Navigating the Future: Cloud Migration Journeys and Data Security

To meet the requirements of DORA and future regulations, business leaders must
adopt a proactive and reflexive approach to cybersecurity. Strong cyber
hygiene practices must be integrated throughout the business, ensuring
consistency in how data is handled, protected, and accessed. It is important
to note at this juncture that enhanced data security isn’t purely focused on
compliance. Modern IT researchers and business analysts have been studying
what differentiates the most innovative companies for decades and have
identified two key principles that help businesses achieve this: Unified
Control and Federated Protection. ... Advancements in data security
technologies are reshaping the cloud landscape, enabling faster and more
secure migrations. Privacy Enhancing Technologies (PETs) like dynamic data
masking (DDM), tokenisation, and format-preserving encryption help businesses
anonymise sensitive data, reducing breach risks while keeping cloud adoption
fast and flexible. However, as businesses will inevitably adopt multi-cloud
strategies to support their processes, they will require interoperable
security platforms that can seamlessly integrate across multiple cloud
environments.
Maximizing AI Payoff in Banking Will Demand Enterprise-Level Rewiring
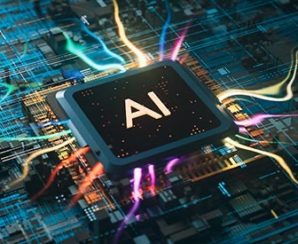
Beyond thinking in broad strokes of AI’s applicability in the bank, McKinsey
holds that an institution has to be ready to adopt multiple kinds of AI set up
in a way to work with each other. This includes analytical AI — the types of
AI that some banks have been using for years for credit and portfolio
analysis, for instance — and generative AI, in the forms of ChatGPT and
others, as well as “agentic AI.” In general, agentic AI uses AI that applies
other types of AI to perform analyses and solve problems as a “virtual
coworker.” It’s a developing facet of AI and, as described in the report, is
meant to manage multiple AI inputs, rather than having a bank lean on one
model. ... “You measure the outcomes you want to achieve and at the end of the
pilot you will typically come out with a very good understanding of how to
scale it,” Giovine says. Over six to 12 months after the pilot, “you can scale
it over a good chunk of the domain.” And here, the consultant says, is where
the bonus kicks in: Often a good deal of the work done to bring AI thinking to
one domain can be re-used. This applies to both the business thinking and
technology.
Synthetic data has its limits — why human-sourced data can help prevent AI model collapse

The more AI-generated content spreads online, the faster it will infiltrate
datasets and, subsequently, the models themselves. And it’s happening at an
accelerated rate, making it increasingly difficult for developers to filter
out anything that is not pure, human-created training data. The fact is, using
synthetic content in training can trigger a detrimental phenomenon known as
“model collapse” or “model autophagy disorder (MAD).” Model collapse is the
degenerative process in which AI systems progressively lose their grasp on the
true underlying data distribution they’re meant to model. This often occurs
when AI is trained recursively on content it generated, leading to a number of
issues:Loss of nuance: Models begin to forget outlier data or less-represented
information, crucial for a comprehensive understanding of any dataset. Reduced
diversity: There is a noticeable decrease in the diversity and quality of the
outputs produced by the models. Amplification of biases: Existing biases,
particularly against marginalized groups, may be exacerbated as the model
overlooks the nuanced data that could mitigate these biases. Generation of
nonsensical outputs: Over time, models may start producing outputs that are
completely unrelated or nonsensical.
The Macy’s accounting disaster: CIOs, this could happen to you

It wasn’t outright fraud or theft. But that’s merely because the employee
didn’t try to steal. But the same lax safeguards that allowed expense dollars
to be underreported could have just as easily allowed actual theft. “What will
happen when someone actually has motivation to commit fraud? They could have
just as easily kept the $150 million,” van Duyvendijk said. “They easily could
have committed mass fraud without this company knowing. (Macy’s) people are
not reviewing manual journals very carefully.” ... “It’s true that most ERPs
are not designed to catch erroneous accounting,” she said. “However, there are
software tools that allow CFOs and CAOs to create more robust controls around
accounting processes and to ensure the expenses get booked to the correct
P&L designation. Initiating, approving, recording transactions, and
reconciling balances are each steps that should be handled by a separate
member of the team. There are software tools that can assist with this
process, such as those that enable use of AI analytics to assess actual spend
and compare that spend to your reported expenses. Some such tools use AI to
look for overriding journal entries that reverse expense items and move those
expenses to a balance sheet account.”
Digital Nomads and Last-Minute Deals: How Online Data Enables Offline Adventures
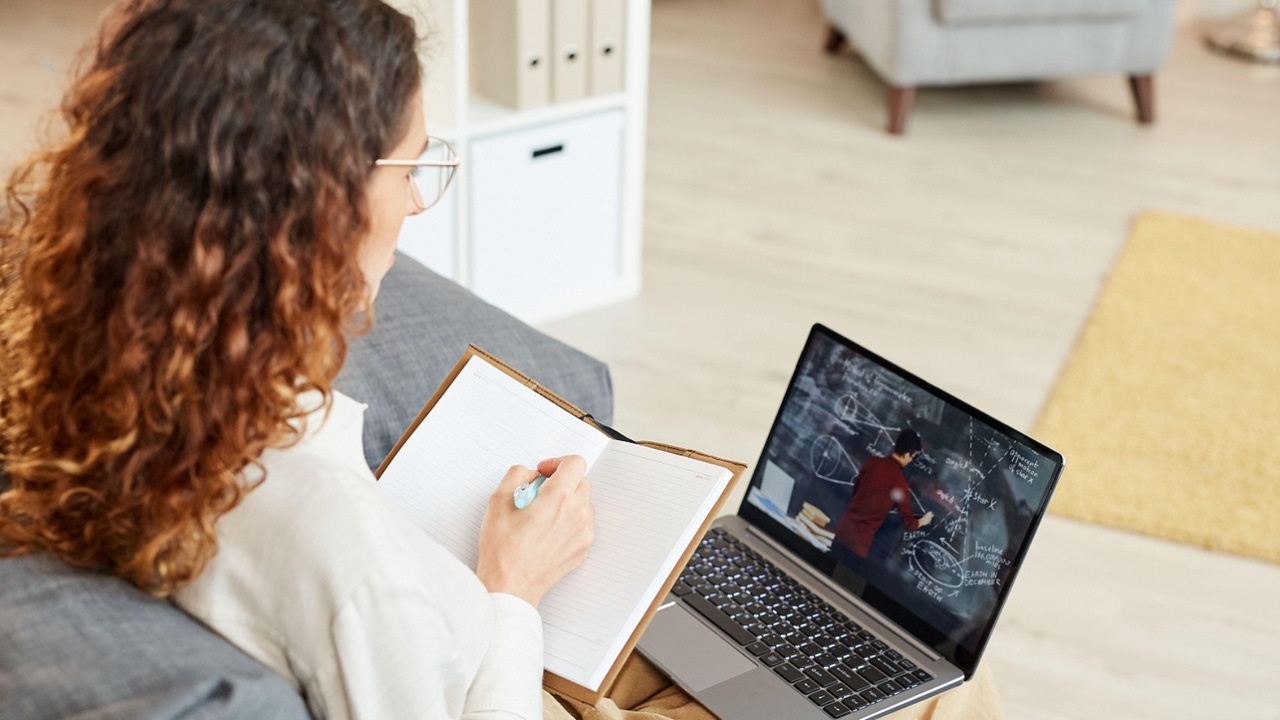
Along with remote work preference, the pandemic boosted another trend. Many
emerged from it more spontaneous, seeing how travel can be restricted so
suddenly and for so long. Even before, millennials were ready to embrace
impromptu travel, with half of them having planned last-minute vacations. For
digital nomads, last-minute deals for flights and hotels are even more
important as they need to adapt to changing situations quickly to strike a
work-life balance on the go. This opens opportunities for websites to offer
services that assist digital nomads in finding the best last-minute deals. ...
Many of the first successful startups by the nomads were teaching about the
nomadic lifestyle or connecting the nomads with each other. For example,
some websites use APIs to aggregate data about the suitability of cities for
remote work. Drawing data from various online sources in real time, such
platforms can constantly provide information relevant to traveling remote
workers. And the relevant information is very diverse. The aforementioned
travel and hospitality prices and deals alone generate volumes of data every
second. Then, there is information about security and internet stability in
various locations, which requires reliable and constantly updated reviews.
It’s not what you know, it’s how you know you know it
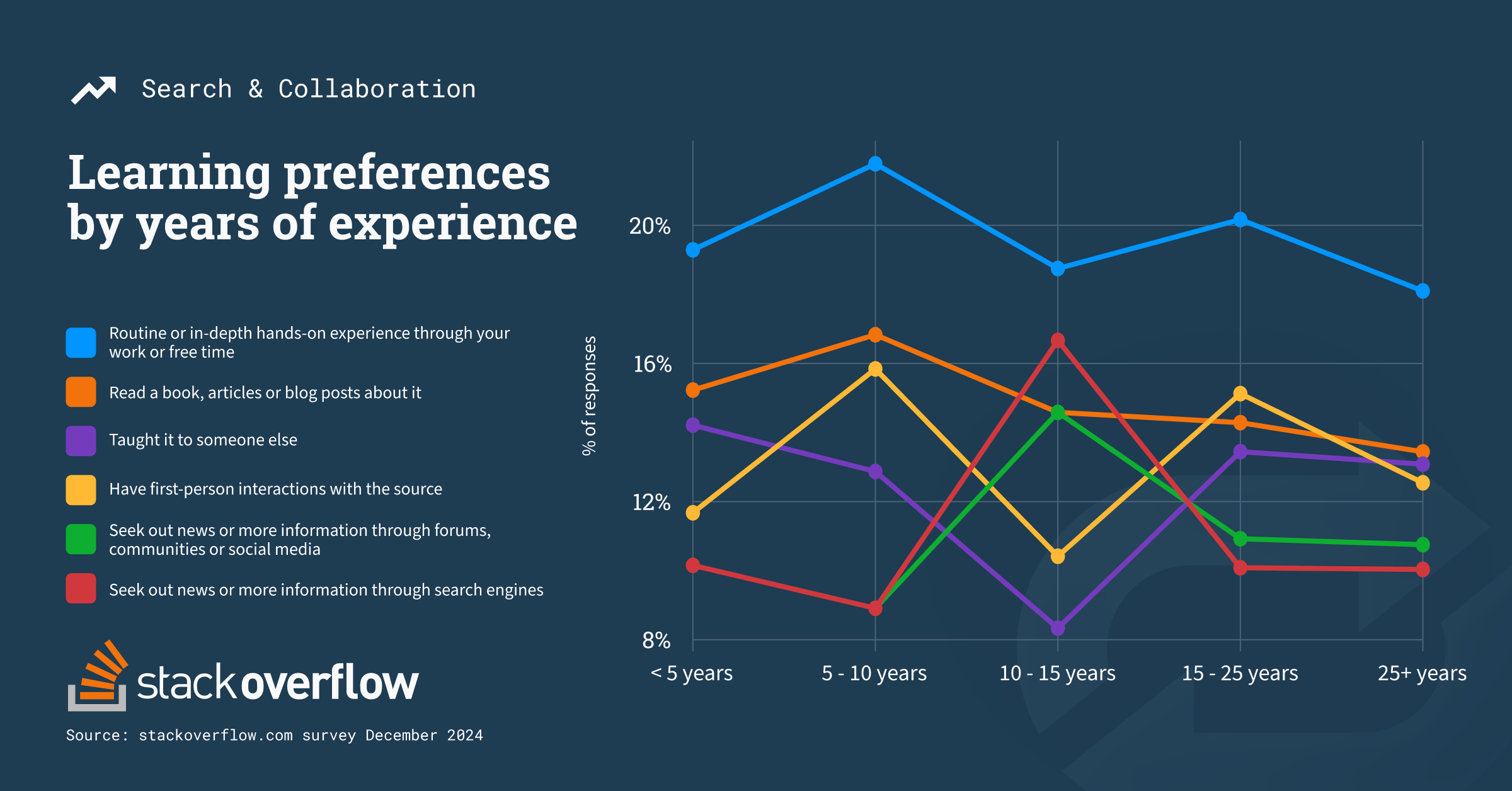
Developers and technologists have been learning to code using online media
such as blogs and videos increasingly in the last four years according to the
Stack Overflow Developer Survey–60% in 2021 increased to 82% in 2024. The
latest resource that developers could utilize for learning is generative AI
which is emerging as a key tool that offers real-time problem-solving
assistance, personalized coding tips, and innovative ways to enhance skill
development seamlessly integrated within daily workflows. There has been a lot
of excitement in the world of software development about AI’s potential to
increase the speed of learning and access to more knowledge. Speculation
abounds as to whether learning will be helped or hindered by AI advancement.
Our recent survey of over 700 developers and technologists reveals the process
of knowing things is just that—a process. New insights about how the Stack
Overflow community learns demonstrate that software professionals prefer to
gain and share knowledge through hands-on interactions. Their preferences for
sourcing and contributing to groups or individuals (or AI) provides color on
the evolving landscape of knowledge work.
What is data science? Transforming data into value

While closely related, data analytics is a component of data science, used to
understand what an organization’s data looks like. Data science takes the
output of analytics to solve problems. Data scientists say that investigating
something with data is simply analysis, so data science takes analysis a step
further to explain and solve problems. Another difference between data
analytics and data science is timescale. Data analytics describes the current
state of reality, whereas data science uses that data to predict and
understand the future. ... The goal of data science is to construct the means
to extract business-focused insights from data, and ultimately optimize
business processes or provide decision support. This requires an understanding
of how value and information flows in a business, and the ability to use that
understanding to identify business opportunities. While that may involve
one-off projects, data science teams more typically seek to identify key data
assets that can be turned into data pipelines that feed maintainable tools and
solutions. Examples include credit card fraud monitoring solutions used by
banks, or tools used to optimize the placement of wind turbines in wind
farms.
Tech Giants Retain Top Spots, Credit Goes to Self-Disruption
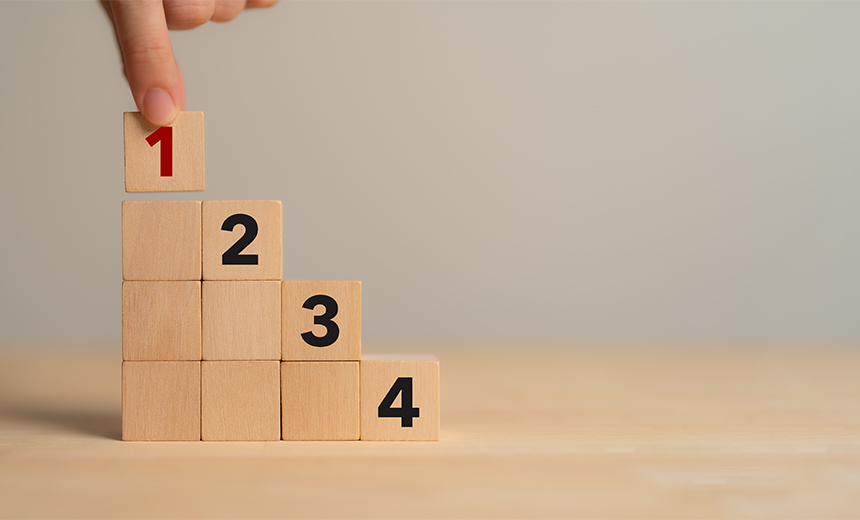
Companies today know they are not infallible in the face of evolving
technologies. They are willing to disrupt their tried and tested offerings to
fully capitalize on innovation. This ability of "dual transformation" -
sustaining as well as reinventing the core business - is a hallmark of
successful incumbents. It enables companies to optimize their existing
operations while investing in the future, ensuring they are not caught
flat-footed when the next wave of disruption hits. And because they have
capital, talent and resources, they are already ahead of newer players. ...
There is also a core cultural shift to encourage innovative thinking. Amazon
implemented its famous "two-pizza teams" approach, where small, autonomous
groups work on focused projects with minimal bureaucracy. Launched during the
dot-com boom, Amazon subsequently ventured into successful innovations,
including Prime, AWS and Alexa. Google's longstanding "20% time" policy, which
allows employees to dedicate a portion of their workweek to passion projects,
resulted in breakthrough products including AdSense and Google News. Drawing
from decades of experience, these organizations know the whole is greater than
the sum of its parts.
The Power of the Collective Purse: Open-Source AI Governance and the GovAI Coalition
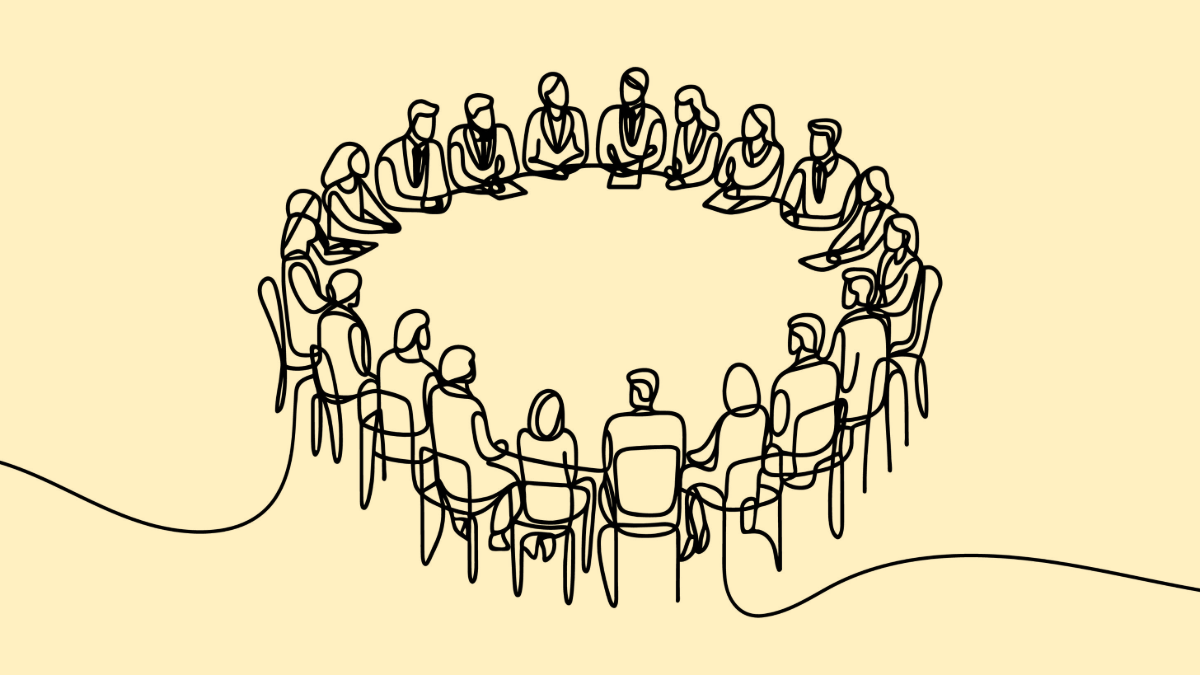
Collaboration and transparency often go hand in hand. One of the most
significant outcomes of the GovAI Coalition’s work is the development of
open-source resources that benefit not only coalition members but also vendors
and uninvolved governments. By pooling resources and expertise, the coalition
is creating a shared repository of guidelines, contracting language, and best
practices that any government entity can adapt to their specific needs. This
collaborative, open-source initiative greatly reduces the transaction costs
for government agencies, particularly those that are understaffed or
under-resourced. While the more expansive budgets and technological needs of
larger state and local governments sometimes lead to outsized roles in
Coalition standard-setting, this allows smaller local governments, which may
lack the capacity to develop comprehensive AI governance frameworks
independently, to draw on the Coalition’s collective institutional expertise.
This crowd-sourced knowledge ensures that even the smallest agencies can
implement robust AI governance policies without having to start from
scratch.
Redefining software excellence: Quality, testing, and observability in the age of GenAI
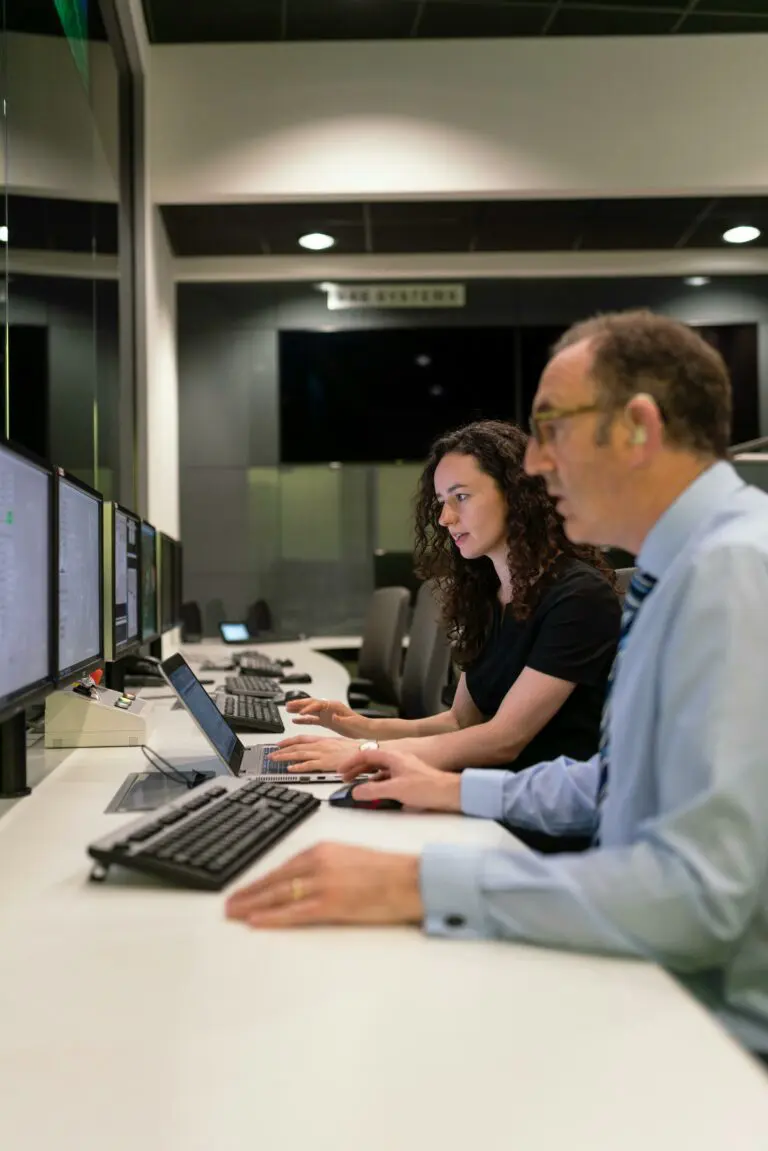
Traditional test automation has long relied on rigid, code-based frameworks,
which require extensive scripting to specify exactly how tests should run.
GenAI upends this paradigm by enabling intent-driven testing. Instead of
focusing on rigid, script-heavy frameworks, testers can define high-level
intents, like “Verify user authentication,” and let the AI dynamically
generate and execute corresponding tests. This approach reduces the
maintenance overhead of traditional frameworks, while aligning testing efforts
more closely with business goals and ensuring broader, more comprehensive test
coverage. ... QA and observability are no longer siloed functions. GenAI
creates a semantic feedback loop between these domains, fostering a deeper
integration like never before. Robust observability ensures the quality of
AI-driven tests, while intent-driven testing provides data and scenarios that
enhance observability insights and predictive capabilities. Together, these
disciplines form a unified approach to managing the growing complexity of
modern software systems. By embracing this symbiosis, teams not only simplify
workflows but raise the bar for software excellence, balancing the speed and
adaptability of GenAI with the accountability and rigor needed to deliver
trustworthy, high-performing applications.
Quote for the day:
"Success is not the key to happiness.
Happiness is the key to success. If you love what you are doing, you will be
successful." -- Albert Schweitzer
No comments:
Post a Comment