The end of AI scaling may not be nigh: Here’s what’s next

The concern is that scaling, which has driven advances for years, may not extend to the next generation of models. Reporting suggests that the development of frontier models like GPT-5, which push the current limits of AI, may face challenges due to diminishing performance gains during pre-training. The Information reported on these challenges at OpenAI and Bloomberg covered similar news at Google and Anthropic. This issue has led to concerns that these systems may be subject to the law of diminishing returns — where each added unit of input yields progressively smaller gains. As LLMs grow larger, the costs of getting high-quality training data and scaling infrastructure increase exponentially, reducing the returns on performance improvement in new models. Compounding this challenge is the limited availability of high-quality new data, as much of the accessible information has already been incorporated into existing training datasets. ... While scaling challenges dominate much of the current discourse around LLMs, recent studies suggest that current models are already capable of extraordinary results, raising a provocative question of whether more scaling even matters.
How to talk to your board about tech debt

Instead of opening the conversation about “code quality,” start talking about
business outcomes. Rather than discuss “legacy systems,” talk about “revenue
bottlenecks,” and replace “technical debt” with “innovation capacity.” When you
reframe the conversation this way, technical debt becomes a strategic business
issue that directly impacts the value metrics the board cares about most. ...
Focus on delivering immediate change in a self-funding way. Double down on
automation through AI. Take out costs and use those funds to compress your
transformation. ... Here’s where many CIOs stumble: presenting technical debt as
a problem that needs to be eliminated. Instead, show how leading companies
manage it strategically. Our research reveals that top performers allocate
around 15% of their IT budget to debt remediation. This balances debt reduction
and prioritizes future strategic innovations, which means committing to
continuous updates, upgrades, and management of end-user software, hardware, and
associated services. And it translates into an organization that’s stable and
innovative. We also found throwing too much money at tech debt can be
counterproductive. Our analysis found a distinct relationship between a
company’s digital core maturity and technical debt remediation.
Why You Need More Than A Chief Product Security Officer In The Age Of AI

Security by design means building digital systems and products that have
security as their foundation. When building software, a security-by-design
approach will involve a thorough risk analysis of the product, considering
potential weaknesses that could be exploited by attackers. This is known as
threat modeling, and it helps to expand on a desire for "secure" software to ask
"security of what?" and "secure from whom?" With these considerations and
recommendations, products are designed with the appropriate security controls
for the given industry and regulatory environment. To do this well, two teams
are needed—the developers and the security team. However, there’s a common
misconception that these teams are trained with the same knowledge and skill set
to work cohesively. ... As the AI landscape rapidly evolves, businesses must
proactively adapt to emerging regulatory requirements; this transformation
begins with a fundamental cultural shift. In an era where AI plays a pivotal
role in driving innovation, threat modeling should no longer be an afterthought
but a pillar of responsible AI leadership. While appointing a chief product
security officer is a smart first step, adopting a security-by-design mindset
starts by bringing together developer and security teams at the early software
design phase.
Enterprise Architecture in 2025 and beyond
The democratisation of AI presents both a challenge and an opportunity for
enterprise architects. While generative AI lowers the barrier to entry for
coding and data analysis, it also complicates the governance landscape.
Organisations must grapple with the reality that, when it comes to skills,
anyone can now leverage AI to generate code or analyse data without the
traditional oversight mechanisms that have historically been in place. ... The
acceleration of technological innovation presents both opportunities and
challenges for enterprise architects. With generative AI leading the charge,
organisations are compelled to innovate faster than ever before. Yet, this
rapid pace raises significant concerns around risk management and regulatory
compliance. Enterprise architects must navigate this tension by implementing
frameworks that allow for agile innovation while maintaining necessary
safeguards. ... In the evolving landscape of EA, the concept of a digital twin
of an organisation (DTO) is emerging as a transformative opportunity, and we
see this being realised in 2025. ... Outside of 'what-ifs', AI could enable
real-time decision-making within DTOs by continuously processing and analysing
live data streams. This is particularly valuable for dynamic industries like
retail or manufacturing, where market conditions, customer demands, or
operational circumstances can shift rapidly.
Clearing the Clouds Around the Shared Responsibility Model
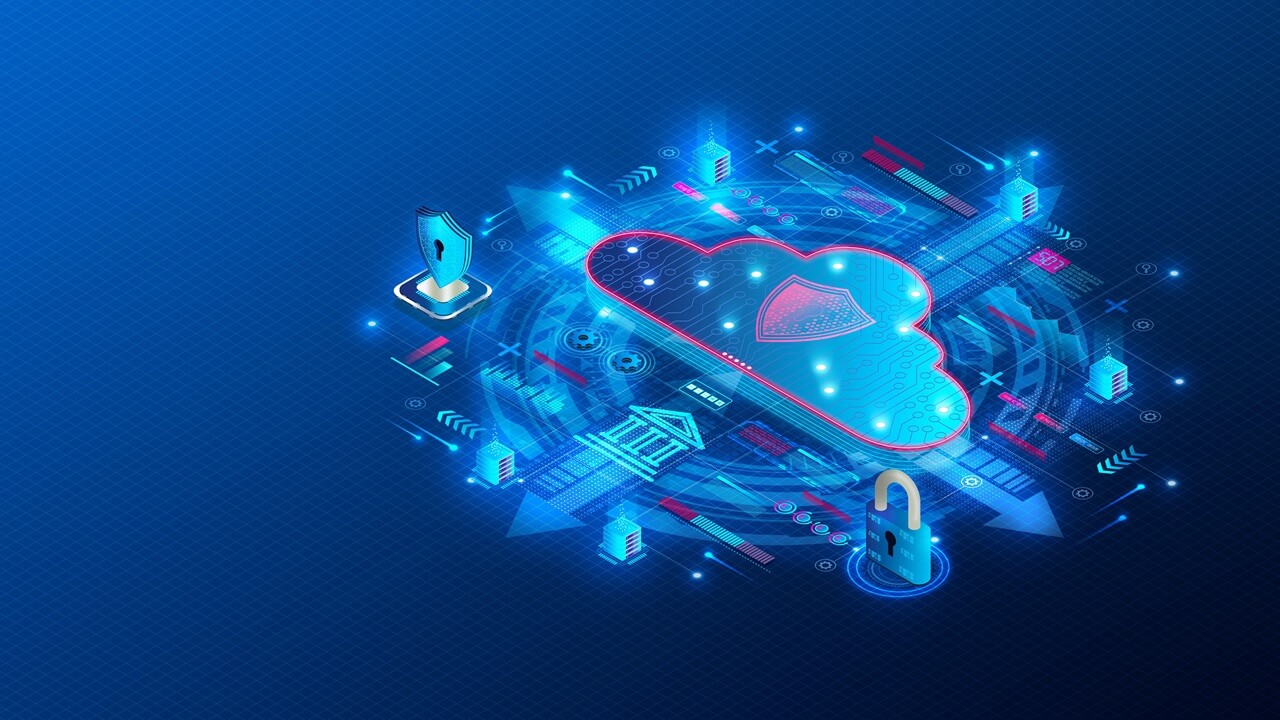
Enterprise leaders need to dig into the documentation for each cloud service
they use to understand their organizational responsibilities and to avoid
potential gaps and misunderstandings. While there is a definite division of
responsibilities, CSPs typically position themselves as partners eager to help
their customers uphold their part of cloud security. “The cloud service
providers are very interested and invested in their customers understanding the
model,” says Armknecht. ... Both parties, customer and provider, have their
security responsibilities, but misunderstandings can still arise. In the early
days of cloud, the incorrect assumption of automatic security was one of the
most common misconceptions enterprise leaders had around cloud. Cloud providers
secure the cloud, so any data plunked in the cloud was automatically safe,
right? Wrong. ... Even if customers fully understand their responsibilities,
they may make mistakes when trying to fulfill them. Misconfigurations are a
potential outcome for customers navigating cloud security. It is also possible
for misconfigurations to occur on the cloud provider side. “The CIA triad:
confidentiality, integrity, and availability. Essentially a misconfiguration or
a lack of configuration is going to put one of those things at risk,” says
Armknecht.
Data centers go nuclear for power-hungry AI workloads

AWS, Google, Meta, Microsoft, and Oracle are among the companies exploring
nuclear energy. “Nuclear power is a carbon-free, reliable energy source that
can complement variable renewable energy sources like wind and solar with firm
generation. Advanced nuclear reactors are considered safer and more efficient
than traditional nuclear reactors. They can also be built more quickly and in
a more modular fashion,” said Amanda Peterson Corio, global head of data
center energy at Google. ... “The NRC has, for the last few years, been
reviewing both preliminary information and full applications for small modular
reactors, including designs that cool the reactor fuel with inert gases,
molten salts, or liquid metals. Our reviews have generic schedules of 2 to 3
years, depending on the license or permit being sought,” said Scott Burnell,
public affairs officer at the NRC. ... Analysts agree that nuclear is an
essential part of a carbon-free, AI-burdened electric grid. “The attraction of
nuclear in a world where you’re trying to take the grid to carbon-free energy
is that it is really the only proven reliable source of carbon-free energy,
one that generates whenever I need it to generate, and I can guarantee that
capacity is there, except for the refuel or the maintenance periods,” Uptime
Institute’s Dietrich pointed out.
How Banking Leaders Can Enhance Risk and Compliance With AI
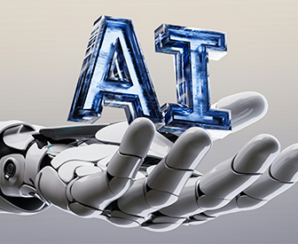
On one hand, AI can reduce risk exposure while making regulatory compliance
more efficient. AI can also enhance fraud and cybersecurity detection. On the
other hand, the complexity of AI models, coupled with concerns around data
privacy and algorithmic transparency, requires careful oversight to avoid
regulatory pitfalls and maintain customer or member trust. How the industry
moves forward will largely depend on pending regulations and the leaps AI
science may take, but for now, here is where the current state of affairs
lies. ... While AI holds immense potential, its adoption hinges on maintaining
account holder confidence. One of the most common concerns expressed by both
financial institutions and their account holders is around transparency in AI
decision-making. While 73% of financial institutions are convinced that AI can
significantly enhance digital account holder experiences, apprehensions about
AI’s impact on account holder trust are significant, with 54% expressing
concerns over potential negative effects. The concern seems valid, as less
than half of consumers feel comfortable with their financial data being
processed by AI, even if it gives them a better digital banking experience.
When Prompt Injections Attack: Bing and AI Vulnerabilities

Tricking a chatbot into behaving badly (by “injecting” a cleverly malicious
prompt into its input) turns out to be just the beginning. So what should you
do when a chatbot tries tricking you back? And are there lessons we can learn
— or even bigger issues ahead? ... While erroneous output is often called an
AI “hallucination,” Edwards has been credited with popularizing the alternate
term “confabulation.” It’s a term from psychology that describes the filling
of memory gaps with imaginings. Willison complains that both terms are still
derived from known-and-observed human behaviors. But then he acknowledges that
it’s probably already too late to stop the trend of projecting humanlike
characteristics onto AI. “That ship has sailed…” Is there also a hidden
advantage there too? “It turns out, thinking of AIs like human beings is a
really useful shortcut for all sorts of things about how you work with them…”
“You tell people, ‘Look, it’s gullible.’ You tell people it makes things up,
it can hallucinate all of those things. … I do think that the human analogies
are effective shortcuts for helping people understand how to use these things
and how they work.”
Refactoring AI code: The good, the bad, and the weird

Generative AI is no longer a novelty in the software development world: it’s
being increasingly used as an assistant (and sometimes a free agent) to write
code running in real-world production. But every developer knows that writing
new code from scratch is only a small part of their daily work. Much of a
developer’s time is spent maintaining an existing codebase and refactoring
code written by other hands. ... “AI-based code typically is syntactically
correct but often lacks the clarity or polish that comes from a human
developer’s understanding of best practices,” he says. “Developers often need
to clean up variable names, simplify logic, or restructure code for better
readability.” ... According to Gajjar, “AI tools are known to overengineer
solutions so that the code produced is bulkier than it really should be for
simple tasks. There are often extraneous steps that developers have to trim
off, or a simplified structure must be achieved for efficiency and
maintainability.” Nag adds that AI can “throw in error handling and edge cases
that aren’t always necessary. It’s like it’s trying to show off everything it
knows, even when a simpler solution would suffice.”
How Businesses Can Speed Up AI Adoption
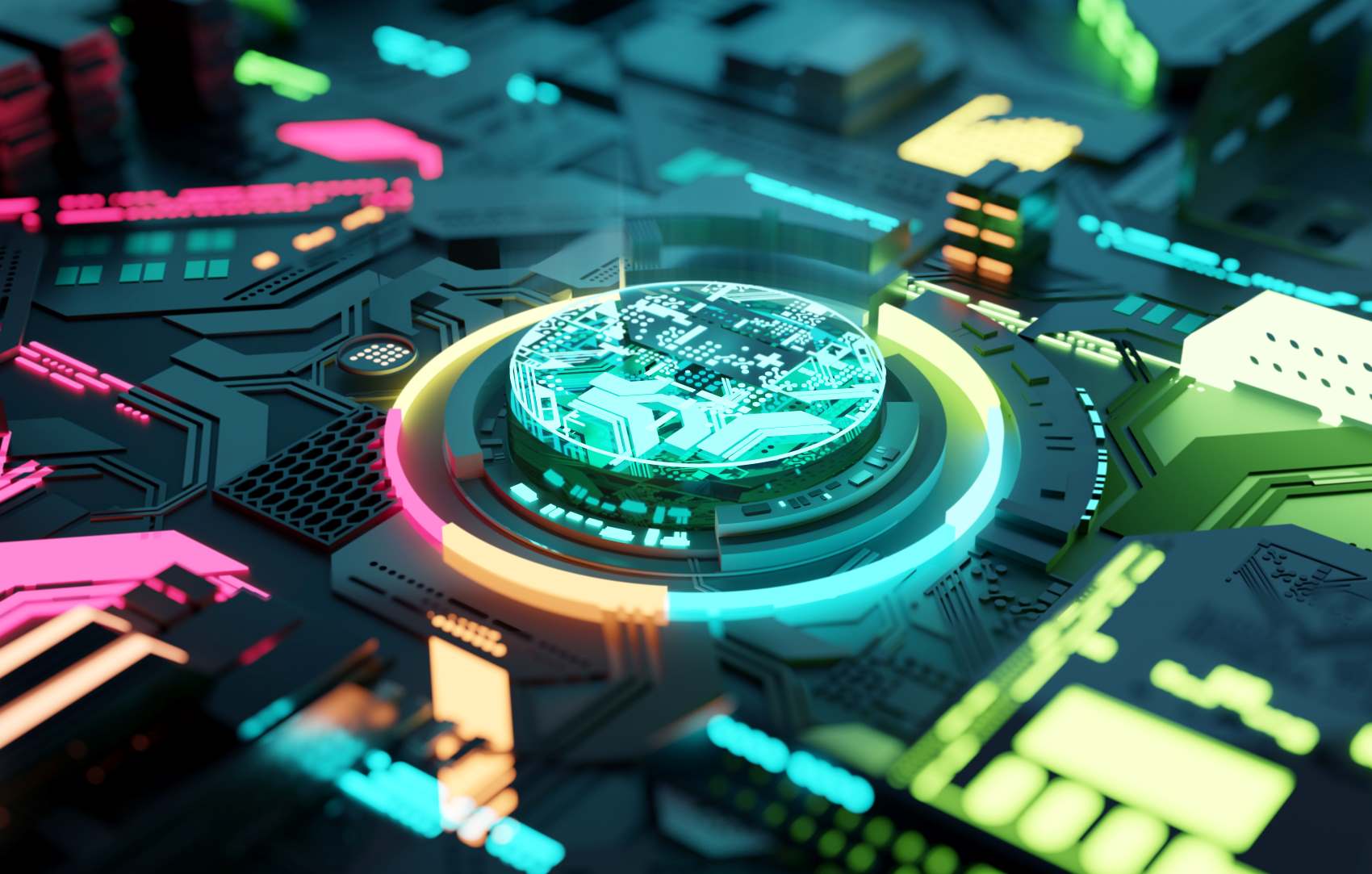
To ensure successful AI adoption, businesses should follow a structured approach
that focuses on key strategic steps. First, they should build and curate their
organisational data assets. A solid data foundation is crucial for effective AI
initiatives, enabling companies to draw meaningful insights that drive accurate
AI results and consumer interactions. Next, identifying applicable use cases
tailored to specific business needs is essential. This may include generative,
visual, or conversational AI applications, ensuring alignment with
organisational goals. When investing in AI capabilities, choosing off-the-shelf
solutions is advisable, unless there is a compelling business justification for
custom development. This allows companies to quickly implement new technologies
without accumulating technical debt. Finally, maintaining an active data
feedback loop is vital for AI effectiveness. Regularly updating data ensures AI
models produce accurate results and helps prevent issues associated with “stale”
data, which can hinder performance and limit insights. ... As external pressures
such as regulatory changes and shifting consumer expectations create a sense of
urgency and complexity, it’s critical that organisations are proactive in
overcoming internal obstacles.
Quote for the day:
“People are not lazy. They simply have important goals – that is, goals that
do not inspire them.” -- Tony Robbins
No comments:
Post a Comment