Navigating Tomorrow: Becoming an Enterprise of the Future
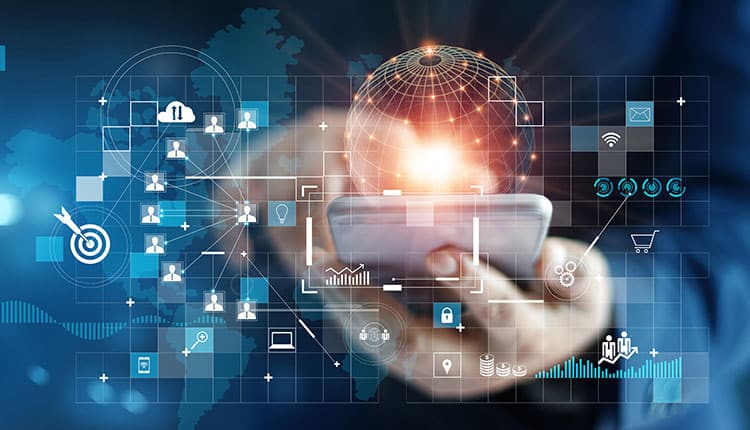
Preparing for what lies ahead goes far beyond just implementing the right
technologies, it is about developing a culture that embraces change with
empathy. Cultivating a mindset across the organisation that values innovation,
continuous learning, and agility ensures that every employee charges forward
with confidence. In times of economic uncertainties and technological
advancements, it is crucial that we practice empathy. Naturally, there is some
fear that technologies like AI will replace human workers. As such, leaders must
help employees understand that technology is here to augment their roles and
empower them to spend more time on other valuable tasks. The key to embracing
any new technology and providing access at scale is to get everyone in the team
on board. Whether greeted with excitement or anxiety, leaders must champion
this culture of change by encouraging employees to seek new ways of working
while ensuring they remain engaged and valued. Certainly, data-driven
decision-making will undoubtedly continue to be the cornerstone of future
business attempts.
The Importance of Enterprise Architecture in the Modern Business Landscape
-1.png?width=750&height=561&name=Untitled%20design%20(43)-1.png)
The field of Enterprise Architecture is constantly evolving, driven by emerging
trends and innovations. One of the significant trends is the adoption of cloud
computing and hybrid IT environments. Cloud-based solutions offer scalability,
flexibility, and cost-efficiency, making them increasingly popular among
businesses. Enterprise Architecture helps organizations leverage these
technologies by designing architectures that integrate cloud services and
on-premises infrastructure, ensuring seamless operations and efficient resource
utilization. Another emerging trend is the incorporation of artificial
intelligence (AI) and machine learning (ML) in Enterprise Architecture
practices. AI and ML technologies enable businesses to automate processes,
analyze vast amounts of data, and gain valuable insights. By integrating AI and
ML into their Enterprise Architecture frameworks, organizations can enhance
decision-making, optimize business processes, and improve overall efficiency.
Furthermore, the rise of digital transformation has had a significant impact on
Enterprise Architecture.
Top 8 challenges IT leaders will face in 2024

To guide an organization through uncertainty, IT leaders must help ensure
everyone in the company is on the same page, Srivastava says. Instead of playing
catch-up, he suggests a proactive approach with clear communication as a guiding
principle. “It starts with establishing a clear set of agreed upon initiatives
and outcomes for the organization,” he says. “We have to make sure everyone
understands what they are doing, why they are doing it, and — most importantly —
how success will be measured.” ... Security is a challenge that makes the list
of top CIO worries perennially, but Grant McCormick, CIO of cybersecurity
company Exabeam, notes a rising need for increased collaboration between IT and
security teams to address the issue. “The role of the CIO has recently seen a
massive convergence with cybersecurity,” says McCormick. “Regardless of whether
or not security reports into the CIO, or another leader within the company, it
is in everyone’s best interest to be conscious of the organization’s security
posture and to enable IT and cybersecurity to work in a highly synchronized
manner.”
Economic Uncertainty Doesn’t Mean Compromising Cybersecurity

This futuristic technology isn’t just something to tap into to enrich
individual experiences; it is also to help solve some of society’s most
pressing challenges and, most of all, to keep people safe. For
cryptocurrencies, where there is estimated to be four times more fraud than in
regular fiat payments, technology providers are devising new innovations to
stay ahead. New solutions can help customers make informed decisions that
protect their business, as well as the entire payments ecosystem. A simple
dashboard can provide visibility of crypto spend, transaction volumes and an
anti-money laundering risk rating exposure. Through solutions like these,
banks and other businesses can earn and, importantly, keep the trust of their
customers—on whom their business depends. Trust is fragile. It can be broken
in a nanosecond. And as the global financial ecosystem expands, it’s getting
harder for organizations to navigate the maze of cyber risks alone.
Businesses, merchants, financial institutions and fintechs need trailblazing
tools and expert knowledge to understand the risks they’re facing.
Redefining Data Governance: Bridging The Gap Between Technical And Domain Experts

As the data industry gravitates toward decentralization, specifically
federated systems, the absence of a robust framework in data governance,
master data and data quality becomes glaringly evident. The prevailing issue
in many companies is not the sheer volume of data or a lack of technological
options but the erroneous assumption that their data is inherently primed for
insights, AI applications and democratization. This misconception overshadows
the real challenge: the need for a comprehensive approach to data management
that integrates the expertise of domain professionals. The advent of practical
AI applications marks a watershed moment in the history of data governance.
This technology is not just a tool for automation; it serves as a bridge
between the technical and business realms. It provides a platform where
business experts can meaningfully contribute to data strategies and
decision-making processes. Technical teams initially assumed the mantle of
data governance out of necessity due to the requisite skill sets.
Orchestrating Resilience Building Modern Asynchronous Systems
/filters:no_upscale()/articles/orchestrating-resilience-modern-asynchronous-systems/en/resources/65figure-1-1704815132419.jpg)
The first one is state management. Basically, the problem here is that you
need to contemplate lots of possible combinations of states and events. For
example, the "review received" message could come in while the campaign is in
pending state instead of the relevant waiting state, or an out of sequence
event could come in from somewhere, and so on. All of those cases need to be
handled, even though they are not the most likely sequence of events and
states. ... Handling retries becomes a task almost as complex as implementing
primary logic, sometimes even more so. You can think of implementing your
retry mechanisms in different ways, for example by storing a retry counter in
the database and incrementing it on each failed attempt until either you
succeed or reach the maximum allowed number of retries. Alternatively, you
could embed the retry counter in the queue message itself, so you dequeue a
message, process it, and, if it fails, re-enqueue the message and increment
the retry count. In both cases this implies a huge overhead for developers.
Attackers deploy rootkits on misconfigured Apache Hadoop and Flink servers

In the attack chain against Hadoop, the attackers first exploit the
misconfiguration to create a new application on the cluster and allocate
computing resources to it. In the application container configuration, they
put a series of shell commands that use the curl command-line tool to download
a binary called “dca” from an attacker-controlled server inside the /tmp
directory and then execute it. A subsequent request to Hadoop YARN will
execute the newly deployed application and therefore the shell commands. Dca
is a Linux-native ELF binary that serves as a malware downloader. Its primary
purpose is to download and install two other rootkits and to drop another
binary file called tmp on disk. It also sets a crontab job to execute a script
called dca.sh to ensure persistence on the system. The tmp binary that’s
bundled into dca itself is a Monero cryptocurrency mining program, while the
two rootkits, called initrc.so and pthread.so, are used to hide the dca.sh
script and tmp file on disk. The IP address that was used to target Aqua’s
Hadoop honeypot was also used to target Flink, Redis, and Spring framework
honeypots
Merck's Cyberattack Settlement: What Does it Mean for Cyber Insurance Coverage?
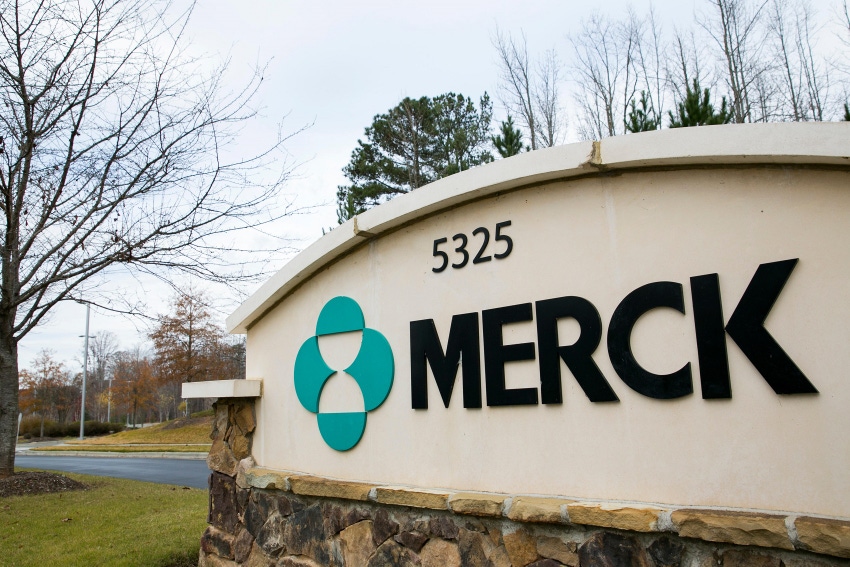
The Merck and Mondelez cases are likely not going to be the last of their
kind. More legal disputes between insurers and insureds, whether regarding war
exclusions or other issues, could arise in the future. “I think that the cyber
litigation is just getting started,” says Stern. More cases could drive change
in the way cyber insurance companies approach risk tied to cyberattacks and
what is considered cyberwarfare. When new risks challenge the existing
approach to coverage, it drives industry change. “Maybe it takes a second or a
third dispute to really achieve a definitive conclusion on that particular
matter,” says Kannry. “Then, what can often happen is insurance industry says,
‘You know what, that type of loss needs to be understood and defined
separately.’” Compared to many other insurance products, cyber insurance is
relatively new. That means there remains plenty of room for the development of
innovative ways to offer cyber insurance coverage. But the road forward likely
won’t be without bumps for insurers and insureds.
Organizations Must Be Prudent To Realize Value In Generative AI
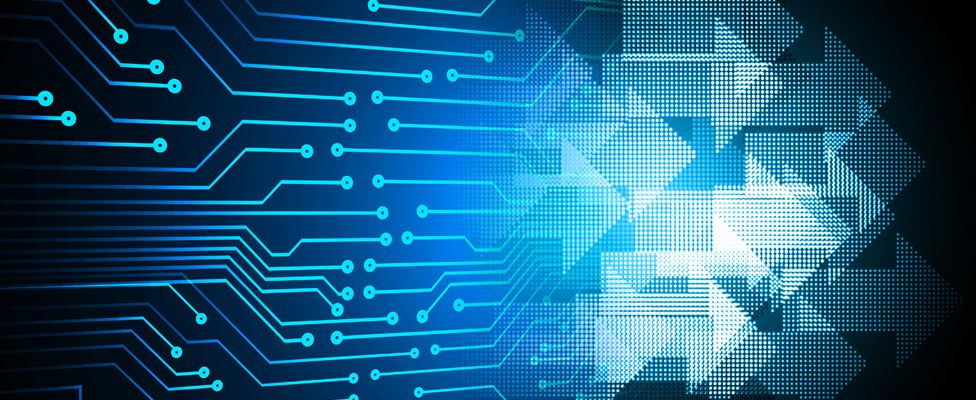
Rather than being swayed by the allure of generative AI capabilities, remain
steadfast about the core features that can genuinely transform and enhance
your operations. This pragmatic approach should be considered a short- to
mid-term strategy for any forward-thinking organization. The reality is that
features closely coupled with generative AI capabilities are still on the
horizon. It will be at least a couple of years before they become commonplace.
To navigate this transformative landscape effectively as an analytics
professional, you must equip yourself with a deep understanding of generative
AI. This proficiency will enable you to distinguish between features loosely
coupled with generative AI and features that are natively and seamlessly
integrated into the technology stack. Furthermore, keep a vigilant eye on the
vendors supplying your critical business software. A vendor's stance and
commitment to generative AI can profoundly impact how your organization
operates in the future.
LLM hype fades as enterprises embrace targeted AI models
LLMs were created by research teams exploring the capabilities of AI
technology rather than as models designed to solve specific business problems.
As a result, their capabilities are broad and shallow — writing a fairly
generic email or press releases, for example. For the modern business, they
have limited capabilities beyond that, requiring more data to produce results
with any depth. While the AI landscape used to be dominated solely by OpenAI,
major names in the tech world are beginning to outperform ChatGPT with their
own LLMs, including Google’s new Gemini model. However, due to the broad
capabilities of these new large language models, the text and image-based
benchmarks used to determine the model’s prowess were just as general. These
benchmarks ranged from simple multi-step reasoning to basic arithmetic. If an
AI company’s gauge for a successful Generative AI platform is how correctly it
can complete rudimentary math equations, that has little to no relevance for
the work of an enterprise organization.
Quote for the day:
"Before you are a leader, success is
all about growing yourself when you become a leader, success is all about
growing others." -- Jack Welch
No comments:
Post a Comment