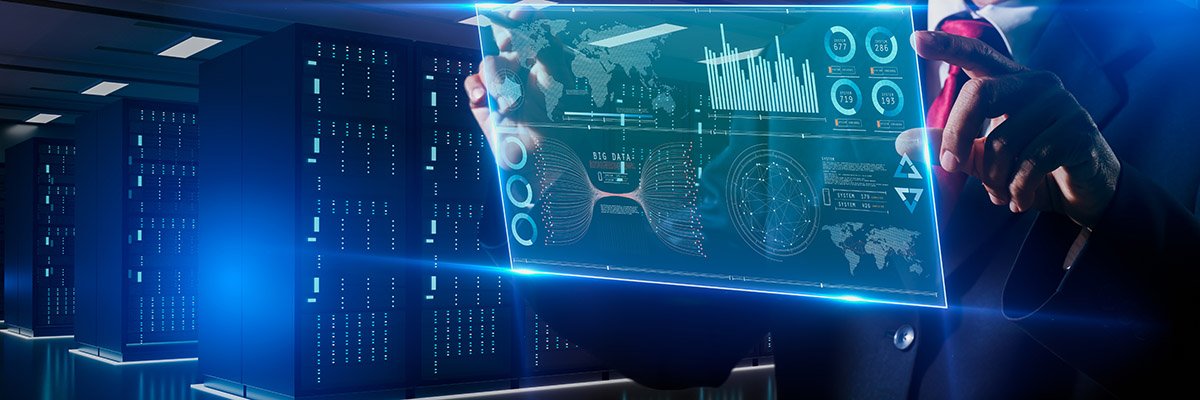
David Semach, a partner and head of AI and automation at Infosys Consulting for
Europe, the Middle East and Africa (EMEA), agrees with the researchers that
satisfaction with the technology in a financial sense is often quite low, partly
because organisations “are mostly still experimenting” with it. This means it
tends to be deployed in pockets rather than widely across the business. “The
investment required in AI is significant, but if it’s just done in silos, you
don’t gain economies of scale, you can’t take advantage of synergies and you
don’t realise the cost benefits, which means it becomes a cost-prohibitive
business model in many instances,” says Semach. Another key issue here is the
fact that most companies “mistakenly” concentrate on using the software to boost
the efficiency of internal processes and operating procedures, rather than for
generating new revenue streams. “Where companies struggle is if they focus on
process efficiencies and the bottom line because of the level of investment
required,” says Semach. “But those that focus on leveraging AI to create new
business and top-line growth are starting to see longer-term benefits.”
How Optimizing MLOps can Revolutionize Enterprise AI
With database-deployment, it only takes one line of code to deploy a model. The
database-deployment system automatically generates a table and trigger that
embody the model execution environment. No more messing with containers. All a
data scientist has to do is enter records of features into the system-generated
predictions database table to do inference on these features. The system will
automatically execute a trigger that runs the model on the new records. This
saves time for future retraining too, since the prediction table holds all the
new examples to add to the training set. This enables predictions to stay
continuously up-to-date, easily--with little to no manual code. ... The other
major bottleneck in the ML pipeline happens during the data transformation
process: manually transforming data into features and serving those features to
the ML model is time-intensive and monotonous work. A Feature Store is a
shareable repository of features made to automate the input, tracking, and
governance of data into machine learning models. Feature stores compute and
store features, enabling them to be registered, discovered, used, and shared
across a company.
How to get started in quantum computing
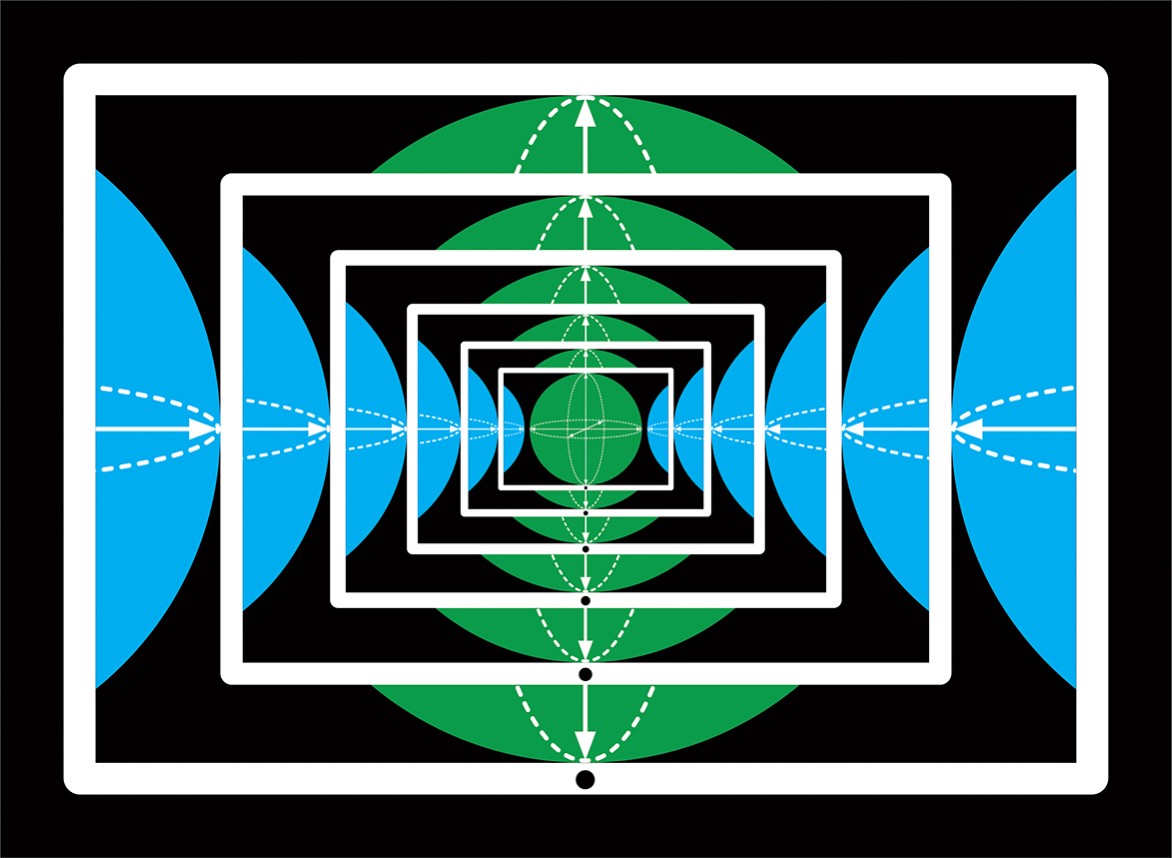
Unlike binary bits, qubits can exist as a ‘superposition’ of both 1 and 0,
resolving one way or the other only when measured. Quantum computing also
exploits properties such as entanglement, in which changing the state of one
qubit also changes the state of another, even at a distance. Those properties
empower quantum computers to solve certain classes of problem more quickly
than classical computers. Chemists could, for instance, use quantum computers
to speed up the identification of new catalysts through modelling. Yet that
prospect remains a distant one. Even the fastest quantum computers today have
no more than 100 qubits, and are plagued by random errors. In 2019, Google
demonstrated that its 54-qubit quantum computer could solve in minutes a
problem that would take a classical machine 10,000 years. But this ‘quantum
advantage’ applied only to an extremely narrow situation. Peter Selinger, a
mathematician and quantum-computing specialist at Dalhousie University in
Halifax, Canada, estimates that computers will need several thousand qubits
before they can usefully model chemical systems.
Non-Invasive Data Governance Q&A
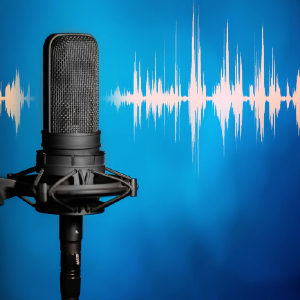
Data Governance can be non-invasive if people are recognized into the role of
data steward based on their existing relationship to the data. People define,
produce and use data as part of their everyday jobs. People automatically are
stewards if they are held formally accountable for how they define, produce
and use data. The main premise of being non-invasive is that the organization
is already governing data (in some form) and the issue is that they are doing
it informally, leading to inefficiency and ineffectiveness in how the data is
being governed. For example, people who use sensitive data are already
expected to protect that data. The NIDG approach assure that these people know
how data is classified, and that people follow the appropriate handling
procedures for the entire data lifecycle. You are already governing but you
can do it a lot better. We are not going to overwhelm you with new
responsibilities that you should already have. ... The easiest answer to
that question is that almost everybody looks at governance like they look at
government. People think that data governance has to be difficult, complex and
bureaucratic, when the truth is that it does NOT have to be that way. People
are already governing and being governed within organizations, but it is being
done informally.
Apache Kafka in a Smart City Architecture
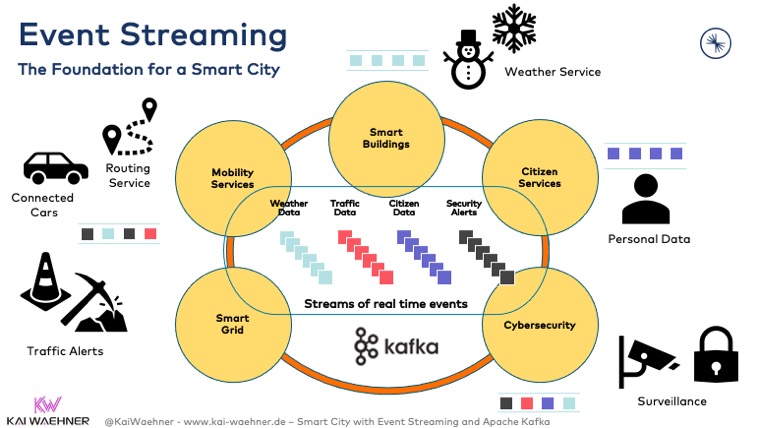
A smart city functions alongside various interfaces, data structures, and
technologies. Many high-volume data streams must be integrated, correlated,
and processed in real-time. Scalability and elastic infrastructure are
essential for success. Many data streams contain mission-critical workloads
and must offer reliability, zero data loss, and persistence. An event
streaming platform based on the Apache Kafka ecosystem provides all these
capabilities. ... A smart city requires more than real-time data integration
and real-time messaging. Many use cases are only possible if the data is also
processed continuously in real-time. That's where Kafka-native stream
processing frameworks such as Kafka Streams and ksqlDB come into play. ... The
public sector and smart city architectures leverage event streaming for
various use cases. The reasons are the same as in all other industries: Kafka
provides an open, scalable, elastic infrastructure. Additionally, it is
battle-tested and runs in every infrastructure (edge, data center, cloud, bare
metal, containers, Kubernetes, fully-managed SaaS such as Confluent Cloud).
But event streaming is not the silver bullet for every problem. Therefore,
Kafka complements other technologies such as MQTT for edge integration, or a
cloud data lake for batch analytics.
Remote work: 4 ways to spot a toxic culture

Trust is the fundamental element of a high-performing culture. Especially in a
remote workplace, it’s difficult to be a lone wolf and not collaborate on
projects. If you notice that your team is avoiding working with someone, look to
see if it’s a pattern. Perhaps that individual is “phoning it in” or making too
many mistakes, and the team can’t trust their work anymore. You need to address
this right away to avoid disappointing the rest of the team. Ask yourself: How
much do folks enjoy redoing someone else’s work? Or watching the employee screw
up and get away with it? Or questioning why they are working so hard while
others aren’t? Worse, your team members may start wondering if they can trust
you as a manager if you won’t handle the problem. ... When you hear someone make
a statement that may be judgmental, ask the person, “What do you know to be
true? What are the facts?” A good way to tell if someone is stating facts or
judgments is to apply the “videotape test:” Can what they describe be captured
by a video camera? For example, “He was late for the meeting” is a fact and
passes the test. In contrast, “He’s lazy” is a judgment and doesn’t pass the
test. Be mindful when you’re hearing judgments and try to dig out the facts.
Changing the AI Landscape Through Professionalization

Organizations should work with Data Architect, Business owners and Solution
architect to develop their AI strategy underpinned by Data strategy, Data
Taxonomy and analyzing the value that their company can and wish to create. For
“Establishing a Data Driven culture is the key—and often the biggest
challenge—to scaling artificial intelligence across your organization.” While
your technology enables business, your workforce is the essential driving force.
It is crucial to democratize data and AI literacy by encouraging skilling,
upskilling, and reskilling. Resources in the organization would need to change
their mindset from experience-based, leadership driven decision making to
data-driven decision making, where employees augment their intuition and
judgement with AI algorithms’ recommendations to arrive at best answers than
either humans or machines could reach on their own. My recommendation would be
to carve out “System of Knowledge & Learning” as a separate stream in
overall Enterprise Architecture, along with System of Records, Systems of
Engagement & Experiences, Systems of Innovation & Insight. AI and data
literacy will help in increasing employee satisfaction because the organization
is allowing its workforce to identify new areas for professional development.
How Skyworks embraced self-service IT
At Skyworks, the democratization of IT is all about giving our business users
access to technology—application development, analytics, and automation—with the
IT organization providing oversight, but not delivery. IT provides oversight in
the form of security standards and release and change management strategies,
which gives our business users both the freedom to improve their own
productivity and the assurance that they are not reinventing the automation
wheel across multiple sites. COVID has been a real catalyst of this new
operating model. As in most companies, when COVID hit, we started to see a
flurry of requests for new automation and better analytics in supply chain and
demand management. Luckily for us, we had already started to put the foundation
in place for our data organization, so we were able capitalize on this
opportunity to move into self-service. ... For IT to shift from being
order-takers to enablers of a self-service culture, we created a new role: the
IT business partner. We have an IT business partner for every function; these
people participate in all of the meetings of their dedicated function, and
rather than asking “What new tool do you need?”, they ask, “What is the problem
you are trying to solve?” IT used to sit at the execution table; with our new IT
business partners, we now sit at the ideation table.
12 Service Level Agreement (SLA) best practices for IT leaders

Smart IT leaders understand that negotiation is not concession. It’s critical to
reach a mutually agreed pathway to providing the service that the client
expects, says Vamsi Alla, CTO at Think Power Solutions. In particular, IT
leaders should work with providers on penalties and opt-out clauses. “A good SLA
has provisions for mutually agreed-upon financial and non-financial penalties
when service agreements are not met,” Alla says. Without that, an SLA is worth
little more than the paper on which it’s written. ... “The most common mistake
is to include performance metrics that are properly reviewed and unattainable,”
Alla says. “This is usually done because the client has asked for it and the
service provider is too willing to oblige. This may cause the contract to come
through, but the road to execution becomes bumpy as the metrics can’t be
achieved.” The level of service requested directly impacts the price of the
service. “It’s important to understand what a reasonable level of performance is
in the market so as not to over-spec expectations,” Fong of Everest Group says.
Security starts with architecture
Security must be viewed as an organizational value that exists in all aspects
of its operation and in every part of the product development life cycle. That
includes planning, design, development, testing and quality assurance, build
management, release cycles, the delivery and deployment process, and ongoing
maintenance. The new approach has to be both strategic and tactical. In
strategic terms, every potential area of vulnerability has to be conceptually
addressed through holistic architectural design. During the design process,
tactical measures have to be implemented in each layer of the technology
ecosystem (applications, data, infrastructure, data transfer, and information
exchange). Ultimately, the responsibility will fall in the hands of the
development and DevOps teams to build secure systems and fix security
problems. The strategic and tactical approach outlined will allow them to
handle security successfully. Security policies must be applied into the
product development life cycle right where code is being written, data systems
are being developed, and infrastructure is being set up.
Quote for the day:
"If you really want the key to
success, start by doing the opposite of what everyone else is doing." --
Brad Szollose
No comments:
Post a Comment