Does An Analytics Head Require A Doctoral Degree?

Obviously, researchers in business are not expected to publish papers or guide
students as their academic counterparts do. They are looked up to analyze
complex business problems methodically as a scientist does. They are expected
to make suitable approximations and define some simple parts in the complex
whole and attack them using known repeatable, robust principles and
techniques. ... Let us say, a large IT services company wants to fill
leadership roles in the data science consulting practice. This person should
have enough technical depth and the ability to identify the business gaps,
communicate with the clients and most importantly build solutions that provide
measurable business value (interestingly, this last skill is never considered
a core competency in any traditional PhD in AI or other Masters and Bachelors
courses). Let us say, an IT product company decides to smarten its application
and wants leadership that can take them to the market quickly and profitably.
The leaders should have the skill to define the product, design the
technicalities, and lead the data science and DevOps teams compassionately and
efficiently for rapid design and development. Hence, A leader in data science
is not necessarily a technical expert who worked in the company long enough or
a business leader who is a taskmaster!
Ripple20 Malware Highlights Industrial Security Challenges
Since availability is critical to ICS systems, and since the systems
themselves can be fragile and quirky, these are generally the responsibility
of operational technology (OT) teams. The information technology (IT) team
usually manages the corporate network. OT employees are familiar with process
technology and the systems they manage, but they do not generally know a great
deal about information security, which can lead to insecure deployments. One
fairly common situation for manufacturers is a divide, sometimes adversarial,
between the IT and OT staff within a company. OT employees do not want
the IT staff to tamper with their systems out of fear of downtime that can
cost the company. From what we have seen, these relationships often resemble
red team versus blue team attitudes at many organizations. The blue team can
resent the efforts of the red team because those efforts create more work for
the blue team and can be considered a criticism of their work. OT employees
also often don't want to consult with their IT counterparts when making
arrangements such as remote access, leading to situations such as RDP on
control networks commonly being exposed to the public Internet.
India can soon be the tech garage of the world

The government has a crucial role to play in positioning India as the Tech
Garage of the World. It should act as a catalyst, and bring together the
synergies of the private sector with the aim of innovating for India and the
world. It has the potential to provide an enabling environment and a
favourable regulatory ecosystem for the development of technology products
and provide the size and scale necessary for their rollout. The product
development should ideally be undertaken through private entrepreneurship,
with the government acting as a facilitator. The key principles of product
design should incorporate transparency, security and ease of access. The
products must have open architecture, should be portable to any hosting
environment and should be available in official and regional languages. The
irrevocable shift brought about by covid-19 presents opportunities to
develop new technology platforms. In this process, data integrity,
authenticity and privacy should be embedded into the design of a product. A
balance needs to be struck between regulation and product design through a
dynamic collaboration between the government and technology entrepreneurs.
The State of Chatbots: Pandemic Edition
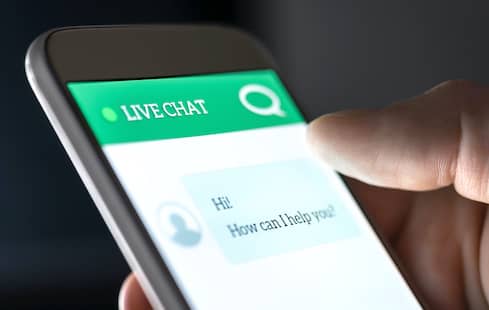
Generally speaking, there are two types of chatbots right now. The first
kind is the more primitive kind that is based on simple question and answer
rules. This kind is the easiest to deploy quickly, in response to some
catastrophic event, like, for instance, a pandemic. It has a scripted set of
answers. The problem with this kind of chatbot is that it is very limited,
and it can't be enhanced or expanded. It's a one-trick chatbot. "The
deterministic-rules based approach chatbots are easy to stand up quickly,"
Ian Jacobs, a principal analyst at Forrester Research, told InformationWeek.
That means there was a huge number of these deployed during the pandemic.
"There was an increase in call volume, and you were doing anything you could
to get answers to customers without hiring another thousand call center
agents," he said. These bots were doing very simple things, but "We are
getting to the point where the value that brands are getting out of those
very simple bots has already been achieved." One example of this type of bot
was deployed by a credit union in the northwestern United States in April
when stimulus checks were on the way, Jacobs said. This organization stood
up a simple bot designed to answer basic questions that people were asking
about the checks.
Digital Transformation Success Elusive For Financial Institutions
When financial institution executives were asked about the importance of
alternative digital transformation strategies, improving the overall
customer experience was considered to be of high or very high importance
by 88% of organizations. The importance of improving the customer
experience was followed closely by the need to improve the use of data, AI
and advanced analytics (76% rated high or very high). Illustrating the
perceived broad scope of digital transformation initiatives at most
financial institutions, the majority of the other possible digital
transformation strategies were each rated almost identically by financial
institution executives in the Digital Banking Report research. Innovation
agility, improving marketing and sales, improved efficiency, improved risk
management and reducing costs were each rated high or very high by roughly
six in ten executives. It is a bit concerning that the need to change the
existing business model and transforming legacy core systems were
considered the least important strategies despite research that indicates
these strategies are of significant importance for transformation success.
Organizations must rethink traditional IT strategy to succeed in the new normal
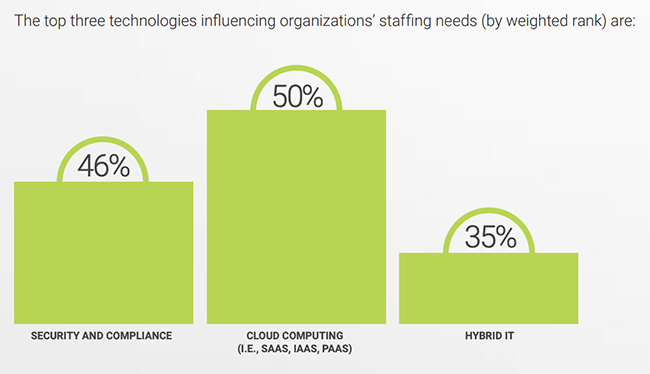
This newfound self-confidence, combined with IT pros’ achievements during
this time, will completely transform how IT is viewed by the business in
the future. IT may earn a more prominent voice in the C-suite, as 40% of
surveyed IT pros believe they will now be involved in more business-level
meetings. Likewise, IT’s role will be up-leveled due to the vast
upskilling 26% of IT pros underwent during this experience. With 31%
admitting there’s a need to rethink internal processes to better
accommodate the rapid change of pace required post-COVID, it’s highly
likely a focus on IT pros’ upskilling will continue into the future. “As
always, with new responsibilities comes the need for new skills. While
almost half of survey respondents felt they received the training required
to adapt to changing IT requirements, nearly one-third experienced the
opposite, and are at risk of being left behind as IT teams continue to
grapple with how best to support the new normal,” said Johnson. IT pros
said they’ve gained an increased sense of confidence in their expanded
roles, responsibilities, and ability to adapt to unexpected change in the
future, despite contending with more challenging working conditions over
the course of the pandemic.
Why Linux still needs a flagship distribution

Now, imagine a single distribution has been chosen, from the hundreds of
currently available distributions, to represent Linux to hardware
manufacturers, vendors, and software companies. That one Linux
distribution would be used by hardware manufacturers and software
companies to create computers and software guaranteed to run on
Linux. That distribution would have only one desktop environment, one
package manager, one init system, and the current stable version of the
Linux kernel. Users could also download this Linux distribution and use it
at will, but the primary purpose of "Flagship Linux" would be to make
things easier for manufacturers and developers. Set aside your
affinity for the Linux distribution you use and ponder this for a moment:
Would you rather argue over which distribution is the best, or would you
rather see Linux enjoy massive growth on the desktop and laptop arenas?
We've already seen a number of manufacturers start the rollout of
preinstalled Linux laptops. Lenovo, Dell, HP are all joining in on the
fun, but the process hasn't been easy. As you can see, those manufacturers
are, for the most part, all winnowing down the selection of Linux
distributions available.
Federated Machine Learning for Loan Risk Prediction
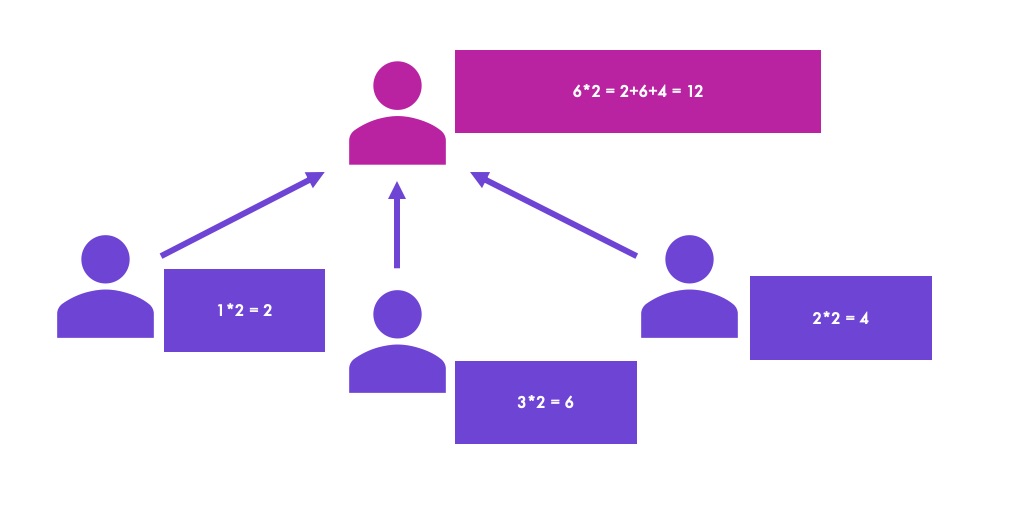
A model is only as strong as the data it’s provided, but what happens when
data isn’t readily accessible or contains personally identifying
information? In this case, can data owners and data scientists work
together to create models on privatized data? Federated learning shows
that it is indeed possible to pursue advanced models while still keeping
data in the hands of data owners. This new technology is readily
applicable to financial services, as banks have extremely sensitive
information ranging from transaction history to demographic information
for customers. In general, it’s very risky to give data to a third party
to perform analytical tasks. However, through federated learning, the data
can be kept in the hands of financial institutions and the intellectual
property of data scientists can also be preserved. In this article, we
will demystify the technology of federated learning and touch upon one of
the many use cases in finance: loan risk prediction. Federated
Learning, in short, is a method to train machine learning (ML) models
securely via decentralization. That is, instead of aggregating all the
data necessary to train a model, the model is instead sent to each
individual data owner.
How to Protect Chatbots from Machine Learning Attacks
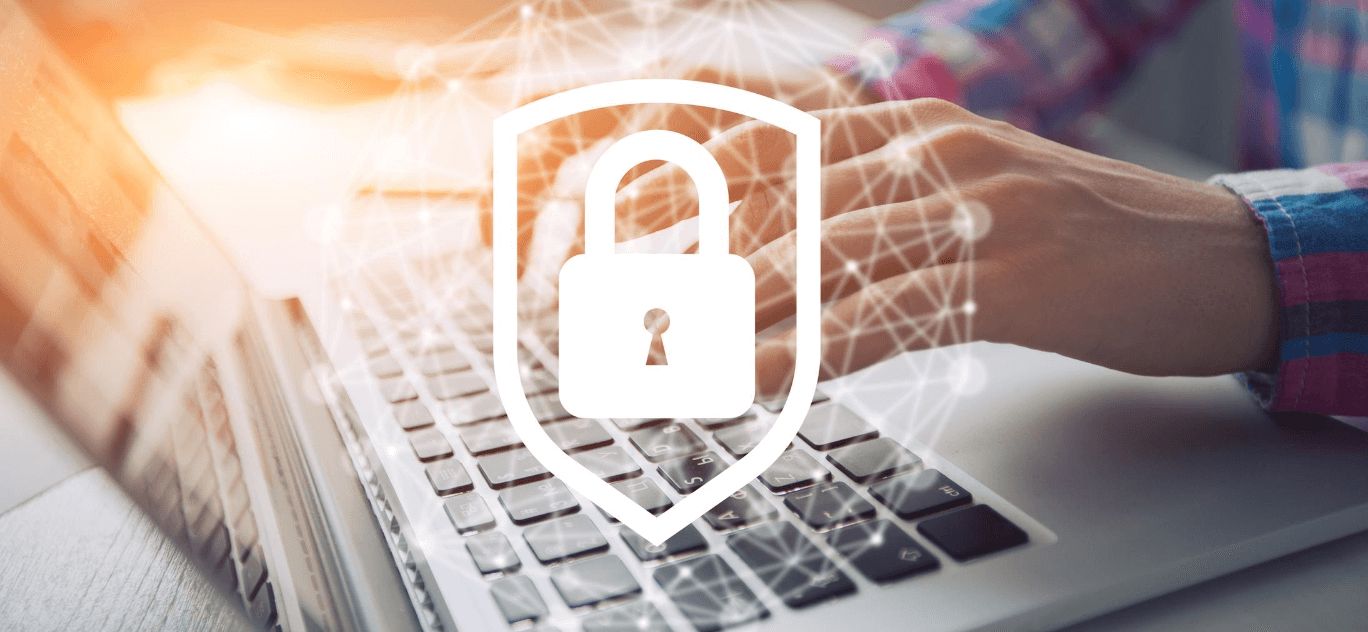
Chatbots are particularly vulnerable to machine learning attacks due to
their constant user interactions, which are often completely unsupervised.
We spoke to Scanta to get an understanding of the most common cyber attacks
that chatbots face. Scanta CTO Anil Kaushik tells us that one of the most
common attacks they see are data poisoning attacks through adversarial
inputs. Data poisoning is a machine learning attack in which hackers
contaminate the training data of a machine learning model. They do this by
injecting adversarial inputs, which are purposefully altered data samples
meant to trick the system into producing false outputs. Systems that are
continuously trained on user-inputted data, like customer service chatbots,
are especially vulnerable to these kinds of attacks. Most modern chatbots
operate autonomously and answer customer inquiries without human
intervention. Often, the conversations between chatbot and user are never
monitored unless the query is escalated to a human staff member. This lack
of supervision makes chatbots a prime target for hackers to exploit. To
help companies protect their chatbots and virtual assistants, Scanta is
continuously improving their ML security system, VA Shield.
The Expanding Role of Metadata Management, Data Quality, and Data Governance
After the data has been accurately defined, it is important to put in place
procedures to assure the accuracy of the data. Imposing controls on the wrong
data does no good at all. Which raises the question: How good is your data
quality? Estimates show that, on average, data quality is an overarching
industry problem. According to data quality expert Thomas C. Redman, payroll
record changes have a 1% error rate; billing records have a 2% to 7% error
rate, and; the error rate for credit records: as high as 30%. But what can a
DBA do about poor quality data? Data quality is a business responsibility, but
the DBA can help by instating technology controls. By building constraints
into the database, overall data quality can be improved. This include defining
Referential Integrity into the database. Additional constraints should be
defined in the database as appropriate to control uniqueness, as well as data
value ranges using check constraints and triggers. Another technology tactic
that can be deployed to improve data quality is data profiling. Data profiling
is the process of examining the existing data in the database and collecting
statistics and other information about that data.
Quote for the day:
"Concentrate all your thoughts upon the work in hand. The Sun's rays do not burn until brought to a focus." -- A.G. Bell
No comments:
Post a Comment