Quote for the day:
"Strategy is not really a solo sport _ even if you_re the CEO." -- Max McKeown
MCP: The new “USB-C for AI” that’s bringing fierce rivals together
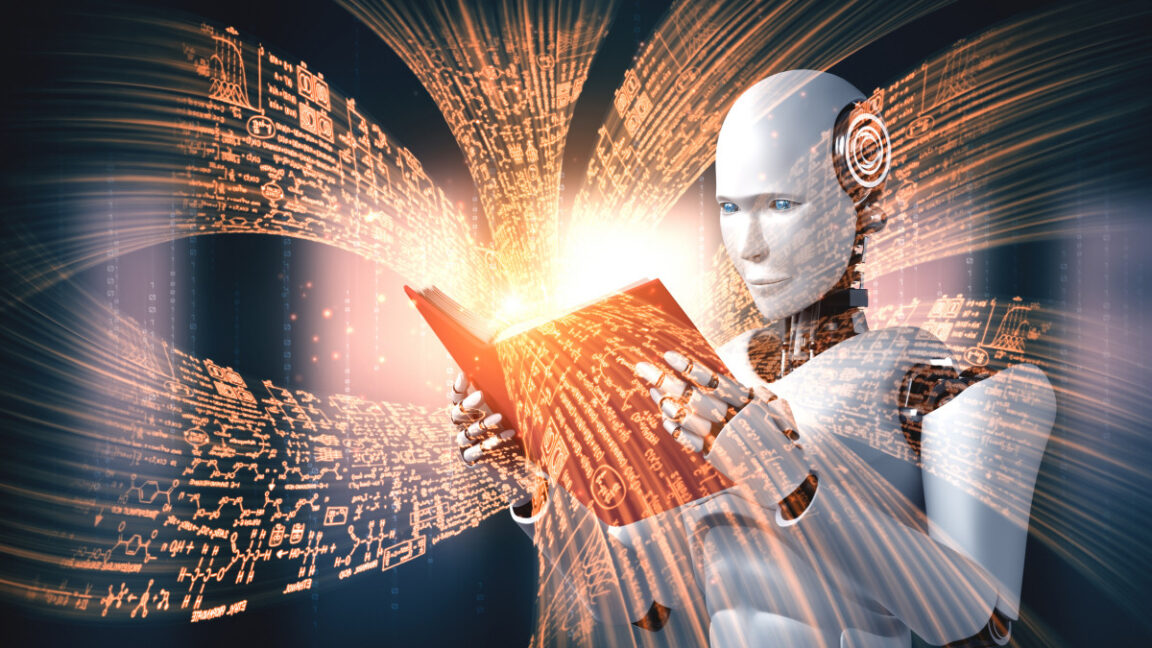
So far, MCP has also garnered interest from multiple tech companies in a rare
show of cross-platform collaboration. For example, Microsoft has integrated MCP
into its Azure OpenAI service, and as we mentioned above, Anthropic competitor
OpenAI is on board. Last week, OpenAI acknowledged MCP in its Agents API
documentation, with vocal support from the boss upstairs. "People love MCP and
we are excited to add support across our products," wrote OpenAI CEO Sam Altman
on X last Wednesday. ... To make the connections behind the scenes between AI
models and data sources, MCP uses a client-server model. An AI model (or its
host application) acts as an MCP client that connects to one or more MCP
servers. Each server provides access to a specific resource or capability, such
as a database, search engine, or file system. When the AI needs information
beyond its training data, it sends a request to the appropriate server, which
performs the action and returns the result. To illustrate how the client-server
model works in practice, consider a customer support chatbot using MCP that
could check shipping details in real time from a company database. "What's the
status of order #12345?" would trigger the AI to query an order database MCP
server, which would look up the information and pass it back to the model.
Why global tensions are a cybersecurity problem for every business
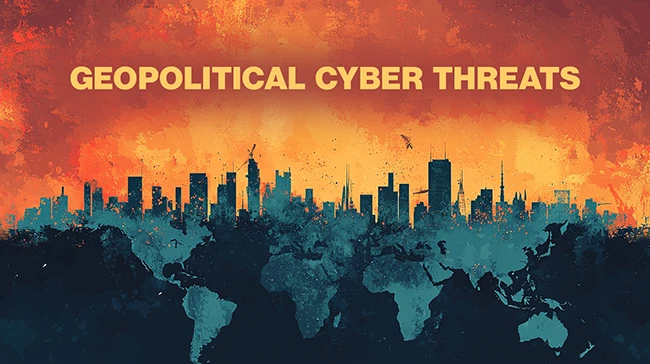
As global polarization intensifies, cybersecurity threats have become
increasingly hybridized, complicating the landscape for threat attribution and
defense. Michael DeBolt, Chief Intelligence Officer at Intel 471, explains:
“Increasing polarization worldwide has seen the expansion of the state-backed
threat actor role, with many established groups taking on financially motivated
responsibilities alongside their other strategic goals.” This evolution is
notably visible in threat actors tied to countries such as China, Iran, and
North Korea. According to DeBolt, “Heightened geopolitical tensions have
reflected this transition in groups originating from China, Iran, and North
Korea over the last couple of years—although the latter is somewhat more
well-known for its duplicitous activity that often blurs the line of more
traditional e-crime threats.” These state-backed groups increasingly blend
espionage and destructive attacks with financially motivated cybercrime
techniques, complicating attribution and creating significant practical
challenges for organizations. DeBolt highlights the implications: “A primary
practical issue organizations are facing is threat attribution, with a follow-on
issue being maintaining an effective security posture against these hybrid
threats.”
How to take your first steps in AI without falling off a cliff
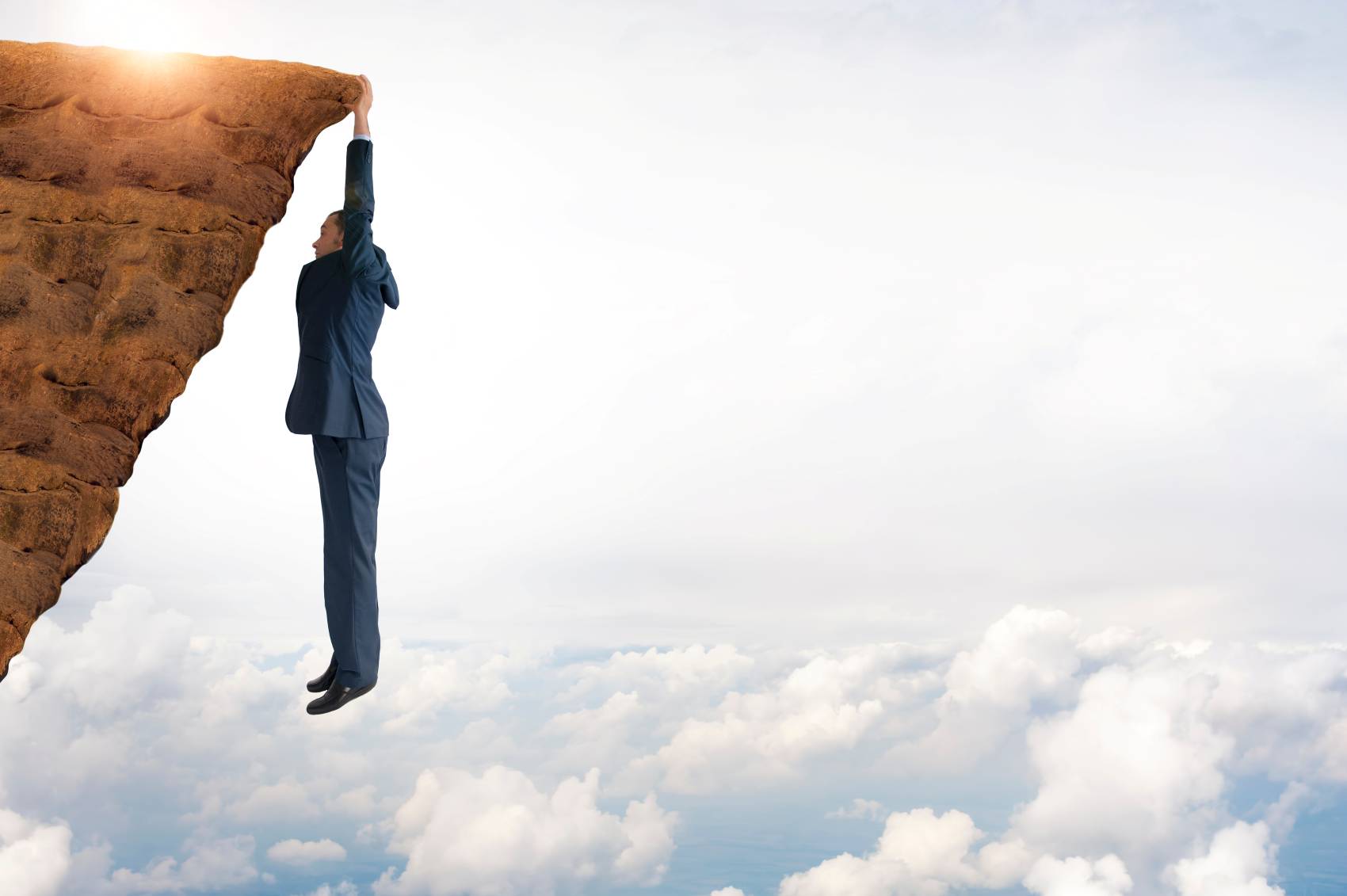
It is critical to bring all stakeholders on board through education and training
on the fundamental building blocks of data and AI. This involves understanding
what’s accessible in the market and differentiating between various AI
technologies. Executive buy-in is crucial, and by planning for internal process
outcomes first, organisations can better position themselves to achieve
meaningful outcomes in the future. ... Don’t bite off more than you can chew!
Trying to deploy a complex AI solution to the entire organisation is asking for
trouble. It is better to identify early adopter departments where specific AI
pilots and proofs of concept can be introduced and their value measured.
Eventually, you might establish an AI assistant studio to develop dedicated AI
tools for each use case according to individual needs. ... People are often wary
of change, particularly change with such far reaching implications in terms of
how we work. Clear communication, training, and ongoing support will all help
reassure employees who fear being left behind. ... In the context of data and
AI, the perspective shifts somewhat. Most organisations already have policies in
place for public cloud adoption. However, the approach to AI and data must be
more nuanced, given the vast potential of the technology involved.
6 hard-earned tips for leading through a cyberattack — from CSOs who’ve been there

Authority under crisis is meaningless if you can’t establish followership. And
this goes beyond the incident response team: CISOs must communicate with the
entire organization — a commonly misunderstood imperative, says Pablo Riboldi,
CISO of nearshore talent provider BairesDev. ... “Organizations should provide
training on stress management and decision-making under pressure, which
includes perhaps mental health support resources in the incident response
plan,” Ngui says. Larry Lidz, vice president of CX Security at Cisco, also
advocates for tabletop exercises as a way to get employees to “look at
problems through a different set of lenses than they would otherwise look at
them.” ... Remaining calm in the face of a cyberattack can be
challenging, but prime performance requires it, New Relic’s Gutierrez says.
“There’s a lot of reaction. There’s a lot of strong feelings and emotions that
go on during incidents,” Gutierrez says. Although they had moments of not
maintaining composure, Gutierrez says they have been generally calm under
cyber duress, which they take pride in. Demonstrating composure as a leader
under fire is important because it can influence how others feel, behave, and
act.
A “Measured” Approach to Building a World-Class Offensive Security Program
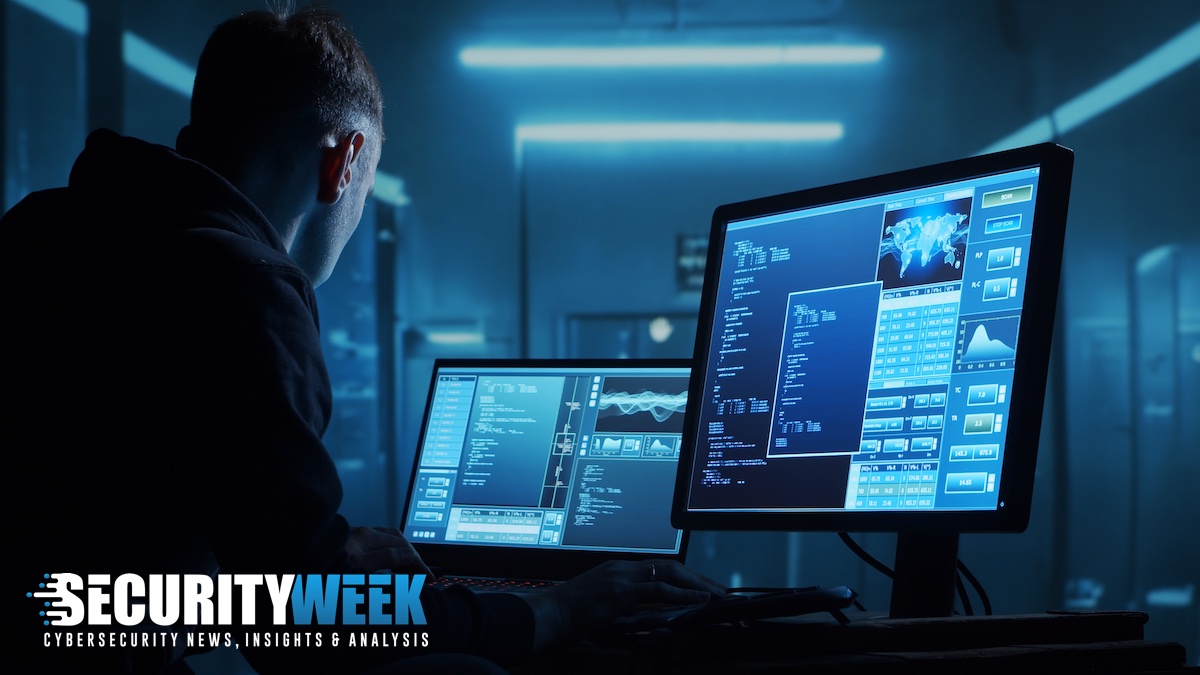
First, mapping the top threats and threat actors, most likely to find your
organization an attractive target. Second, the top “crown jewel” systems they
would target for compromise. Remaining at the enterprise level, the next step is
to establish an internal framework and underlying program that graphs threats
and risks, and provides a repeatable mechanism to track and refresh that
understanding over time. This includes graphs of all enterprise systems, and
their associated connections and dependencies, as well as attack graphs that
represent all the potential paths through your architecture that would lead an
attacker to their prize. Finally, the third element is an architectural security
review that discerns from the graphs what paths are most possible and probable.
Installing a program that guides and tracks three activities will also pay
dividends down the line in better informing and increasing the efficacy of
adversarial simulations. We all know the devil resides in the details. At this
stage we begin understanding the actual vulnerability of individual assets and
systems. The first step is a comprehensive inventory of elements that exist
across the organization. This includes internal endpoint assets, and external
perimeter and cloud systems. As you’d likely expect, the next step is
vulnerability scanning of the full asset inventory that was established.
How AI Agents Are Quietly Transforming Frontend Development

Traditional developer tools are passive. You run a linter, and it tells you
what’s wrong. You run a build tool, and it compiles. But AI agents are
proactive. They don’t wait for instructions; they interpret high-level goals and
try to execute them. Want to improve page performance? An agent can analyze your
critical rendering path, optimize image sizes, and suggest lazy loading. Want a
dark mode implemented across your UI library? It can crawl through your
components and offer scoped changes that preserve brand integrity. ... Frontend
development has always been plagued by complexity. Thousands of packages,
constantly changing frameworks, and pixel-perfect demands from designers. AI
agents bring sanity to the chaos, rendering cloud security the only thing to
worry about. But if you decide to run an agent locally, that problem is resolved
as well. They can serve as design-to-code translators, turning Figma files into
functional components. They can manage breakpoints, ARIA attributes, and
responsive behaviors automatically. They can even test components for edge cases
by generating test scenarios that a developer might miss. Because these agents
are always “on,” they notice patterns developers sometimes overlook. That
dropdown menu that breaks on Safari 14? Flagged. That padding inconsistency
between modals? Caught.
Agentic AI won’t make public cloud providers rich

Agentic AI isn’t what most people think it is. When I look at these systems, I
see something fundamentally different from the brute-force AI approaches we’re
accustomed to. Consider agentic AI more like a competent employee than a
powerful calculator. What’s fascinating is how these systems don’t need
centralized processing power. Instead, they operate more like distributed
networks, often running on standard hardware and coordinating across different
environments. They’re clever about using resources, pulling in specialized small
language models when needed, and integrating with external services on demand.
The real breakthrough isn’t about raw power—it’s about creating more
intelligent, autonomous systems that can efficiently accomplish tasks. The big
cloud providers emphasize their AI and machine learning capabilities alongside
data management and hybrid cloud solutions, whereas agentic AI systems are
likely to take a more distributed approach. These systems will integrate with
large language models primarily as external services rather than core
components. This architectural pattern favors smaller, purpose-built language
models and distributed processing over centralized cloud resources. Ask me how I
know. I’ve built dozens for my clients recently.
Cloud a viable choice amid uncertain AI returns
Enterprises can restrict data using internal controls and limit data movement to
chosen geographical locations. The cluster can be customized and secured to meet
the specific requirements of the enterprise without the constraints of using
software or hardware configured and operated by a third party. Given these
characteristics, for convenience, Uptime Institute has labeled the method as
“best” in terms of customization and control. ... The challenge for enterprises
is determining whether the added reassurance of dedicated infrastructure
provides a real return on its substantial premium over the “better” option. Many
large organizations - from financial services to healthcare - already use the
public cloud to hold sensitive data. To secure data, an organization may encrypt
data at rest and in transit, configure appropriate access controls, such as
security groups, and set up alerts and monitoring. Many cloud providers have
data centers approved for government use. It is unreasonable to view the cloud
as inherently insecure or non-compliant, considering its broad use across many
industries. Although dedicated infrastructure gives reassurance that data is
being stored and processed at a particular location, it is not necessarily more
secure or compliant than the cloud.
Why no small business is too small for hackers - and 8 security best practices for SMBs

To be clear, the size of your business isn't particularly relevant to bulk
attacks. It's merely the fact that you are one of many businesses that can be
targeted through random IP number generation or email harvesting or some other
process that makes it very, very cost-effective for a hacker to be able to
deliver a piece of malware that opens up computers in your business for
opportunistic activities. ... Attackers -- who could be affiliated with
organized crime groups, individual hackers, or even teams funded by
nation-states -- often use pre-built hacking tools they can deploy without a
tremendous amount of research and development. For hackers, this tactic is
roughly the equivalent of downloading an app from an app store, although the
hacking tools are usually purchased or downloaded from hacker-oriented websites
and hidden forums (what some folks call "the dark web"). ... "Many SMB owners
assume cybersecurity is too costly or too complex and think they don't have the
IT knowledge or resources to set up reliable security. Few realize that they
could set up security in a half hour. Moreover, the lack of dedicated cyber
staff further complicates the situation for SMBs, making it even more daunting
to implement and manage effective security measures."
AI is making the software supply chain more perilous than ever
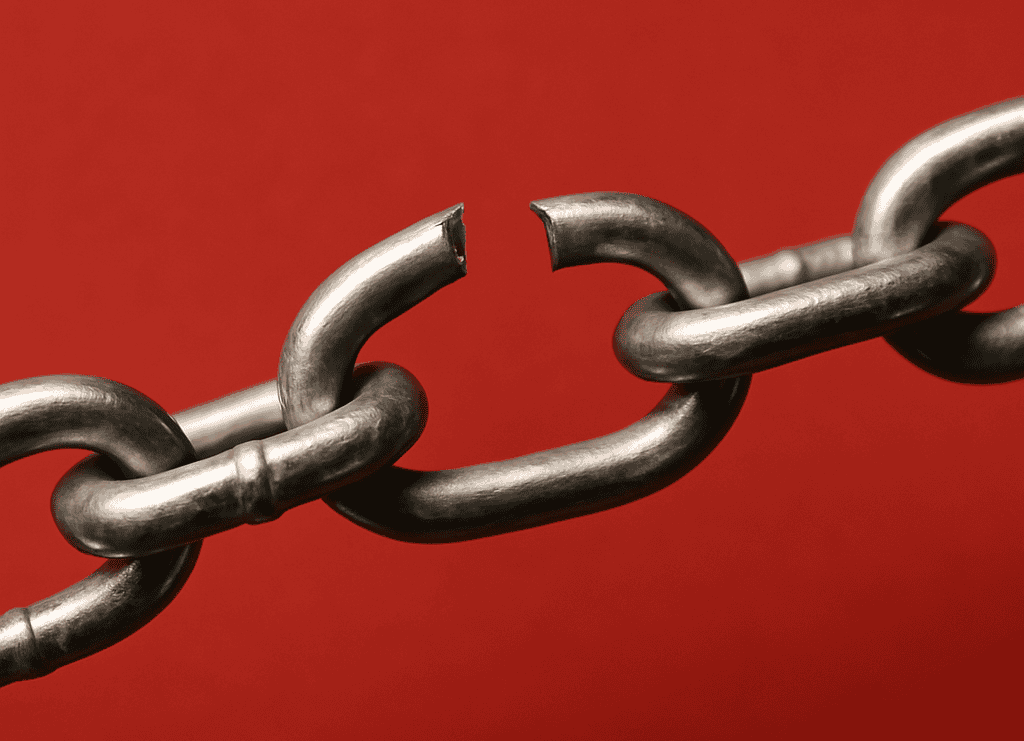
The software supply chain is a link in modern IT environments that is as crucial
as it is vulnerable. The new research report by JFrog, released during KubeCon +
CloudNativeCon Europe in London, shows that organizations are struggling with
increasing threats that are amplified by, how could it be otherwise, the rise of
AI. ... The report identifies a “quad-fecta” of threats to the integrity and
security of the software supply chain: vulnerabilities (CVEs), malicious
packages, exposed secrets and configuration errors/human error. JFrog’s research
team detected no fewer than 25,229 exposed secrets and tokens in public
repositories – an increase of 64% compared to last year. Worryingly, 27% of
these exposed secrets were still active. This interwoven set of security dangers
makes it particularly difficult for organizations to keep their digital walls
consistently in order. ... “More is not always better,” the report states.
The collection of tools can make organizations more vulnerable due to increased
complexity for developers. At the same time, visibility in the programming code
remains a problem: only 43% of IT professionals say that their organization
applies security scans at both the code and binary level. This is a decrease
from 56% compared to last year and indicates that teams still have large blind
spots when identifying software risks.