TIN coalition calls for industry action against cyber fraud
The vision for overcoming social engineering challenges is to reduce the opportunities to establish false trust and to ensure that all remaining threats are well publicised and understood. The vision also requires organisations to interact with customers and staff in a way that reinforces security and to ensure that the security of interactions with individuals becomes less dependent on public information. To address operating in silos, the vision is to ensure that cyber fraud is understood across functions within and between organisations, to ensure that organisations are recognised for sharing useful information, not punished for suffering an attack, and to ensure that business and law enforcement collaborate effectively to tackle cyber fraud. And to reduce the gap between cyber security and anti-fraud operations, the vision is to ensure that the response to cyber attacks minimises the broader impact of data loss on society, that fraud teams in business and law enforcement are fully engaged in tackling cyber attacks as a precursor to fraud, that enforcement is globalised to tackle all forms of cyber fraud
Big Data Is Dead. Long Live Big Data AI.
“The value of the data analytics market can’t be ignored. The Looker and Tableau acquisitions demonstrate that even the biggest tech players are snapping up data analytics companies with big price tags, clearly demonstrating the value these companies have in the larger cloud ecosystem. And in terms of what this means for the evolution of AI, we’ve reached a point where we have more than enough anonymized data to train the system, and now it’s a matter of honing how we use the AI to extract the maximum value from data”—Amir Orad, CEO, Sisense “The Google Cloud/Looker and Salesforce/Tableau acquisitions are a direct reaction to the rate at which analytics workloads have been shifting to the cloud over the past few years. The state of AI is a reflection of this shift as machine learning, AI and analytics have become the primary growth opportunities for the cloud today. Yet, it's this same growth that is causing barrier to success as AI project overwhelming face the same problem -- data quality”—Adam Wilson, CEO, Trifacta
What can you do with the Microsoft Graph?
Working with the APIs can be tricky; it can be hard to construct the right query, especially if you're looking for more complex graph queries. Microsoft offers tools to help build and test queries, as well as SDKs that can simplify adding Graph support to your apps. One, the web-based Graph Explorer, allows you to try out queries without logging in to an Office 365 account. It provides sample queries that show how to extract specific information from the service, with a library of different queries to get started. You can only use GET queries against sample data; POST requires your account details and your data. Once you're ready to start working with live data, you can log in with a Microsoft account, and start using your Microsoft 365 tenant. The list of query categories is long, covering working with users, with mail and calendar, as well as files and apps. The Graph Explorer doesn't only show production queries, it supports beta APIs, so you can experiment before adding them in your code. Queries can be cut-and-pasted from the Explorer, and you can see any request headers or bodies that need to be constructed and delivered with the REST HTTP query.
Offensive Security launches OffSec Flex, a new cybersecurity training program
Organizations can now use OffSec Flex to purchase blocks of Offensive Security’s industry-leading practical, hands-on training, certification and virtual lab offerings, allowing them to proactively increase and enhance the level of cybersecurity talent available within their organizations. With Offensive Security’s hands-on courses, labs and exams readily available, organizations are able to offer educational opportunities to new hires and non-security team members alike, improving their security posture and equipping their employees with the adversarial mindset necessary to protect modern enterprises from today’s threats. “Cybersecurity training is not just for security professionals anymore,” said Kerry Ancheta, VP of Worldwide Sales, Offensive Security. “Increasingly we see organizations recommend pentest training courses for their software development or application security teams in order to improve their understanding for how their systems and applications are attacked.
Calculating The Cost of Software Quality in Your Organization
Basically, the costs of software quality (COSQ) are those costs incurred through both meeting and not meeting the customer’s quality expectations. In other words, there are costs associated with defects, but producing a defect-free product or service has a cost as well. Calculating these costs serves the purpose of identifying just how much the organization spends to meet the customer’s expectations, and how much it spends (or loses) when it does not. Knowing these values allows management and team members across the company to take action in ensuring high quality at a lower cost. While analyzing the COSQ at an organization may lead to the revelation of uncomfortable truths about the state of quality management at the company, the process is important for eliminating waste associated with poor quality. This often requires a mindset and culture shift from viewing software quality defects as individual failures to seeing them as opportunities to improve as a collective team.
Machine learning has been used to automatically translate long-lost languages

It’s not hard to imagine that recent advances in machine translation might help. In just a few years, the study of linguistics has been revolutionized by the availability of huge annotated databases, and techniques for getting machines to learn from them. Consequently, machine translation from one language to another has become routine. And although it isn’t perfect, these methods have provided an entirely new way to think about language. Enter Jiaming Luo and Regina Barzilay from MIT and Yuan Cao from Google’s AI lab in Mountain View, California. This team has developed a machine-learning system capable of deciphering lost languages, and they’ve demonstrated it by having it decipher Linear B—the first time this has been done automatically. The approach they used was very different from the standard machine translation techniques. First some background. The big idea behind machine translation is the understanding that words are related to each other in similar ways, regardless of the language involved.
The Agile Manifesto: A Software Architect's Perspective
Specifications with an architectural impact (in the form of new user stories) should be tracked by the architect and assessed in a pragmatic approach by the whole development team, including experienced developers, test engineers, and devops. Bad habits from the past, when the architect created on paper the full blown technical design for the team, do not fit within modern agile environments. There are multiple flaws with this model, which I also faced in my daily basic work. First and most important, the architect might be wrong. This happened to me after I created a detailed upfront technical design and presented it to development team during Sprint refinements. I got questions related to cases I did not think about or I failed to take into account. In most of the cases, it turned out the initial design was either incomplete or impractical, and required extra work. Big upfront design limits the creativity and autonomy of the team members, since they must follow a recipe which is already granted. From a psychological standpoint, even the author might become biased and more reluctant to change it afterwards, trying to prove it is correct rather than to admit its flaws.
Essential tips for scaling quality AI data labeling

Data scientists are using labeled data and natural language processing (NLP) to automate legal contract review and predict patients who are at higher risk of chronic illness. The success of these systems depends on skilled humans in the loop, who label and structure the data for machine learning (ML). High-quality data yields better model performance. When data labeling is low quality, an ML model will struggle to learn. According to a report by analyst firm Cognilytica, about 80 percent of AI project time is spent on aggregating, cleaning, labeling, and augmenting data to be used in ML models. Just 20 percent of AI project time is spent on algorithm development, model training and tuning, and ML operationalization. These tasks are at the heart of AI development and require strategic thinking, along with a more advanced set of engineering or computer science skills. It’s best to deploy more expensive human resources — such as data scientists and ML engineers — on tasks that require expertise, collaboration, and analytical skills.
Effective or Not? The Real Impact of GDPR
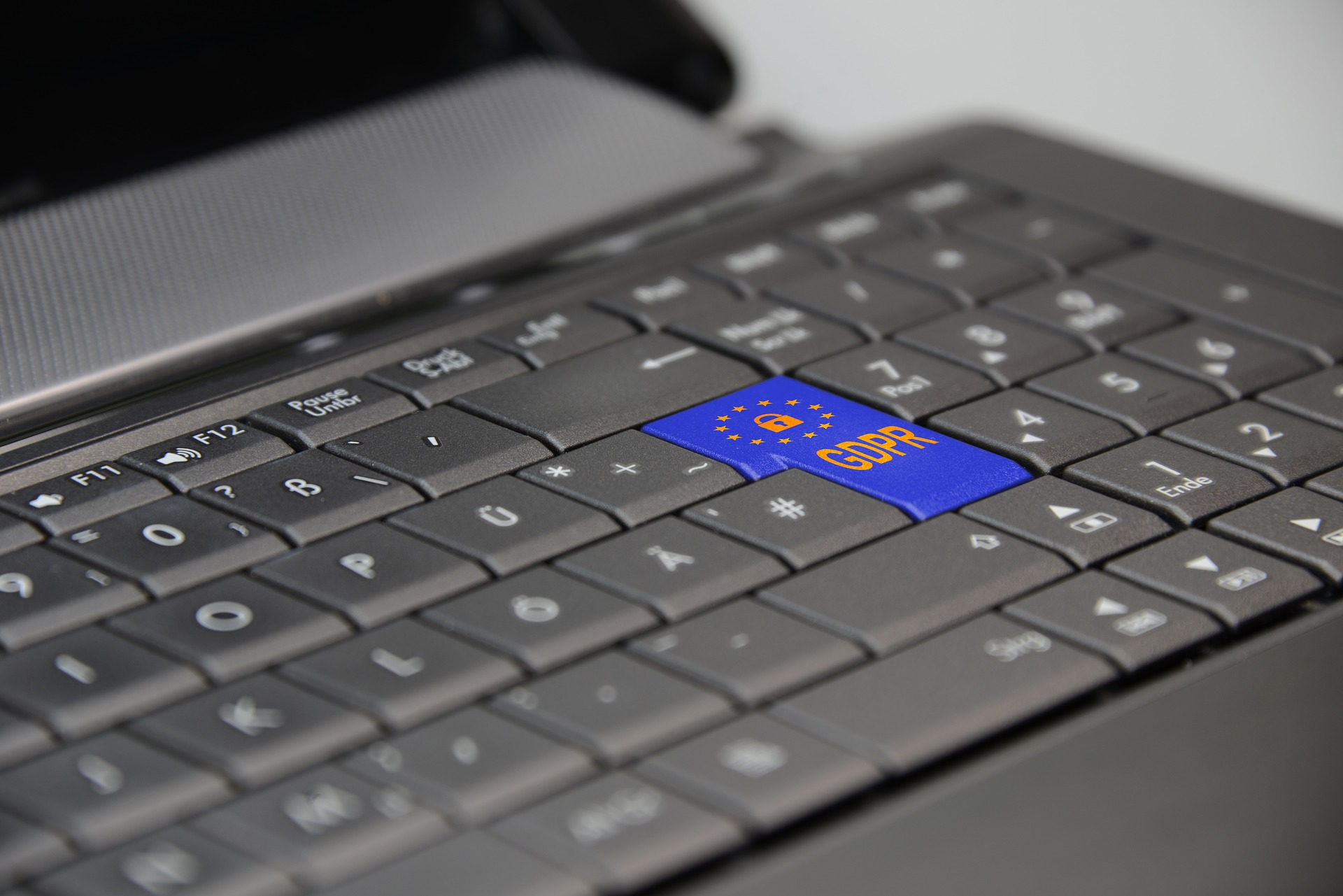
The General Data Protection Regulation wasn’t just meant to give governments the means to enforce data security rules. Another key objective was to change how both companies and users behave when it comes to ensuring personal data remains private and protected. In this sense, GDPR seems to have had the desired impact. ... Another interesting fact the data shows is that users may have moved some of their own responsibility to GDPR enforcers. Two indicators led to this observation: “Respondents are less likely to read privacy statements than they were in 2015 (-7 percentage points) “17% say it is enough for them to see the website has a privacy policy” so they choose not to read the document at all. A similar behavior pattern emerges when dealing with social media usage. Less users – 56% in 2019 vs 60% in 2015 – actually change their privacy settings for their personal profile. The three most common reasons social network users give for not trying to change their personal profile’s default settings are that they trust the sites to set appropriate privacy settings (29%) that they do not know how to (27%), or that they are not worried about sharing their personal data (20%).
5 steps for digital workplace transformation
Start by recognizing actionable opportunities within your business operations. Approach the prospects for digital transformation from a business instead of technology perspective. Line-of-business (LOB) teams should lead this effort, coordinating closely with senior IT staffers to identify critical barriers to success. Of course, each organization faces its own set of challenges. But, at the onset, step back and identify key themes -- accelerating innovation, enhancing productivity, improving governance or reshaping the steps in the customer journey -- that make good business sense. Consider operations as a whole, while focusing on people and processes, and determine your target audiences: employees, partners and/or customers. Then, engage a cross section of these audiences in conversations about what they are doing and how they understand the underlying business purposes. Develop both the technology and the business insights about what is happening from the participants' perspectives. Listen carefully as they describe their tasks, and be sure to observe how they do their work to determine where bottlenecks occur.
Quote for the day:
“The real voyage of discovery consists not in seeking new landscapes but in having new eyes.” -- Marcel Proust
No comments:
Post a Comment