Self-supervised learning is the future of AI
Supervised deep learning has given us plenty of very useful applications, especially in fields such as computer vision and some areas of natural language processing. Deep learning is playing an increasingly important role in sensitive applications, such as cancer detection. It is also proving to be extremely useful in areas where the scale of the problem is beyond being addressed with human efforts, such as—with some caveats—reviewing the huge amount of content being posted on social media every day. “If you take deep learning from Facebook, Instagram, YouTube, etc., those companies crumble,” LeCun says. “They are completely built around it.” But as mentioned, supervised learning is only applicable where there’s enough quality data and the data can capture the entirety of possible scenarios. As soon as trained deep learning models face novel examples that differ from their training examples, they start to behave in unpredictable ways. In some cases, showing an object from a slightly different angle might be enough to confound a neural network into mistaking it with something else.
Banks Need to Learn What Big Tech Teaches
Tech advancements can be revolutionary. Let’s consider the case of the smartphone – be it iPhone or Android, a smartphone is essentially a group of services packaged together in a physical phone. Those services put an amazing amount of power and capabilities literally into the palm of your hand. Software updates occur frequently (talk about a rapid pace of change), yet users are supremely indifferent – and often unaware – of which version operating system they are using… regardless, they welcome new features that are delivered as part of nonintrusive upgrades that are installed while they sleep or at whatever time they specify. Similarly, smart-equipped cars such as Tesla regularly receive over-the-air software updates that add new features and enhance functionality. No one asked for the addition of Tesla’s Sentry Mode (not even Tesla) when the car was designed. It was an afterthought (albeit a brilliant one), delivered as part of a continuous upgrade. Now drivers can monitor their Tesla wherever it’s parked and receive alerts whenever a security incident occurs.
Enabling Manufacturing using IOTA – A possible approach post Covid-19 paradigm
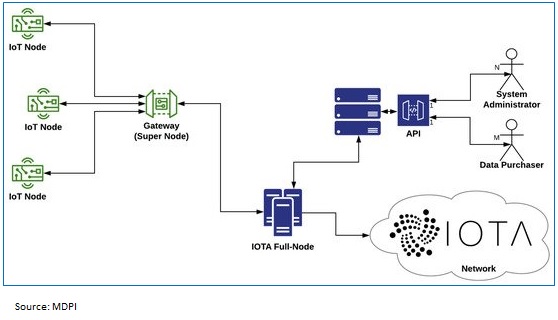
Internet of Things is no more a technological breakthrough. Industrial applications have been faster in adoption of IoT and it has been playing a significant role for businesses that requires internal tracking, attaining near to zero error with less manual intervention, enabling machine to machine talking along with prognostic maintenance. RFID chips and other sensors are much cheaper in terms of cost and easier to manufacture than most of the sizeable and lumbering consumer electronics. The future of IoT will continue in these lines, especially post COVID with lot of manufacturing concerns embracing automation at a massive scale gradually shaping the smart industrial applications concept. However, the block-chain of IoT also calls for distributed and secure exchange of data captured through these sensors or devices. The interconnection of block-chain technology and IoT have been in the scenario since 2015, to solve critical IoT challenges related to security and data privacy. The IOTA protocol has been able to enter into collaborations which technically differentiates itself from most of the cryptocurrencies by its underlying technology that uses Directed Acyclic Graph (DAG) as a distributed ledger which stores the transactional data of the IOTA network, instead of block-chain enabled transactions.
A Reassessment of Enterprise Architecture Implementation
The research question in this contribution is: What are factor combinations for successful EA implementation beyond the mere notion of maturity? As a basis of our analysis we will employ a description framework which has been developed in the course of various practitioners’ workshops over the last eight years. Based on this description framework we will analyze six cases and discuss why certain companies have been rather successful in implementing EA while others did not leverage their EA invest. The analysis will show that EA success is not necessarily a matter of maturity of a number of EA functions but a complex set of factors that have to be observed for implementing EA. Also there is no perfect set of EA factor combinations guaranteeing successful EA because EA always is part of a complex socio-technical network. However, we will identify successful factor combinations as well as common patterns prohibiting EA success.
Supercomputers hacked across Europe to mine cryptocurrency

The malware samples were reviewed earlier today by Cado Security, a US-based cyber-security firm. The company said the attackers appear to have gained access to the supercomputer clusters via compromised SSH credentials. The credentials appear to have been stolen from university members given access to the supercomputers to run computing jobs. The hijacked SSH logins belonged to universities in Canada, China, and Poland. Chris Doman, Co-Founder of Cado Security, told ZDNet today that while there is no official evidence to confirm that all the intrusions have been carried out by the same group, evidence like similar malware file names and network indicators suggests this might be the same threat actor. According to Doman's analysis, once attackers gained access to a supercomputing node, they appear to have used an exploit for the CVE-2019-15666 vulnerability to gain root access and then deployed an application that mined the Monero (XMR) cryptocurrency. Making matters worse, many of the organizations that had supercomputers go down this week had announced in previous weeks that they were prioritizing research on the COVID-19 outbreak, which has now most likely been hampered as a result of the intrusion and subsequent downtime.
How AI & Blockchain Can Reshape Healthcare Industry?

Blockchain technology is one of the most important and disruptive technologies in the world that is being used to unlock unexplored innovations in the healthcare industry. Blockchain technology is expected to improve medical record management and the insurance claim process, accelerate clinical and biomedical research and advance biomedical and healthcare data ledger. These expectations are based on the key aspects of blockchain technology, such as decentralized management, immutable audit trail, data provenance, robustness, and improved security and privacy. Although several possibilities have been discussed, the most notable innovation that can be achieved with blockchain technology is the recovery of data subjects’ rights. Medical data should be possessed, operated, and allowed to be utilized by data subjects other than hospitals. This is a key concept of patient-centered interoperability that differs from conventional institution-driven interoperability. There are many challenges arising from patient-centered interoperability, such as data standards, security, and privacy, in addition to technology-related issues, such as scalability and speed, incentives, and governance.
Five Strategies for Putting AI at the Center of Digital Transformation

Specifically, quick wins are smaller projects that involve optimizing internal employee touch points. For example, companies might think about specific pain points that employees experience in their day-to-day work, and then brainstorm ways AI technologies could make some of these tasks faster or easier. Voice-based tools for scheduling or managing internal meetings or voice interfaces for search are some examples of applications for internal use. While these projects are unlikely to transform the business, they do serve the important purpose of exposing employees, some of whom may initially be skeptics, to the benefits of AI. These projects also provide companies with a low-risk opportunity to build skills in working with large volumes of data, which will be needed when tackling larger AI projects. The second part of the portfolio approach, long-term projects, is what will be most impactful and where it is important to find areas that support the existing business strategy.
For all its sophistication, AI isn't fit to make life-or-death decisions
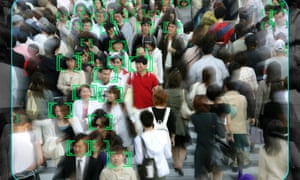
Reckoning is essentially calculation: the ability to manipulate data and recognise patterns. Judgment, on the other hand, refers to a form of “deliberative thought, grounded in ethical commitment and responsible action, appropriate to the situation in which it is deployed”. Judgment, Smith observes, is not simply a way of thinking about the world, but emerges from a particular relationship to the world that humans have and machines do not. Humans are both embodied and embedded in the world. We are able to recognise the world as real and as unified but also to break it down into distinct objects and phenomena. We can represent the world but also appreciate the distinction between representation and reality. And, most importantly, humans possess an ethical commitment to the real over the representation. What is morally important is not the image or mental representation I have of you, but the fact that you exist in the world. A system with judgment must, Smith insists, not simply be able to think but also to “care about what it is thinking about”. It must “give a damn”. Humans do. Machines don’t.
The Different Kind of Value That EA & EA Framework Return to the Enterprise

Reference Architecture is a generic architecture adopted as a standard for the analysis and design of systems in the same class. To be validated as a reference, rather than declared as such by its promoters, a generic architecture must be adopted enough, having been reused and proved in many developments. A reference architecture, in addition to a generic architecture, exhibits the benefits of standards. A reference architecture facilitates wide acceptance and reuse, predictable and comparable designs, reproducibility and as such productivity which saves time and costs. TOGAF is no reference architecture though because it proposes no architecture. It is called a standard though because is specified by a standards organization with wide industry participation. TOGAF is not even a standard enterprise architecture method though because it is hard to comply or prove compliance with it with due to its size and organic organisation and, most importantly, it does not deliver the enterprise architecture we are after but most good development practices.
Change-mapping: Plan and Action
In reality, that apparent sequence exists only because of the dependencies between each of those domains: we need to know something about Context in order to define Scopes, we need to know Scope-boundaries for any Plan, we need to be clear about the Plan and preparation before we start any Action, and we need the results of any Action, and all the setup and Scope and Context, before we can do the respective Review. There may well be quite a lot of back-and-forth between the domains as details get fleshed out and call for a rethink of what happened earlier, which would break up the sequence somewhat. And there can also be multiple instances of each domain: a context may spin off several Scopes, a Scope may require multiple projects or Plans, and each Plan my have multiple Actions, each of which will require their own Review. In that sense, no, it’s not just a straightforward single-pass linear sequence: it can often be a lot more complex than that. Yet the overall flow does line up well with that pattern – which is why it’s simplest to show it that way.
Quote for the day:
"Mistakes are always forgivable, if one has the courage to admit them." -- Bruce Lee
No comments:
Post a Comment