AI experts question tech industry’s ethical commitments
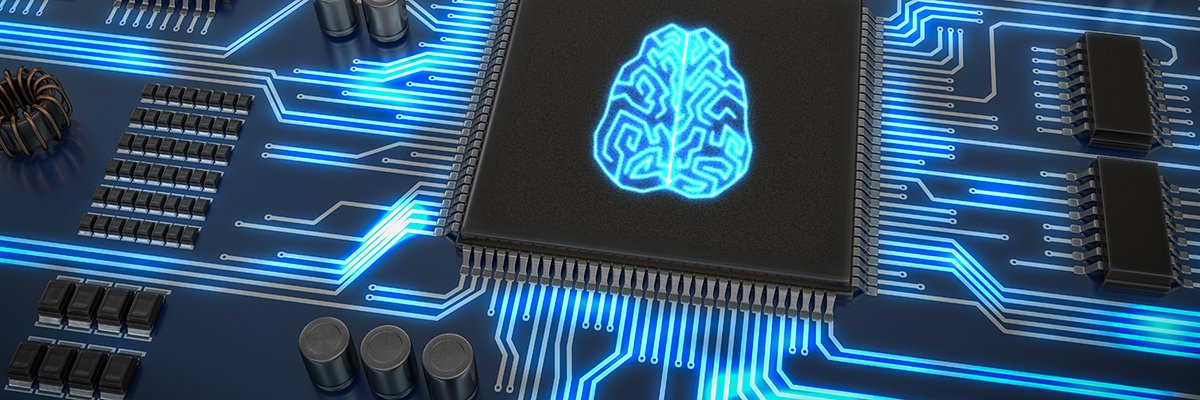
For Wachter, the “cutting costs and saving time” mindset that permeates AI’s
development and deployment has led practitioners to focus almost exclusively
on correlation, rather than causation, when building their models. “That
spirit of making something quick and fast, but not necessarily improving it,
also translates into ‘correlation is good enough – it gets the job done’,” she
says, adding that the logic of austerity that underpins the technology’s
real-world use means that the curiosity to discover the story between the data
points is almost entirely absent. “We don’t actually care about the causality
between things,” says Wachter. “There is an intellectual decline, if you will,
because the tech people don’t really care about the social story between the
data points, and social scientists are being left out of that loop.” She adds:
“Really understanding how AI works is actually important to make it fairer and
more equitable, but it also costs more in resources. There is very little
incentive to figure out what is going on [in the models].” Taking the point
further, McQuillan describes AI technology as a “correlation machine” that, in
essence, produces conspiracy theories.
The Next Generation of Supply Chain Attacks Is Here to Stay
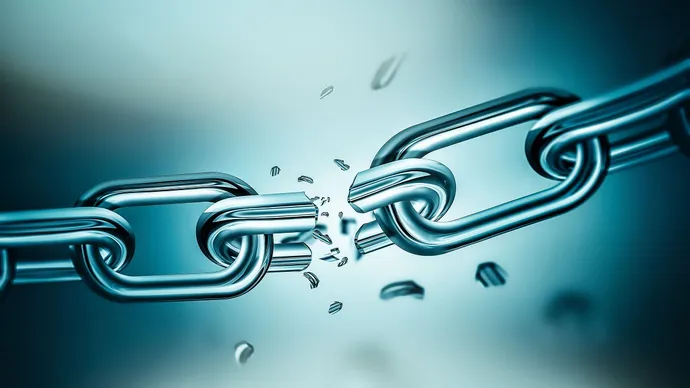
As the vast majority of the workforce has gone digital, organizations' core
systems have been moving to the cloud. This accelerated cloud adoption has
exponentially increased the use of third-party applications and the
connections between systems and services, unleashing an entirely new
cybersecurity challenge. There are three main factors that lead to the rise in
app-to-app connectivity: Product-led growth (PLG): In an era of PLG and
bottom-up software adoption, with software-as-a-service (SaaS) leaders like
Okta and Slack; DevOps: Dev teams are freely generating and embedding API keys
in. Hyperautomation: The rise of hyperautomation and low code/no code
platforms means "citizen developers" can integrate and automate processes with
the flip of a switch. The vast scope of integrations are now easily accessible
to any kind of team, which means time saved and increased productivity. But
while this makes an organization's job easier, it blurs visibility into
potentially vulnerable app connections, making it extremely difficult for
organizational IT and security leaders to have insight into all of the
integrations deployed in their environment, which expands the organization's
digital supply chain.
Cultivating social emotional learning in the metaverse

Interactions and learning trigger feelings and emotions. There is a need to
develop emotional awareness, to pause and notice the emotional signals of the
body. The practice of pause – the conscious allotting of space and time to
look inwards and notice physical sensations like a ‘racing pulse’, a ‘shaking
leg’ or a ‘clammy hand’ is a must for well-being. When things seem to be
falling apart, it is useful to breathe. Evidence suggests that, by counting
our breaths and centring our breathing, we calm our minds. Whether dealing
with difficult conversations with colleagues, family, friends, teachers or
students, the ability to regulate emotion and attention is a well-being
practice proven to mitigate accompanying anxiety, fear, anger or despair. ...
Feeling a pit in one’s stomach or a thumping heart are physical symptoms that
often accompany intense emotional responses. At such times, a friend; app;
conscious trained practice like counting numbers, breaths or tiles on the
floor; time-out or break; or walking can all be good ways to physically
distract focus and allow some of the intensity of the emotion to diminish.
How Much Automation Is Too Much?
For many forms of automation, deskilling isn’t a serious problem. Knowledge
workers in general, including ops personnel, may face many routine, repeatable
tasks in their day-to-day work that don’t require a level of skill that would
cause an issue if that skill were lost. All such routine tasks are subject to
automation without concern. At the other extreme, organizations may aspire to
"lights out" production environments, so fully automated that there’s no
reason to keep the lights on, because there are no people on duty. Any
organization with such a lights out environment is likely to lose any staff
who might be able to fix something if it goes wrong, either via deskilling or
attrition. As AI-based automation becomes increasingly sophisticated,
therefore, organizations will reach some optimal point where the advantages of
automation sufficiently balance any disadvantages. Finding this optimum
depends upon the people involved — the skilled workers who must somehow
accommodate automation in their day-to-day work. Be sure to listen to the
senior-level people who are adept at analogizing. They can solve problems that
automation will never be able to solve.
Bringing a Product Mindset into DevOps
/filters:no_upscale()/articles/product-mindset-devops/en/resources/22%20product%20market%20fit-1668435929840.jpeg)
A product mindset is about delivering things that provide value to our
users, within the context of the organisation and their strategy, and do so
sustainability (i.e. balancing the now and the future). For the purpose of
this article, I will use product thinking, product mindset and product
management very much interchangeably. ... In practice this means achieving
product-market-fit by balancing what our users need, want and find valuable
(desirability), what we need to achieve (and can afford) as an organisation
(viability) and what is possible technically, culturally, legally, etc
(feasibility), and doing this without falling into the trap of premature
optimisation or closing options too early. To give a tiny, very specific,
but quite telling example: for the medical device organisation we chose Bash
as scripting language because the DevOps lead was comfortable with it.
Eventually we realised that the client’s engineers had no Bash experience,
but as a .Net shop were far more comfortable with Python. Adding a
user-centric approach which is part of a product mindset at an early stage
would have prevented this mistake and the resulting rework.
Solving brain dynamics gives rise to flexible machine-learning models

Last year, MIT researchers announced that they had built “liquid” neural
networks, inspired by the brains of small species: a class of flexible,
robust machine learning models that learn on the job and can adapt to
changing conditions, for real-world safety-critical tasks, like driving and
flying. The flexibility of these “liquid” neural nets meant boosting the
bloodline to our connected world, yielding better decision-making for many
tasks involving time-series data, such as brain and heart monitoring,
weather forecasting, and stock pricing. But these models become
computationally expensive as their number of neurons and synapses increase
and require clunky computer programs to solve their underlying, complicated
math. And all of this math, similar to many physical phenomena, becomes
harder to solve with size, meaning computing lots of small steps to arrive
at a solution. Now, the same team of scientists has discovered a way to
alleviate this bottleneck by solving the differential equation behind the
interaction of two neurons through synapses to unlock a new type of fast and
efficient artificial intelligence algorithms.
4 Examples of Microservices Architectures Done Right
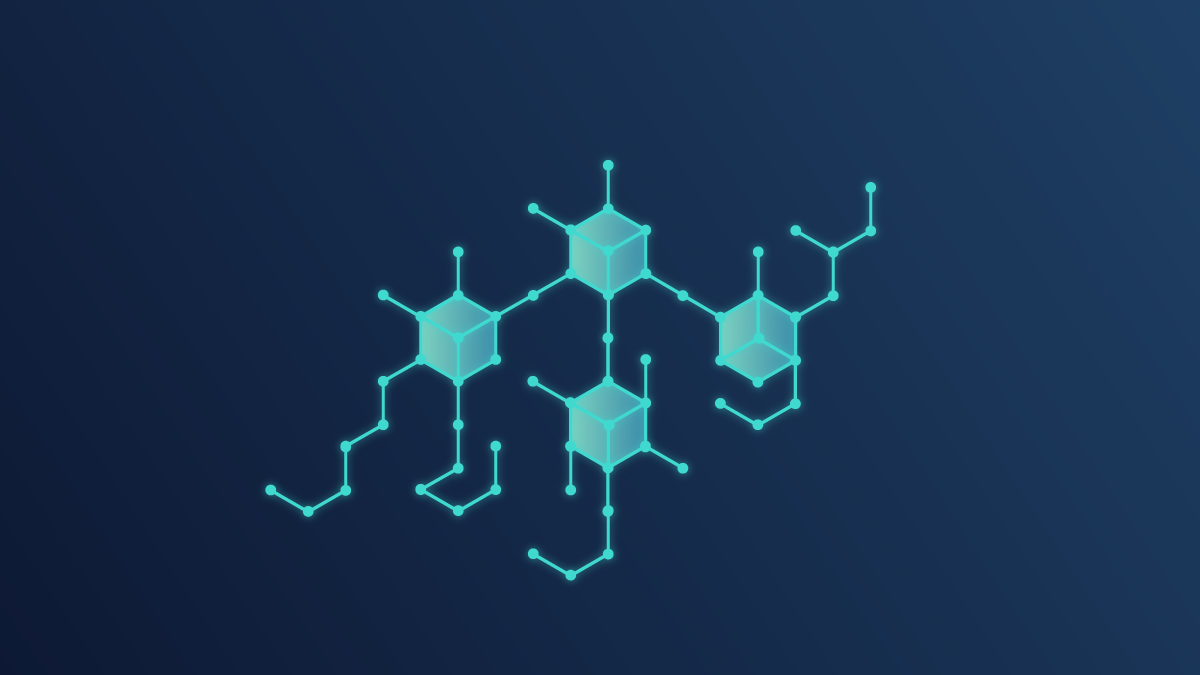
Microservices are everywhere in today’s increasingly virtual, decentralized
world. 85% of organizations with 5,000 or more employees use microservices
in their organization in some capacity as of 2021. Even more tellingly, 0%
report having no intention of adopting microservices in their company.
Clearly, microservices are here to stay, meaning more and more businesses
will be adopting microservices in the coming months and years. This is good
news, as microservices are capable of so much. This popularity comes with
its own risks, though. Some businesses that integrate microservices into
their existing workflow will need help figuring out what to do with them.
... Uber would not be able to exist if not for microservices. Although very
brief, their monolithic structure resulted in insurmountable hurdles to
their growth. Without microservices, the ride-sharing app couldn’t fix bugs
when they occurred, develop or launch new services, or transition to a
global marketplace. Their monolithic structure was prohibitively complex,
requiring developers to have extensive experience working with the system to
make even simple changes.
Chief engineering officer: A day in the life

It’s easy to get caught up in the tactical work, so I make sure to reserve
time for high-level plans and strategy. This means following tech news and
blogs to stay abreast of the latest in technology trends, keeping an eye on
market news, reading the latest analyst research, meeting ups with my peers
in our private equity portfolio companies, and more. It’s important for me
not just to be on top of the technology, but to understand where we’re
taking the business in the future. This kind of thinking is what helped lead
to Gartner positioning Boomi as a Leader in the 2021 Gartner Magic Quadrant
for Enterprise Integration Platform as a Service (EiPaaS) for eight
consecutive years. ... If you’re thinking of getting into software
engineering, here’s my advice: Just do it. It’s a high-demand career, and
there is a continual lack of strong talent in the industry. You’ll find
almost limitless opportunities once you get started. And there has never
been a better time to do so, with many technological advancements that have
lowered barriers to entry. As you move into management, though, it’s
important to remember that your job is no longer to code – it’s to satisfy
customer requirements and meet business goals.
5 unexpected ways to improve your architecture team

A key consideration for large organizations is ensuring that no major
component can function with complete autonomy or in a silo. You don't want a
squad to be incapable of getting things done without any input from other
squads. Quite the contrary, for minor decisions or implementations, each
squad is empowered to move as quickly as possible. For major decisions with
little or no recourse to make changes later, collaboration is key to the
"measure twice, cut once" approach to critical decision-making. This
enforced "checks and balances" means that no one chapter can unilaterally
stray too far outside our strategic bounds. At a lower level, the Delivery
Management squad team members work within other squads but all report to the
same manager. Embedding them within other teams helps make every squad's
activities as consistent as possible. This allows minimal impact on sprints
when, for example, a delivery manager is out on leave, because process
alignment is a goal within the Delivery Management function.
Why companies can no longer hide keys under the doormat
CIOs need to be asking questions to their teams to assess this potential
exposure and understand the risk, as well as putting plans in place to
address it. Fortunately, recent breakthroughs have been able to eliminate
this encryption gap and maintain full protection for private keys. Leading
CPU vendors have added security hardware within their advanced
microprocessors that prevents any unauthorized access to code or data during
execution or afterwards in what remains in memory caches. The chips are now
in most servers, particularly those used by public cloud vendors, involving
a technology generally known as confidential computing. This “secure
enclave” technology closes the encryption gap and protects private keys, but
it has required changes to code and IT processes that can involve a
significant amount of technical work. It is specific to a particular cloud
provider (meaning it must be altered for use in other clouds) and
complicates future changes in code or operational processes. Fortunately,
new “go-between” technology eliminates the need for such modifications and
potentially offers multi-cloud portability with unlimited scale.
Quote for the day:
"The leadership team is the most important asset of the company and can be
its worst liability" -- Med Jones
No comments:
Post a Comment