How Conscious Unbossing Is Reshaping Leadership And Career Growth

Conscious unbossing presents both challenges and opportunities for
organizations. On the one hand, fewer employees pursuing traditional
leadership tracks can create gaps in decision-making, team development, and
operational consistency. On the other hand, organizations that embrace
unbossing as a cultural strategy can thrive. Novartis is a prime example,
fostering a culture of curiosity and empowerment that drives both engagement
and innovation. By breaking down rigid hierarchies, they’ve shown how
unbossed leadership can be a strategic advantage rather than a liability.
... Conscious unbossing is transforming how we think about leadership and
career progression. Organizations that adapt by redefining leadership roles,
offering flexible career pathways, and building cultures rooted in curiosity
and empathy will thrive. Companies like Novartis, Patagonia, and Microsoft
have proven that unbossed leadership isn’t a limitation—it’s an opportunity
to innovate and grow. By embracing this shift, businesses can create
resilient, dynamic teams and ensure leadership continuity. However, this
approach also comes with challenges that organizations must navigate to
ensure its success. One potential downside is the risk of role
ambiguity.
Why agentic AI and AGI are on the agenda for 2025
We’re ready to move beyond basic now, and what we’re seeing is an evolution
towards a digital co-worker – an agent. Agents are really those digital
coworkers, our friends, that are going to help us to do research, write a
text, and then publish it somewhere. So you set the goal – let’s say, run
research on some telco and networking predictions for next year – and an
agent would do the research and run it by you, and then push it to where it
needs to go to get reviewed, edited, and more. You would provide it with an
outcome, and it will choose the best path to get to that outcome. Right now,
Chatbots are really an enhanced search engine with creative flair. But
Agentic AI is the next stage of evolution, and will be used across
enterprises as early as next year. This will require increased network
bandwidth and deterministic connectivity, with compute closer to users – but
these essentials are already being rolled out as we speak, ensuring Agentic
AI is firmly on the agenda for enterprises in the new year. ... Amid
the AI rush, we’ve been focused on the outcomes rather than the
practicalities of how we’re accessing and storing the data being generated.
But concerns are emerging. Where does the data go? Does it disappear in a
big cloud? Concerns are obviously being raised in many sectors, particularly
in the medical space in which, medical records cannot leave state/national
borders.
Robust Error Detection to Enable Commercial Ready Quantum Computers from Quantum Circuits
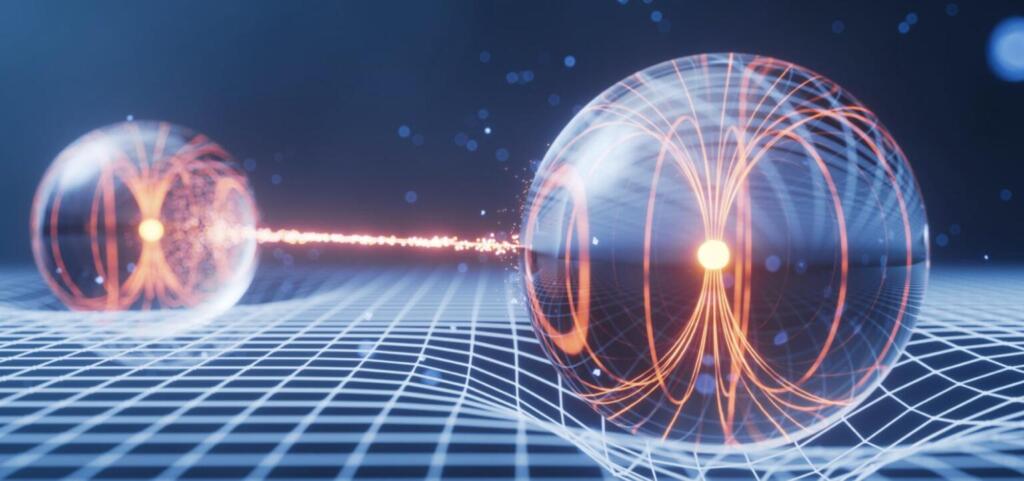
Quantum Circuits has the goal of first making components that are correct
and then scaling the systems. This is part of the larger goal of making
commercial ready quantum computers. What is meant by commercial ready
quantum computers ? This means you can bet your business or company on the
results of a quantum computer. Just as we rely today on servers and
computers than provide services via cloud computer systems. Being able trust
and rely on quantum computers means systems that are repeatable, predictable
and trusted. They have built an 8 qubit system and enterprise customers have
been using them. Customers have said that using error mitigation and error
detection can enable them to get far more utility from Quantum Circuits than
competing quantum computers. Error suppression and error mitigation are
common techniques and have intensive efforts by most quantum computer
companies and the entire Quantum computer community. Quantum Circuits’
error-detecting dual-rail qubits innovation allows errors to be detected and
corrected first to avoid disrupting performance at scale. This system will
enable a 10x reduction in resource requirements for scalable error
correction.
5 reasons why Google's Trillium could transform AI and cloud computing - and 2 obstacles

Trillium is designed to deliver exceptional performance and cost savings,
featuring advanced hardware technologies that set it apart from earlier TPU
generations and competitors. Key innovations include doubled High Bandwidth
Memory (HBM), which improves data transfer rates and reduces bottlenecks.
Additionally, as part of its TPU system architecture, it incorporates a
third-generation SparseCore that enhances computational efficiency by
directing resources to the most important data paths. There is also a
remarkable 4.7x increase in peak compute performance per chip, significantly
boosting processing power. These advancements enable Trillium to tackle
demanding AI tasks, providing a strong foundation for future developments
and applications in AI. ... Trillium is not just a powerful TPU; it is
part of a broader strategy that includes Gemini 2.0, an advanced AI model
designed for the "agentic era," and Deep Research, a tool to streamline the
management of complex machine learning queries. This ecosystem approach
ensures that Trillium remains relevant and can support the next generation
of AI innovations. By aligning Trillium with these advanced tools and
models, Google is future-proofing its AI infrastructure, making it adaptable
to emerging trends and technologies in the AI landscape.
How Industries Are Using AI Agents To Turn Data Into Decisions

In the past, this required hours of manual work to standardize the various
file formats — such as converting PDFs to spreadsheets — and reconcile
inconsistencies like differing terminologies for revenue or varying date
formats. Today, AI agents automate these tasks with human supervision,
adapting to schema changes dynamically and normalizing data as it comes in.
... While extracting insights is vital, the ultimate goal of any data
workflow is to drive action. Historically, this has been the weakest link in
the chain. Insights often remain in dashboards or reports, waiting for human
intervention to trigger action. By the time decisions are made, the window
of opportunity may already have closed. AI agents, with humans in the loop,
are expediting the entire cycle by bridging the gap between analysis and
execution. ... The advent of AI agents signals a new era in data management
— one where workflows are no longer constrained by team bandwidth or static
processes. By automating ETL, enabling real-time analysis and driving
autonomous actions, these agents, with the right guardrails and human
supervision, are creating dynamic systems that adapt, learn and improve over
time.
The Power of Stepping Back: How Rest Fuels Leadership and Growth
It's essential to fully step back from work sometimes, especially when
balancing the demands of running a business and being a parent. I find that
I'm most energised and focused in the mornings, so I like to use that time to
read, take notes, and reflect on different aspects of the business - whether
it's strategy, growth, or new ideas. It's my creative time to think deeply and
plan ahead. ... It's also important to carve out weekend days when I can fully
switch off. This time away from the business helps me come back refreshed and
with a clearer perspective. Even though I aim to disconnect, Lee (my husband
and co-founder) and I often find ourselves discussing business because it's
something we're both passionate about - strangely enough, those conversations
don't feel like work. ... Stepping back from the day-to-day grind gave me the
mental space to realise that while small tests have their place, they can
sometimes limit your potential by encouraging cautious, safe moves. By
contrast, thinking bigger and aiming for more ambitious goals has opened up a
new level of creativity and opportunity. This shift in mindset has been a
game-changer for us - it's unlocked several key growth areas, including new
product opportunities and ways to engage with customers.
Navigating the Future of Big Data for Business Success

Big data is no longer just a tool for competitive advantage – it has become
the backbone of innovation and operational efficiency across key industries,
driving billion-dollar transformations. ... The combination of artificial
intelligence and big data, especially through machine learning (ML), is
pushing the boundaries of what’s possible in data analysis. These technologies
automate complex decision-making processes and uncover patterns that humans
might miss. Google’s DeepMind AI, for instance, made a breakthrough in medical
research by using data to predict protein folding, which is already speeding
up drug discovery. ... Tech giants like Google and Facebook are increasing
their data science teams by 20% annually, underscoring the essential role
these experts play in unlocking actionable insights from vast datasets. This
growing demand reflects the importance of data-driven decision-making across
industries. ... AI and machine learning will also continue to revolutionize
big data, playing a critical role in data-driven decision-making across
industries. By 2025, AI is expected to generate $3.9 trillion in business
value, with organizations leveraging these technologies to automate complex
processes and extract valuable insights.
Five Steps for Creating Responsible, Reliable, and Trustworthy AI
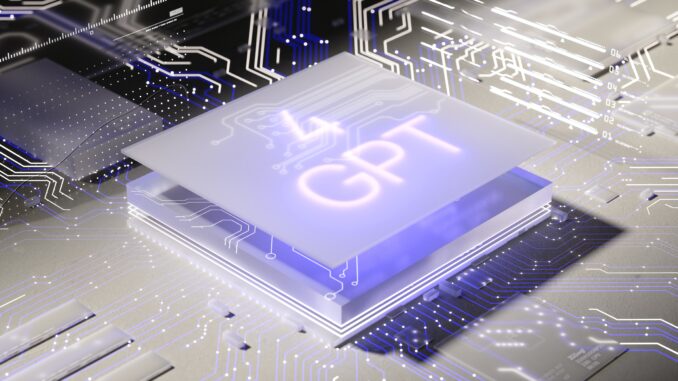
Model testing with human oversight is critically important. It allows data
scientists to ensure the models they’ve built function as intended and root
out any possible errors, anomalies, or biases. However, organizations should
not rely solely on the acumen of their data scientists. Enlisting the input of
business leaders who are close to the customers can help ensure that the
models appropriately address customers’ needs. Being involved in the testing
process also gives them a unique perspective that will allow them to explain
the process to customers and alleviate their concerns.Be transparent Many
organizations do not trust information from an opaque “black box.” They want
to know how a model is trained and the methods it uses to craft its responses.
Secrecy as to the model development and data computation processes will only
serve to engender further skepticism in the model’s output. ... Continuous
improvement might be the final step in creating trusted AI, but it’s just part
of an ongoing process. Organizations must continue to capture, cultivate, and
feed data into the model to keep it relevant. They must also consider customer
feedback and recommendations on ways to improve their models. These steps form
an essential foundation for trustworthy AI, but they’re not the only practices
organizations should follow.
With 'TPUXtract,' Attackers Can Steal Orgs' AI Models
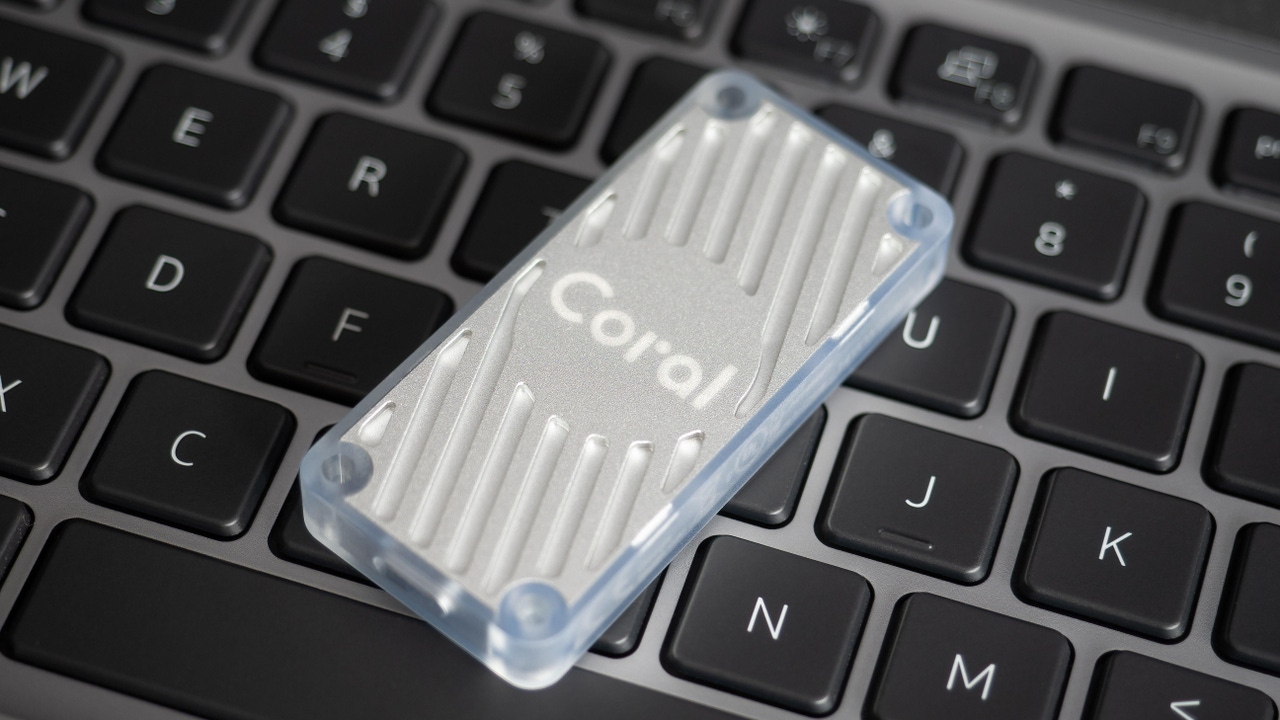
The NCSU researchers used a Riscure EM probe station with a motorized XYZ
table to scan the chip's surface, and a high sensitivity electromagnetic probe
for capturing its weak radio signals. A Picoscope 6000E oscilloscope recorded
the traces, Riscure's icWaves field-programmable gate array (FPGA) device
aligned them in real-time, and the icWaves transceiver used bandpass filters
and AM/FM demodulation to translate and filter out irrelevant signals. As
tricky and costly as it may be for an individual hacker, Kurian says, "It can
be a competing company who wants to do this, [and they could] in a matter of a
few days. For example, a competitor wants to develop [a copy of] ChatGPT
without doing all of the work. This is something that they can do to save a
lot of money." Intellectual property theft, though, is just one potential
reason anyone might want to steal an AI model. Malicious adversaries might
also benefit from observing the knobs and dials controlling a popular AI
model, so they can probe them for cybersecurity vulnerabilities. And for the
especially ambitious, the researchers also cited four studies that focused on
stealing regular neural network parameters.
Artificial Intelligence Looms Large at Black Hat Europe
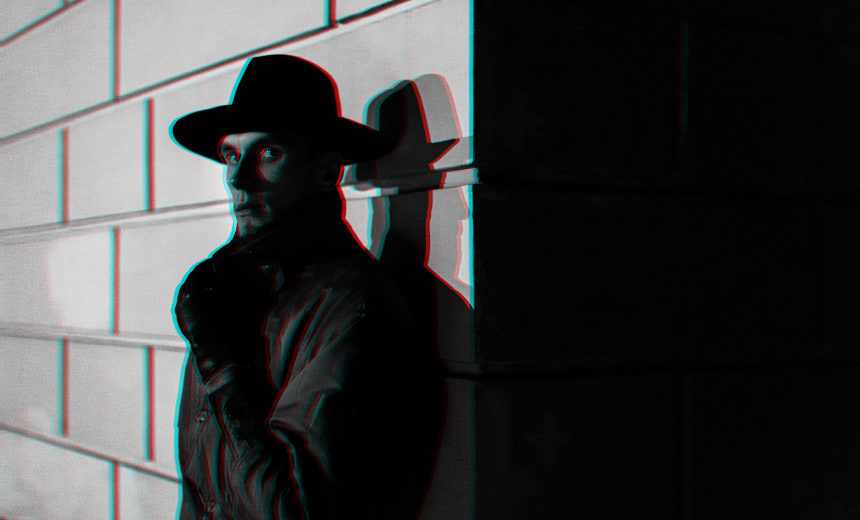
From a business standpoint, advances in AI are going to "make those
predictions faster and faster, cheaper and cheaper," he said. Accordingly, "if
I was in the business of security, I would try to make all of my problems
prediction problems," so they could get solved by using prediction engines.
What exactly these prediction problems might be remains an open question,
although Zanero said other good use cases include analyzing code, and
extracting information from unstructured text - for example, analyzing logs
for cyberthreat intelligence purposes. "So it accelerates your investigation,
but you still have to verify it," Moss said. "The verify part escapes most
students," Zanero said. "I say that from experience." One verification
challenge is AI often functions like a very complex, black box API, and people
have to adapt their prompt to get the proper output, he said. The problem:
that approach only works well when you know what the right answer should be,
and can thus validate what the machine learning model is doing. "The real
problematic areas in all machine learning - not just using LLMs - is what
happens if you do not know the answer, and you try to get the model to give
you knowledge that you didn't have before," Zanero said. "That's a deep area
of research work."
Quote for the day:
"The only person you are destined to become is the person you decide to
be." -- Ralph Waldo Emerson
No comments:
Post a Comment