The Top 25 Security Predictions for 2025

“Malicious actors will go full throttle in mining the potential of AI in
making cyber crime easier, faster and deadlier. But this emerging and
ever-evolving technology can also be made to work for enterprise security and
protection by harnessing it for threat intelligence, asset profile management,
attack path prediction and remediation guidance. As SOCs catch up to secure
innovations still and yet unraveling, protecting enterprises from tried and
tested modes of attack remains essential. While innovation makes for novel
ways to strike, criminals will still utilize what is easy and what has worked
for them for years.” ... Organizations are urged to embrace scalable,
cloud-native security information and event management (SIEM) solutions. These
tools improve threat detection and response by integrating logs from cloud and
endpoint systems and automating incident management with security
orchestration, automation, and response (SOAR) features. ... While targets
like edge devices will continue to capture the attention of threat actors,
there’s another part of the attack surface that defenders must pay close
attention to over the next few years: their cloud environments. Although cloud
isn’t new, it’s increasingly piquing the interest of cyber criminals.
Why AI language models choke on too much text
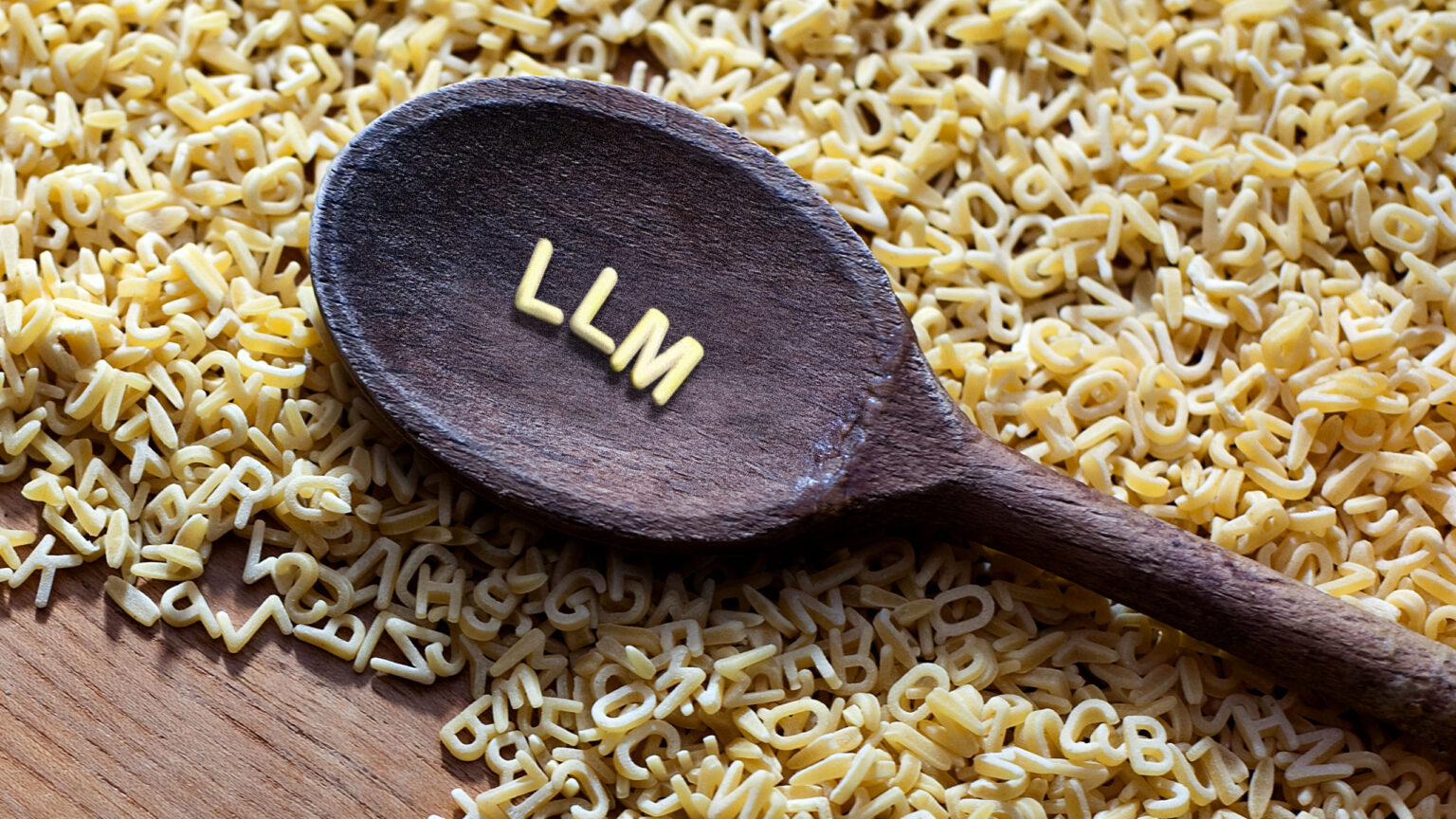
Although RNNs have fallen out of favor since the invention of the transformer,
people have continued trying to develop RNNs suitable for training on modern
GPUs. In April, Google announced a new model called Infini-attention. It’s
kind of a hybrid between a transformer and an RNN. Infini-attention handles
recent tokens like a normal transformer, remembering them and recalling them
using an attention mechanism. However, Infini-attention doesn’t try to
remember every token in a model’s context. Instead, it stores older tokens in
a “compressive memory” that works something like the hidden state of an RNN.
This data structure can perfectly store and recall a few tokens, but as the
number of tokens grows, its recall becomes lossier. ... Transformers are good
at information recall because they “remember” every token of their
context—this is also why they become less efficient as the context grows. In
contrast, Mamba tries to compress the context into a fixed-size state, which
necessarily means discarding some information from long contexts. The Nvidia
team found they got the best performance from a hybrid architecture that
interleaved 24 Mamba layers with four attention layers. This worked better
than either a pure transformer model or a pure Mamba model.
The End of ‘Apps,’ Brought to You by AI?
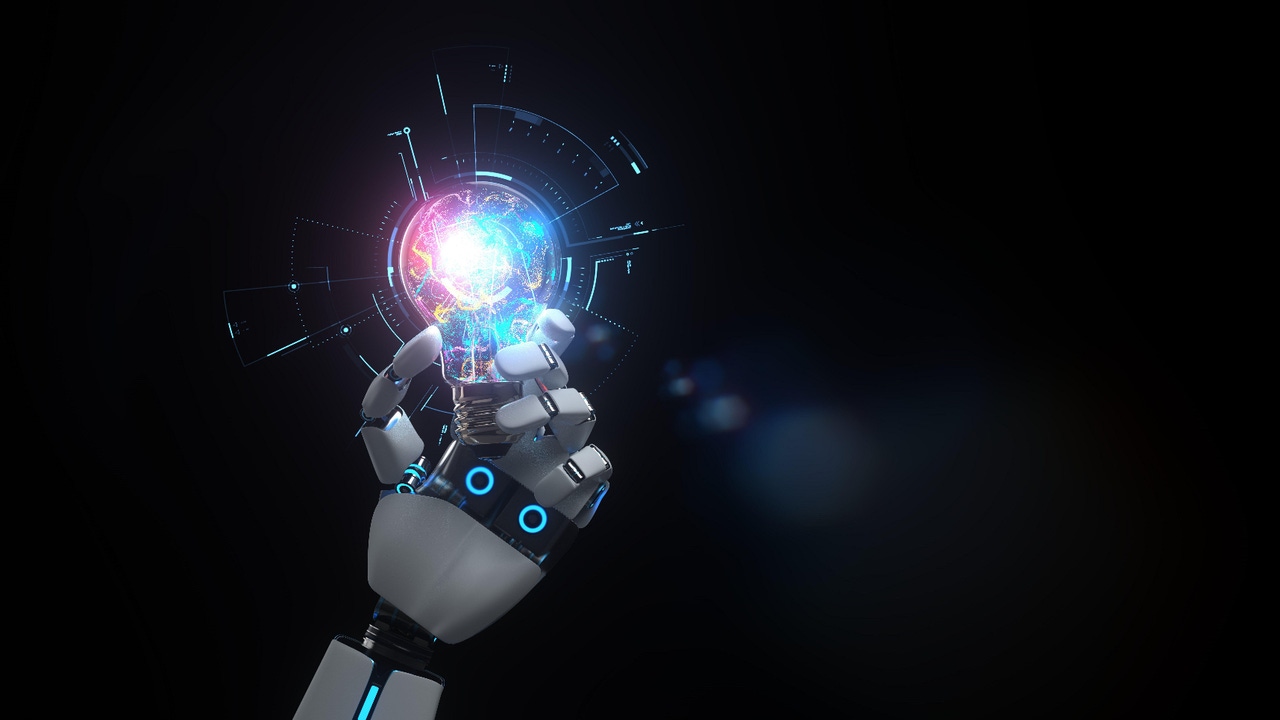
Achieving the dream of a unified customer experience is possible, not by
building a bigger app but by AI super agents. Much of the groundwork has
already been done: AI language models like Claude and GPT-4 are already
designed to support many use cases, and Agentic AI takes that concept further.
OpenAI, Google, Amazon, and Meta are all making general-purpose agents that
can be used by anyone for any purpose. In theory, we might eventually see a
vast network of specialized AI agents running in integration with each other.
These could even serve customers’ needs within the familiar interfaces they
already use. Crucially, personalization is the big selling point. It’s the
reason AI super agents may succeed where super apps failed in the West. A
super agent wouldn’t just aggregate services or fetch a gadget’s price when
prompted. It would compare prices across frequented platforms, apply
discounts, or suggest competing gadgets based on reviews you’ve left for
previous models. ... This new ‘super agents’ reality would yield significant
benefits for developers, too, possibly even redefining what it means to be a
developer. While lots of startups invent good ideas daily, the reality of the
software business is that you’re always limited by the number of developers
available.
A Starter’s Framework for an Automation Center of Excellence

An automation CoE is focused on breaking down enterprise silos and promoting
automation as a strategic investment imperative for achieving long-term value.
It helps to ensure that when teams want to create new initiatives, they don’t
duplicate previous efforts. There are various cost, efficiency and agility
benefits to setting up such an entity in the enterprise. ... Focus on projects
that deliver maximum impact with minimal effort. Use a clear, repeatable
process to assess ROI — think about time saved, revenue gained and risks
reduced versus the effort and complexity required. A simple question to ask
is, “Is this process ready for automation, and do we have the right tools to
make it work?” ... Your CoE needs a solid foundation. Select tools and systems
that integrate seamlessly with your organization’s architecture. It might seem
challenging at first, but the long-term cultural and technical benefits are
worth it. Ensure your technology supports scalability as automation efforts
grow. ... Standardize automation without stifling team autonomy. Striking this
balance is key. Consider appointing both a business leader and a technical
evangelist to champion the initiative and drive adoption across the
organization. Clear ownership and guidelines will keep teams aligned while
fostering innovation.
What is data architecture? A framework to manage data

The goal of data architecture is to translate business needs into data and
system requirements, and to manage data and its flow through the enterprise.
Many organizations today are looking to modernize their data architecture as a
foundation to fully leverage AI and enable digital transformation. Consulting
firm McKinsey Digital notes that many organizations fall short of their
digital and AI transformation goals due to process complexity rather than
technical complexity. ... While both data architecture and data modeling seek
to bridge the gap between business goals and technology, data architecture is
about the macro view that seeks to understand and support the relationships
between an organization’s functions, technology, and data types. Data modeling
takes a more focused view of specific systems or business cases. ... Modern
data architectures must be scalable to handle growing data volumes without
compromising performance. A scalable data architecture should be able to scale
up and to scale out. ... ... Modern data architectures must ensure data
remains accurate, consistent, and unaltered through its lifecycle to preserve
its reliability for analysis and decision-making. They must prevent issues
like data corruption, duplication, or loss.
Cybersecurity At the Crossroads: The Role Of Private Companies In Safeguarding U.S. Critical Infrastructure
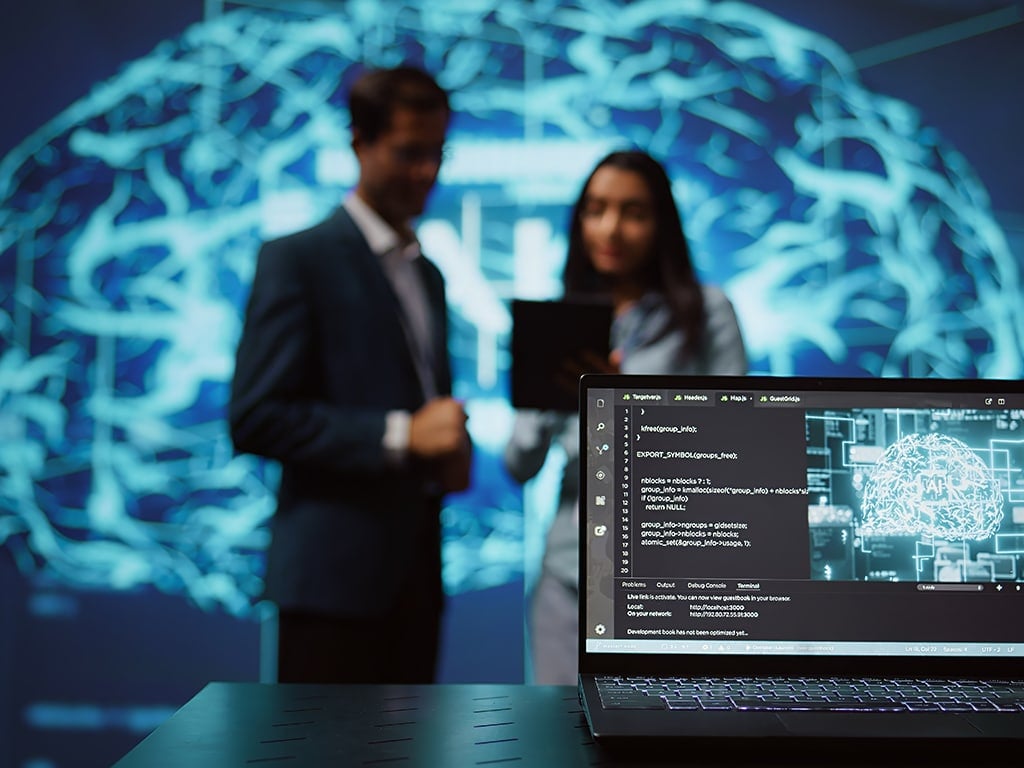
Regulation alone is not a solution, but it does establish baseline security
standards and provide much-needed funding to support defenses. Standards have
come a long way and are relatively mature. Though there is still a tremendous
amount of gray area, and a lack of relevance or attainability for certain
industries and smaller organizations. The federal government must prioritize
injecting funds into cybersecurity initiatives, ensuring that even the
smallest entities managing critical infrastructure can implement strong
security measures. With this funding, we must build a strong defense posture
and cyber resiliency within these private sector organizations. This involves
more than deploying advanced tools; it requires developing skilled personnel
capable of responding to incidents and defending against attacks. Upskilling
programs should focus on blue teaming and incident response, ensuring that
organizations have the expertise to manage their security proactively.A
critical component of effective cybersecurity is understanding and applying
the standard risk formula: Risk = Threat x Vulnerability x Consequence. This
formula emphasizes that risk is determined by evaluating the likelihood of an
attack (Threat), the weaknesses in defenses (Vulnerability), and the potential
impact of a breach (Consequence).
Achieving Network TCO
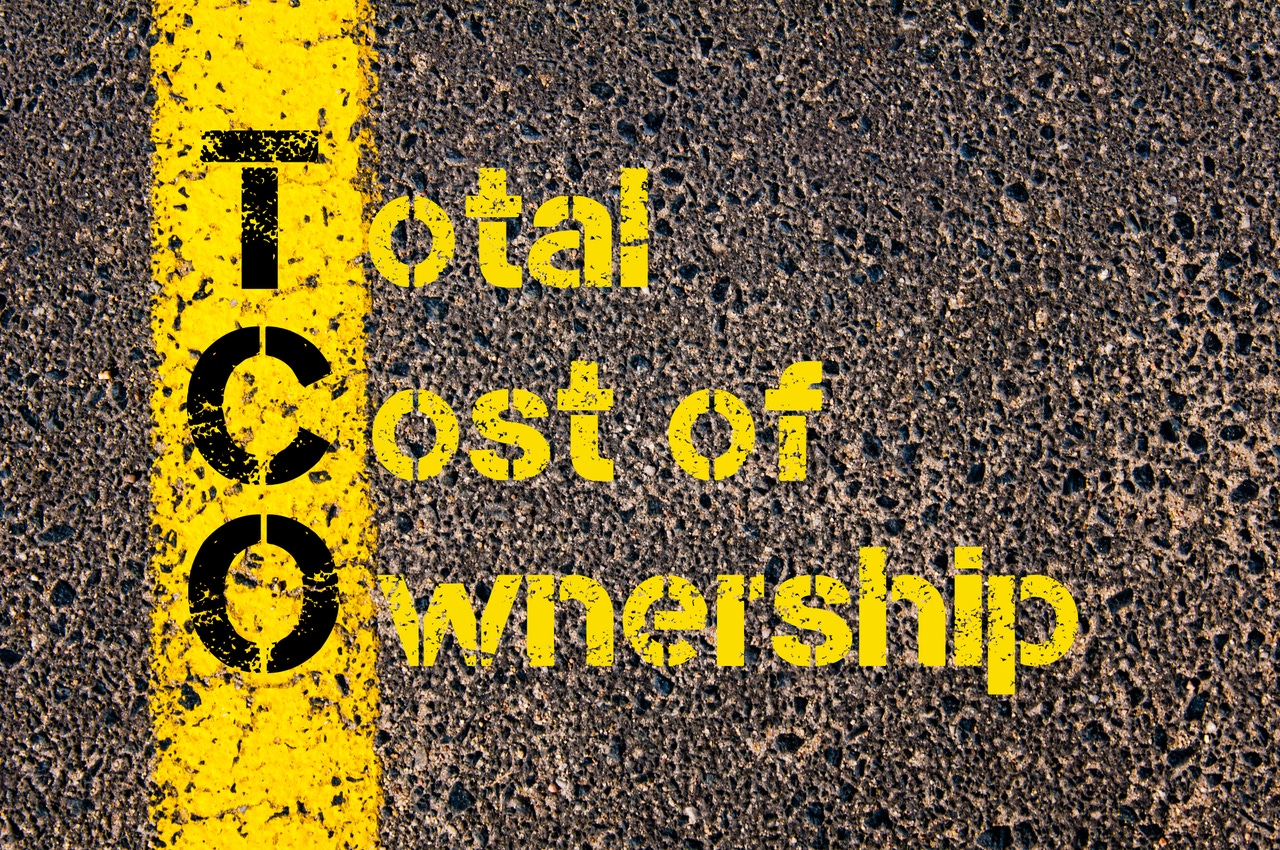
TCO discussion should shift from a unilateral cost justification (and payback)
of technology that is being proposed to a discussion of what the opportunity
costs for the business will be if a network infrastructure investment is
canceled or delayed. If a company determines strategically to decentralize
manufacturing and distribution but is also wary of adding headcount, it's
going to seek out edge computing and network automation. It’s also likely to
want robust security at its remote sites, which means investments in
zero-trust networks and observability software that can assure that the same
level of enterprise security is being applied at remote sites as it is at
central headquarters. In cases like this, it shouldn’t be the network manager
or even the CIO who is solely responsible for making the budget case for
network investments. Instead, the network technology investments should be
packaged together in the total remote business recommendation and investment
that other C-level executives argue for with the CIO and/or network manager,
HR, and others. In this scenario, the TCO of a network technology investment
is weighed against the cost of not doing it at all and missing a corporate
opportunity to decentralize operations, which can’t be accomplished without
the technology that is needed to run it.
The coming hardware revolution: How to address AI’s insatiable demands
The US forecast for energy consumption on AI is alarming. Today’s AI queries
require roughly 10x the electricity of traditional Google queries - a ChatGPT
request runs 10x watt-hours versus a Google request. A typical CPU in a data
center uses approximately 300 watts per hour (Electric Power Research
Institute), while a Nvidia H100 GPU uses up to 700 watts per hour, a similar
usage of an average household in the US per month. Advancements in AI model
capabilities, and greater use of parameters, continue to drive energy
consumption higher. Much of this demand is centralized in data centers as
companies like Amazon, Microsoft, Google, and Meta build more and more massive
hyperscale facilities all over the country. US data center electricity
consumption is projected to grow 125 percent by 2030, using nine percent of
all national electricity. ... While big tech companies certainly have the
benefit of incumbency and funding advantage, the startup ecosystem will play
an absolutely crucial role in driving the innovation necessary to enable the
future of AI. Large public tech companies often have difficulty innovating at
the same speed as smaller, more nimble startups.
Agents are the 'third wave' of the AI revolution

"Agentic AI will be the next wave of unlocked value at scale," Sesh Iyer,
managing director and senior partner with BCG X, Boston Consulting Group's
tech build and design unit, told ZDNET. ... As with both analytical and gen
AI, AI agents need to be built with and run along clear ethical and
operational guidelines. This includes testing to minimize errors and a
governance structure. As is the case with all AI instances, due diligence to
ensure compliance and fairness is also a necessity for agents, Iyer said. As
is also the case with broader AI, the right skills are needed to design, build
and manage AI agents, he continued. Such talent is likely already available
within many organizations, with the domain knowledge needed, he added.
"Upskill your workforce to manage and use agentic AI effectively. Developing
internal expertise will be key to capturing long-term value from these
systems." ... To prepare for the shift from gen AI to agentic AI, "start small
and scale strategically," he advises. "Identify a few high-impact use cases --
such as customer service -- and run pilot programs to test and refine agent
capabilities. Alongside these use cases, understand the emerging platforms and
software components that offer support for agentic AI."
Having it both ways – bringing the cloud to on-premises data storage
“StaaS is an increasingly popular choice for organisations, with demand only
likely to grow soon. The simple reason for this is two-fold: it provides both
convenience and simplicity,” said Anthony Cusimano, Director of Technical
Marketing at Object First, a supplier of immutable backup storage appliances.
There is more than one flavour of on-premises StaaS, as was pointed out by A3
Communications panel member Camberley Bates, Chief Technology Advisor at IT
research and advisory firm The Futurum Group. Bates pointed out that the two
general categories of on-premises StaaS service are Managed and Non-Managed
StaaS. Managed StaaS sees vendors handling the whole storage stack, by both
implementing and then fully managing storage systems on customers’ premises.
However, Bates said enterprises are more attracted to Non-Managed StaaS. ...
“Non-managed StaaS has become surprisingly of interest in the market. This is
because enterprises buy it ‘once’ and do not have to go back for a capex
request over and over again. Rather, it becomes a monthly bill that they can
true-up over time. We have found the fully managed offering of less interest,
with enterprises opting to use their own resources to handle the storage
management,” continued Bates.
Quote for the day:
“If you don’t try at anything, you
can’t fail… it takes back bone to lead the life you want” --
Richard Yates
No comments:
Post a Comment