Will AI help doctors decide whether you live or die?

One of the things GPT-4 “was terrible at” compared to human doctors is causally
linked diagnoses, Rodman said. “There was a case where you had to recognize that
a patient had dermatomyositis, an autoimmune condition responding to cancer,
because of colon cancer. The physicians mostly recognized that the patient had
colon cancer, and it was causing dermatomyositis. GPT got really stuck,” he
said. IDC’s Shegewi points out that if AI models are not tuned rigorously and
with “proper guardrails” or safety mechanisms, the technology can provide
“plausible but incorrect information, leading to misinformation. “Clinicians may
also become de-skilled as over-reliance on the outputs of AI diminishes critical
thinking,” Shegewi said. “Large-scale deployments will likely raise issues
concerning patient data privacy and regulatory compliance. The risk for bias,
inherent in any AI model, is also huge and might harm underrepresented
populations.” Additionally, AI’s increasing use by healthcare insurance
companies doesn’t typically translate into what’s best for a patient. Doctors
who face an onslaught of AI-generated patient care denials from insurance
companies are fighting back — and they’re using the same technology to automate
their appeals.
The Rise Of ‘Quiet Hiring’: 5 Ways To Use Trend For A Career Advantage

Adaptability is key in quiet hiring. When I interviewed Ross Thornley,
Co-founder of AQai, an organization that provides adaptability training, he
said, "We’re entering a period of volatility where expanding adaptability
skills is essential." Whether it’s learning to manage budgets, mastering new
software, or brushing up on leadership skills, the more versatile you are, the
more indispensable you become. ... You might feel uncomfortable tooting your
own horn, but staying silent about your successes can hurt you in the long
run. Keep track of your achievements as you take on extra responsibilities.
Highlight the skills you’re building and the results you’re delivering. Then,
share them in conversations with your manager or during performance reviews.
By showcasing your value, you ensure your work doesn’t go unnoticed. ... When
holding onto status-quo ways, employees limit themselves from reaching heights
that might improve engagement. Without exploration, there’s a greater
potential to be misaligned with a job or responsibility that isn’t motivating.
Every new role—whether formal or not—is an opportunity to grow and explore.
Use this time to test out roles you might not have considered. See if you
enjoy the work or if it’s a stepping stone to something even better.
Creating a unified data, AI and infrastructure strategy to scale innovation ambitions
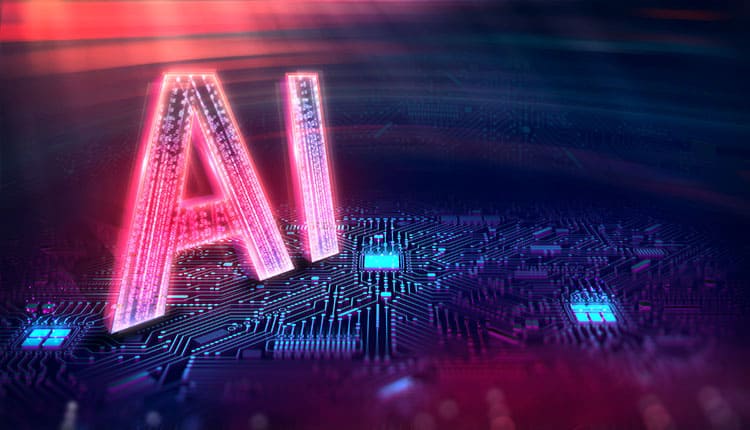
To effectively leverage data and AI, organisations must first shift their
mindset from merely collecting data to actively connecting the dots. This
involves identifying the core problem that needs to be addressed and focusing
on use cases that will yield maximum business impact, rather than isolating
data collection and AI model development. ... To enhance AI implementation,
organisations should shift from a use-case-driven approach to a
capability-driven strategy, focusing on building reusable AI capabilities such
as conversational AI and voice analytics for both internal and external
service desks. A company exploring numerous use cases can then group them into
distinct capabilities for greater efficiency. Establishing a centralised team
dedicated to data, AI and infrastructure is essential to create a robust
foundation and platform while allowing business units to develop their own
AI-powered applications on top, ensuring consistency across the
organisation. ... To succeed in scaling innovation and AI, organisations
must move from merely collecting data to actively connecting data, AI and
infrastructure. Today’s advancements in cloud and data management technologies
enable this integration, fostering collaboration and driving innovation at
scale.
AWS introduces S3 Tables, a new bucket type for data analytics
The new bucket type is S3 Table, for storing data in Apache Iceberg format.
Iceberg is an open table format (OTF) used for storing data for analytics, and
with richer features than Parquet alone. Parquet is the format used by Hadoop
and by many data processing frameworks. Parquet and Iceberg are already widely
used on S3, so why a new bucket type? Warfield said the popularity of Parquet
in S3 was the rationale for S3 Tables. "We actually serve about 15 million
requests per second to Parquet tables," he told us, but there is a maintenance
burden. Internally, he said, "the structure of them is a lot like git, a
ledger of changes, and the mutations get added as snapshots. Even with a
relatively low rate of updates into your OTF you can quickly end up with
hundreds of thousands of objects under your table." The consequence is poor
performance. "In the OTF world it was anticipated that this would happen, but
it was left to the customer to do the table maintenance tasks," Warfield said.
The Iceberg project includes code to expire snapshots and clean up metadata,
but it is still necessary "to go and schedule and run those Spark jobs."
Apache Spark is a SQL engine for large scale data. Parquet on S3 was "a
storage system on top of a storage system," said Warfield, making it
sub-optimal.
Innovation Is Fun, but Infrastructure Pays the Bills

Innovation and platform infrastructure are intertwined — each move affects the
other. Yet, many companies are stumbling because they’re too focused on
innovation. They’re churning out apps, features, and updates at breakneck
speed, all while standing on a wobbly foundation. It’s a classic case of
putting the cart before the horse, and it affects the intended impact of some
really great ideas. A strong platform infrastructure is your ticket to
scalability and flexibility. It lets you pivot quickly to meet new market
demands, integrate cutting-edge technologies, and expand your services without
tearing everything down and starting from scratch. Plus, it trims the fat off
your development and deployment times, letting you bring innovative ideas to
market faster. Sidestepping platform infrastructure is a recipe for disaster.
It can make your application sluggish, prone to crashes, and a sitting duck
for cyberattacks. This isn’t just a headache for users — it’s a surefire way
to tarnish your product’s reputation and negatively affect its success. Think
of it like building a mansion on a shaky foundation; it doesn’t matter how
grand it looks if it’s doomed to collapse.
Open-washing and the illusion of AI openness

Open-washing in AI refers to companies overstating their commitment to
openness while keeping critical components proprietary. This approach isn’t
new. We’ve seen cloud-washing, AI-washing, and now open-washing, all called
out here. Marketing firms want the concept of being “open” to put them in a
virtuous category of companies that save baby seals from oil spills. I don’t
knock them, but let’s not get too far over our skis, billion-dollar tech
companies. ... At the heart of open-washing is a distortion of the principles
of openness, transparency, and reusability. Transparency in AI would entail
publicly documenting how models are developed, trained, fine-tuned, and
deployed. This would include full access to the data sets, weights,
architectures, and decision-making processes involved in the models’
construction. Most AI companies fall short of this level of transparency. By
selectively releasing parts of their models—often stripped of key details—they
craft an illusion of openness. Reusability, another pillar of openness, is
much the same. Companies allow access to their models via APIs or lightweight
downloadable versions but prevent meaningful adaptation by tying usage to
proprietary ecosystems.
Microsoft hit with more litigation accusing it of predatory pricing

“All UK businesses and organizations that bought licenses for Windows Server
via Amazon’s AWS, Google Cloud Platform, and Alibaba Cloud may have been
overcharged and will be represented in this new ‘opt-out’ collective action,”
the law firm statement said. The accusations make sense when viewed from a
compliance/regulatory perspective. Although companies are allowed to give
volume discounts and to offer other pricing differences for different
customers, compliance issues kick in when the company controls an especially
high percentage of the market. ... “Put simply, Microsoft is punishing UK
businesses and organizations for using Google, Amazon, and Alibaba for cloud
computing by forcing them to pay more money for Windows Server. By doing so,
Microsoft is trying to force customers into using its cloud computing service,
Azure, and restricting competition in the sector,” Stasi said. “This lawsuit
aims to challenge Microsoft’s anti-competitive behavior, push them to reveal
exactly how much businesses in the UK have been illegally penalized, and
return the money to organizations that have been unfairly overcharged.”
Balancing tradition and innovation in the digital age
It’s easy to get carried away by the hype of cutting-edge technology. For me,
it’s about making sure that you always ask yourself if you’re solving an
actual business problem. That has to be front of mind, as opposed to being
solution- or tech-first. You also have to ask yourself if the business problem
requires nascent or proven tech? Once you figure that out, the tech side
answer is relatively straightforward. So, even with leveraging emerging tech,
you need to think congruently about your business model. ... Security is the
first thing I looked at. Even in my interview, I said it would be the first
thing I looked at, and it has been. Security and privacy are the basic
foundations of trust, and customer and community trust is what our business is
built on. So, my approach is to spend money to bring in deep expertise, which
I have, and empower them to go deep into our current state and be honest about
any gaps we might have. And to think about where we implement both tactical
and strategic ways to bridge those gaps. It’s also important to be clear about
the risk we hold and how long we want to hold it for and focus on building a
response plan. So, if and when an incident occurs, we can recover and respond
gracefully and have solid comms plans and playbooks in place.
Threat intelligence and why it matters for cybersecurity
Cyber threat intelligence – who needs it? The short answer is everyone. Cyber
threat intelligence is for anyone with a vested interest in the cybersecurity
infrastructure of an organization. Although CTI can be tailored to suit any
audience, in most cases, threat intelligence teams work closely with the
Security Operation Centre (SOC) that monitors and protects a business on a
daily basis. Research shows that CTI has proved beneficial to people at all
levels of government (national, regional or local), from security officers,
police chiefs and policymakers, to information technology specialists and law
enforcement officers. It also provides value to many other professionals, such
as IT managers, accountants and criminal analysts. ... The creation of cyber
threat intelligence is a circular process known as an “intelligence cycle”. In
this cycle, which consists of five stages, data collection is planned,
implemented and evaluated; the results are then analysed to produce
intelligence, which is later disseminated and re-evaluated against new
information and consumer feedback. The circularity of the process means that
gaps are identified in the intelligence delivered, initiating new collection
requirements and launching the intelligence cycle all over again.
Securing AI’s new frontier: Visibility, governance, and mitigating compliance risks
Securing and governing the use of data for AI/ML model training is perhaps the
most challenging and pressing issue in AI security. Using confidential or
protected information during the training or fine-tuning process comes with
the risk that data could be recoverable through model extraction techniques or
using common adversarial techniques (i.e., prompt injection, jailbreak).
Following data security and least-privilege access best practices is essential
for protecting data during development, but bespoke AI runtime threat
detection is response is required to avoid exfiltration of data via model
responses. ... Securing AI applications in production is equally important as
securing the underlying infrastructure and is a key component of maintaining a
secure data and AI lifecycle. This requires real-time monitoring of both
prompts and responses to identify, notify, and block security and safety
threats. A robust AI security solution prevents adversarial attacks like
prompt injection, masks sensitive data to prevent exfiltration via a model
response, and also addresses safety concerns such as bias, fairness, and
harmful content.
Quote for the day:
"Leading people is like cooking. Don_t stir too much; It annoys the
ingredients_and spoils the food" -- Rick Julian
No comments:
Post a Comment