A virtuous cycle: how councils are using AI to manage healthier travel

With local lockdowns being a new threat, councils face fresh calls to gather
and understand social distancing requirements. This isn’t just in large towns
and cities; local authorities need to be able to assess and understand risk
across broader geographical areas to keep people safe. More small towns and
villages are already installing cameras and sensors (or upgrading their
current infrastructure) to capture data in their streets to identify places
where people struggle to social distance. The city of Oxford, too, has
implemented a large scale deployment of cycling specific sensors. Councils and
other local authorities are taking their responsibilities seriously. Aiding
all this is AI. Artificial intelligence can underpin a council’s strategy for
coping with the Active Travel boom. In practical terms, this means positioning
cameras at busy junctions, on popular footpaths, and around town and city
centres, then analysing what those cameras see. It’s not just a numbers game,
although knowing with confidence how many people are travelling in a certain
area on a given day will certainly be useful. AI can quickly identify where
social distancing is struggling to be adhered to due to road or path layout,
and spot dangerous behaviour such as undertaking or cyclists riding on
pavements.
Inclusion And Ethics In Artificial Intelligence
The computer science and Artificial Intelligence (AI) communities are starting to awaken to the profound ways that their algorithms will impact society and are now attempting to develop guidelines on ethics for our increasingly automated world. The systems we require for sustaining our lives increasingly rely upon algorithms to function. More things are becoming increasingly automated in ways that impact all of us. Yet, the people who are developing the automation, machine learning, and the data collection and analysis that currently drive much of this automation do not represent all of us and are not considering all our needs equally. However, not all ethics guidelines are developed equally — or ethically. Often, these efforts fail to recognize the cultural and social differences that underlie our everyday decision making and make general assumptions about both what a “human” and “ethical human behavior”. As part of this approach, the US federal government launched AI.gov to make it easier to access all of the governmental AI initiatives currently underway. The site is the best single resource from which to gain a better understanding of the US AI strategy.Our quantum internet breakthrough could help make hacking a thing of the past

Our current way of protecting online data is to encrypt it using mathematical
problems that are easy to solve if you have a digital “key” to unlock the
encryption but hard to solve without it. However, hard does not mean
impossible and, with enough time and computer power, today’s methods of
encryption can be broken. Quantum communication, on the other hand, creates
keys using individual particles of light (photons) , which – according to the
principles of quantum physics – are impossible to make an exact copy of. Any
attempt to copy these keys will unavoidably cause errors that can be detected.
This means a hacker, no matter how clever or powerful they are or what kind of
supercomputer they possess, cannot replicate a quantum key or read the message
it encrypts. This concept has already been demonstrated in satellites and over
fibre-optic cables, and used to send secure messages between different
countries. So why are we not already using in everyday life? The problem is
that it requires expensive, specialised technology that means it’s not
currently scalable. Previous quantum communication techniques were like
pairs of children’s walkie talkies.
Two Tools Every Data Scientist Should Use For Their Next ML Project
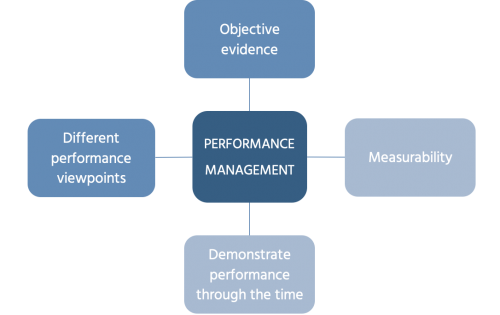
One of the key value propositions of data management is to deliver data to
internal and external stakeholders in required quality for different purposes.
Data management sets up data value chains that turn raw data into meaningful
information. Different data management capabilities should enable data value
chains. The core data management capabilities taken into the “Orange” model
are data modeling, information systems architecture, data quality, and data
governance. In Figure 1, they are marked orange. These capabilities are
performed by data management professionals. Other capabilities that belong to
other domains like IT, security, and other business support functions. To
implement a data management capability, a company should establish a formal
data management function. The data management function will become operational
by implementing four key components that enable data management capability
such as processes, roles, tools, and data ... To make the evidence objective,
it should be measurable. This is the second criterion. For example, you can
prove your progress by demonstrating the number of data quality issues
resolved within a specified period. You should also compare the planned and
achieved resolved issues.
The fourth generation of AI is here, and it’s called ‘Artificial Intuition’
The fourth generation of AI is ‘artificial intuition,’ which enables computers
to identify threats and opportunities without being told what to look for,
just as human intuition allows us to make decisions without specifically being
instructed on how to do so. It’s similar to a seasoned detective who can enter
a crime scene and know right away that something doesn’t seem right, or an
experienced investor who can spot a coming trend before anybody else. The
concept of artificial intuition is one that, just five years ago, was
considered impossible. But now companies like Google, Amazon and IBM are
working to develop solutions, and a few companies have already managed to
operationalize it. So, how does artificial intuition accurately analyze
unknown data without any historical context to point it in the right
direction? The answer lies within the data itself. Once presented with a
current dataset, the complex algorithms of artificial intuition are able to
identify any correlations or anomalies between data points. Of course,
this doesn’t happen automatically. First, instead of building a quantitative
model to process the data, artificial intuition applies a qualitative model.
Vulnerability Management: Is In-Sourcing or Outsourcing Right for You?

While size is a factor, both small and large companies can benefit from
leveraging the expertise of a partner. Small companies can get
enterprise-level services for a fraction of the cost of supporting full time
employees; large companies can relieve their IT departments of time-consuming
tasks and still save money. This allows for both to focus on their core
competencies – the outsource provider brings platform and process expertise to
the table to help guide program maturity while handling the grind of scanning,
analysis and reporting. This frees up the customer organization to focus on
operating their business and handling strategic technology initiatives. A
qualified third-party company that specializes in VM already has the certified
security professionals on board who are not only up to speed with the latest
threats, but always use the most effective detection tools and are in the loop
of important new information. If you answered in the affirmative to
outsourcing VM, you’ll want to know how to select a company that is truly
going to help you shore up the weaknesses in your defenses. First, you want
one that has years of experience protecting businesses and offers dedicated
support 24/7.
How to drive business value through balanced development automation
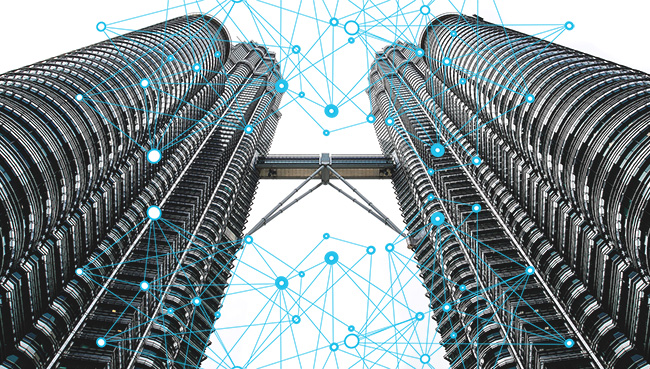
Operationally, challenges stem from misalignment in understanding who the end
customer really is. Companies often design products and services for
themselves and not for the end customer. Once an organization focuses on the
end user and how they are going to use that product and service, the shift in
thinking occurs. Now it’s about looking at what activities need to be done to
provide value to that end customer. Thinking this way, there will be features,
functions, and processes never done before. In the words of Stephen Covey,
“Keep the main thing the main thing”. What is the main thing? The customer.
What features and functionality do you need for each of them from a value
perspective? And you need to add governance to that. Effective governance
ensures delivery of a quality product or service that meets your objectives
without monetary or punitive pain. The end customer benefits from that product
or service having effective and efficient governance. That said, heavy
governance is also waste. There has to be a tension and a flow or a balance
between Hierarchical Governance and Self Governance where the role of every
person in the organization is clearly aligned in their understanding of value
contributed to the end customer.
Microservices Governance and API Management
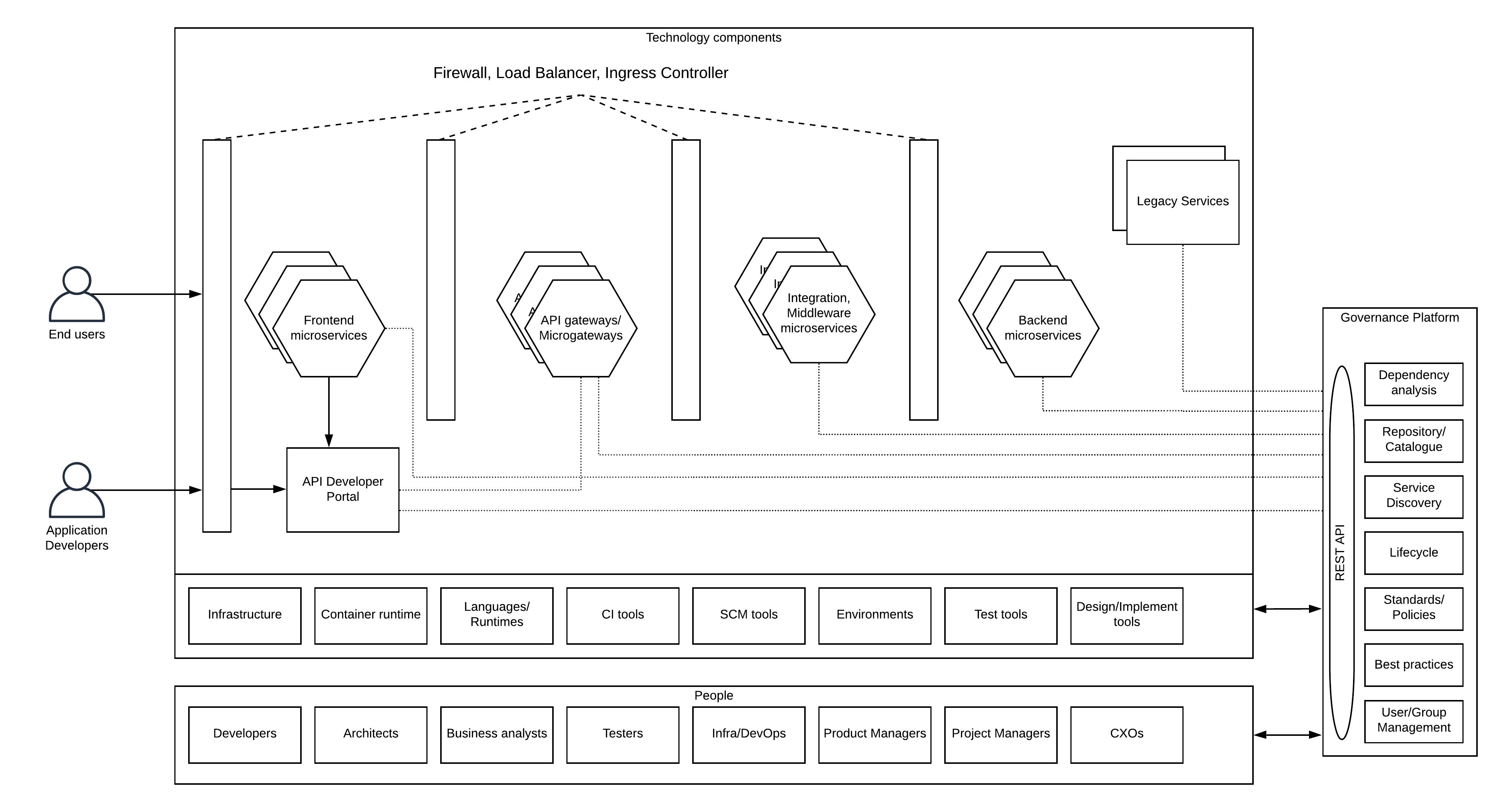
Different microservices teams can have their own lifecycle definitions and
different user roles to manage the lifecycle state transfer. That allows teams
to work autonomously. At the same time, WSO2 digital asset governance solution
allows these teams to create custom lifecycles and attach them to the services
that they implement. As part of that, there can be roles that verify the
overall governance across multiple teams by making sure that everyone follows
the industry best practices that are accepted by the business. As an example,
If the industry best practice is to use Open API Specification for API
definitions, every microservices team needs to adhere to that standard since
it is technology-neutral. At the same time, teams should have the autonomy to
select the programming language and the libraries used in their development.
Another key aspect of design-time governance is the reusable aspect. Given
that microservices teams are stemmed from ideas, there can be situations where
certain services that are required to retrieve data for this new microservices
implementation is already available via a service developed by another team.
Why Observability Is The Next Big Thing In Security
Cloud-native infrastructures and security observability are purposefully
designed to remove the security speed bumps that slow innovation down, and
instead, leverage a security guardrails approach that supports even faster
software integration and delivery. Developers may then focus on serving the
customer when they have tailored observability available—driven by automated
security feedback cycles—so teams can quickly learn from mistakes and rapidly
deliver value and innovation to customers. Optimizing customer experiences on
the fly, for example, is just one cloud-native advantage made possible by
event-driven architectures (EDAs). DevOps teams are now smartly requiring
embedded security context across the development life cycle in order to
understand what is going on and to help automate security of their
cloud-delivered applications. Any migration into application programming
interface (API) and event-driven architectures like cloud-native environments
can enjoy the benefits paid forward from preexisting, automated, observable
security deployed across your application development life cycle.
Why some artificial intelligence is smart until it's dumb

While practical uses get the most attention, machine learning also offers
advantages for basic scientific research. In high-energy particle accelerators,
such as the Large Hadron Collider near Geneva, protons smashing together produce
complex streams of debris containing other subatomic particles (such as the
famous Higgs boson, discovered at the LHC in 2012). With bunches containing
billions of protons colliding millions of times per second, physicists must
wisely choose which events are worth studying. It’s kind of like deciding which
molecules to swallow while drinking from a firehose. Machine learning can help
distinguish important events from background noise. Other machine algorithms can
help identify particles produced in the collision debris. “Deep learning has
already influenced data analysis at the LHC and sparked a new wave of
collaboration between the machine learning and particle physics communities,”
physicist Dan Guest and colleagues wrote in the 2018 Annual Review of Nuclear
and Particle Science. Machine learning methods have been applied to data
processing not only in particle physics but also in cosmology, quantum computing
and other realms of fundamental physics, quantum physicist Giuseppe Carleo and
colleagues point out in another recent review.
Quote for the day:
"You do not lead by hitting people over the head. That's assault, not leadership." - Dwight D. Eisenhower
No comments:
Post a Comment