A New Era of Adobe Document Cloud and Acrobat DC
PDF started out as a noun, but quickly became a verb. The adjective “portable,” an essential descriptor in the pre-internet era of 1993, doesn’t begin to capture all the rich capabilities of today’s PDF. The PDF is still portable of course, but it’s also editable, reviewable, reliable and sign-able. And it’s universal: in the last year alone, some 200 billion PDFs were opened in Adobe products alone. And we’re accelerating the pace of innovation in PDF and the role it will play for future generations. Today, we’re announcing major advancements in Adobe Document Cloud and Acrobat DC, redefining what’s possible with PDF. This launch includes new PDF share and review services, dramatic enhancements to touch-enabled editing on tablets, and a redesigned way to send documents for signature with Adobe Sign, all built in to Acrobat DC. It’s all about making it easier to create, share and interact with PDFs, wherever you are. New Adobe Sensei-powered functionality automates repetitive tasks and saves time, whether you’re scanning a document, filling out a form or signing an agreement.
Establishing an AI code of ethics will be harder than people think
In an attempt to highlight how divergent people’s principles can be, researchers at MIT created a platform called the Moral Machine to crowd-source human opinion on the moral decisions that should be followed by self-driving cars. They asked millions of people from around the world to weigh in on variations of the classic "trolley problem" by choosing who a car should try to prioritize in an accident. The results show huge variation across different cultures. Establishing ethical standards also doesn’t necessarily change behavior. In June, for example, after Google agreed to discontinue its work on Project Maven with the Pentagon, it established a fresh set of ethical principles to guide its involvement in future AI projects. Only months later, many Google employees feel those principles have been placed by the wayside with a bid for a $10 billion Department of Defense contract. A recent study out of North Carolina State University also found that asking software engineers to read a code of ethics does nothing to change their behavior.
Quantum computing: A cheat sheet

Using quantum computation, mathematically complex tasks that are at present typically handled by supercomputers — protein folding, for example — can theoretically be performed by quantum computers at a lower energy cost than transistor-based supercomputers. While current quantum machines are essentially proof-of-concept devices, the algorithms which would be used on production-ready machines are being tested presently, to ensure that the results are predictable and reproducible. At the current stage of development, a given problem can be solved by both quantum and traditional (binary) computers. As manufacturing processes used to build quantum computers is refined, it is anticipated that they will become faster at computational tasks than traditional, binary computers. Further, quantum supremacy is the threshold at which quantum computers are theorized to be capable of solving problems, which traditional computers would not (practically) be able to solve. Practically speaking, quantum supremacy would provide a superpolynomial speed increase over the best known (or possible) algorithm designed for traditional computers.
The Untapped Potential in Unstructured Text
Unstructured text is the largest human-generated data source, and it grows exponentially every day. The free-form text we type on our keyboards or mobile devices is a significant means by which humans communicate our thoughts and document our efforts. Yet many companies don’t tap into the potential of their unstructured text data, whether it be internal reports, customer interactions, service logs or case files. Decision makers are missing opportunities to take meaningful action around existing and emerging issues. ... Natural language processing (NLP) is a branch of artificial intelligence (AI) that helps computers understand, interpret and manipulate human language. In general terms, NLP tasks break down language into shorter, elemental pieces, and tries to understand relationships among those pieces to explore how they work together to create meaning. The combination of NLP, machine learning and human subject matter expertise holds the potential to revolutionize how we approach new and existing problems.
How AI Is Shaking Up Banking and Wall Street
San Francisco–based Blend, for one, provides its online mortgage-application software to 114 lenders, including lending giant Wells Fargo, shaving at least a week off the approval process. Could it have prevented the mortgage meltdown? Maybe not entirely, but it might have lessened the severity as machines flagged warning signs sooner. “Bad decisions around data can be found instantaneously and can be fixed,” says Blend CEO and cofounder Nima Ghamsari. While banks are not yet relying on A.I. for approval decisions, lending executives are already observing a secondary benefit of the robotic process: making home loans accessible to a broader swath of America. Consumers in what Blend defines as its lowest income bracket—a demographic that historically has shied away from applying in person—are three times as likely as other groups to fill out the company’s mobile application. Says Mary Mack, Wells Fargo’s consumer banking head: “It takes the fear out.”
The Hidden Risks of Unreliable Data in IIoT
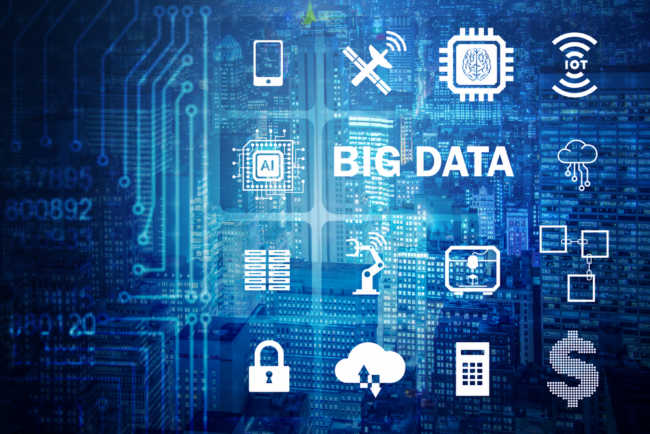
One of the key goals of Industry 4.0 is business optimization, whether it’s from predictive maintenance, asset optimization, or other capabilities that drive operational efficiencies. Each of these capabilities is driven by data, and their success is dependent on having the right data at the right time fed into the appropriate models and predictive algorithms. Too often data analysts find that they are working with data that is incomplete or unreliable. They have to use additional techniques to fill in the missing information with predictions. While techniques such as machine learning or data simulations are being promoted as an elixir to bad data, they do not fix the original problem of the bad data source. Additionally, these solutions are often too complex, and cannot be applied to certain use cases. For example, there are no “data fill” techniques that can be applied to camera video streams or patient medical data. Any data quality management effort should start with collecting data in a trusted environment.
AI can’t replace doctors. But it can make them better.

It’s not that we don’t have the data; it’s just that it’s messy. Reams of data clog the physician’s in-box. It comes in many forms and from disparate directions: objective information such as lab results and vital signs, subjective concerns that come in the form of phone messages or e-mails from patients. It’s all fragmented, and we spend a great deal of our time as physicians trying to make sense of it. Technology companies and fledging startups want to open the data spigot even further by letting their direct-to-consumer devices—phone, watch, blood-pressure cuff, blood-sugar meter—send continuous streams of numbers directly to us. We struggle to keep up with it, and the rates of burnout among doctors continue to rise. How can AI fix this? Let’s start with diagnosis. While the clinical manifestations of asthma are easy to spot, the disease is much more complex at a molecular and cellular level. The genes, proteins, enzymes, and other drivers of asthma are highly diverse, even if their environmental triggers overlap.
4 steps for solution architects to overcome RPA challenges

As important as it is for solution architects to talk about the technology, it’s equally crucial that they listen to concerns too. Especially when discussing automation – or even just mentioning the word robotics, architects must realize it’s natural for some groups to have anxiety about being replaced or their positions eliminated. This belief often stems from a lack of education about the technology. In times like these, solution architects need to understand where clients are coming from and leverage the questions as opportunities to communicate the real benefit of RPA: which is to make employee lives easier by removing tedious tasks like data entry that most workers don’t enjoy. Managers also need to know that this level of automation will likely result in greater trust in the accuracy of the data, which is something that can’t be easily assessed when humans are doing the data extraction and entry. Overcoming executives’ fears about job security is vital to a successful implementation, because with better client education, comes more comfort with RPA
Feds Charge Russian With Midterm Election Interference
It's unclear how prosecutors zeroed in on Khusyaynova, who presumably remains in Russia. The U.S. and Russia do not have an extradition treaty, which means she can likely avoid arrest indefinitely, provided she remains there. The complaint against Khusyaynova contains a surprising amount of detail about how Project Lakhta was funded and its finances managed. The level of detail suggests that investigators managed to work with a source who was part of or closely affiliated with the project. The complaint describes financial documents, emails and paperwork that allegedly lay bare the source of the project's funding and its aims. The Justice Department alleges Project Lakhta was funded and ultimately controlled by a trio of companies operating under the name of Concord, run by Yevgeniy Viktorovich Prigozhin, described in the complaint as being "a Russian oligarch who is closely identified with Russian President Vladimir Putin."
The OSI model explained: How to understand the 7 layer network model
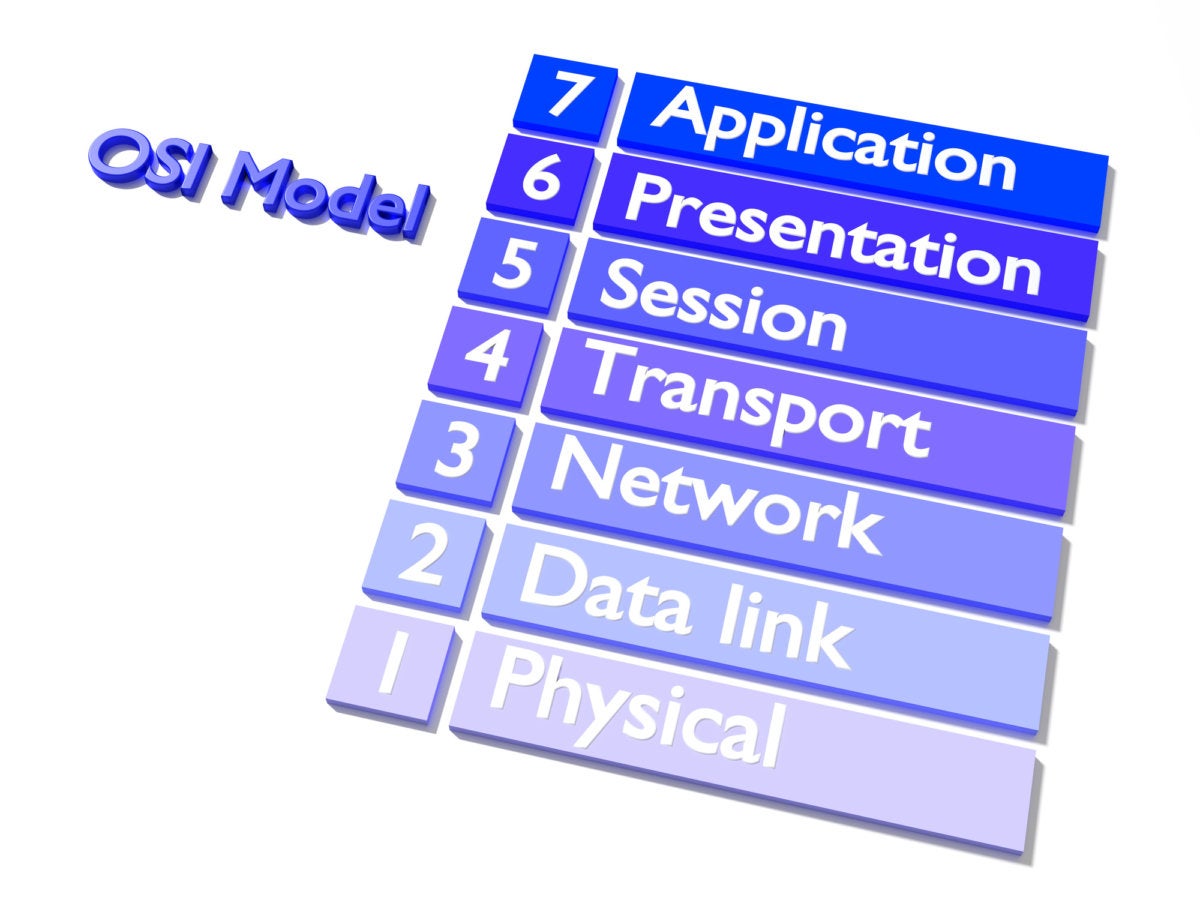
For IT professionals, the seven layers refer to the Open Systems Interconnection (OSI) model, a conceptual framework that describes the functions of a networking or telecommunication system. The model uses layers to help give a visual description of what is going on with a particular networking system. This can help network managers narrow down problems (Is it a physical issue or something with the application?), as well as computer programmers (when developing an application, which other layers does it need to work with?). Tech vendors selling new products will often refer to the OSI model to help customers understand which layer their products work with or whether it works “across the stack”. Conceived in the 1970s when computer networking was taking off, two separate models were merged in 1983 and published in 1984 to create the OSI model that most people are familiar with today. Most descriptions of the OSI model go from top to bottom, with the numbers going from Layer 7 down to Layer 1.
Quote for the day:
"Have courage. It clears the way for things that need to be." -- Laura Fitton
No comments:
Post a Comment