‘Black Mirror’ or better? The role of AI in the future of learning and development

An AI-assisted learning-development tool can search a variety of sources
internally or externally to find content that is relevant to a particular
learning or performance outcome. Digital marketers and online publishers have
been using AI to generate content for simple stories for years now. Odds are you
have read an online article or blog post created by a bot and didn’t even
realize it. In the learning space, there are tools such as Emplay and IBM’s
Watson that can support this. For example, let’s say a designer wants to create
a quick microlearning on how a vacuum pump works. The designer could engage an
AI bot to crawl internal or external networks for potential resources —
including videos and images. The AI agent then analyzes them, aligning pieces to
specific learning outcomes, prioritizing resources for relevance and tagging
them by modality. Ultimately, this would free up the designer to focus more on
learner-centric design and delivery. ... As you can see, there are many
potential benefits to the adoption of AI in the learning space. However, before
we invest in AI, it is important to first explore the risks and practical issues
of adopting AI across the enterprise.
Facebook is making a bracelet that lets you control computers with your brain

The wristband, which looks like a clunky iPod on a strap, uses sensors to detect
movements you intend to make. It uses electromyography (EMG) to interpret
electrical activity from motor nerves as they send information from the brain to
the hand. The company says the device, as yet unnamed, would let you navigate
augmented-reality menus by just thinking about moving your finger to scroll. A
quick refresher on augmented reality: It overlays information on your view of
the real world, whether it’s data, maps, or other images. The most successful
experiment in augmented reality was Pokémon Go, which took the world by storm in
2016 as players crisscrossed neighborhoods in search of elusive Pokémon
characters. That initial promise has faded over the intervening years, however,
as companies have struggled to translate the technology into something
appealing, light, and usable. Google Glass and Snap Spectacles bombed, for
example: people simply did not want to use them. Facebook thinks its wristband
is more user friendly. Too soon to tell. The product is still in research and
development at the company’s internal Facebook Reality Labs, and I didn’t get to
have a go.
Uncertainty And Innovation At Speed
As uncertainty continues to rise and the unexpected becomes more common,
organizations may not always have the luxury to conduct extensive analysis
before acting. Indeed, high uncertainty and rapid change tend to reduce the
relevance of the data that companies may have traditionally used for planning.
They may need to place bets on multiple possible futures. Above all, they will
need capacity for rapid innovation—every day, not just in a crisis. Companies
executed the rapid innovations described above by repurposing existing
knowledge, resources and technology. A recent article suggests that
organizations in all industries may be able to use repurposing to achieve
ultrafast innovation to develop new solutions to our current and future
challenges. Some innovation thinkers take inspiration from venture capital.
Venture capital firms tend to tie funding to the achievement of milestones that
reduce investment risk, such as proving technical feasibility or product-market
fit. This approach instills a sense of urgency in startup companies: Their very
survival may depend on achieving a funding milestone. A crisis such as the
COVID-19 pandemic can produce a sense of urgency in even large organizations.
But banking on effective innovation in response to a crisis is not a robust
strategy.
What is data governance? A best practices framework for managing data assets
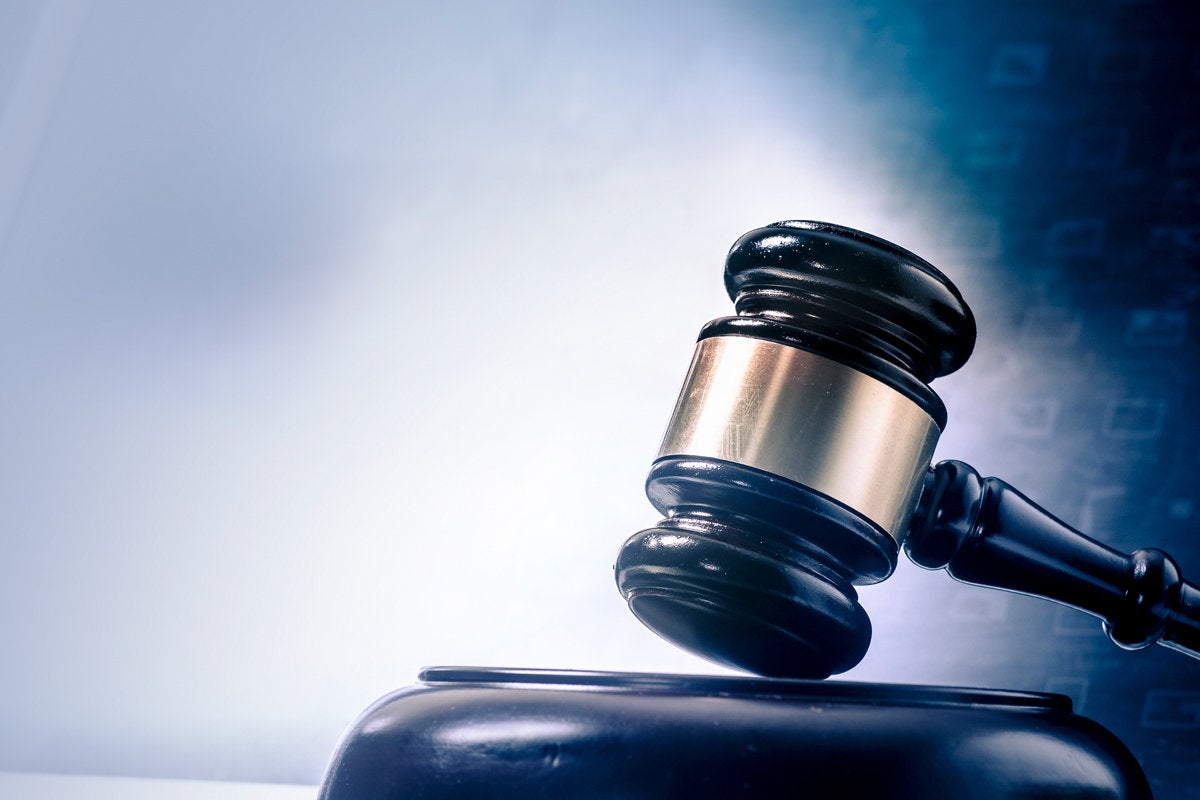
When establishing a strategy, each of the above facets of data collection,
management, archiving, and use should be considered. The Business Application
Research Center (BARC) warns it is not a “big bang initiative.” As a highly
complex, ongoing program, data governance runs the risk of participants losing
trust and interest over time. To counter that, BARC recommends starting with a
manageable or application-specific prototype project and then expanding across
the company based on lessons learned. ... Most companies already have some form
of governance for individual applications, business units, or functions, even if
the processes and responsibilities are informal. As a practice, it is about
establishing systematic, formal control over these processes and
responsibilities. Doing so can help companies remain responsive, especially as
they grow to a size in which it is no longer efficient for individuals to
perform cross-functional tasks. ... Governance programs span the enterprise,
generally starting with a steering committee comprising senior management, often
C-level individuals or vice presidents accountable for lines of
business.
How Google's balloons surprised their creator
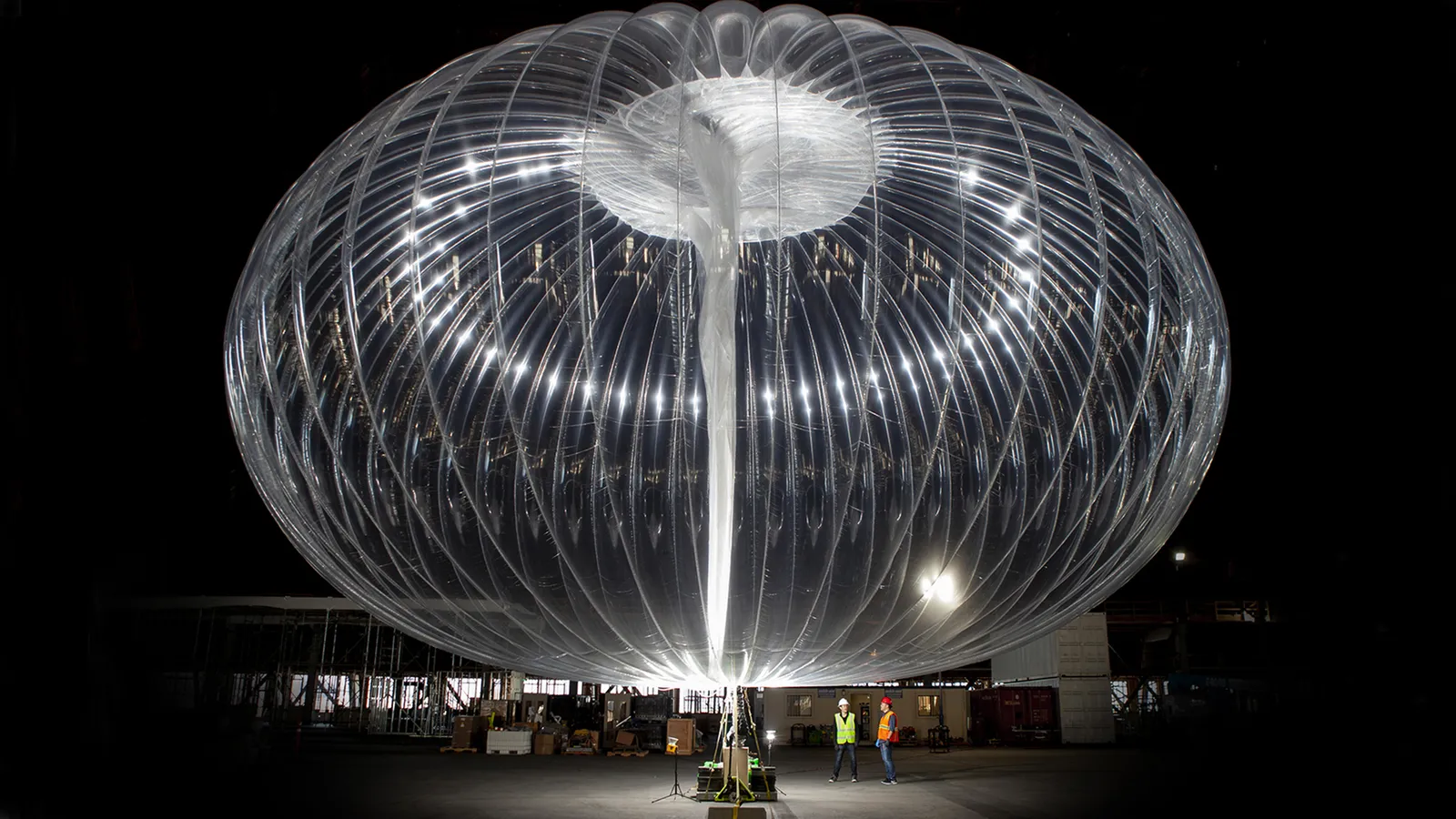
In the AI community, there's one example of AI creativity that seems to get
cited more than any other. The moment that really got people excited about what
AI can do, says Mark Riedl at the Georgia Institute of Technology, is when
DeepMind showed how a machine learning system had mastered the ancient game Go –
and then beat one of the world's best human players at it. "It ended up
demonstrating that there were new strategies or tactics for countering a player
that no one had really ever used before – or at least a lot of people did not
know about," explains Riedl. And yet even this, an innocent game of Go, provokes
different feelings among people. On the one hand, DeepMind has proudly described
the ways in which its system, AlphaGo, was able to "innovate" and reveal new
approaches to a game that humans have been playing for millennia. On the other
hand, some questioned whether such an inventive AI could one day pose a serious
risk to humans. "It's farcical to think that we will be able to predict or
manage the worst-case behaviour of AIs when we can't actually imagine their
probable behaviour," wrote Jonathan Tapson at Western Sydney University after
AlphaGo's historic victory.
AI Can Help Companies Tap New Sources of Data for Analytics

Just as Google applications can tell you, on the basis of your home address,
calendar entries, and map information, that it’s time to leave for the airport
if you want to catch your flight, companies can increasingly take advantage of
contextual information in their enterprise systems. Automation in analytics —
often called “smart data discovery” or “augmented analytics” — is reducing the
reliance on human expertise and judgment by automatically pointing out
relationships and patterns in data. In some cases the systems even recommend
what the user should do to address the situation identified in the automated
analysis. Together these capabilities can transform how we analyze and consume
data. Historically, data and analytics have been separate resources that needed
to be combined to achieve value. If you wanted to analyze financial or HR or
supply chain data, for example, you had to find the data — in a data warehouse,
mart, or lake — and point your analytics tool to it. This required extensive
knowledge of what data was appropriate for your analysis and where it could be
found, and many analysts lacked knowledge of the broader context. However,
analytics and even AI applications can increasingly provide context.
5 Reasons to Make Machine Learning Work for Your Business
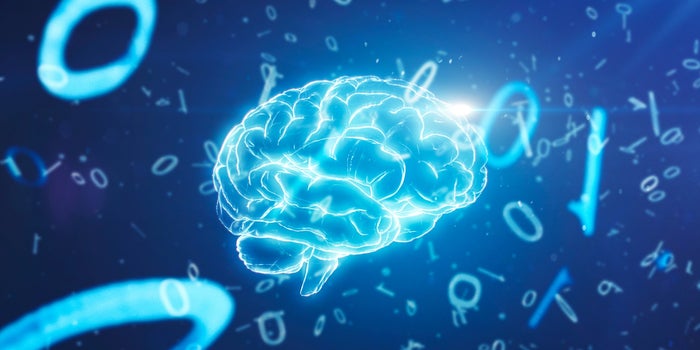
The original promise of machine learning was efficiency. Even as its uses have
expanded beyond mere automation, this remains a core function and one of the
most commercially viable use cases. Using machine learning to automate routine
tasks, save time and manage resources more effectively has a very attractive
paid of side effects for enterprises that do it effectively: reducing expenses
and boosting net income. The list of tasks that machine learning can automate is
long. As with data processing, how you use machine learning for process
automation will depend on which functions exert the greatest drag on your time
and resources. ... Machine learning has also proven its worth in detecting
trends in large data sets. These trends are often too subtle for humans to tease
out, or perhaps the data sets are simply too large for “dumb” programs to
process effectively. Whatever the reason for machine learning’s success in this
space, the potential benefits are clear as day. For example, many small and
midsize enterprises use machine learning technology to predict and reduce
customer churn, looking for signs that customers are considering competitors and
trigger retention processes with higher probabilities of success.
Accelerating data and analytics transformations in the public sector
Too often, the lure of exciting new technologies influences use-case
selection—an approach that risks putting scarce resources against low-priority
problems or projects losing momentum and funding when the initial buzz wears
off, the people who backed the choice move on, and newer technologies emerge.
Organizations can find themselves in a hype cycle, always chasing something new
but never achieving impact. To avoid this trap, use cases should be anchored to
the organization’s (now clear) strategic aspiration, prioritized, then sequenced
in a road map that allows for deployment while building capabilities. There are
four steps to this approach. First, identify the relevant activities and
processes for delivering the organization’s mission—be that testing,
contracting, and vendor management for procurement, or submission management,
data analysis, and facilities inspection for a regulator—then identify the
relevant data domains that support them.... Use cases should be framed as
questions to be addressed, not tools to be built. Hence, a government agency
aspiring to improve the uptime of a key piece of machinery by 20 percent while
reducing costs by 5 percent might first ask, “How can we mitigate the risk of
parts failure?” and not set out to build an AI model for predictive
maintenance.
Quantum computing breaking into real-world biz, but not yet into cryptography
A Deloitte Consulting report echoed Baratz's views, stating that quantum
computers would not be breaking cryptography or run at computational speeds
sufficient to do so anytime soon. However, it said quantum systems could pose a
real threat in the long term and it was critical that preparations were carried
out now to plan for such a future. On its impact on Bitcoin and blockchain, for
instance, the consulting firm estimated that 25% of Bitcoins in circulation were
vulnerable to a quantum attack, pointing in particular to the cryptocurrency
that currently were stored in P2PK (Pay to Public Key) and reused P2PKH (Pay to
Public Key Hash) addresses. These potentially were at risk of attacks as their
public keys could be directly obtained from the address or were made public when
the Bitcoins were used. Deloitte suggested a way to plug such gaps was
post-quantum cryptography, though, these algorithms could pose other challenges
to the usability of blockchains. Adding that this new form of cryptography
currently was assessed by experts, it said: "We anticipate that future research
into post-quantum cryptography will eventually bring the necessary change to
build robust and future-proof blockchain applications."
What Is Open RAN (Radio Access Network)?

Open radio access network (RAN) is a term for industry-wide standards for RAN
interfaces that support interoperation between vendors’ equipment. The main goal
for using open RAN is to have an interoperability standard for RAN elements such
as non-proprietary white box hardware and software from different vendors.
Network operators that opt for RAN elements with standard interfaces can avoid
being stuck with one vendor’s proprietary hardware and software. Open RAN is not
inherently open source. The Open RAN standards instead aim to undo the siloed
nature of the RAN market, where a handful of RAN vendors only offer equipment
and software that is totally proprietary. The open RAN standards being developed
use virtual RAN (vRAN) principles and technologies because vRAN brings features
such as network malleability, improved security, and reduced capex and opex
costs. ... An open RAN ecosystem gives network operators more choice in RAN
elements. With a multi-vendor catalog of technologies, network operators have
the flexibility to tailor the functionality of their RANs to the operators’
needs. Total vendor lock-in is no longer an issue when organizations are able to
go outside of one RAN vendor’s equipment and software stack.
Quote for the day:
"Challenges in life always seek leaders
and leaders seek challenges." -- Wayde Goodall
No comments:
Post a Comment