Just how big a deal is Google’s new Meena chatbot model?

Meena can chat, over a few turns of a conversation, believably. Meena, however, cannot reliably teach you anything. Meena is not trying to help you finish a task or learn something new specifically. It converses with no explicit goal or purpose. While we probably spend too much of our time chatting about not much of importance, we tend to be looking for something specific when interacting with a bot-powered digital service. We want to get a ticket booked or a customer support issue resolved. Or we want to get accurate information about a particular domain or emotional or psychological support for a challenge we are facing. Conversational products have a purpose, and even if they fail at the more open-ended questions, they are trying to work with you to complete a task. Meena places the human-likeness of the conversation above all. However, there is much for us to learn about what is an appropriate conversational approach given different types of tasks. There is research that shows that more “robot” like responses are preferable in certain situations (especially where sensitive personal information is involved) and that being human-like is not the end-all and be-all of bots. Where does Meena, with the conversations it has learned from social media interactions, find a role?
Environmental IoT is one area they say could benefit. In smart cities, for example, bacteria could be programmed to sense for pollutants. Microbes have good chemical-sensing functions and could turn out to work better than electronic sensors. In fact, the authors say that microbes share some of the same sensing, actuating, communicating and processing abilities that the computerized IoT has. In the case of sensing and actuating, bacteria can detect chemicals, electromagnetic fields, light, mechanical stress and temperature — just what’s required in a traditional printed circuit board-based sensor. Plus, the microbes respond. They can produce colored proteins, for example. And not only that, they respond in a more nuanced way compared to the chip-based sensors. They can be more sensitive, as one example. ... Bacteria should become a “substrate to build a biological version of the Internet of Things,” the scientists say. Interestingly, similar to how traditional IoT has been propelled forward by tech hobbyists mucking around with Arduino microcontrollers and Raspberry Pi educational mini-computers, Kim and Posland reckon it will be do-it-yourself biology that will kick-start IoBNT.
AI still doesn’t have the common sense to understand human language

The test was originally designed with the idea that such problems couldn’t be answered without a deeper grasp of semantics. State-of-the-art deep-learning models can now reach around 90% accuracy, so it would seem that NLP has gotten closer to its goal. But in their paper, which will receive the Outstanding Paper Award at next month’s AAAI conference, the researchers challenge the effectiveness of the benchmark and, thus, the level of progress that the field has actually made. They created a significantly larger data set, dubbed WinoGrande, with 44,000 of the same types of problems. To do so, they designed a crowdsourcing scheme to quickly create and validate new sentence pairs. (Part of the reason the Winograd data set is so small is that it was hand-crafted by experts.) Workers on Amazon Mechanical Turk created new sentences with required words selected through a randomization procedure. Each sentence pair was then given to three additional workers and kept only if it met three criteria: at least two workers selected the correct answers, all three deemed the options unambiguous, and the pronoun’s references couldn’t be deduced through simple word associations.
A new bill could punish web platforms for using end-to-end encryption
/cdn.vox-cdn.com/uploads/chorus_image/image/66227166/acastro_180406_1777_facebook_Congress_0002.0.jpg)
The bill doesn’t lay out specific rules. But the committee — which would be chaired by the Attorney General — is likely to limit how companies encrypt users’ data. Large web companies have moved toward end-to-end encryption (which keeps data encrypted for anyone outside a conversation, including the companies themselves) in recent years. Facebook has added end-to-end encryption to apps like Messenger and Whatsapp, for example, and it’s reportedly pushing it for other services as well. US Attorney General William Barr has condemned the move, saying it would prevent law enforcement from finding criminals, but Facebook isn’t required to comply. Under the EARN IT Act, though, a committee could require Facebook and other companies to add a backdoor for law enforcement. Riana Pfefferkorn, a member of the Stanford Law School’s Center for Internet and Society, wrote a detailed critique of the draft. She points out that the committee would have little oversight, and the Attorney General could also unilaterally modify the rules. The Justice Department has pushed encryption backdoors for years, citing threats like terrorism, but they haven’t gotten legal traction. Now, encryption opponents are riding the coattails of the backlash against big tech platforms and fears about child exploitation online.
Technologies of the future, but where are AI and ML headed to?
The fluid nature of data science allows people from multiple fields of expertise to come and crack it. Shantanu believes if JRR Tolkien, being the brilliant linguist that he was, pursued data science to develop NLP models, he would have been the greatest NLP expert ever, and that is the kind of liberty and scope data science offers. ... For a country like India, acquiring new skills is not something of a luxury but a necessary requirement, and the trends of upskilling and reskilling are also currently on the rise to complement with the same. But data science, machine learning, and artificial intelligence are those fields where mere book-reading and formulaic interpretation and execution just does not cut it. If one aspires to have a competitive career in futuristic technologies, machine learning and data science have a larger spectrum of required understanding of probability, statistics, and mathematics on a fundamental level. To break the myths around programmers and software developers entering this market, machine learning involves understanding of basic programming languages (Python, SQL, R), linear algebra and calculus, as well as inferential and descriptive statistics.
Why Cybersecurity Training Needs a Serious Refresh

It’s easy to understand that if the technology market moves very fast, the security segments of it move even faster. This is the very definition of a dynamic environment—new dangers appear on the threat matrix every day, which means the ground is always shifting. It’s also easy to see how good security technology meets this challenge by constantly updating itself to combat new incoming threats. But here’s where it gets murky: Can we as individuals keep pace with the threats? And if we can’t, can even the most sophisticated tools ward off all dangers? No, we can’t, and that’s a big reason why the bad guys are usually ahead. Think of it as the human factor. The tools keep getting better, but inside this swirling vortex of innovation and sophistication, we as people—consumers, business professionals, and security specialists—have to scramble to understand new dangers and newer defenses. Even for tech teams dedicated to protecting the network, it’s a constant nightmare. For the rest of us, the reality is that while the threat matrix changes by the hour, IT security sessions take place maybe a few times a year, and it’s hard to even fit those into a busy schedule.
10 Key Challenges for Fintech Startups Worth Your Attention

The financial services industry is arguably the most regulated in the world. Laws are enacted to safeguard financial systems from abuse. The emergence of fintech has changed the way we view and handle money, creating a grey area for regulation. This issue has drawn the attention of regulators and lawmakers. Therefore, fintech startups have to contend with different regulatory hiccups on day to day basis because of their unstructured operating models. Besides, regulations on fintech operations vary from one jurisdiction to the other. Therefore, startups should fully understand the legal complications before operating in a particular country. While Fintech has brought much disruption in the financial industry, banks will not just sit pretty and watch as they lose their market share. Also, fintech ventures don’t only compete with existing financial powerhouses, such as PayPal. They soon will have to contend with new players, such as Amazon and other technology behemoths foraying into financial services. Due to their strong asset base, banks wield clout and can either buy out fintech companies or partner with them. As a venture, you should decide if you want to confront the big guys head-on or if you should instead explore greener pastures.
Why we’re failing to regulate the most powerful tech we’ve ever faced
Given the force of this technology, shouldn’t governments be bracing for its effect with robust regulations? The U.S. government so far is taking a mostly hands-off approach. U.S. Chief Technology Officer Michael Kratsios warned federal agencies against over-regulating companies developing artificial intelligence. There are views, too, that the U.S. government doesn’t want to issue meaningful regulation, that the administration finds regulation antithetical to its core beliefs. There is greater movement underway by the European Union (EU), which will issue a paper in February proposing new AI regulations for “high-risk sectors,” such as healthcare and transport. These rules could inhibit AI innovation in the EU, but officials say they want to harmonize and streamline rules in the region. China is pursuing a different strategy designed to tilt the playing field to its advantage as exemplified by its standards efforts for facial recognition. Ultimately, it is in the worldwide public interest for the AI superpowers, the U.S. and China, to collaborate on common AI principles.
Great Powers Must Talk to Each Other About AI
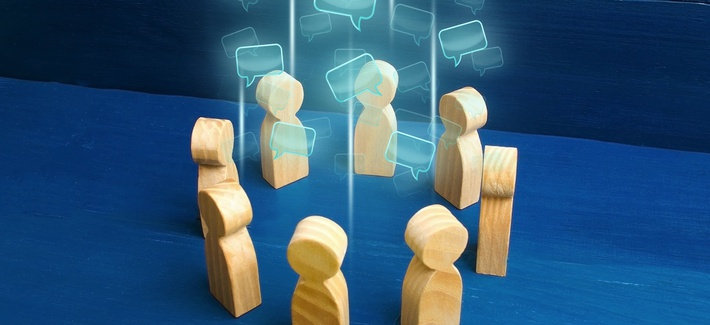
While the dynamics of artificial intelligence and machine learning, or ML, research remain open and often collaborative, the military potential of AI has intensified competition among great powers. In particular, Chinese, Russian and American leaders hail AI as a strategic technology critical to future national competitiveness. The military applications of artificial intelligence have generated exuberant expectations, including predictions that the advent of AI could disrupt the military balance and even change the very nature of warfare. At times, the enthusiasm of military and political leaders appears to have outpaced their awareness of the potential risks and security concerns that could arise with the deployment of such nascent, relatively unproven technologies. In the quest to achieve comparative advantage, military powers could rush to deploy AI/ML-enabled systems that are unsafe, untested or unreliable. As American strategy reorients toward strategic competition, critical considerations of surety, security and reliability around AI/ML applications should not be cast aside.
Burn, drown, or smash your phone: Forensics can extract data anyway

JTAG stands for Joint Task Action Group, the industry association that formed to create a standard for the manufacturing of Integrated Circuits. The NIST study only included Android devices because most Android devices are "J-taggable," while iOS devices aren't. The forensic technique takes advantage of taps, short for test access ports, which are usually used by manufacturers to test their circuit boards. By soldering wires onto taps, investigators can access the data from the chips. To perform a JTAG extraction, Reyes-Rodriguez first broke the phone down to access the printed circuit board (PCB). She carefully soldered thin wires the size of a human hair onto small metal components called taps, which are about the size of a tip of a thumbtack. "JTAG is very tedious and you do need a lot of training," says Ayers. "You need to have good eyes and very steady hand." The researchers compared JTAG to the chip-off method, which is another forensic technique. While JTAG work was done at NIST, the chip-off extraction was conducted by the Fort Worth Police Department Digital Forensics Lab and a private forensics company in Colorado called VTO Labs.
Quote for the day:
"A leadership disposition guides you to take the path of most resistance and turn it into the path of least resistance." -- Dov Seidman
No comments:
Post a Comment