Is Open Source More Secure Than Closed Source?

Open source software offers greater transparency to the teams that use it;
visibility into both the code itself and how it is maintained. Giving
organizations access to the source code allows them the opportunity to evaluate
the security of the code for themselves. Additionally, users have more
visibility into how and what changes are made to the code base, including the
pre-release review process, how often dependencies are updated and how
developers and organizations respond to security vulnerabilities. As a result,
open source software users have a more complete picture of the overall security
of the software they’re using. Another major benefit is found in the communities
which drive the growth and development of open source software. The vast
majority of open source software is backed by communities of forward-thinking
developers, many of whom use the same software they build and maintain as a
primary means of communicating with team members. Open source developers and the
communities around the software value users’ input to a significant degree, and
many user suggestions end up getting incorporated into new versions.
Let’s Not Regulate A.I. Out of Existence
A.I. is being used to analyze vast amounts of space data and is having an
enormous impact on health care. A.I. image and scan analysis are, for example,
helping doctors identify breast and colon cancer. It’s also showing potential in
vaccine creation. I guarantee that A.I. will someday save lives. It’s those
kinds of A.I.-driven data analysis that gets shoved aside by news of an A.I.
beating a world-champion GO player or the world’s best-known entrepreneur
raising alarms about a situation where “A.I. is vastly smarter than humans.”
That kind of fear-mongering leads consumers, who don’t understand the
differences between A.I. that scans a crowd of 10,000 faces for one suspect and
one that can create recipes based on pleasing ingredient combinations, to
mistrust all A.I., and to write the kind of stifling regulation produced by the
EU. Even if you still think the negatives outweigh the benefits, we’ll arguably
need better and bigger A.I. to manage and sift through the mountains of data we
produce every single day. To deny A.I.’s role in this is like saying we don’t
need garbage collection services and that our debris can just pile up on street
corners indefinitely.
AutoNLP: Automatic Text Classification with SOTA Models

AutoNLP is a tool to automate the process of creating end-to-end NLP models.
AutoNLP is a tool developed by the Hugging Face team which was launched in
its beta phase in March 2021. AutoNLP aims to automate each phase that makes up
the life cycle of an NLP model, from training and optimizing the model to
deploying it. “AutoNLP is an automatic way to train and deploy state-of-the-art
NLP models, seamlessly integrated with the Hugging Face ecosystem.” — AutoNLP
team One of the great virtues of AutoNLP is that it implements state-of-the-art
models for the tasks of binary classification, multi-class classification, and
entity recognition, supported in 8 languages which are: English, German,
French, Spanish, Finnish, Swedish, Hindi, and Dutch. Likewise, AutoNLP takes
care of the optimization and fine-tuning of the models. In the security and
privacy part, AutoNLP implements data transfers protected under SSL, also the
data is private to each user account. As we can see, AutoNLP emerges as a tool
that facilitates and speeds up the process of creating NLP models. In the next
section, will see how the experience was like from start to finish when creating
a text classification model using AutoNLP.
5 Reasons Why Artificial Intelligence Won’t Replace Physicians
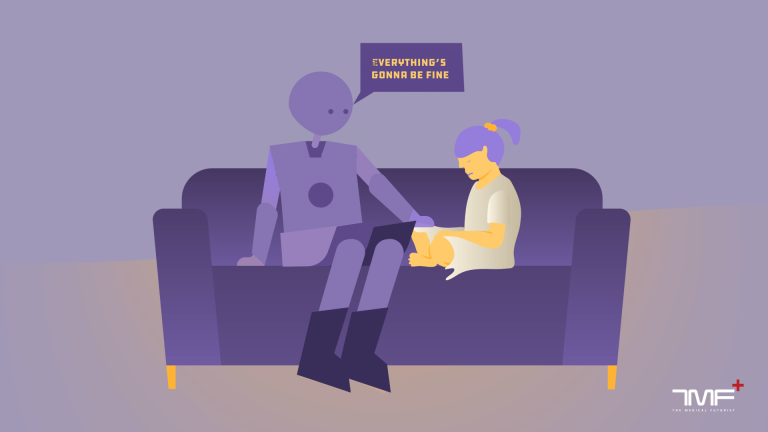
Even if the array of technologies offered brilliant solutions, it would be
difficult for them to mimic empathy. Why? Because at the core of compassion,
there is the process of building trust: listening to the other person, paying
attention to their needs, expressing the feeling of understanding and responding
in a manner that the other person knows they were understood. At present, you
would not trust a robot or a smart algorithm with a life-altering decision; or
even with a decision whether or not to take painkillers, for that matter. We
don’t even trust machines in tasks where they are better than humans – like
taking blood samples. We will need doctors holding our hands while telling us
about a life-changing diagnosis, their guide through therapy and their overall
support. An algorithm cannot replace that. ... More and more sophisticated
digital health solutions will require qualified medical professionals’
competence, no matter whether it’s about robotics or A.I. The human brain is so
complex and able to oversee such a vast scale of knowledge and data that it
merely is not worth developing an A.I. that takes over this job – the human
brain does it so well. It is more worthwhile to program those repetitive,
data-based tasks, and leave the complex analysis/decision to the person.
Mimicking the brain: Deep learning meets vector-symbolic AI
Machines have been trying to mimic the human brain for decades. But neither the
original, symbolic AI that dominated machine learning research until the late
1980s nor its younger cousin, deep learning, have been able to fully simulate
the intelligence it’s capable of. One promising approach towards this more
general AI is in combining neural networks with symbolic AI. In our paper
“Robust High-dimensional Memory-augmented Neural Networks” published in Nature
Communications, we present a new idea linked to neuro-symbolic AI, based on
vector-symbolic architectures. We’ve relied on the brain’s high-dimensional
circuits and the unique mathematical properties of high-dimensional spaces.
Specifically, we wanted to combine the learning representations that neural
networks create with the compositionality of symbol-like entities, represented
by high-dimensional and distributed vectors. The idea is to guide a neural
network to represent unrelated objects with dissimilar high-dimensional vectors.
In the paper, we show that a deep convolutional neural network used for image
classification can learn from its own mistakes to operate with the
high-dimensional computing paradigm, using vector-symbolic architectures.
How to master manufacturing's data and analytics revolution
The Manufacturing Data Excellence Framework, developed by a community of
companies hosted by the World Economic Forum’s Platform for Shaping the Future
of Advanced Manufacturing and Production, serves this purpose. We introduced
this framework, comprising 20 different dimensions with five different maturity
levels, in our recent white paper, “Data Excellence: Transforming manufacturing
and supply systems”. “One of the challenges we face when discussing the industry
transformation towards data ecosystems is the lack of commonality of
terminology. It’s very powerful to have a tool in which we have created common
definitions and explanations, and around which we can build the foundations
towards data sharing excellence in manufacturing,” says Niall Murphy, CEO and
Co-founder of EVRYTHNG. The first step is an assessment of the status quo using
the framework. Companies will be able to objectively assess their maturity in
implementing applications and technological and organizational enablers. They
will then be able to compare their individual maturity versus the benchmark and
define their individual target state.
Ethics of AI: Benefits and risks of artificial intelligence
Ethical issues take on greater resonance when AI expands to uses that are far
afield of the original academic development of algorithms. The industrialization
of the technology is amplifying the everyday use of those algorithms. A report
this month by Ryan Mac and colleagues at BuzzFeed found that "more than 7,000
individuals from nearly 2,000 public agencies nationwide have used technology
from startup Clearview AI to search through millions of Americans' faces,
looking for people, including Black Lives Matter protesters, Capitol
insurrectionists, petty criminals, and their own friends and family members."
Clearview neither confirmed nor denied BuzzFeed's' findings. New devices are
being put into the world that rely on machine learning forms of AI in one way or
another. For example, so-called autonomous trucking is coming to highways, where
a "Level 4 ADAS" tractor trailer is supposed to be able to move at highway speed
on certain designated routes without a human driver. A company making that
technology, TuSimple, of San Diego, California, is going public on Nasdaq. In
its IPO prospectus, the company says it has 5,700 reservations so far in the
four months since it announced availability of its autonomous driving software
for the rigs.
Dale Vince has a winning strategy for sustainability
Fundamentally, it’s more economic to do the right thing than the wrong thing.
Renewable energy, for example, is a great democratizing force in world affairs
because the wind and the sun are available to every country on the planet,
whereas oil and gas are not. We fight wars over oil and gas quite literally
because it’s such a precious resource. And here in Britain, we spend £55
billion [US$76 billion] every year buying fossil fuels from abroad to bring
them here to burn them. And if we spent that money on wind and solar machines
instead, we could make our own electricity, create jobs, and be independent
from fluctuating global fossil fuel markets and currency exchanges. We can
create a stronger, more resilient economy, as well as a cleaner one. ... I
think businesses historically reinvent themselves. They move with the times or
they die, and that’s a natural order of things. And some businesses just get
left behind because their business model becomes outdated. A nimble, adaptive
business will move from the old way of doing things and will still be
here.
A Deeper Dive into the DOL’s First-of-Its-Kind Cybersecurity Guidance
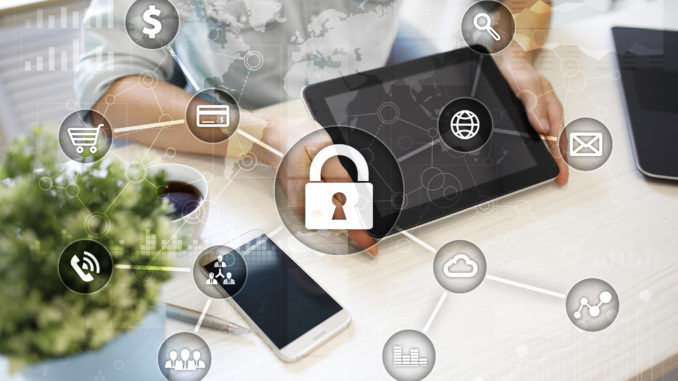
ERISA’s duty of prudence requires fiduciaries to act “with the care, skill,
prudence, and diligence under the circumstances then prevailing that a prudent
man acting in a like capacity and familiar with such matters would use in the
conduct of an enterprise of a like character and with like aims.” It has
become generally accepted that ERISA fiduciaries have some responsibility to
mitigate the plan’s exposure to cybersecurity events. But, prior to this
guidance, it was not clear what the DOL considered to be prudent with respect
to addressing cybersecurity risks associated, including those related to
identity theft and fraudulent withdrawals. Each of the three new pieces of
guidance addresses a different audience. The first, Tips for Hiring a Service
Provider with Strong Cybersecurity Practices (Tips for Hiring a Service
Provider), provides guidance for plan fiduciaries when hiring a service
provider, such as a recordkeeper, trustee, or other provider that has access
to a plan’s nonpublic information. The second, Cybersecurity Program Best
Practices (Cybersecurity Best Practices), is, as the name indicates, a
collection of best practices for recordkeepers and other service providers,
and may be viewed as a reference for plan fiduciaries when evaluating service
providers’ cybersecurity practices. The third, Online Security Tips (Online
Security Tips), contains online security advice for plan participants and
beneficiaries. We have summarized each piece of guidance below along with our
key observations.
Less complexity, more control: The role of multi-cloud networking in digital transformation
The panellists agreed that it means going back to layer by layer design
principles with clean APIs up and down the protocol stack from application to
the lowest levels of connectivity. Without such design rigour, programming or
operator errors in a complex highly distributed system could have profound
consequences. Cisco’s Pandey says that while it appeared “horribly scary” in
terms of connectivity to take monolithic apps and make them cloud-native, the
upside is that the resulting discrete components of the application can be
swapped out or taken down with fewer consequences to the rest of the system
and ultimately to customers. But, he warned, “you need to have the tools and
capabilities to monitor it – the full-stack observability piece. You need to
have discoverability and you need to have security at the API layer all the
way down so that you can manage things properly”. His comments were echoed by
Alkira’s Khan, who pointed out that the problems of a distributed architecture
are particularly acute for enterprises trying to apply a security posture in a
multi-cloud environment.
Quote for the day:
"It is the responsibility of
leadership to provide opportunity, and the responsibility of individuals to
contribute." - William Pollard
No comments:
Post a Comment