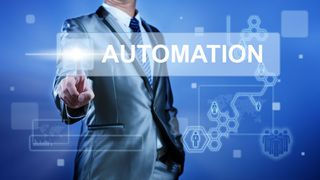
Python has brought computer programming to a vast new audience

Not all Pythonistas are so ambitious, though. Zach Sims, Codecademy’s boss, believes many visitors to his website are attempting to acquire skills that could help them in what are conventionally seen as “non-technical” jobs. Marketers, for instance, can use the language to build statistical models that measure the effectiveness of campaigns. College lecturers can check whether they are distributing grades properly. For professions that have long relied on trawling through spreadsheets, Python is especially valuable. Citigroup, an American bank, has introduced a crash course in Python for its trainee analysts. A jobs website, eFinancialCareers, reports a near-fourfold increase in listings mentioning Python between the first quarters of 2015 and 2018. The thirst for these skills is not without risk. Cesar Brea, a partner at Bain & Company, a consultancy, warns that the scariest thing in his trade is “someone who has learned a tool but doesn’t know what is going on under the hood”. Without proper oversight, a novice playing with AI libraries could reach dodgy conclusions.
Top 10 Data Science Use Cases in Insurance

The customers are always willing to get personalized services which would match their needs and lifestyle perfectly well. The insurance industry is not an exception in this case. The insurers face the challenge of assuring digital communication with their customers to meet these demands. Highly personalized and relevant insurance experiences are assured with the help of the artificial intelligence and advanced analytics extracting the insights from a vast amount of the demographic data, preferences, interaction, behavior, attitude, lifestyle details, interests, hobbies, etc. The consumers tend to look for personalized offers, policies, loyalty programs, recommendations, and options. The platforms collect all the possible data to define the major customers` requirements. After that, the hypothesis on what will work or won`t work is made. ... Modern technologies have brought the promotion of products and services to a qualitatively new level. Different customers tend to have specific expectations for the insurance business. Insurance marketing applies various techniques to increase the number of customers and to assure targeted marketing strategies. In this regard, customer segmentation proves to be a key method.
The Evolution Of Data

Traditionally, a platform was used to address an enterprise process workflow — human resources (HR), finance, manufacturing, etc. They are what we categorize as enterprise resource planning (ERP), customer relationship management (CRM), human capital management (HCM), functional setup manager (FSM), information technology operations (ITOps), etc. The data generated by these workflows was then analyzed using analytics or business intelligence applications to make further modifications to workflow. These workflow applications were customized as the data warranted any changes in the workflow. ... The workflow actions will be passed on to the traditional applications or directly to the people or system that will perform the actions. These new systems of intelligence will emerge and will force existing workflow applications to change to be end-user targeted. We are already seeing a trend where AI platforms are slowly becoming a playground for new intelligent applications. More importantly, because open source intelligent platforms in this area are as rich as the enterprise platforms, we are also noticing new generations of applications.
6 trends that are changing the face of UX
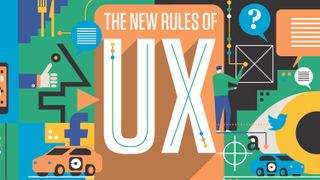
A pattern library acts as a centralised hub for all components of the user interface. Effective pattern libraries provide pattern descriptions, annotations and contextual information. They also showcase the code and pattern variations, and have the ability to add real data into the pattern structure. Once a design system is up and running, it’s only the first step in the journey. It needs to be living. Nathan Curtis, a co-founder of UX firm EightShapes, says: “A design system isn’t a project. It’s a product, serving products.” Like any good product, a design system needs maintenance and improvements to succeed. Both Google and Salesforce have teams dedicated to improving their design systems. The goal is a workflow where changes to the design system update the documentation and the code. The benefits realised by a thoughtful, unified design system outweigh the effort involved in establishing one. There is a consistency across the entire user experience. Engineers and designers share a common language and systems are more sustainable. Designers can spend their time solving harder problems and the actual user experience.
What’s so special about 5G and IoT?
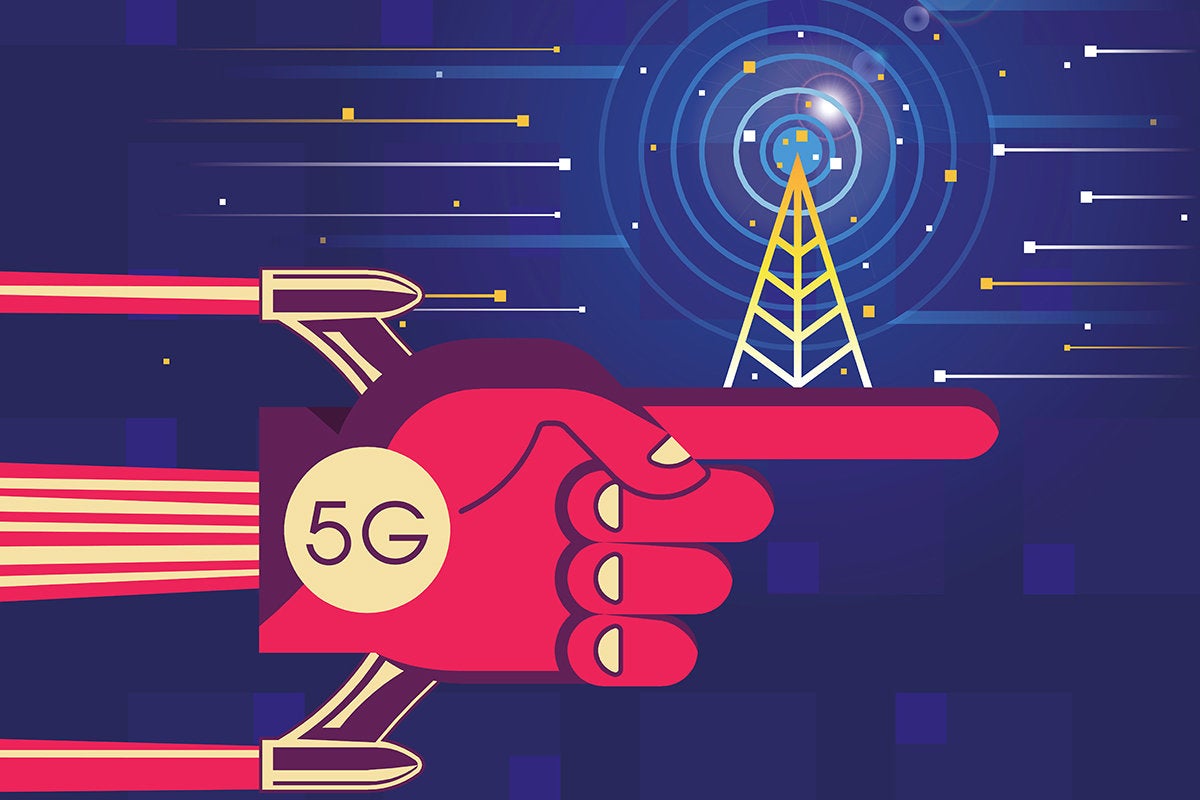
If we think about our current needs for IoT, what we care about are three things: price, coverage, and lower power consumption. But 5G is focused on increasing bandwidth, and while increased data transfer and speeds are nice, they are not entirely necessary for IoT products. The GSMA outlines 5G will possibly offer 1000x bandwidth per unit area. However, as they state in their own report, bandwidth per unit area is not dependent upon 5G, but more devices connecting with higher bandwidths for longer durations. While it is great that 5G aims to improve this service, the rollout of LTE has already had a significant effect on bandwidth consumption. We should be excited about continued incremental improvements on Cat-M1 and NB-IoT as we get even lower cost and lower power solutions for our IoT applications. Unlike LTE, 5G lacks a solid definition, which means cellular providers could eventually label a slightly-faster-than-LTE connection as 5G. And truly, the only thing that is certain about 5G is we won’t know what it can and cannot do until it arrives.
Microsoft's Linux love-in continues as PowerShell Core comes to Ubuntu Snap Store
Evidence of that newfound affection has been evident throughout 2018: with Ubuntu 18.04 being made available in the Microsoft Store, Windows File Explorer gaining the ability to launch a Linux Shell and a new option to install Windows Subsystem for Linux (WSL) distros from the command line. That's without mentioning Microsoft's release of the Linux-based Azure Sphere operating system. Now Microsoft has released its command-line shell and scripting language PowerShell Core for the Ubuntu Snap Store, as part of PowerShell Core's release as a snap package. Snap packages are containerized applications that can be installed on many Linux distributions, which Joey Aiello, PM for PowerShell at Microsoft, says has several advantages. "Snap packages carry all of their own dependencies, so you don't need to worry about the specific versions of shared libraries installed on your machine," he said, adding updates to Snaps happen automatically, and are "safe to run" as they don't interact with other applications or system files without your permission.Why Design Thinking Should Also Serve As A Leadership Philosophy
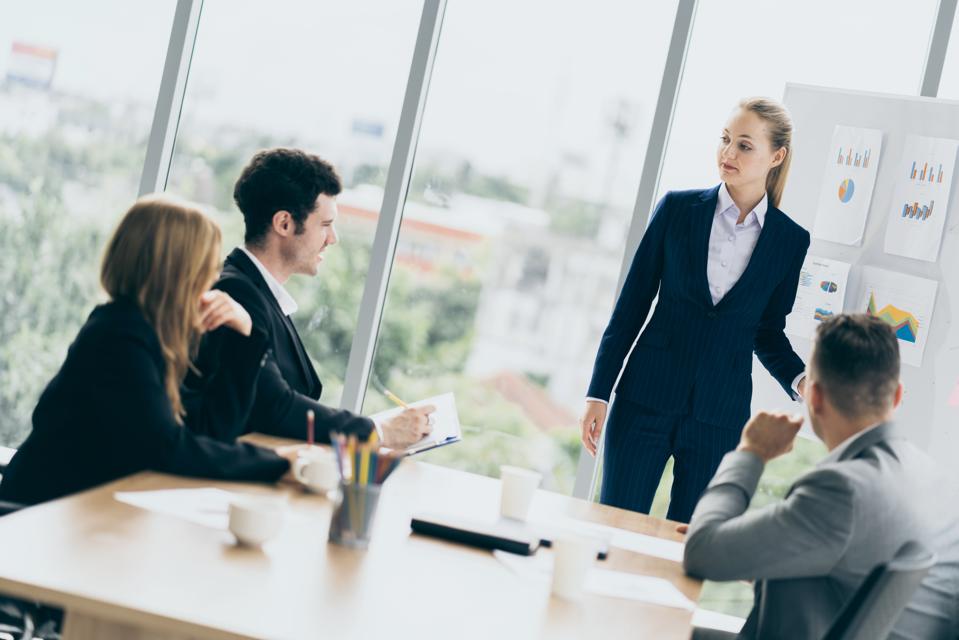
Managing Engineering Complexity: Are You Ready?
So, here is the complexity loop we are in: customers demanding more capabilities leads to more complexity in IoT systems, constantly feeding data into the development processes, leading to new security and safety standards requirements, new use cases, and the need to adapt fast to changes that companies cannot always predict. These actions lead to the demand for even more complex IoT systems. And, with these new changes, new customers’ demands arise and the loop continues perpetually. Let’s zoom in for a second and see what that means for one of the most exciting industries today – automotive engineering, i.e., how we build a car. What characterizes the OEM leaders today is the desire for speed in product development and a capability of overcoming the complexity of connecting requirements, design, development, validation, and deployment within their engineering process and throughout their supply chain. And how they do that?The Role of Randomization to Address Confounding Variables in Machine Learning
Machine learning practitioners are typically interested in the skill of a predictive model and less concerned with the statistical correctness or interpretability of the model. As such, confounding variables are an important topic when it comes to data selection and preparation, but less important than they may be when developing descriptive statistical models. Nevertheless, confounding variables are critically important in applied machine learning. The evaluation of a machine learning model is an experiment with independent and dependent variables. As such, it is subject to confounding variables. What may be surprising is that you already know this and that the gold-standard practices in applied machine learning address this. ... Randomization is a simple tool in experimental design that allows the confounding variables to have their effect across a sample. It shifts the experiment from looking at an individual case to a collection of observations, where statistical tools are used to interpret the finding.Quote for the day:
"Leaders must be good listeners. It_s rule number one, and it_s the most powerful thing they can do to build trusted relationships." -- Lee Ellis
No comments:
Post a Comment