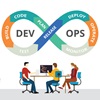
While building automation-ready test designs and ensuring system testability are key steps to achieving automated testing, businesses can take their automation to the next level by implementing continuous testing (CT). Continuous testing can lead to faster feedback, quicker release turnaround, and higher customer satisfaction and loyalty, giving businesses the best chance of not only surviving the future of software delivery but thriving in it. Because continuous testing is the most advanced form of testing, it is also the most challenging. ... Testers should also consider the test agents when provisioning test machines (on a PC, laptop, etc.) or virtual machines (in the cloud, containers, etc.). Once the machine is set, the dispatcher should efficiently distribute the tests, and any developer or tester should kick off the tests to run in parallel. This way, if there is more than one development or scrum team with different needs, they can run automated tests without waiting for another team to complete their run.
Businesses Improve Their Data Security, But Privacy — Not So Much
"Privacy is one of the major pieces of collateral damage that no one talks about in our reaction to September 11," he says. "It set us on a path to use data and the Internet as a tool to combat terrorism, and I understand why, rather than really moving forward on where the President's instincts were on putting the consumer first." For the past decade, companies have been focused on dodging online criminals — and then nation-state actors — intent on stealing data. With the passage of the GDPR, focusing on data security became a business imperative to avoid larger fines. Yet the policy discussion and legal landscape have become more nuanced, says Ackerly. Companies are beginning to understand that customers want privacy, he says. "I am optimistic as I've ever been on this journey that we will end up in a place where individuals will be able to take control over their data where ever it is shared," Ackerly says. "I think it is a combination of technology evolving and society just waking up to the trade-offs that we have made over the past 15 or 20 years."
SaaS transformation: Tax Automation partners with Pulsant to reduce costs and improve delivery

Initially VAT Controller was hosted on dedicated servers in one of Pulsant’s data centres, with failover to another of its data centres in the event of unplanned downtime. With the development of Pulsant Enterprise Cloud, a combination of private cloud and managed hosting, Tax Automation worked with Pulsant to migrate to this hosting model because it features the best hardware, robust cloud delivery and proven management software. In the next phase of the relationship, Tax Automation worked with the Pulsant team to find the best way to host its Capital Assets Database software and make it quicker and easier to access for clients, without hosting the infrastructure on its own site. The Capital Assets Database is a client-server application, which necessitated the need for a more robust hosting solution. The software is accessed through a user interface, in this case Citrix, and not just a web browser. As a result, Pulsant needed to ensure a smooth integration between the hosted server and user interface. Rainer and his team worked with Pulsant to devise the most appropriate solution to meet requirements.
Opinion: ‘Scale’ is the magic word for digital transformation in 2020
Smart manufacturing requires convergence between IT and OT data to drive visibility, collaboration, and efficiency within plants and facilities and across operations. However, two decades after automation networks on the plant floor became ubiquitous, it’s still generally true that information accessibility between plant floor devices (OT) – and the people and systems that can create new value from them (IT) – proves to be a significant challenge. To remove the complexity and domain expertise required to access plant floor devices and systems, manufacturers are turning to auto-discovery tools that identify assets, collect and integrate data with full OT context, and produce models fully shareable with IT systems. By connecting existing OT infrastructure to smart factory networks and IT initiatives, and continuously generating relevant data insights and measurements, auto-discovery capabilities reduce the technical knowledge and time needed by OT teams to map industrial infrastructure and improve operational efficiency.
There are two types of Unsupervised Learning: discriminative models and generative models. Discriminative models are only capable of telling you, if you give it X then the consequence is Y. Whereas the generative model can tell you the total probability that you’re going to see X and Y at the same time. So the difference is as follows: the discriminative model assigns labels to inputs, and has no predictive capability. If you gave it a different X that it has never seen before it can’t tell what the Y is going to be because it simply hasn’t learned that. With generative models, once you set it up and find the baseline you can give it any input and ask it for an answer. Thus, it has predictive ability – for example it can generate a possible network behavior that has never been seen before. So let’s say some person sends a 30 megabyte file at noon, what is the probability that he would do that? If you asked a discriminative model whether this is normal, it would check to see if the person had ever sent such a file at noon before… but only specifically at noon.
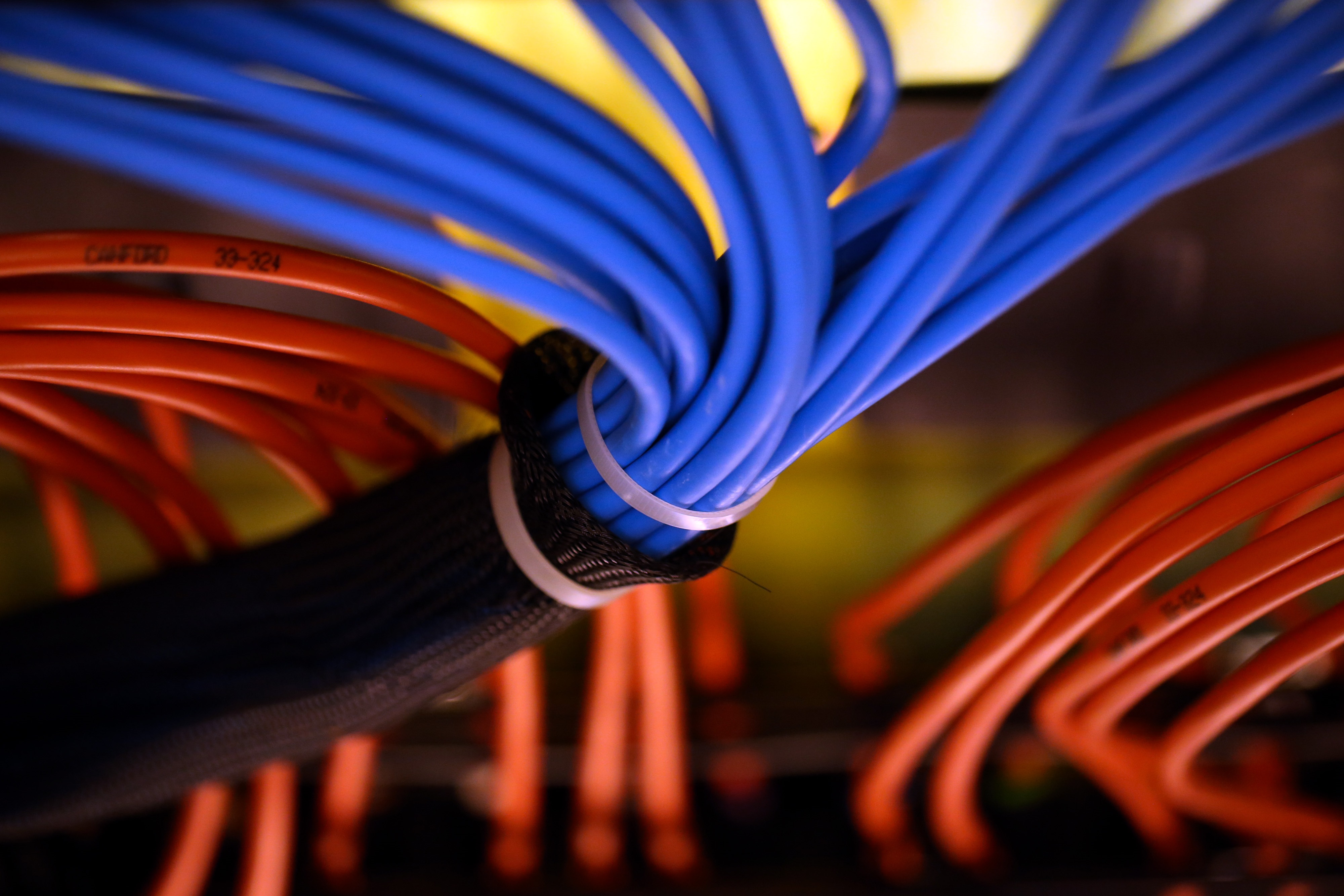
Having the right data at the right time and with the right level of confidence at the point of use is priceless, but all these unexpected, unannounced and unending changes to data, collectively termed data drift, is beyond our control and leads to operational risk. While still in its early days, I believe that in 2020, we will see more pervasive interest in DataOps. DataOps is the set of practices and technologies that brings the end-to-end automation and monitoring sensibilities of DevOps to data management and integration. But what makes it DataOps are drift-resilient smart data pipelines, from which living, breathing end-to-end data topologies emerge. Instead of ignoring or fighting data drift, DataOps embraces and harnesses it to speed up data analytics, with confidence. Some indicators that we’ve noticed here at StreamSets include a small, but burgeoning cross-section of customers that are embracing DataOps approaches. The recent DataOps Summit highlighted many of their use cases and resulting business impact. Searches for the term “DataOps” have tripled, vendors are entering the space with DataOps offerings, and we’re seeing a number of DataOps business titles appearing on LinkedIn profiles.
NHS suffers fewer ransomware attacks, but threat persists
Nevertheless, noted Bischoff, the decrease in attack volumes appears, at face value, to show that the money invested in security, coupled with the launch of NHSX, since the WannaCry attacks has had the desired effect, to some extent. Even so, the NHS spends only about 2% of its total budget on IT, compared with 4-10% in other sectors, according to Saira Ghafur, digital health lead at Imperial College London’s Institute for Global Health Innovation. So the health service still needs more funding to replace ageing infrastructure and secure both endpoint devices and connected healthcare equipment, she said. Speaking at a think-tank event on security in October 2019, Ghafur said the NHS faced other security challenges, particularly around skills. “We can’t compete with other sectors in terms of attracting cyber security professionals – we need to work with the industry to attract them into healthcare – and all NHS staff need better education in terms of risks,” she said.
Which cloud strategy is right for your business in 2020?

Hybrid cloud shouldn’t be an afterthought — it should instead be viewed as a fundamental design principle upon which vital infrastructure building blocks are built. We recommend beginning with a hybrid cloud infrastructure built from the ground up to ensure flexibility and choice. Companies need to consider developer enablement and productivity, allowing them to build and deploy apps to a hybrid cloud. After all, the needs of an app might change, from on premise today to public cloud tomorrow. In line with this, management tools that orchestrate workloads and automation tools to simplify day-to-day operations are essential to delivering the complete value of a hybrid cloud. Making a conscious decision to retain on-premise data centres and continuing to invest where it makes sense — for specific workloads — is often an important part of a hybrid cloud strategy. It’s not about having on-premise data centres, adopting a bit of public cloud, ending up with two and calling that hybrid cloud.
Implications of Using Artificial Intelligence in Film Studio Decision-Making
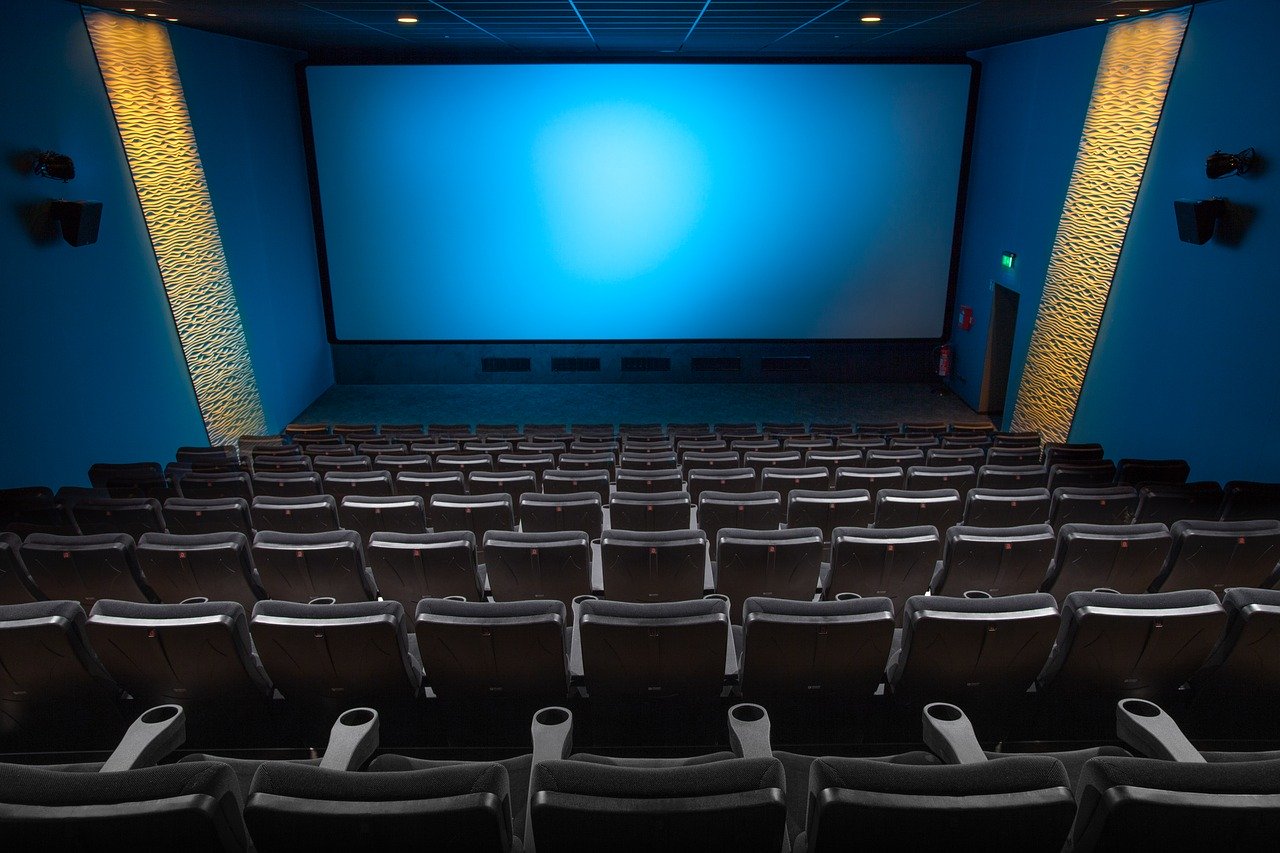
Predictive analytics could be run against a variety of factors concerning potential actors, screenwriters, and directors: such characteristics could include one’s gender, age, race, ethnicity, disability or impairment, sexual orientation, and so on. As we have seen from studies on machine learning in the criminal justice context, algorithms can perpetuate human biases. It is foreseeable that the AI could become path dependent, err on the side of caution, and fail to account for cultural shifts in audience attitudes. For instance, of the top 100 top grossing movies made between 2017 and 2018, only 33 per cent of all speaking or named characters were girls or women. If this metric were analysed in isolation, it is not impossible to consider that a machine learning algorithm would lean towards viewing male protagonists as a safer choice for higher profits. As Norwegian filmmaker Tonje Hessen Schei told Screen Daily, a concern with this new process is that it may become “harder and harder to get a diverse span of voices that will be heard in the market.” The legal implications or responses on this point are somewhat unclear.
Securing Containers with Zero Trust
From the perspective of a firewall, all it would see is a packet coming from that host, a machine it has been told to trust. It will allow that packet, which in turn allows attackers to exfiltrate data, encrypt the data, or use SQL itself to move further across the network toward their target. Now let's add a second container to the host. In a Docker classic environment, all the containers are network-address translated to look like the host, so it's impossible to determine where the traffic originated. In a bridging scenario, there are multiple ways to impersonate the Java microservice inside the container. And just as with other network plug-ins, the Linux machine serving as the host has a large network attack surface. ... If the purpose of a policy is to only allow this specific Java microservice to communicate with a SQL database, in a firewall model, this all has to be transformed into a long series of network addresses, which have to change on the fly as the network infrastructure itself changes.
Quote for the day:
"Give whatever you are doing and whoever you are with the gift of your attention." -- Jim Rohn
No comments:
Post a Comment