DigitalOcean aligns with MongoDB for managed database service

There is, of course, no shortage of DBaaS options these days. DigitalOcean is
betting that its Managed MongoDB service will not only extend the appeal of its
cloud service to developers, but also to SMBs that are looking for less costly
alternatives to the three major cloud service providers, Cooks said. MongoDB
already has a strong focus on developers who prefer to download an open source
database to build their applications. In addition to not having to pay an
upfront licensing fees, in many cases developers don’t need permission from a
centralized IT function to download a database. However, once that application
is deployed in a production environment, some person or entity will have to
manage the database. That creates the need for the DBaaS platform from MongoDB
that DigitalOcean is now reselling as an OEM partner, said Alan Chhabra, senior
vice president for worldwide partners at MongoDB. The DigitalOcean Managed
MongoDB service is an extension of an existing relationship between the two
companies that takes managed database services to the next logical level,
Chhabra asserted. “We have a long-standing relationship,” he said.
Digital transformation at SKF through data driven manufacturing approach using Azure Arc enabled SQL
As SKF looked for a solution that supported their data-driven manufacturing
vision for the Factories of the Future, they wanted a solution that was able to
support distributed innovation and development, high availability, scalability
and ease of deployment. They wanted each of their factories to be able to
collect, process, analyze data to make real-time decisions autonomously while
being managed centrally. At the same time, they had constraints of data latency,
data resiliency and data sovereignty for critical production systems that could
not be compromised. The drivers behind adopting a hybrid cloud model came from
factories having to meet customer performance requirements, many of which depend
on ability to analyze and synthesize the data. Recently, the Data Analytics
paradigms have shifted from Big Data Analysis in the cloud to more Data-Driven
Manufacturing at the machine, production line and factory edge. Adopting cloud
native operating models but in such capacity where they can execute workloads
physically on-premises at their factories turned out to be the right choice for
SKF.
A new dawn for enterprise automation – from long-term strategy to an operational imperative

To drive sustainable change, organisations need to take a large-scale,
end-to-end strategic approach to implementing enterprise automation solutions.
On one level, this is a vital step to avoid any future architecture problems.
Businesses need to spend time assessing their technology needs and scoping out
how technology can deliver value to their organisation. Take, for example, low
code options like Drag and Drop tools. This in vogue technology is viewed by
companies as an attractive, low-cost option to create intuitive interfaces for
internal apps that gather employee data – as part of a broad automation
architecture. The issue is lots of firms rush the process, failing to account
for functionality problems that regularly occur when integrating into existing,
often disparate systems. It is here where strategic planning comes into its own,
ensuring firms take the time to get the UX to the high standard required, as
well as identify how to deploy analytics or automation orchestration solutions
to bridge these gaps, and successfully deliver automation. With this strategic
mindset, there is a huge opportunity for businesses to use this thriving market
for automation to empower more innovation from within the enterprise.
The Rise Of NFT Into An Emerging Digital Asset Class
The nature of NFTs being unique, irreplaceable, immutable, and non-fungible
makes them an attractive asset for investors and creators alike. NFTs have
empowered creators to monetize and value their digital content, be it music,
videos, memes, or art on decentralized marketplaces, without having to go
through the hassles that a modern-day creator typically goes through. NFTs, at
their core, are digital assets representing real-world objects. ... NFTs solve
the age-old problems that creators like you and I have always faced when
protecting our intellectual property from being reproduced or distributed across
the internet. The most popular standard for NFTs today are ERC-721 and ERC-1155.
ERC-721 has been used in a majority of early NFTs until ERC-1155 was introduced.
With that said, these token standards have laid the foundation for assets that
are programmable and modifiable; therefore, setting the cornerstone for digital
ownership leading to all sorts of revolutionary possibilities. The NFT ecosystem
has found its way into various industries as more people join hands and dive
deeper into its novel possibilities.
Three Principles for Selecting Machine Learning Platforms
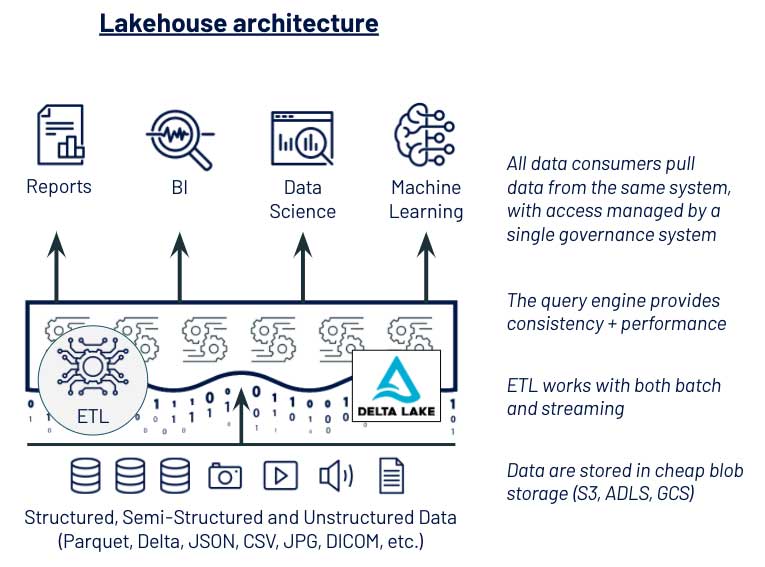
Of the challenges this company faced from its previous data management system,
the most complex and risky was in data security and governance. The teams
managing data access were Database Admins, familiar with table-based access.
But the data scientists needed to export datasets from these governed tables
to get data into modern ML tools. The security concerns and ambiguity from
this disconnect resulted in months of delays whenever data scientists needed
access to new data sources. These pain points led them towards selecting a
more unified platform that allowed DS & ML tools to access data under the
same governance model used by data engineers and database admins. Data
scientists were able to load large datasets into Pandas and PySpark dataframes
easily, and database admins could restrict data access based on user identity
and prevent data exfiltration. ... A data platform must simplify collaboration
between data engineering and DS & ML teams, beyond the mechanics of data
access discussed in the previous section. Common barriers are caused by these
two groups using disconnected platforms for compute and deployment, data
processing and governance.
Introduction To AutoInt: Automatic Integration For Fast Neural Volume Rendering
AutoInt, also known as Automatic integration, is a modern image rendering
library used for high volume rendering using deep neural networks. It is used
to learn closed-form solutions to an image volume rendering equation, an
integral equation that accumulates transmittance and emittance along rays to
render an image. While conventional neural renderers require hundreds of
samples along each ray to evaluate such integrals and require hundreds of
costly forward passes through a network, AutoInt allows evaluating these
integrals with far fewer forward passes. For training, it first instantiates
the computational graph corresponding to the derivative of the
coordinate-based network. The graph is then fitted to the signal to integrate.
After optimization, it reassembles the graph to obtain a network that
represents the antiderivative. Using the fundamental theorem of calculus
enables the calculation of any definite integral in two evaluations of the
network. By applying such an approach to neural image rendering, the tradeoff
between rendering speed and image quality is improved on a greater scale, in
turn improving render times by greater than 10× with a tradeoff of slightly
reduced image quality.
How Google is Using Artificial Intelligence?
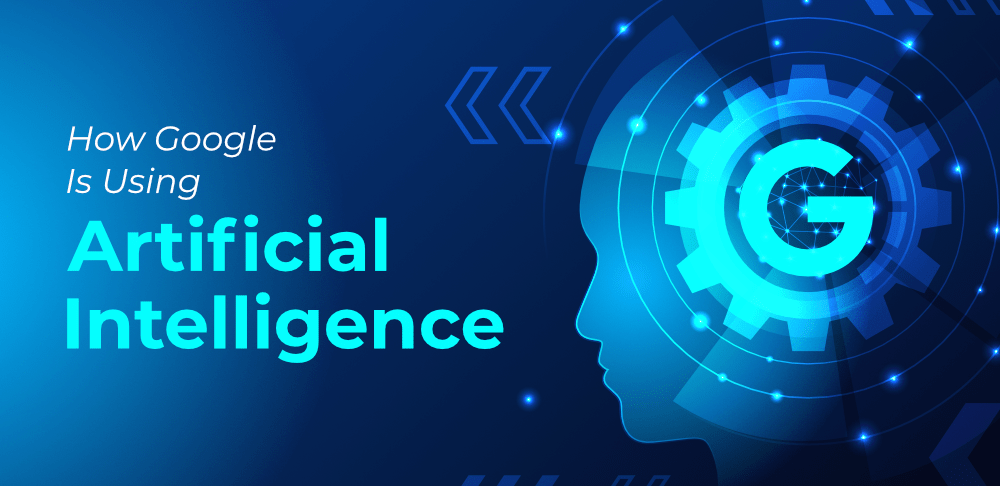
In the old times, we were much dependent on the paper map or the suggestions
of people well-versed with the tracks of our destinations. But with that, the
problem was we never reached on time to our spots. Now, you need not seek such
suggestions from the people or a paper Map as Google Maps has solved the
related difficulties. With territories and over 220 countries like Delhi, the
United States, Pakistan, Australia, etcetera one can affordably reach the
places already decided. You may curiously ask about the technology embedded
and the answer for this is Artificial Intelligence. The main concept is global
localization which is relying on AI. This helps Google Maps understand your
current or futuristic orientation. Later, it lets the application precisely
spot your longitudinal and latitudinal extent and as you or your vehicle
proceed further, Google Maps starts localizing hundreds of trillions of street
views. As you keep on traversing, the application announces a series of
suggestions thereby helping you reach a shopping mall, airport, or other
transit stations. Apart from this, you can prepare a list of places you will
visit, set routing options as per your preferences, explore the Street View
option in Live mode, and so on.
What is edge computing and why does it matter?
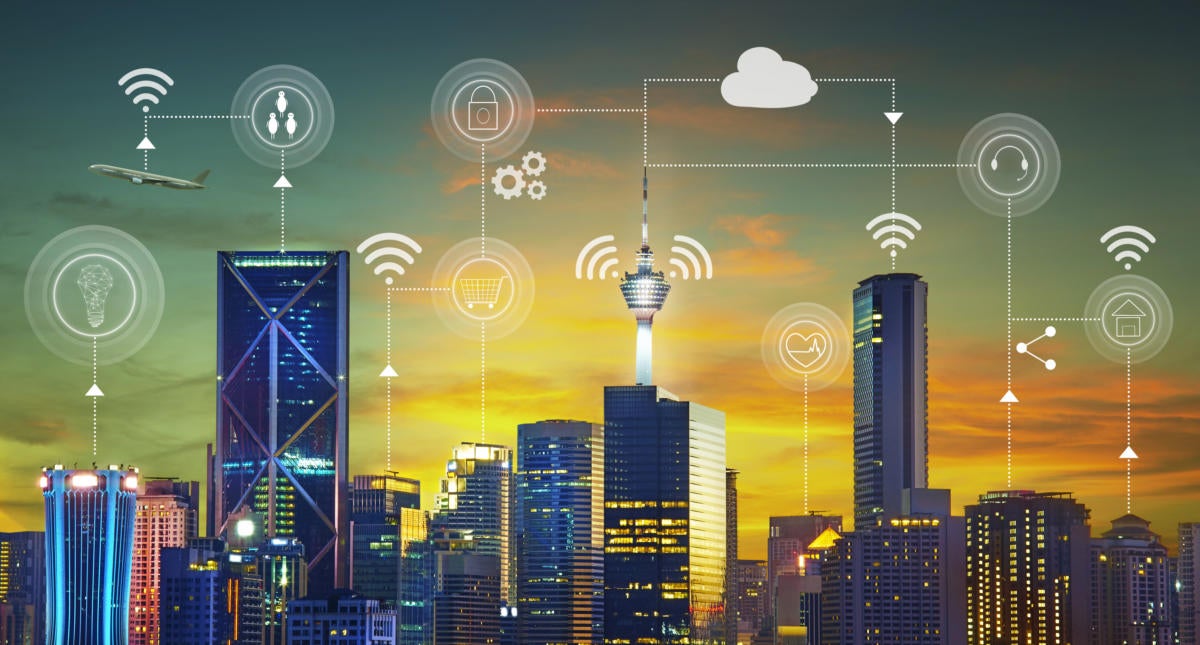
There are as many different edge use cases as there are users – everyone’s
arrangement will be different – but several industries have been particularly
at the forefront of edge computing. Manufacturers and heavy industry use edge
hardware as an enabler for delay-intolerant applications, keeping the
processing power for things like automated coordination of heavy machinery on
a factory floor close to where it’s needed. The edge also provides a way for
those companies to integrate IoT applications like predictive maintenance
close to the machines. Similarly, agricultural users can use edge computing as
a collection layer for data from a wide range of connected devices, including
soil and temperature sensors, combines and tractors, and more. The
hardware required for different types of deployment will differ substantially.
... Connected agriculture users, by contrast, will still require a rugged edge
device to cope with outdoor deployment, but the connectivity piece could look
quite different – low-latency might still be a requirement for coordinating
the movement of heavy equipment, but environmental sensors are likely to have
both higher range and lower data requirements – an LP-WAN connection, Sigfox
or the like could be the best choice there.
Artificial Intelligence (AI): 4 novel ways to build talent in-house

To discover the gems hidden across your organization, you must start
maintaining a self-identified list of skills for every employee. The list must
be updated every six months and be openly searchable by associates to make it
useful and usable. Palmer recommends self-classifying each individual’s skills
into four categories: expert, functioning, novice, and desired stretch
assignment. This allows teams with hiring needs to scout for individuals with
ready skills and those with growth aspirations in the five competencies needed
for AI. Finding the right content to upskill your in-house teams is a
challenge. Despite the rapid mushrooming of training portals and MOOCs
(massive open online courses), the curriculums may not meet your
organization’s specific needs. However, with access to such great content
online, often for free, it may not make sense to recreate your content. “You
must design your own curriculum by curating content from multiple online
sources,” says Wendy Zhang, director of data governance and data strategy at
Sallie Mae. Base the training plan on your team’s background, roles, and what
they need to succeed.
Solving Mysteries Faster With Observability
Let's start by looking at the sources that we turn to when we look for clues. We
often begin with observability tooling. Logs, metrics, and traces are the three
pillars of observability. Logs give a richly detailed view of an individual
service and provide the service a chance to speak its own piece about what went
right or what went wrong as it tried to execute its given task. Next, we have
metrics. Metrics indicate how the system or subsets of the system, like
services, are performing at a macro scale. Do you see a high error rate
somewhere, perhaps in a particular service or region? Metrics give you a bird's
eye view. Then we have traces, which follow individual requests through a
system, illustrating the holistic ecosystem that our request passes through. In
addition to observability tooling, we also turn to metadata. By metadata, I mean
supplemental data that helps us build context. For us at Netflix, this might be,
what movie or what show was a user trying to watch? What type of device were
they using? Or details about the build number, their account preferences, or
even what country they're watching from. Metadata helps add more color to the
picture that we're trying to draw.
Quote for the day:
"A sense of humor is part of the art
of leadership, of getting along with people, of getting things done." --
Dwight D. Eisenhower
No comments:
Post a Comment