An an individual, I can demand to receive my personal data from a supplier, and I can demand that the supplier then deletes all the personal information they have on me (subject to legal constraints – e.g. companies have a right to keep prior billing information, since it’s an statutory part of the accounting record). This is far from being an agreed-upon full “legal ownership right” to the information, but (as the famous saying goes), possession is nine-tenths of the law. As any economist can tell you, ownership rights have a profound impact on how markets are structured — so this might just be the start of a truly fundamental change to the entire industry. Revenge. What can you do today if you have an awful customer experience with a company? You can complain directly to the company, or to the world in general on social media. But you now have another potent weapon: you can demand that the company gives you all your personal data, and then exercise your “right to be forgotten”. Given the complex nature of computer systems in most organizations, this is currently likely to be a very manual and expensive process for the companies involved.
How to control state for so-called stateless microservices
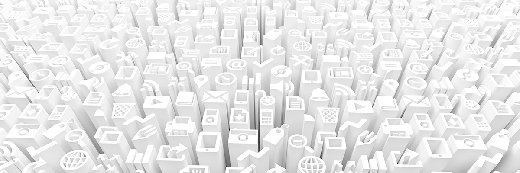
Front-end state control is suitable if the transactions are handled by a web or mobile GUI/app, and this front-end server controls the sequence of steps being taken. Sometimes, the front-end process can make truly stateless requests to microservices. When it doesn't, the front end can provide the state as part of the data it sends to the microservice, and the microservice can then adjust its processing based on that state. This approach doesn't add any complexity or processing delay to the app's design. Back-end state control is the more complex approach to take with stateless microservices, from developmental and operational perspectives. With back-end state control, the microservice maintains a database of state information, and it accesses that database when it has to process a message. If the microservice supports numerous transactions, a problem arises because it must determine which back-end database record corresponds with the current message. Sometimes, a transaction ID, timestamp or other unique identifier is provided for logging and can be used for state control as well.
Switzerland seeks to regain cryptocurrency crown
Swiss banks are urging the authorities to give them more clarity on the rules that apply to cryptocurrency projects before providing services to the market, and at least two important players have withdrawn for now. Zuercher Kantonalbank (ZKB), the fourth largest Swiss bank and one of the few big banks in the world to welcome issuers of cryptocurrencies, has closed the accounts of more than 20 companies in the last year, industry sources told Reuters. A spokesman for ZKB declined to comment on any former or existing clients relationships, but said the bank does not do business with any cryptocurrency groups. Another large Swiss bank kicked out crypto project Smart Valor at around the same time, said a person familiar with the project. The source declined to name the bank. Only a handful of Switzerland’s 250 banks ever allowed companies to deposit the cash equivalent of cryptocurrencies raised in ICOs. At least two still do, Reuters has established. But the involvement of a large bank like ZKB helped to establish Switzerland as an early cryptocurrency hub.
Tracking Disinformation by Reading Metadata

When we read metadata that’s been exploited or gamed in social media platforms as data craft, we can decode the signals and noise found in automated disinformation campaigns. Data craftwork not only gives us insight into the emerging techniques of manipulators, it is also a way of understanding the power structures of platforms themselves, a means of apprehending the currents and flows of personalization algorithms that underwrite the classification mechanisms that now structure our digital lives. But before we can understand how metadata categories are harnessed and hacked, it’s necessary to have a fuller picture of what platform metadata is, how it is encoded and decoded, and how it is created and collected for use by a range of actors — from technologists and providers, to individual users, to governments, to media manipulators. Currently, there is a range of known manipulation tactics for gaming engagement data. Social media professionals are known to inflate engagement by increasing likes, views, follower counts, and comments for profit.
Justice Department unveils strategy to fight election meddling, cybercrime
In the encryption section, DOJ notes that it cannot rely solely on purchasing workarounds like Cellebrite or GrayKey. “Expanding the government’s exploitation of vulnerabilities for law enforcement purposes will likely require significantly higher expenditures — and in the end it may not be a scalable solution,” the report warns. “All vulnerabilities have a limited lifespan and may have a limited scope of applicability.” Another problem relevant to election security is that the Computer Fraud and Abuse Act only empowers DOJ to prosecute people who hack internet-connected devices. “In many conceivable situations, electronic voting machines will not meet those criteria, as they are typically kept off the Internet,” the report notes. “Consequently, should hacking of a voting machine occur, the government would not, in many conceivable circumstances, be able to use the CFAA to prosecute the hackers.” At the Aspen event, Rosenstein said the report underscored how DOJ “must continually adapt criminal justice and intelligence tools to combat hackers and other cybercriminals.”
The cashless society is a con – and big finance is behind it
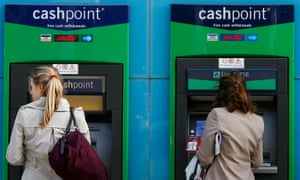
A cashless society brings dangers. People without bank accounts will find themselves further marginalised, disenfranchised from the cash infrastructure that previously supported them. There are also poorly understood psychological implications about cash encouraging self-control while paying by card or a mobile phone can encourage spending. And a cashless society has major surveillance implications. Despite this, we see an alignment between government and financial institutions. The Treasury recently held a public consultation on cash and digital payments in the new economy. It presented itself as attempting to strike a balance, noting that cash was still important. But years of subtle lobbying by the financial industry have clearly paid off. The call for evidence repeatedly notes the negative elements of cash – associating it with crime and tax evasion – but barely mentions the negative implications of digital payments. The UK government has chosen to champion the digital financial services industry. This is irresponsible and disingenuous. We need to stop accepting stories about the cashless society and hyper-digital banking being “natural progress”.
Putting the promises of artificial intelligence to the test

To ensure valid, reliable, safe and ethical AI decision-making, we therefore need to develop robust approaches to teaching and training AI applications. This calls for a new testing regime, tailor-made for AI applications, that ensures adequate transparency in the decisioning mechanism in a way that users can understand; and provides an assurance of fairness and non-discrimination in the decision process. The key challenge in developing such a testing regime is that AI software has many moving parts. When testing AI applications, engineers must consider many variables which include processing unstructured data, managing the variety and veracity of data, the choice of algorithms, evaluating the accuracy and performance of the learning models and ensuring ethical and unbiased decisioning by the new system along with regulatory and compliance adherence. New testing and monitoring processes which account for the data-dependent nature of these systems also need to be developed. One way to break down the development and validation requirements for AI is to divide the work into two stages. The first stage is the ‘Teach’ stage, where the system is trained to produce a set of outputs by learning patterns in training data through various algorithms.
Are you scared yet? Meet Norman, the psychopathic AI
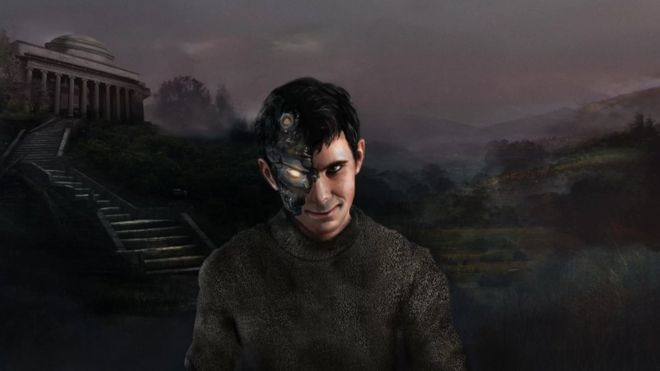
The program flagged that black people were twice as likely as white people to reoffend, as a result of the flawed information that it was learning from. Predictive policing algorithms used in the US were also spotted as being similarly biased, as a result of the historical crime data on which they were trained. Sometimes the data that AI "learns" from comes from humans intent on mischief-making so when Microsoft's chatbat Tay was released on Twitter in 2016, the bot quickly proved a hit with racists and trolls who taught it to defend white supremacists, call for genocide and express a fondness for Hitler. ... "When we train machines by choosing our culture, we necessarily transfer our own biases," she said. "There is no mathematical way to create fairness. Bias is not a bad word in machine learning. It just means that the machine is picking up regularities." What she worries about is the idea that some programmers would deliberately choose to hard-bake badness or bias into machines. To stop this, the process of creating AI needs more oversight and greater transparency, she thinks.
UK alerted to potential cyber risks of Huawei equipment
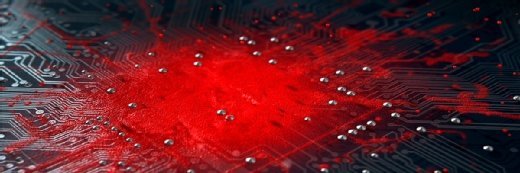
The report said Huawei is failing to follow agreed security processes around the use of third-party components. “In particular, security critical third-party software used in a variety of products was not subject to sufficient control.” ... A company spokesman said: “We are grateful for this feedback and are committed to addressing these issues. Cyber-security remains Huawei's top priority, and we will continue to actively improve our engineering processes and risk management systems.” The report said the National Security Adviser Mark Sedwill had been alerted to the issues in February and that work continues to remediate the engineering process issues in other products that are deployed in the UK, prioritised based on risk profiles and deployment volumes. “This work should give us the ability to provide end-to-end assurance that the code analysed by HCSEC is the constituent code used to build the binary packages executed on the network elements in the UK,” the report said, adding that until this work is completed, the Oversight Board can offer only limited assurance due to the lack of the required end-to-end traceability from source code examined by HCSEC through to executables use by the UK operators.
The reasons are simple. Reactive maintenance work costs four to five times as much as proactively replacing worn parts. When equipment fails because there is a lack of awareness of degraded performance there are immediate costs as a result of lost productivity, inventory backup, delays in completing the finished product, and more. A study by The Wall Street Journal and Emerson reported that unplanned downtime, which is caused 42% of the time by equipment failure, amounts to an estimated $50 billion per year for industrial manufacturers. Even after production begins again, the costs of interrupting operations continue. According to the Customers‘ Voice: Predictive Maintenance in Manufacturing report by Frenus, approximately 50% of all large companies face quality issues after an unplanned shutdown. In addition to savings, predictive maintenance can also result in competitive differentiation. When machine data can be used to perform predictive maintenance with a high level of precision, manufacturers can focus on differentiating products using digital capabilities like self-healing based on an awareness of technical health.
Quote for the day:
"Do not follow where the path may lead. Go instead where there is no path and leave a trail." -- Muriel Strode
No comments:
Post a Comment