How AI will kill the smartphone

The great thing about AI is that it’s software-upgradable. When you buy an AI
phone, the phone gets better mainly through software updates, not hardware
updates. ... As we’re talking back and forth with AI agents, people will use
earbuds and, increasingly, AI glasses to interact with AI chatbots. The
glasses will use built-in cameras for photo and video multimodal AI input. As
glasses become the main interface, the user experience will likely improve
more with better glasses (not better phones), with improved light engines,
speakers, microphones, batteries, lenses, and antennas. With the inevitable
and inexorable miniaturization of everything, eventually a new class of AI
glasses will emerge that won’t need wireless tethering to a smartphone at all,
and will contain all the elements of a smartphone in the glasses themselves.
... Glasses will prove to be the winning device, because glasses can position
speakers within an inch of the ears, hands-free microphones within four inches
of the mouth and, the best part, screens directly in front of the eyes.
Glasses can be worn all day, every day, without anything physically in the ear
canal. In fact, roughly 4 billion people already wear glasses every day.
Million Dollar Lines of Code - An Engineering Perspective on Cloud Cost Optimization
Storage is still cheap. We should really still be thinking about storage as
being pretty cheap. Calling APIs costs money. It's always going to cost money.
In fact, you should accept that anything you do in the cloud costs money. It
might not be a lot; it might be a few pennies. It might be a few fractions of
pennies, but it costs money. It would be best to consider that before you call
an API. The cloud has given us practically infinite scale, however, I have not
yet found an infinite wallet. We have a system design constraint that no one
seems to be focusing on during design, development, and deployment. What's the
important takeaway from this? Should we now layer one more thing on top of
what it means to be a software developer in the cloud these days? I've been
thinking about this for a long time, but the idea of adding one more thing to
worry about sounds pretty painful. Do we want all of our engineers agonizing
over the cost of their code? Even in this new cloud world, the following quote
from Donald Knuth is as true as ever.
The five-stage journey organizations take to achieve AI maturity

We are far from seeing most organizations fully versed in and comfortable with
AI as part of their company strategy. However, Asana and Anthropic have
outlined five stages of AI maturity; a guide executives can use to gauge where
their company stands in implementing real transformative outcomes. Many
respondents say they’re in either the first or second stage. Only seven
percent claim they’ve achieved the highest stage. ... Asana and Anthropic
conclude that boosting comprehension is important, offering resources,
training programs and support structures for knowledge workers to improve
their education. Companies must also prioritize AI safety and reliability,
meaning that AI vendors should be selected with “complete, integrated data
models and invest in high-quality data pipelines and robust governance
practices.” AI responses must be interpretable to facilitate decision-making
and should always be controlled and directed by human operators. Other
elements of organizations in Stage 5 include embracing a human-centered
approach, developing strong comprehensive policies and principles to navigate
AI adoption responsibly, and being able to measure AI’s impact and value
Unauthorized AI is eating your company data, thanks to your employees

A major problem with shadow AI is that users don’t read the privacy policy or
terms of use before shoveling company data into unauthorized tools, she says.
“Where that data goes, how it’s being stored, and what it may be used for in
the future is still not very transparent,” she says. “What most everyday
business users don’t necessarily understand is that these open AI
technologies, the ones from a whole host of different companies that you can
use in your browser, actually feed themselves off of the data that they’re
ingesting.” ... Using AI, even officially licensed ones, means organizations
need to have good data management practices in place, Simberkoff adds. An
organization’s access controls need to limit employees from seeing sensitive
information not necessary for them to do their jobs, she says, and
longstanding security and privacy best practices still apply in the age of AI.
Rolling out an AI, with its constant ingestion of data, is a stress test of a
company’s security and privacy plans, she says. “This has become my mantra: AI
is either the best friend or the worst enemy of a security or privacy
officer,” she adds. “It really does drive home everything that has been a best
practice for 20 years.”
How a data exchange platform eases data integration
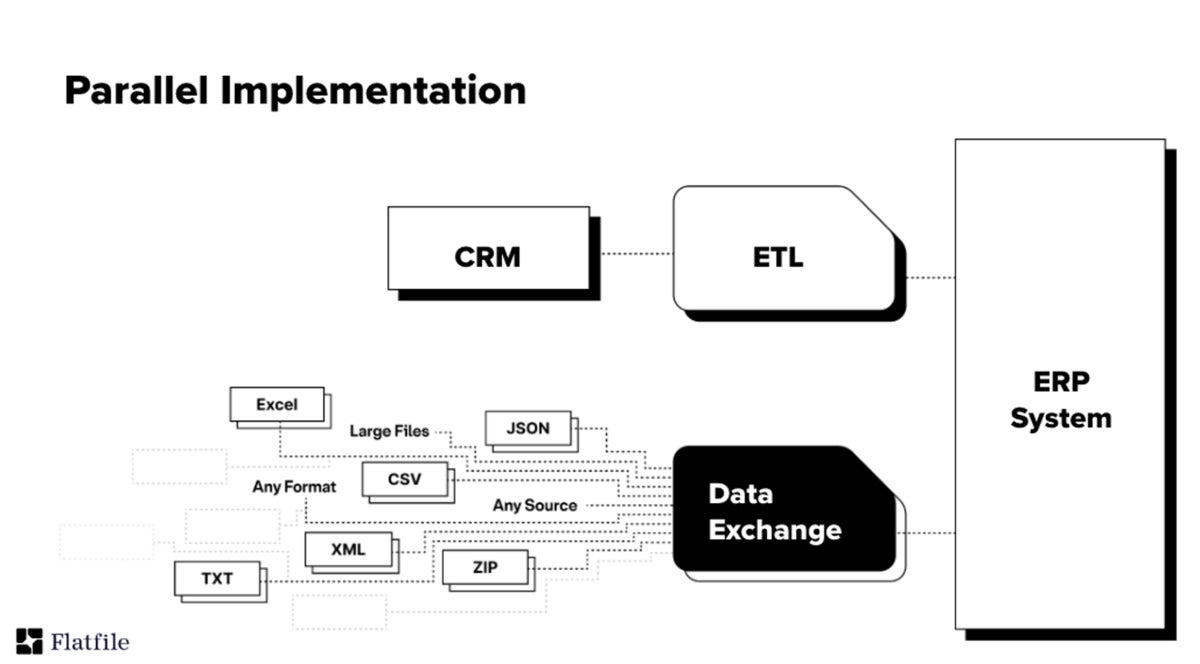
As our software-powered world becomes more and more data-driven, unlocking and
unblocking the coming decades of innovation hinges on data: how we collect it,
exchange it, consolidate it, and use it. In a way, the speed, ease, and
accuracy of data exchange has become the new Moore’s law. Safely and
efficiently importing a myriad of data file types from thousands or even
millions of different unmanaged external sources is a pervasive, growing
problem. ... Data exchange and import solutions are designed to work
seamlessly alongside traditional integration solutions. ETL tools integrate
structured systems and databases and manage the ongoing transfer and
synchronization of data records between these systems. Adding a solution for
data-file exchange next to an ETL tool enables teams to facilitate the
seamless import and exchange of variable unmanaged data files. The data
exchange and ETL systems can be implemented on separate, independent, and
parallel tracks, or so that the data-file exchange solution feeds the
restructured, cleaned, and validated data into the ETL tool for further
consolidation in downstream enterprise systems.
AI is used to detect threats by rapidly generating data that mimics realistic cyber threats
When we talk about AI, it’s essential to understand its fundamental
workings—it operates based on the data it’s fed. Hence, the data input is
crucial; it needs to be properly curated. Firstly, ensuring anonymisation is
key; live customer data should never be directly integrated into the model to
comply with regulatory standards. Secondly, regulatory compliance is
paramount. We must ensure that the data we feed into the framework adheres to
all relevant regulations. Lastly, many organisations grapple with outdated
legacy tech stacks. It’s essential to modernise and streamline these systems
to align with the requirements of contemporary AI technology. Also, mitigating
bias in AI is crucial. Since the data we use is created by humans, biases can
inadvertently seep into the algorithms. Addressing this issue requires careful
consideration and proactive measures to ensure fairness and impartiality. ...
It’s important for people to be highly aware of biases and misconceptions
surrounding AI. We need to be conscious of the potential biases in AI
systems.
Tackling Information Overload in the Age of AI
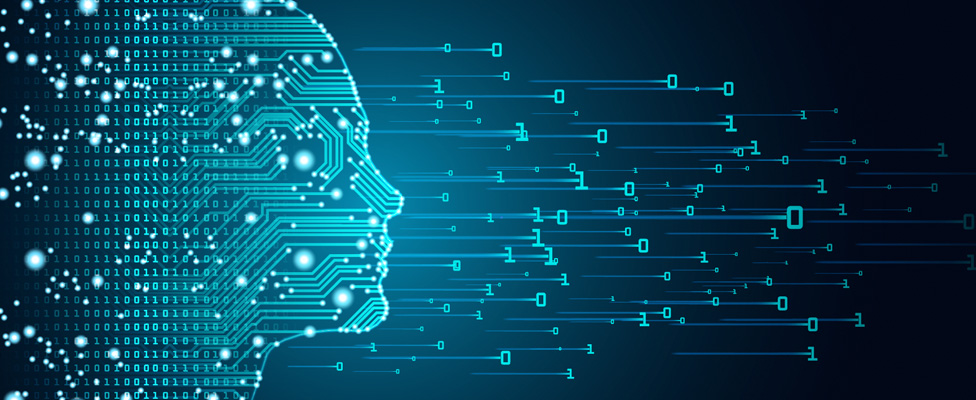
The reason this story is so universal is that the kind of information that
drives knowledge-intensive workflows is unstructured data, which has
stubbornly resisted the automation wave that has taken on so many other
enterprise workflows using software and software-as-a-service (SaaS). SaaS has
empowered teams with tools they can use to efficiently manage a wide variety
of workflows involving structured data. However, SaaS offerings have been
unable to take on the core “jobs to be done” in the knowledge-intensive
enterprise because they can’t read and understand unstructured data. They
aren’t capable of performing human-like services with autonomous
decision-making abilities. As a result, knowledge workers are still stuck
doing a lot of monotonous and undifferentiated data work. However, newly
available large language models (LLMs) and generative AI excel at processing
and extracting meaning from unstructured data. LLM-powered “AI agents” can
perform services such as reading and summarizing content and prioritizing work
and can automate multistage knowledge workflows autonomously.
CDOs Should Understand Business Strategy to Be Outcome-focused
To be outcome-focused, the CDO has to prioritize understanding the business or
corporate strategy, he says. In addition, one needs to comprehend the
organizational aspirations and how to deliver on key business outcomes, which
could include monetization of commercial opportunities, risk mitigation, cost
savings, or providing client value. Next, Thakur advises leaders to focus on
the foundational data and analytic capabilities to drive business outcomes.
There must be a well-organized data and analytic strategy to start with, a
good tech stack, an analytic environment, data management, and governance
principles. While delivering on some of the use cases may take time, it is
imperative to have quick wins along the way, says Thakur. He recommends CDOs
create reusable data products and assets while having an agile
operationalization process. Then, Thakur suggests data leaders create a solid
engagement model to ensure that the data analytics team is in sync with
business and product owners. He urges leaders to put an effective ideation and
opportunity management framework into action to capture business ideas and
prioritize use cases.
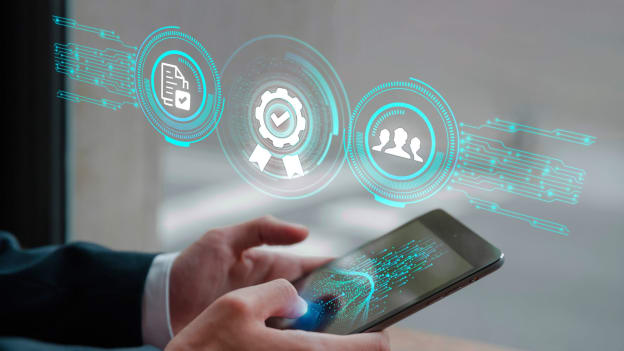
Besides the traditional functions of sales and finance, there is a growing
demand for tech-driven talent in the sector. It’s important to note that the
demand for technology expertise isn’t limited to software development but
encompasses different competencies, such as cybersecurity, UI/UX
development, AI/ML engineering, digital marketing and data analytics. This
is expected as there has been an increase in the use of AI and ML in the
BFSI landscape, most prominently in fraud detection, KYC verification, sales
and marketing processes. ... Now, new-age competencies such as digital
skills, data analysis, AI and cybersecurity are increasingly becoming part
of these programmes. To meet the growing demand for specialised skills and
roles, many BFSI organisations encourage employees with financial expertise
to develop digital skills that enable them to work more efficiently. ... At
the crossroads of significant industry-level transformations, employers
expect a variety of soft skills in addition to technical
competencies.
Cyber Resilience Act Bans Products with Known Vulnerabilities
In future, manufacturers will no longer be allowed to place smart products
with known security vulnerabilities on the EU market – if they do, they could
face severe penalties ... When it comes to cyber resilience, the legislation
of the Cyber Resilience Act makes it clear that customers – both residential
and commercial – have an effective right to secure software. However, the race
to be the first to discover vulnerabilities continues: organisations would be
well advised to implement both effective CVE detection and impact assessment
now to better scrutinise their own products and protect themselves against the
serious consequences of vulnerability scenarios. “The CRA requires all vendors
to perform mandatory testing, monitoring and documentation of the
cybersecurity of their products, including testing for unknown vulnerabilities
known as ‘zero days’,” said Jan Wendenburg, CEO of ONEKEY, a cybersecurity
company based in Duesseldorf, Germany. ... Many manufacturers and distributors
are not sufficiently aware of potential vulnerabilities in their own
products.
Quote for the day:
"Life always begins with one step
outside of your comfort zone." -- Shannon L. Alder
No comments:
Post a Comment