The role of CDOs in building trust
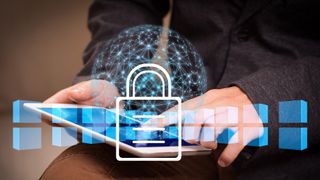
Data is fundamental to organisations. But at a time when companies have access to more data about their customers than ever before, an important characteristic of ethical, trustworthy organisations is how responsibly they manage that data. Perceptive business leaders understand they don’t own that data — the customer does. With that in mind, they know they must work to win trust by demonstrably acting as good custodians of customer data, keeping it safe and using it only for permitted purposes. With consumer sentiment in parallel with the demands of new and emerging data privacy laws, data governance and privacy are foundational to build and preserve customer trust and enhance customer experience and engagement. Regardless of industry, the work invested in response to the GDPR helps to build trust with customers. That could, in turn, lead to better all-round customer experiences. Much of the work to meet the requirements for GDPR compliance, required businesses to have a joined-up view of an individual’s personal data across multiple internal systems and cloud databases, with many initially focusing on customers.
Artificial Neural Networks For Blockchain: A Primer
The introduction of artificial intelligence, RNNs and especially LSTMs has enabled complex time-series forecasting, which is the sector of machine learning focused on predicting parameters in the future by referencing parameters from the past. Using data on bitcoin’s (or any cryptocurrency, for that matter) previous price points, RNNs can be trained in order to estimate its future price. This enables players in the retail industry to account for future price increases/decreases, possibly facilitating the transition to the implementation of digital currencies. It's important for technology professionals to learn as much as they can about the future of AI and neural networks in order to stay ahead of the curve. There are many great resources that can help you with this, including blogs such as Learn Neural Networks and videos from GoogleTechTalks and Geoffrey E. Hinton. Take a look around the web, and get invested in the future -- it will behoove you in more ways than you know.
What Are Plug & Platy Launguage Models
The PPLM models have three main phases. Firstly, a forward pass which is performed through the language model to compute the likelihood of the desired attribute using an attribute model that predicts probability. Secondly, by a backward pass that updates the internal latent representations using gradients from the attribute model. And, thirdly, a new distribution over the vocabulary is generated from the updated latent. This process of updating the latent is repeated at each time-step until it leads to a gradual transition towards the desired attribute. To validate the approaches of PPLM models, the researchers at Caltech and Uber AI, used both automatic and human annotators. For instance, perplexity is an automated measure of fluency, though its effectiveness has been questioned in open-domain text generation. Perplexity was then measured using the infamous pre-trained GPT model. In case of human annotation, annotators were asked to evaluate the fluency of each individual sample on a scale of 1-5, with 1 being “not fluent at all” and 5 being “very fluent”.
How to Effectively Employ an AI Strategy in your Business

Community plays a vital role in driving a change in any company. There are ways to connect with the community both online (webinars) as well as offline (meetups). Organizing meetups, webinars and training sessions enable one to exchange knowledge and learn from others. Learning from others, participating in sessions and sharing relevant knowledge is a great way to connect to the community. It doesn’t matter where you are. There are machine learning communities all around the world, and there may be a local chapter just next to your place. Another important reason for connecting to the community is that most of the data scientists and researchers today want to collaborate with others. The technologies in AI space are advancing at a rapid pace, and by connecting, people can ask all the right questions, share with others, participate with them and learn from everyone. Needless to say, in the last ten years, most of the cutting-edge research has come from the academic community and the open source community.
Why the quantum internet should be built in space

The problem is that entanglement is fragile and hard to preserve. Any small interaction between one of the photons and its environment breaks the link. Indeed, this is exactly what happens when physicists transmit entangled photons directly through the atmosphere or through optical fibers. The photons interact with other atoms in the atmosphere or the glass, and the entanglement is destroyed. It turns out the maximum distance over which entanglement can be shared in this way is just a few hundred kilometers. How then to build a quantum internet that shares entanglement across the globe? One option is to use “quantum repeaters”—devices that measure the quantum properties of photons as they arrive and then transfer these properties to new photons that are sent on their way. This preserves entanglement, allowing it to hop from one repeater to the next. However, this technology is highly experimental and several years from commercial exploitation. So another option is to create the entangled pairs of photons in space and broadcast them to two different base stations on the ground.
Top minds in machine learning predict where AI is going in 2020
In AI, the phrase “black box” has been around for years now. It’s used to critique neural networks’ lack of explainability, but Kidd believes 2020 may spell the end of the perception that neural networks are uninterpretable. “The black box argument is bogus … brains are also black boxes, and we’ve made a lot of progress in understanding how brains work,” she said. In demystifying this perception of neural networks, Kidd looks to the work of people like Aude Oliva, executive director of the MIT-IBM Watson AI Lab. “We were talking about this, and I said something about the system being a black box, and she chastised me reasonably [saying] that of course they’re not a black box. Of course you can dissect them and take them apart and see how they work and run experiments on them, the same [as] we do for understanding cognition,” Kidd said. Last month, Kidd delivered the opening keynote address at the Neural Information Processing Systems (NeurIPS) conference, the largest annual AI research conference in the world. Her talk focused on how human brains hold onto stubborn beliefs, attention systems, and Bayesian statistics.
Artificial Intelligence will be useful where Intelligence is!
To better understand what it is exactly that we are talking about, we will use the definitions but also the differentiations that Max Tegmark provides in popular Life 3.0. As third level life he describes the one that has the ability to design its own hardware and software (technological stage). Contrary to us, humans, Life 2.0, who modify our hardware through evolution but design most part of our software (cultural stage). Life 1.0 is life that modifies its hardware and software only through evolution (biological stage), meaning primitive organisms. That’s the stage we examine, the third stage defined as Artificial General Intelligence, meaning the ability of a system to successfully carry out any cognitive labor at least equally as good as a human would do. According to Tegmark, technosceptics believe we are still far from approaching that skill, unlike technology polemicists, digital utopians and the movement of beneficial AI who, despite their differences, think we are close to achieving that goal. As we mentioned during the introduction, Microsoft’s research neither projects nor predicts.
Deepwave: A Recurrent Neural Network For Real Time Acoustic Imaging
Though CNNs enjoy the status of being one of the most widely used architectures across many machine learning applications, they falter in the presence of more complex image reconstruction problems where the input data may not consist of an image, as is the case in biomedical imagery, interferometry, or acoustic imaging. Moreover, the authors have also observed that the standard convolutional architectures cannot handle images with non-Euclidean domains such as spherical maps produced by omnidirectional acoustic cameras. And, this is where recurrent networks have proven to be useful. A cascade of recurrent layers with trainable parameters — a variant of RNN that was proposed by Yann Lecun and his peers, was good at learning shortcuts in the reconstruction space, allowing it to achieve a prescribed reconstruction accuracy faster than gradient-based iterative methods. With techniques like pruning, the recurrent networks got even smaller with fewer parameters.
Three Considerations For Realizing Real Digital Transformation
Experience as the true north is one of the most powerful drivers of digital innovation. The science behind experience as a compass, capability and organizational muscle is well laid out. We go from problem definition to journey mapping to future state definition to tech landscaping to component architecture to building the new experience, allowing us to go from piecemeal automation to full transformation. ... To innovate at scale, you need to build the right talent, namely "bilinguals." A bilingual is neither the most evolved machine learning engineer nor the highest performing supply chain planner. This is someone who understands enough of the two to realize the value at the intersection, such as financial traders who understand machine learning or assembly line operators who understand analytics and data science. These intersections involve cross-skilling employees across disciplines and promoting a culture of curiosity and change.
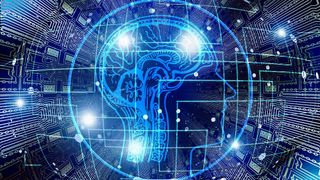
Concerns over AI range between how it could make jobs in almost every sector obsolete, to existential worries about the threat a super intelligent, self-learning machine could pose to humanity. For every stakeholder in artificial intelligence — from the programmer to the end user — it’s important to remain focused on using this powerful technology to support humans, not replace them. AI can be designed to empower people to share their skills and knowledge. If you think about an organisation or a community, the scope of human intelligence is vast. You may have hundreds of thousands of human brains, all with different perspectives, experiences and understanding. Unlocking this insight with AI could enhance people’s intelligence and fuel their careers. Teams can access answers and support when they need it (and pick up new skills of their own in the process). For business leaders, using AI has clear positive knock-on effects from boosted productivity and efficiency, to greater workplace happiness and employee retention.
Quote for the day:
"People who enjoy meetings should not be in charge of anything." -- Thomas Sowell
No comments:
Post a Comment