Power With Purpose: The Four Pillars Of Leadership

A leader is defined by a purpose that is bigger than themselves. When that
purpose serves a greater good, it becomes the platform for great leadership.
Gandhi summed up his philosophy of life with these words: “My life is my
message.” That one statement speaks volumes about how he chose to live his life
and share his message of non-violence, compassion, and truth with the world.
When you have a purpose that goes beyond you, people will see it and identify
with it. Being purpose-driven defines the nobility of one’s character. It
inspires others. At its core, your leadership purpose springs from your
identity, the essence of who you are. Purpose is the difference between a
salesman and a leader, and in the end, the leader is the one that makes the
impact on the world. ... The earmark of a great leader is their care and concern
for their people. Displaying compassion towards others is not about a photo-op,
but an inherent characteristic that others can feel and hear when they are with
you. It lives in the warmth and timbre of your voice. It shows in every action
you take. Caring leaders take a genuine interest in others.
Using GPUs for Data Science and Data Analytics
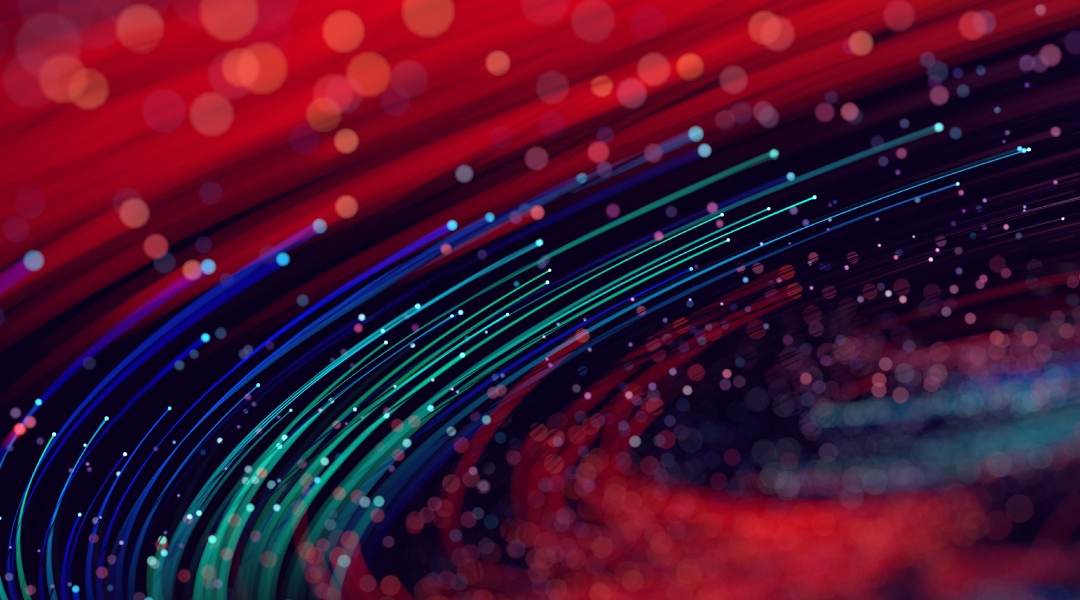
It is now well established that the modern AI/ML systems’ success has been
critically dependent on their ability to process massive amounts of raw data in
a parallel fashion using task-optimized hardware. Therefore, the use of
specialized hardware like Graphics Processing Units (GPUs) played a significant
role in this early success. Since then, a lot of emphasis has been given to
building highly optimized software tools and customized mathematical processing
engines (both hardware and software) to leverage the power and architecture of
GPUs and parallel computing. While the use of GPUs and distributed computing is
widely discussed in the academic and business circles for core AI/ML tasks (e.g.
running a 100-layer deep neural network for image classification or
billion-parameter BERT speech synthesis model), they find less coverage when it
comes to their utility for regular data science and data engineering tasks.
These data-related tasks are the essential precursor to any ML workload in an AI
pipeline and they often constitute a majority percentage of the time and
intellectual effort spent by a data scientist or even an ML engineer.
The Use of Deep Learning across the Marketing Funnel

Simply put, Deep Learning is an ML technique where very large Neural networks
are used to learn from the large quantum of data and deliver highly accurate
outcomes. The more the data, the better the Deep Learning model learns and the
more accurate the outcome. Deep Learning is at the centre of exciting innovation
possibilities like Self Driven Cars, Image recognition, virtual assistants,
instant audio translations etc. The ability to manage both structured and
unstructured data makes this a truly powerful technology advancement. ...
Differentiation not only comes from product proposition and comms but also how
consumers experience the brand/service online. And here too strides in Deep
Learning are enabling marketers with more sophisticated ways to create
differentiation. Website Experience: Based on the consumer profile and cohort,
even the website experience can be customized to ensure that a customer gets a
truly relevant experience creating more affinity for the brand/service. A great
example of this is Netflix where no 2 users have a similar website experience
based on their past viewing of content.
Navigating the 2021 threat landscape: Security operations, cybersecurity maturity
When it comes to cybersecurity teams and leadership, the report findings
revealed no strong differences between the security function having a CISO or
CIO at the helm and organizational views on increased or decreased cyberattacks,
confidence levels related to detecting and responding to cyberthreats or
perceptions on cybercrime reporting. However, it did find that security function
ownership is related to differences regarding executive valuation of cyberrisk
assessments (84 percent under CISOs versus 78 percent under CIOs), board of
director prioritization of cybersecurity (61% under CISOs versus 47% under CIOs)
and alignment of cybersecurity strategy with organizational objectives (77%
under CISOs versus 68% under CIOs). The report also found that artificial
intelligence (AI) is fully operational in a third of the security operations of
respondents, representing a four percent increase from the year before.
Seventy-seven percent of respondents also revealed they are confident in the
ability of their cybersecurity teams to detect and respond to cyberthreats, a
three-percentage point increase from last year.
Don’t become an Enterprise/IT Architect…

The world is somewhere on the rapid growth part of the S-curve of the
information revolution. It is at the point that the S-curve is going into
maturity that speed of change slows down. It is at that point that the gap
between expectation of change capacity by upper management and the reality
of the actual capacity for change is going to increase. And Enterprise/IT
Architects–Strategists are amongst other things tasked with bridging that
worsening gap. Which means that — for Enterprise/IT Architects–Strategists
and many more people who actually are active in shaping that digital
landscape — as long as there is no true engagement of top management, the
gap between them and their upper management looks like this red curve, which
incidentally also represents how enjoyable/frustrating EA-like jobs are ...
We’re in the period of rapid growth, the middle of the blue curve. That is
also the period where (IT-related, which is much) change inside
organisations (and society) gets more difficult every day, and thus
noticeably slows down. Well run and successful projects that take more than
five years are no exception.
A Journey in Test Engineering Leadership: Applying Session-Based Test Management
Testing is a complex activity, just like software engineering or any craft
that takes study, critical thinking and commitment. It is not possible to
encode everything that happens during testing into a document or artifact
such as a test case. The best we can do is report our testing in a way that
tells a compelling and informative story about risk to people who matter,
i.e. those making the decisions about the product under test. ... SBTM is a
kind of activity-based test management method, which we organize around test
sessions. The method focuses on the activities testers perform during
testing. There are many activities that testers perform outside of testing,
such as attend meetings, help developers troubleshoot problems, attend
training, and so on. Those activities don’t belong in a test session. To
have an accurate picture of only the testing performed and the duration of
the testing effort, we package test activity into sessions.
Beware of blind spots in data centre monitoring

The answer is to combine the tools that tell you about the past and present
states, with a tool that will shine a light on how the environment will
behave in the future. Doing this requires the use of Computational Fluid
Dynamics (CFD). A virtual simulation of an entire data center, CFD-based
simulation enables operators to accurately calculate the environmental
conditions of the facility. Virtual sensors, for instance, ensure the
simulated data reflects the sensor data. Consequently, the results can be
used to investigate conditions anywhere you want, in fine detail. CFD also
extends beyond temperature maps and includes humidity, pressure and air
speed. Airflow, for instance, can be traced to show how it is getting from
one place to another, offering unparalleled insight into the cause of
thermal challenges. Critically, CFD enables operators to simulate future
resilience. A validated CFD model will offer information about any
configuration of your data centre, simulating variations in current
configurations, or in new ones you haven’t yet deployed.
SolarWinds CEO Talks Securing IT in the Wake of Sunburst
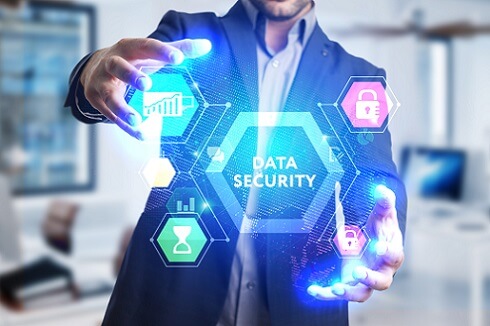
Specific to the pandemic, a lot of technologies, endpoint security, cloud
security, and zero trust, which have proliferated after the pandemic --
organizations have changed how they talk about how they are deploying these.
Previously there may have been a cloud security team and an infrastructure
security team, very soon the line started getting blurred. There was very
little need for network security because not many people were coming to
work. It had to be changed in terms of organization, prioritization, and
collaboration within the enterprise to leverage technology to support this
kind of workforce. ... Every team has to be constantly vigilant about what
might be happening in their environment and who could be attacking them. The
other side of it is constant learning. You constantly demonstrate awareness
and vigilance and constantly learn from it. The red team can be a very
effective way to train an entire organization and sensitize them to let’s
say a phishing attack. As common as phishing attacks are, a large majority
of people, including in the technology sectors, do not know how to fully
prevent them despite the fact there are lot of phishing [detection]
technology tools available.
Is your network AI as smart as you think?
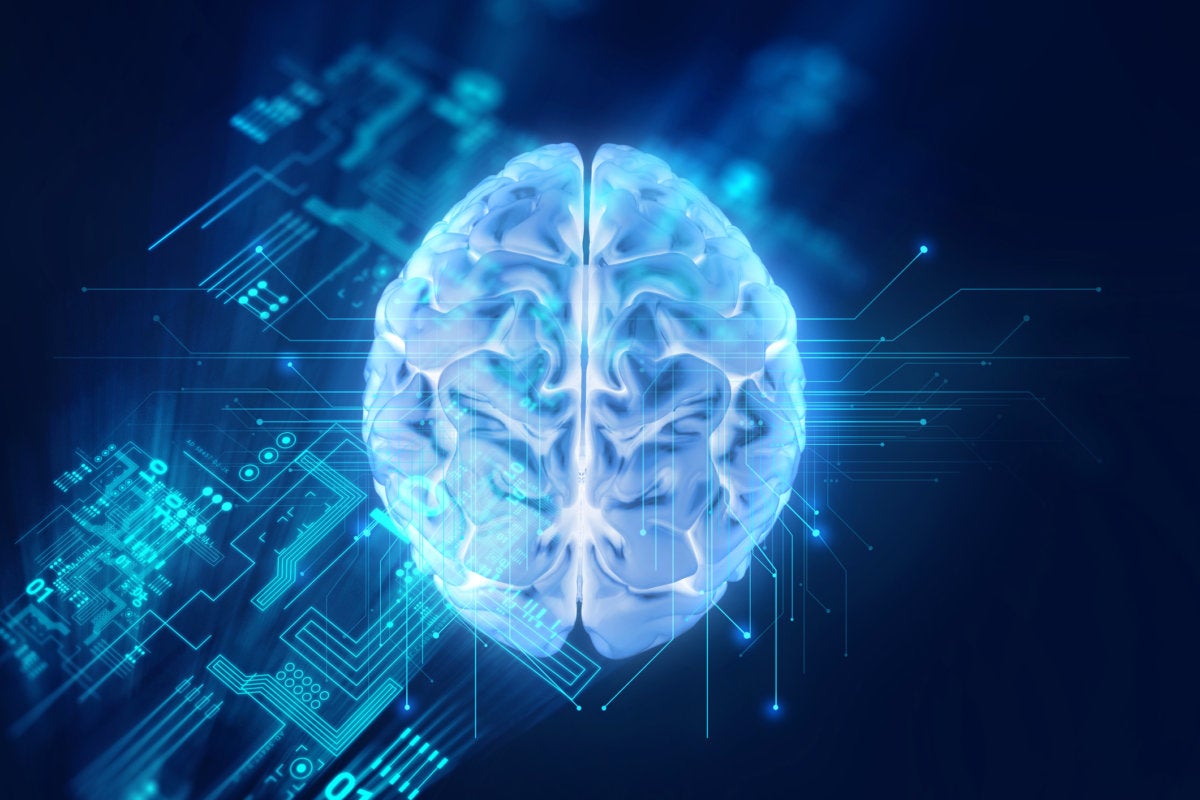
The challenge comes when we stop looking at collections as independent
elements and start looking at networks as collections of collections. A
network isn’t an anthill, it’s the whole ecosystem the anthill is inside of
including trees and cows and many other things. Trees know how to be trees,
cows understand the essence of cow-ness, but what understands the ecosystem?
A farm is a farm, not some arbitrary combination of trees, cows, and
anthills. The person who knows what a farm is supposed to be is the farmer,
not the elements of the farm or the supplier of those elements, and in your
network, dear network-operations type, that farmer is you. In the early
days, the developers of AI explicitly acknowledged the separation between
the knowledge engineer who built the AI framework and the subject-matter
expert whose knowledge shaped the framework. In software, especially DevOps,
the management tools aim to achieve a goal state, which in our farm analogy,
describes where cows, trees, and ants fit in. If the current state isn’t the
goal state, they do stuff or move stuff around to converge on the goal. It’s
a great concept, but for it to work we have to know what the goal
is.
Milvus 2.0: Redefining Vector Database
The microservice design of Milvus 2.0, which features read and write
separation, incremental and historical data separation, and CPU-intensive,
memory-intensive, and IO-intensive task separation. Microservices help
optimize the allocation of resources for the ever-changing heterogeneous
workload. In Milvus 2.0, the log broker serves as the system's backbone: All
data insert and update operations must go through the log broker, and worker
nodes execute CRUD operations by subscribing to and consuming logs. This
design reduces system complexity by moving core functions such as data
persistence and flashback down to the storage layer, and log pub-sub make
the system even more flexible and better positioned for future scaling.
Milvus 2.0 implements the unified Lambda architecture, which integrates the
processing of the incremental and historical data. Compared with the Kappa
architecture, Milvus 2.0 introduces log backfill, which stores log snapshots
and indexes in the object storage to improve failure recovery efficiency and
query performance.
Quote for the day:
"Expression is saying what you wish
to say, Impression is saying what others wish to listen." --
Krishna Sagar
No comments:
Post a Comment