India gears up for historic data protection law

India is getting ready for the law after the Narendra Modi government listed it as one of the bills in the Parliament last week, in the first session after the general elections. The election in April gave Modi a second term with a massive majority. The bill will create a legal regime for how data can be shared, stored and used in India. The proposed law, once passed by Parliament, will have major consequences for technology companies hoping to build businesses that access user data. Most technology companies like Google, Facebook and others now thrive on data generated by their users to earn billions of dollars worldwide. Mukesh Ambani who heads Reliance Industries, India’s biggest firm working in the energy, telecommunications and retail space, pointed out in January this year that “data is the new oil“. However, while the cabinet is yet to clear the final draft of the data protection bill, technology and privacy experts are concerned about the implications of the proposed new law. Many companies, including the technology giants hoping to tap into India’s massive markets, are apprehensive about what this entails for their core business models.

“You have to be careful when there is sensitivity around personal data,” Kampman said. Whether it’s AI or any identity-related effort, “you need governance over this to be clear about what can be used and what can’t be used for a given purpose. You are a custodian of data and when you aggregate that data your responsibilities increase exponentially.” Broadly, the looking-before-leaping paradigm is in full force here. As government IT leaders and their business-line peers seek to better manage access and identity in an emerging cloud-driven enterprise, they’ll need to be thoughtful not just about the how, but about the why behind their efforts. “There needs to be a strategy,” Kampman said. “What is the outcome going to be? The technology world can solve these problems but it needs to be done with a viewpoint toward how it will appear to the end user. You want to have control over the technologies but you also want all the stakeholders to have an opportunity to contribute toward governance.”
Google has more deep data knowledge than any company in the world, and it is no slouch in the discipline of design. It’s only natural that the company would combine this expertise. Initially, the audience for the new data design guidelines was Google itself, but much as it did for Material Design, the company decided to publicize these best practices and encourage others to adopt them—anyone from app developers to everyday people who are left wondering why their PowerPoint chart sucks. “We started doing this internally as a way to guide [employees] through the do’s and don’ts of chart creation,” Lima tells Fast Company. “After conducting various research studies and partnering with teams across the company, the do’s and don’ts evolved into a set of high-level principles that were strongly rooted in Google-wide tenets crucial to the company’s growth, brand, and culture. These principles are meant to be generative and not prescriptive. We hope they can help any chart creator during ideation and evaluation.” The six principles read something like an introductory data design course.
Blockchain Technology: Enabling Enterprise Innovation
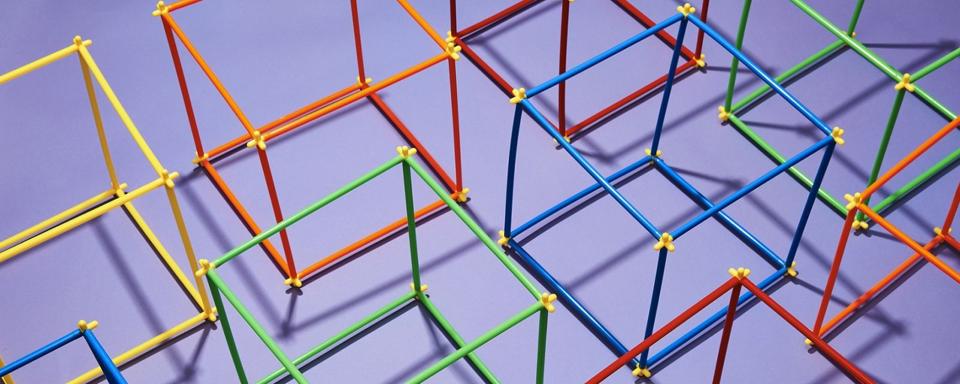
The most satisfying finding from Deloitte is that business leaders are taking blockchain as seriously as we’d hoped. Deloitte found that “53 percent of respondents say that blockchain technology has become a critical priority for their organizations in 2019—a 10-point increase over last year.” That more than half of the respondents name blockchain technology as a critical priority is, in my eyes, the first tremor in what promises to be a substantial shake up of the business technology landscape. Accordingly, when the authors report that many leaders are focusing less on whether blockchain works (spoiler: it does) and more on what business models it might disrupt, they quote Deloitte Consulting LLP Principal Linda Pawczuk, Deloitte consulting leader for blockchain and cryptocurrency. She says, “We believe executives should no longer ask a single question about blockchain but, rather, a broad set of questions reflecting the role blockchain can play within their organization.”
Here Is A Look At Where Fintech Is Leading Us And Why
The business consultancy powerhouse reports that there is an abundance of fintech enterprises entering the market using novel business models and delivering fresh consumer offerings. Furthermore, says E&Y, the emerging fintech revolution is driving information sharing and the development of open-source Application Program Interfaces (APIs) as well as recent technological breakthroughs in artificial intelligence (AI) and biometrics. Around the world, lawmakers are following the example of Europe by promoting open access Application Programming Interfaces (APIs). By doing so, legislators desire to enhance consumer choice by increasing competition between banks and fintech enterprises. For new fintech firms, open-source APIs streamline the launch of new products and services and decrease costs customarily used for research and development. New fintech banks that build their organization around a digital business model represent the fastest growing segment of startups nurtured by this movement.
Image Classification Using Neural Networks in .NET
Image classification is one of the most common use cases for non-recurrent neural networks. The basic concept is that a neural network is given an input image, whose input layer has the same number of neurons as the pixels in the image (assuming the image is grayscale). Also depending on the number of classifications to be made available, this neural network should have the same number of output neurons. The neural network could use either convolutional, fully connected layers or a combination of both. Convolutional networks are faster as they squish the input image and convolute them using multiple kernels to extract important features. More details on convolution can be found here. Convolution greatly reduces the size of the fully connected networks which are used to classify the image after series of convolutions and pooling. As the neural network using appropriate activation functions can only have inputs and outputs as a double ranging from 0 to 1, to input an image to a neural network will require some pre-processing on the input end to normalize the pixels into this form.
Fortune 100 passwords corporate secrets left exposed on unsecured Amazon S3 server

Some of the world’s biggest companies have had 750GB worth of their innermost secrets revealed on unsecured Amazon S3 buckets, available for anybody to download – no password required. The startling revelation came from researchers at UpGuard, who discovered three publicly accessible Amazon S3 buckets related to Attunity, a leading provider of data integration and big data management software solutions, on May 13th 2019. The fact that Attunity is at the centre of the security breach is a concern, simply because of its impressive list of customers. On its website, the company boasts that it counts more than 2,000 enterprises and half the Fortune 100 in its customer base. According to screenshots published on UpGuard’s blog, Fortune 100 companies such as Netflix, Ford, and TD Bank were amongst those who had their data recklessly exposed. For instance, the researchers discovered files containing the usernames and passwords of Netflix database systems, and internal Ford presentations.
NotPetya Retrospective
Each of the companies impacted by NotPetya (and WannaCry before it) had some degree of security protection in place—the usual stuff like firewalls, antivirus, and patch management. That defense obviously wasn’t perfect or the attack would have been thwarted, but a perfect defense costs $∞ and is therefore impractical. As we deal with the realities of an imperfect defense, it becomes necessary to choose between preventative and reactive measures. Security expert Bruce Schneier makes the point on his resilience tag: ‘Sometimes it makes more sense to spend money on mitigation than it does to spend it on prevention.’ An investment in mitigation can also pay off in all kinds of ways that have nothing to do with attacks: that change that was just accidentally made to production when it should have been in test—fixed in seconds, by reverting to the last snapshot. NotPetya is unlikely to keep its ‘most devastating cyber attack’ title for long. There will be another attack, and we should expect it to be worse. Moving away from a trusted network model to a zero-trust model is the most effective way to defend against such attacks. But, effort should also focus on measures that allow speedy recovery.
Managing Machine Learning Models The Uber Way

With access to the rich dataset coming from the cabs, drivers, and users, Uber has been investing in machine learning and artificial intelligence to enhance its business. Uber AI Labs consists of ML researchers and practitioners that translate the benefits of the state of the art machine learning techniques and advancements to Uber’s core business. From computer vision to conversational AI to sensing and perception, Uber has successfully infused ML and AI into its ride-sharing platform. Since 2017, Uber has been sharing the best practices of building, deploying, and managing machine learning models. Some of the internal tools and frameworks used at Uber are built on top of popular open source projects such as Spark, HDFS, Scikit-learn, NumPy, Pandas, TensorFlow and XGBoost. Let’s take a closer look at Uber’s projects in the ML domain. Michelangelo is a machine learning platform that standardized the workflows and tools across teams through an end-to-end system. It enabled developers and data scientists across the company to easily build and operate machine learning systems at scale.
Are You Choosing Fintech—or Is Fintech Choosing You?

The type of financial technology solutions that are best suited for any particular institution vary tremendously. Some institutions may be looking to digitize or modernize processes from within, others may be looking to add-on a single solution such as mobile payments. Fintech solutions could also involve data aggregation or lead generation activities as well as arrangements to buy assets, such as small business loans, from leading online lenders. Once the fintech solution is identified, each institution needs to identify the best strategy for itself to either compete or collaborate with emerging players—and capitalize on trends and capabilities to position itself with the most competitive advantage going forward. There are generally two broad strategies that financial institutions can pursue: invest in or build emerging technologies on your own, or buy, partner or network with fintech companies. ... Consider building in-house if there are sufficient internal resources, expertise and scale to innovate and customize unique capabilities. These strategies may be more appropriate for regional or larger banks than smaller community banks.
Quote for the day:
"There are some among the so-called elite who are overbearing and arrogant. I want to foster leaders, not elitists." - Daisaku Ikeda
No comments:
Post a Comment