A new artificial eye mimics and may outperform human eyes
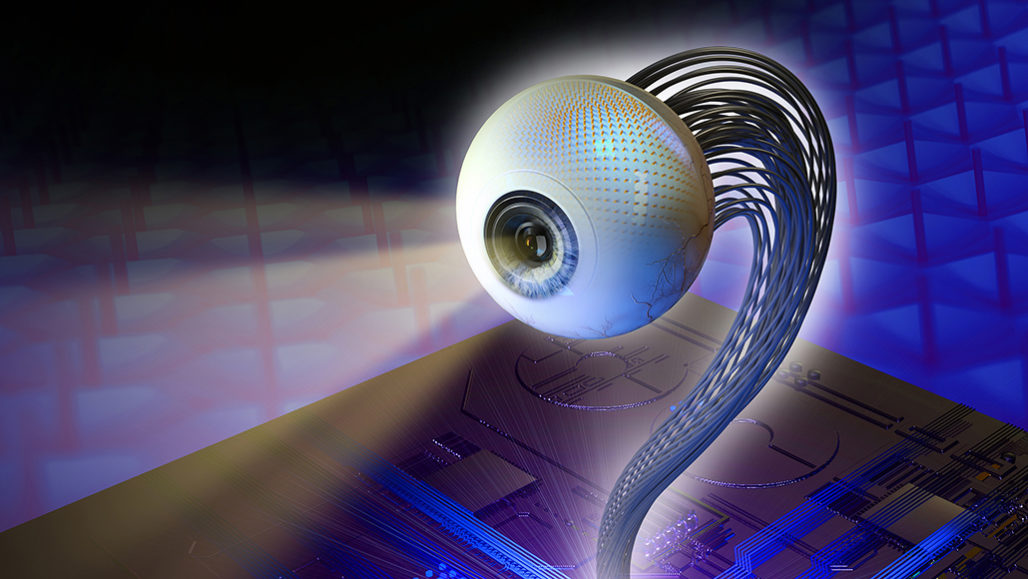
This device, which mimics the human eye’s structure, is about as sensitive to light and has a faster reaction time than a real eyeball. It may not come with the telescopic or night vision capabilities that Steve Austin had in The Six Million Dollar Man television show, but this electronic eyepiece does have the potential for sharper vision than human eyes, researchers report in the May 21 Nature. “In the future, we can use this for better vision prostheses and humanoid robotics,” says engineer and materials scientist Zhiyong Fan of the Hong Kong University of Science and Technology. The human eye owes its wide field of view and high-resolution eyesight to the dome-shaped retina — an area at the back of the eyeball covered in light-detecting cells. Fan and colleagues used a curved aluminum oxide membrane, studded with nanosize sensors made of a light-sensitive material called a perovskite (SN: 7/26/17), to mimic that architecture in their synthetic eyeball. Wires attached to the artificial retina send readouts from those sensors to external circuitry for processing, just as nerve fibers relay signals from a real eyeball to the brain.
Given the clear finding that people with covid-19 can be highly contagious even if they display few or no symptoms, a growing number of companies and health experts argue that reopening plans must also include wide-scale and continual testing of workers. “It’s less a question of if testing becomes a part of workplace strategies, than when and what will prompt that,” says Rajaie Batniji, chief health officer at Collective Health. Measures like temperature checks may even do more harm than good by giving workers and employers a false sense of confidence, he says. The San Francisco company, which manages health benefits for businesses, has developed a product called Collective Go that, among other things, includes detailed health protocols for companies looking to reopen. Developed in partnership with researchers at Johns Hopkins, the University of California, San Francisco, and elsewhere, the guidelines include when and how often workers in various job types and locations should be tested.
How effective security training goes deeper than ‘awareness’
While the approach may be up for debate, its effectiveness is not. Almost 90% of organisations report an improvement in employee awareness following the implementation of a consequence model. The model itself is secondary here. The key takeaway is that time and effort matter. The more hands-on training workers receive, the better they are at spotting phishing attempts. Organisations must strive to develop training programmes that leave employees equipped with the skills to spot and defend against attacks – before anyone is left to face the consequences. The goal of any security training programme is to eradicate behaviours that put your organisation at risk. The best way to achieve this is through a mix of the broad and the granular. Start by cultivating a security-first culture. This means a continuous, company-wide training programme that acknowledges everyone’s role in keeping your organisation safe.
Gaming: A gold mine for datasets

While working on a project, I came across a problem where the object detector that I was using did not recognize all the objects in the image frame. I was trying to index all the objects present in the images frame, which later would make searching of images easier. But all the images are labeled human, not being able to detect the other objects in the image frames, the search was not working as I wanted. The ideal solution for this problem would be to gather data for those objects and re-train the object detector to also identify the new objects. This would not only be boring but time-consuming. I could use GANs, a type of machine learning algorithm famous for its use of creating artificial and similar examples to its inputs, to create more samples after organizing a few samples manually, but this is also boring and will require resources to train the GANs to generate more data. Now the only thing I could do was using internet services, like ScaleAI and Google Cloud Platform, to create a dataset.
Identity Silos: shining a light on the problem of shadow identities
It’s important to stress that identity silos – sometimes referred to as ‘shadow identities’ because, similar to shadow IT, they are created without central organisational approval – come about during routine business expansion. If a business unit wishes to roll out a new digital service, in the absence of an existing centralised identity management function that can do the job, they often end up either buying an off-the-shelf identity and access management (IAM) system or create their own. When a business merges or acquires a new organisation, the new unit often keeps its own IAM infrastructure. In both cases, the result is the same: hundreds of invisible silos of duplicated user identities. The chances are you’ve experienced the problems caused by identity silos. If you use the same broadband, mobile, and television provider, you’ve probably had to update the same information multiple times for each account, rather than just once. Or if you’ve been subjected to marketing calls (even though you’re already a customer!) that try to sell you products you already have. This is all because your customer data is siloed in each department throughout the company, thereby ruling out cohesive customer experiences.
How to overcome AI and machine learning adoption barriers
Many industries have effectively reached a sticking point in their adoption of AI and ML technologies. Typically, this has been driven by unproven start-up companies delivering some type of open source technology and placing a flashy exterior around it, and then relying on a customer to act as a development partner for it. However, this is the primary problem – customers are not looking for prototype and unproven software to run their industrial operations. Instead of offering a revolutionary digital experience, many companies are continuing to fuel their initial scepticism of AI and ML by providing poorly planned pilot projects that often land the company in a stalled position of pilot purgatory, continuous feature creep and a regular rollout of new beta versions of software. This practice of the never ending pilot project is driving a reluctance for customers to then engage further with innovative companies who are truly driving digital transformation in their sector with proven AI and ML technology.
Coronavirus to Accelerate ASEAN Banks’ Digital Transformation

The shift towards a digital-channel strategy is likely to be significantly amplified now that customer preferences are abruptly adjusted. DBS Bank Ltd. (AA-/Rating Watch Negative) has in the past reported that the cost-to-income ratio of its digital customers is roughly 20pp lower than its non-digital banking clients, implying considerable potential productivity to be gained in the longer term should the trend persists. That said, actual investments in IT are likely to be tempered in the near term as banks look to cut overall costs in the face of significant business uncertainty. We believe that the significantly higher adoption rate of digital banking is likely to help more of the well-established, digitally advanced banks to widen their competitive advantage further against the less agile players as well as the incoming digital-only banks in the medium term. Regulators around the region have already extended the deadline for awarding virtual bank licences as a result of the pandemic, which we expect to also weed out weaker, aspiring online banks from competing for the licences.
7 ways to catch a Data Scientist’s lies and deception
In Machine Learning, there is often a trade-off between how well a model performs and how easily its performance, especially poor performance, can be explained. Generally, for complex data, more sophisticated and complicated models tend to do better. However, because these models are more complicated, it becomes difficult to explain the effect of input data on the output result. For example, let us imagine that you are using a very complex Machine Learning model to predict the sales of a product. The inputs to this model are the amounts of money spent on advertising on TV, newspaper and radio. The complicated model may give you very accurate sales predictions but may not be able to tell you which of the 3 advertisement outlets, TV, radio or newspaper, impacts the sales more and is more worth the money. A simpler model, on the other hand, might have given a less accurate result, but would have been able to explain which outlet is more worth the money. You need to be aware of this trade-off between model performance and interpretability. This is crucial because where the balance should lie on the scale of explainability vs performance, should depend on the objective and hence, should be your decision to make.
Supercomputer Intrusions Trace to Cryptocurrency Miners
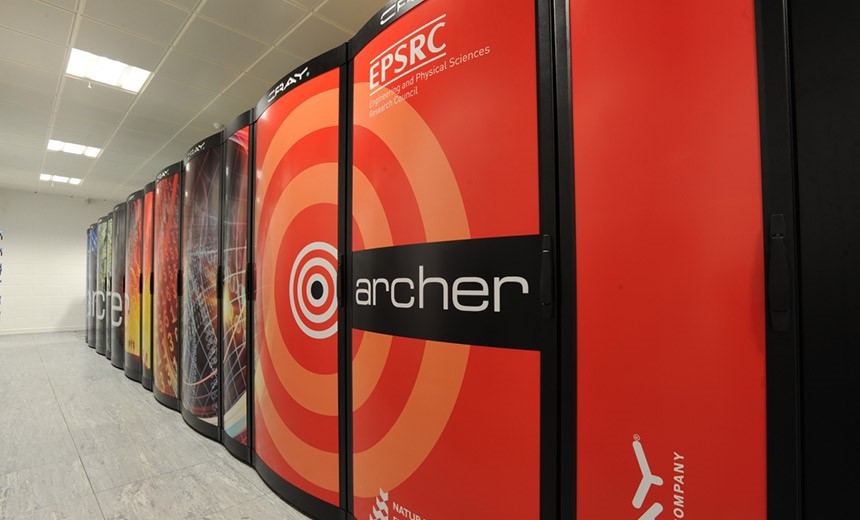
Attacks against high-performance computing labs, which first came to light last week, appear to have targeted victims in Canada, China, the United States and parts of Europe, including the U.K., Germany and Spain. Security experts say all of the incidents appear to have involved attackers using SSH credentials stolen from legitimate users, which can include researchers at universities and consortiums. SSH - aka Secure Shell or Secure Socket Shell - is a cryptographic network protocol for providing secure remote login, even over unsecured networks. Supercomputer and high-performance clusters offer massive computational power to researchers. But any attackers able to sneak cryptomining malware into such environments could use them to mine for cryptocurrency, referring to solving computationally intensive equations in exchange for potentially getting digital currency as a reward. On May 11, the bwHPC consortium, comprising researchers and 10 universities in the German state of Baden-Württemberg, reported that it had suffered a "security incident" and as a result took offline multiple supercomputers and clusters.
Apache Arrow and Java: Lightning Speed Big Data Transfer
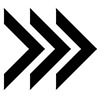
Apache Arrow puts forward a cross-language, cross-platform, columnar in-memory data format for data. It eliminates the need for serialization as data is represented by the same bytes on each platform and programming language. This common format enables zero-copy data transfer in big data systems, to minimize the performance hit of transferring data. ... Conceptually, Apache Arrow is designed as a backbone for Big Data systems, for example, Ballista or Dremio, or for Big Data system integrations. If your use cases are not in the area of Big Data systems, then probably the overhead of Apache Arrow is not worth your troubles. You’re likely better off with a serialization framework that has broad industry adoption, such as ProtoBuf, FlatBuffers, Thrift, MessagePack, or others. Coding with Apache Arrow is very different from coding with plain old Java objects, in the sense that there are no Java objects. Code operates on buffers all the way down. Existing utility libraries, e.g., Apache Commons, Guava, etc., are no longer usable. You might have to re-implement some algorithms to work with byte buffers. And last but not least, you always have to think in terms of columns instead of objects.
Quote for the day:
"If you don't write your own story, you'll be nothing but a footnote in someone else's." -- Peter Shankman
No comments:
Post a Comment