Back to basics: the top five technical issues to address in industrial cyber security

While industrial facilities are facing more cyber security challenges than they used to, the good news is that awareness around these challenges is increasing. That said, there’s still a marked difference between how well cyber security is understood in the consumer and corporate IT worlds, and how well it’s understood in industrial environments driven by OT. In a sense that’s not surprising. After all, most well-publicised attacks have been in consumer and corporate IT. But with attacks on critical industrial environments now becoming more frequent, people are starting to wake up to the operational, financial, reputational and even human and environmental damage they can inflict. Awareness is one thing. But the fundamentals of cyber security are still not being practised regularly. What are those fundamentals? In our cyber security work with organisations operating critical infrastructures around the world in sectors including power, oil and gas, water management, manufacturing and maritime, we’ve identified the top five technical issues that need addressing.
Deep learning is about to get easier - more widespread

Deep learning algorithms often require millions of training examples to perform their tasks accurately. But many companies and organizations don’t have access to such large caches of annotated data to train their models (getting millions of pictures of cats is hard enough; how do you get millions of properly annotated customer profiles — or, considering an application from the health care realm, millions of annotated heart failure events?). On top of that, in many domains, data is fragmented and scattered, requiring tremendous efforts and funding to consolidate and clean for AI training. In other fields, data is subject to privacy laws and other regulations, which may put it out of reach of AI engineers. This is why AI researchers have been under pressure over the last few years to find workarounds for the enormous data requirements of deep learning. And it’s why there’s been a lot of interest in recent months as several promising solutions have emerged — two that would require less training data, and one that would allow organizations to create their own training examples.
Universities use data analytics to tackle student mental health
“What we are finding is that students with mental health conditions are far more likely to generate an alert than their peers,” says Ed Foster, the university’s student engagement manager. The alerts, which in its first year have been triggered for 3% to 4% of students, are sent to personal tutors, who can then contact the student. They also have access to a detailed dashboard on students’ engagement. “It helps them to prepare for that first discussion with a student, giving them some pointers,” says Foster. Nottingham Trent also provides students with access to their own dashboard, allowing them to see how they compare to averages for their peer group. Foster says that some students have found themselves motivated by the realisation they were falling behind. But some students at University of Leeds oppose such dashboards, according to Professor Neil Morris, dean of digital education.
Why Invest In Cloud-Based Machine Learning For Cybersecurity?
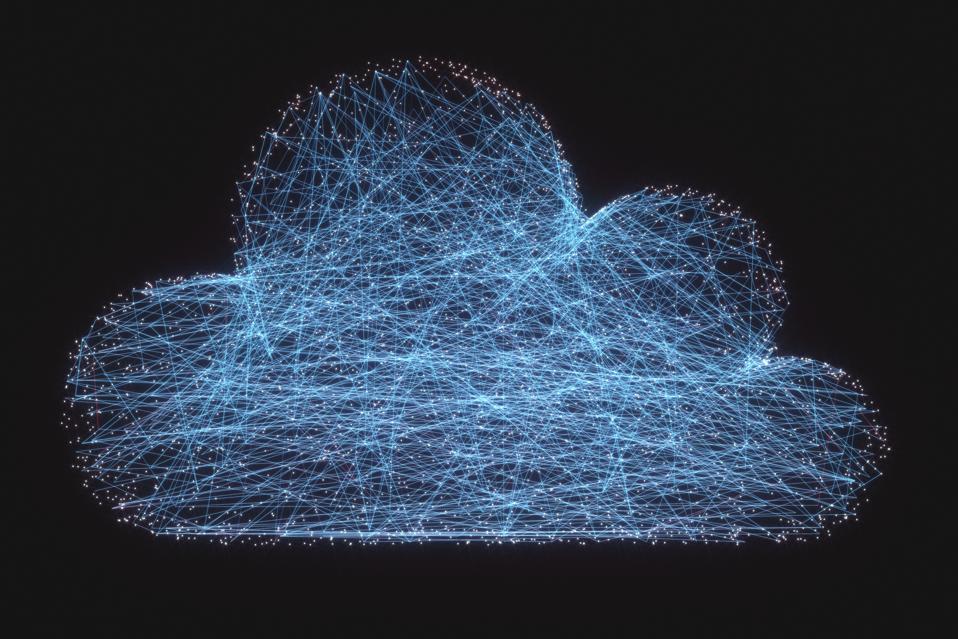
Offloading compute-intensive ML workloads to the cloud frees up precious local resources. Systems that do ML on the same local sensor that gathers the data itself must sacrifice performance and fidelity. By doing all the work on a local box, these tools spread themselves too thin and put a low cap on the value they can provide. Networks today move many gigabytes of data per second, and ML is only as accurate and valuable as the data you feed it; this means that the ideal scenario is one in which ML models can take advantage of all that data flying across the network. That simply isn't possible for products that restrict their ML processing to the compute power of an appliance in the data center. Moving ML to the cloud is the only way to take advantage of the sheer volume of data produced by today's enterprise networks. More often than not, modern enterprises are distributed across more than a single campus. Cloud-based ML architectures allow you to take full and cost-effective advantage of the intelligence, detection, and automation provided by ML-based technologies across all on-premises and cloud environments through a single deployment instead of getting limited insights that only apply to individual network regions where a box is installed.
Robotic Process Automation (RPA): 6 things people get wrong

“As companies look to digitally transform themselves, they are looking to streamline and modernize processes,” says John Thielens, CTO at Cleo. “While RPA perhaps can be viewed as a form of streamlining, it streamlines processes in place, but by itself does not necessarily improve them.” Thielens notes that this misunderstanding can occur in organizations that are looking for process improvements as part of a broader digital transformation; they might see RPA as a solution to process woes when it’s better looked at as a tool for achieving new efficiencies and productivity gains with well-established processes. There’s a related mistake people make with RPA: Automating a process you don’t fully understand. Eggplant COO Antony Edwards recently told us that this is a common pitfall: “Most people don’t have clearly defined processes, so they start automating, and either automate the wrong thing or get lost in trying to reverse-engineer the process.”
Govt panel says no need to mirror personal data in India

The government panel has, however, agreed with the Srikrishna panel on health data where it had said personal data relating to health should be permitted to be transferred abroad for reasons of prompt action or emergency. The relaxation by a government panel with regard to storage and processing of personal data comes after last month RBI also relaxed its April 2018 circular which required that all payment data generated in India should be stored within the country. This came as some sort of relaxation for major international firms such as American Express, Visa, Mastercard, Amazon, Paypal, Western Union, etc. In its amended circular, RBI has allowed such international firms to store data abroad in cases where the transaction originates in the country but gets completed overseas, with a proviso that a mirror copy in such transactions be stored in India. However, for end-to-end domestic transactions all storage still needs to be done within the country. Earlier, the RBI had not made a clear-cut distinction between transactions which are completed within the country and transactions which originate here and get completed overseas.
Why AI is entering its golden age

AI is working everywhere. To take one framework, think about the product lifecycle: You have to figure out what products or services to create, figure out how to price it, decide how to market and sell and distribute it so it can get to customers. After they’ve bought it, you have to figure out how to support them and sell them related products and services. If you think about this entire product lifecycle, AI is helping with every single one of those [stages]. For example, when it comes to creating products or services, we have this fantasy of people in a garage in Silicon Valley, inventing something from nothing. Of course, that will always happen. But we’ve also got companies that are mining Amazon and eBay data streams to figure out, what are people are buying? What’s an emerging category? If you think about Amazon’s private label businesses like Amazon Basics, product decisions are all data-driven. They can look to see what’s hot on the platform and make decisions like “oh, we have to make an HDMI cable, or we have to make a backpack.”
Managed security services will take center stage at Black Hat

A global cybersecurity skills shortage, that’s what. ESG research indicates that 53% of organizations say they have a problematic shortage of cybersecurity skills (note: I am an ESG employee). Furthermore, the recently published research report from ESG and the information systems security association (ISSA) indicates that 73% of organizations have been impacted by the cybersecurity skills shortage. Sixty-six percent of those impacted say the cybersecurity skills shortage has increased the workload on the infosec team, 47% say the cybersecurity skills shortage has led to the inability to learn or use cybersecurity technologies to their full potential, and 41% have had to hire and train junior employees rather than hiring more experienced staff. There’s one more implication around the cybersecurity skills shortage — nearly one-third (32%) of organizations have had to increase their use of professional/managed services because they remain understaffed and lacking advanced cybersecurity skills. Like I said, organizations can no longer tow the cybersecurity line alone — they need help.
DMARC's abysmal adoption explains why email spoofing is still a thing
Companies are not taking advantage of the protocol, despite the fact that DMARC has been around for years. This means that most companies are still vulnerable to business email compromise (BEC) attacks, phishing emails, and other types of email scams, as hackers can easily make their emails look authentic and pass their scams as legitimate communications. The good news is that DMARC adoption rate is better than previous years. However, the bad news is that the needle is moving too slowly to make a difference. An FTC report from 2017 found that only 10% of 569 businesses with a significant online presences had deployed DMARC policies with their domains. A November 2018 Agari report found that half of the Fortune 500 companies were supporting DMARC, but that only 13% of companies had actually bothered to set up DMARC rejection policies -- meaning the DMARC protocol was installed, but wasn't actually used to stop spam, phishing, and scams from spoofed domains. A quarter later, in February 2019, the same Agari reported that the percentage of Fortune 500 companies actively using DMARC policies had gone up to 15%. A small increase, but still insufficient, since this still left hundreds of the world's biggest companies open to attacks.
Rediscovering Lean
The trouble is, deep down, I knew I was not! My engineering metrics were great, better than ever. But my Pirate metrics (AAARRR!) sucked. Where was the real user impact I wanted? I grew weary. Each new initiative and with every one of those practices I adopted, I changed how I worked, which required conscious effort to discover, learn, implement and refine. Spending all my mental energy on improving delivery metrics was bad; continuously improving the wrong thing was worse. In the middle of this crisis of confidence, my head of engineering asked me to lead the development of a new mobile product. This was a big deal based on a real user need we could address. Along with it also came a large chance of failure. A year previously, I would have jumped on it. But with more self-awareness around my limitations delivering on such a large project, I wavered. With my approach not working, I needed to change. Was a killer process to solve my problems just around the corner, awaiting discovery?
Quote for the day:
"Leadership is developed daily, not in a day." -- John C. Maxwell
No comments:
Post a Comment