
Humanizing experiences (HX) are disrupting and driving the democratization and
commoditization of AI. These more human experiences rely on immersive AI. By
2030, immersive AI has the potential to co-create innovative products and
services navigating through adjacencies and double up the cash flow, opposed to
a potential 20% decline in cash flow with nonadopters, according to McKinsey.
GAFAM has been an influential force in pioneering and championing deep learning
with its core business fabric. NATU and BAT have deeply embedded AI into their
most profound route. Google’s Maps and Indoor Navigation, Google Translate and
Tesla’s autonomous cars all exemplify immersive AI. Global AI marketplace is an
innovative business model that provides a common marketplace for AI product
vendors, AI studios and sector/service enterprises to offer their niche ML
models through a multisided platform and a nonlinear commercial model. Think
Google Play, Amazon or the Appstore. SingularityNet, Akira AI and Bonseyes are
multisided marketplace examples.
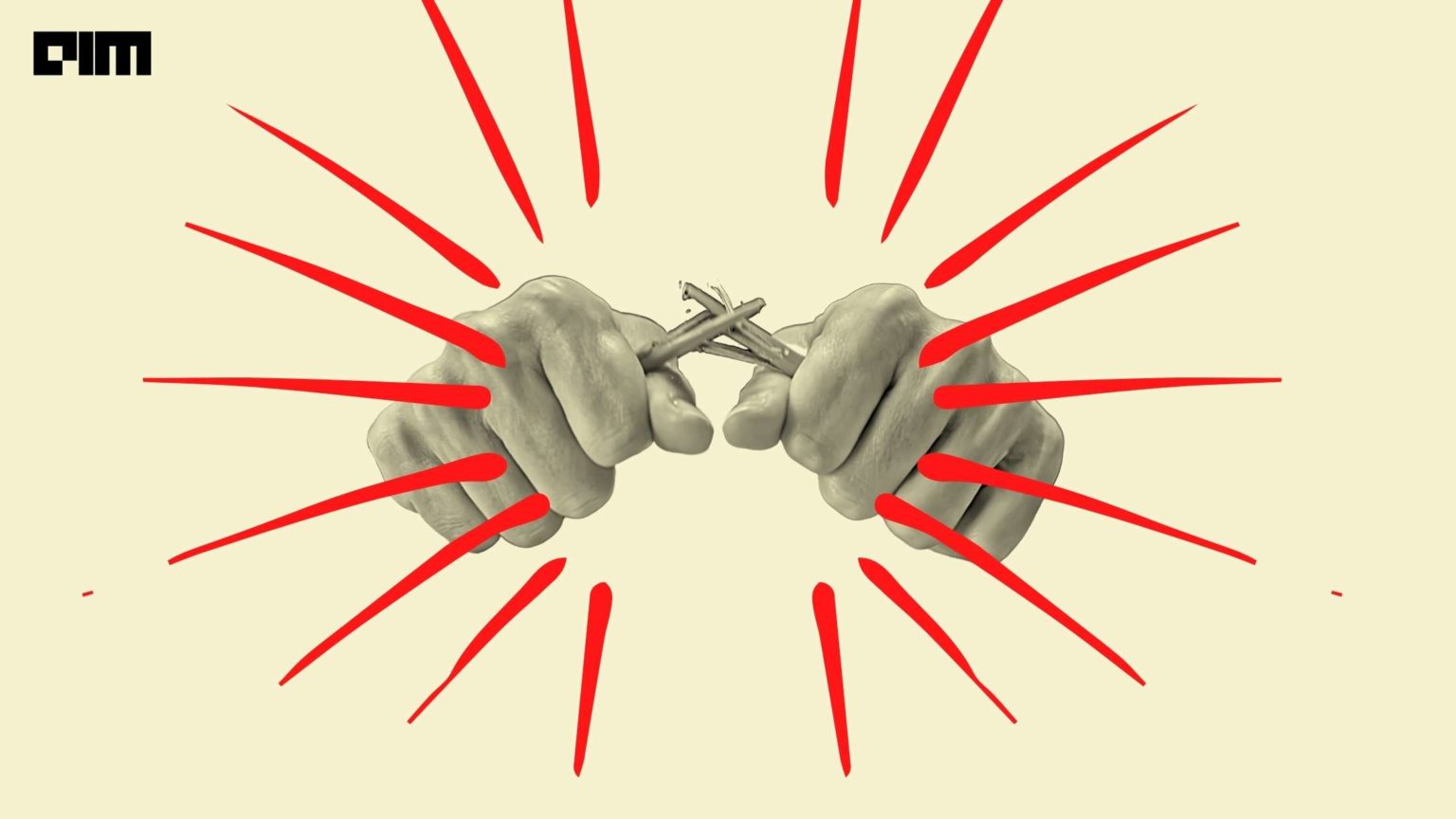
In the case of supervised learning, the AI systems are fed with labelled data.
But as we work with bigger models, it becomes difficult to label all the data.
Additionally, there is just not enough labelled data for a few tasks, such as
training translation systems for low-resource languages. In a 2020 AAAI
conference, Facebook’s chief AI scientist Yann LeCun introduced self-supervised
learning to overcome these challenges. This technique obtains a supervisory
signal from the data by leveraging the underlying structure. The general method
for self-supervised learning is to predict unobserved or hidden part of the
input. For example, in NLP, the words of a line are predicted using the
remaining words in the sentence. Since self-supervised learning uses the data
structure to learn, it can use various supervisory signals across large datasets
without relying on labels. A self-supervised learning system aims at creating a
data-efficient artificial intelligent system. It is generally referred to as
extension or even improvement over unsupervised learning methods. However, as
opposed to unsupervised learning, self-supervised learning does not focus on
clustering and grouping.

As trivial as it looks, the gigantic volume of blog posts, articles, books,
videos, tutorials, talks, slides and presentations, online courses, … are in
your service, most of them for FREE, to guide you in the direction you want to
go. Use them and use them often! Use these resources to not only learn new
skills but also to learn more about the differences between career paths in data
science- from product analysts, business analysts, statisticians, …-, get a
sense of the trends in data science and to figure out where you see yourself a
fit! Read consistently: data science is a vast field and the more you read and
learn, the more valuable you become for your future employer! Use your network
to connect to data scientists and speak with them about their roles,
experiences, projects, and a career path in analytics. Use your network to
connect to the opportunities you may not be aware of! Let them know you want to
transition to data science and you appreciate if they can help you along the
way. Use your network to find roles with an overlap between your roles,
responsibilites and skills and data science roles

Artificial intelligence, the technology that is seen as a home name today is
poised to become a transformational force in healthcare. Healthcare industry is
where a lot of challenges are encountered and opportunities open up. Starting
from chronic diseases and radiology to cancer and risk assessment, artificial
intelligence has shown its power by deploying precise, efficient, and impactful
interventions at exactly the right moment in a patient’s care. The complexity
and rise of data in healthcare have unveiled several types of artificial
intelligence. Today, artificial intelligence and robotics have evolved to the
stage where they can take better care of patients better than medical staff and
human caretakers. The global artificial intelligence in the healthcare market is
expected to grow from US$4.9 billion in 2020 and reach US$45.2 billion by 2026
with a projected CAGR of 44.9% during the forecast period. Artificial
intelligence and relevant technologies are prevalent in business and society and
are rapidly moving into the healthcare sector.
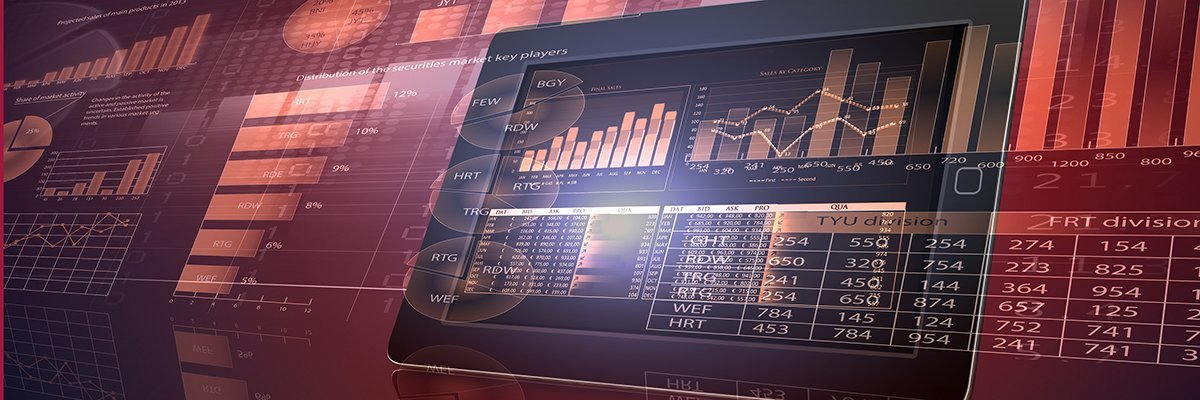
The fundamental architectural difference between Hadoop and Spark relates to how
data is organized for processing. In Hadoop, all the data is split into blocks
that are replicated across the disk drives of the various servers in a cluster,
with HDFS providing high levels of redundancy and fault tolerance. Hadoop
applications can then be run as a single job or a directed acyclic graph (DAG)
that contains multiple jobs. In Hadoop 1.0, a centralized JobTracker service
allocated MapReduce tasks across nodes that could run independently of each
other, and a local TaskTracker service managed job execution by individual
nodes. ... In Spark, data is accessed from external storage repositories, which
could be HDFS, a cloud object store like Amazon Simple Storage Service or
various databases and other data sources. While most processing is done in
memory, the platform can also "spill" data to disk storage and process it there
when data sets are too large to fit into the available memory. Spark can run on
clusters managed by YARN, Mesos and Kubernetes or in a standalone mode. Similar
to Hadoop, Spark's architecture has changed significantly from its original
design.
Personalized recommendation engines have been a mainstay of shopping for years.
There’s a folk legend in data mining circles, which claims Target has such
powerful data mining and analytics, it once recommended baby clothing to a girl
before she knew she was pregnant. Sadly, it’s just a myth, dating from a
hype-filled 2012 New York Times report. But while big data and AI use cases for
online shopping are still largely based in centralized data centers, a growing
number of use cases are seeing retailers embrace Edge computing and AI, both at
the Edge and in the cloud. Fulfillment centers are increasingly being used to
automate warehouses in order to speed up deliveries and optimize space, which
can make supply chains and logistics more efficient. In-store, robots are being
used to stack shelves and clean floors. Machine vision is being brought in to
scan shelves and manage inventory, suggest fashion ideas to customers, and in
the case of Amazon Go and other competitors, remove the need for cashiers and
traditional checkouts.

Designing for behavior change doesn’t require a specific product development
methodology—it is intended to layer on top of your existing approach, whether it
is agile, lean, Stage-Gate, or anything else. But to make things concrete,
Figure 4 shows how the four stages of designing for behavior change can be
applied to a simple iterative development process. At HelloWallet, we use a
combination of lean and agile methods, and this sample process is based on what
we’ve found to work. The person doing the work of designing for behavior change
could be any one of these people. At HelloWallet, we have a dedicated person
with a social science background on the product team (that’s me). But this work
can be, and often is, done wonderfully by UX folks. They are closest to the look
and feel of the product, and have its success directly in their hands. Product
owners and managers are also well positioned to seamlessly integrate the skills
of designing for behavior change to make their products effective. Finally,
there’s a new movement of behavioral social scientists into applied product
development and consulting at organizations like ideas42 and
IrrationalLabs.
A scenario-based analysis makes it easier to understand the risk, without a high
degree of technical jargon or acumen. The longstanding practices of safety
engineers can provide an excellent template for this kind of analysis. For
instance, by performing a hazard and operability (HAZOP) analysis process that
examines and manages risk as it relates to the design and operation of
industrial systems. One common method for performing HAZOPs is a process hazards
analysis (PHA) that uses specialized personnel to develop scenarios that would
result in an unsafe or hazardous condition. It is not a risk reduction strategy
that simply looks at individual controls, but considers more broadly how the
system works in unison and the different scenarios that could impact it.
Cybersecurity threats are the work of deliberate and thoughtful adversaries,
whereas safety scenarios often result from human or system error and failures.
As a result, a safety integrity level can be measured with some confidence by
failure rates, such as one every 10 years or 100 years.

Microsoft’s SQL server can store two types of spatial data, the so-called
geometry for two-dimensional environments and the geography for
three-dimensional parts of the world. The elements can be built out of simpler
points or lines or more complex curved sections. The company has also added a
set of geographic data formats and indexing to its cloud-based Azure Cosmos DB
NoSQL database. It is intended to simplify geographic analysis of your data set
for tasks such as computing store performance by location. Noted for a strong
lineage in geographic data processing, ESRI, the creator of ArcGIS, is also
expanding to offer cloud services that will first store geographic information
and then display it in any of the various formats the company pioneered. ESRI,
traditionally a big supplier to government agencies, has developed sophisticated
tools for rendering geographic data in a way that’s useful to fire departments,
city planners, health departments, and others who want to visualize how a
variety of data looks on a map. There is a rich collection of open source
databases devoted to curating geographic information.

Right now, the Internet of Things is more dolphin than human. Connections are
disparate and clunky, and connecting devices does not create automatic value
like connecting people. Intelligence has to be connected for the conjoining to
add value. But IoT is becoming more intelligent by the day. Edge computing—where
Moore’s law empowers each IoT sensor with the computing power to make
artificially intelligent decisions without relying on a central cloud
hub—creates this intelligence. In the words of Stan Lee, with great power comes
great responsibility. So we return to the question: Who controls IoT? In a world
with 86 billion devices, each equipped with on-the-edge intelligence, the answer
to this question concerns the future of humanity. IoT is notoriously fractured.
Countless use cases require domain expertise. As a result, no analogous winner
takes all to the internet where network effects anointed masters in search
(Google) and social (Facebook). According to Statista, at the end of 2019, there
were 620 IoT platforms, including tech behemoths Microsoft and Amazon.
Quote for the day:
"Real leaders are ordinary people with
extraordinary determinations." -- John Seaman Garns
No comments:
Post a Comment